Reactivation strength during cued recall is modulated by graph distance within cognitive maps
Figures
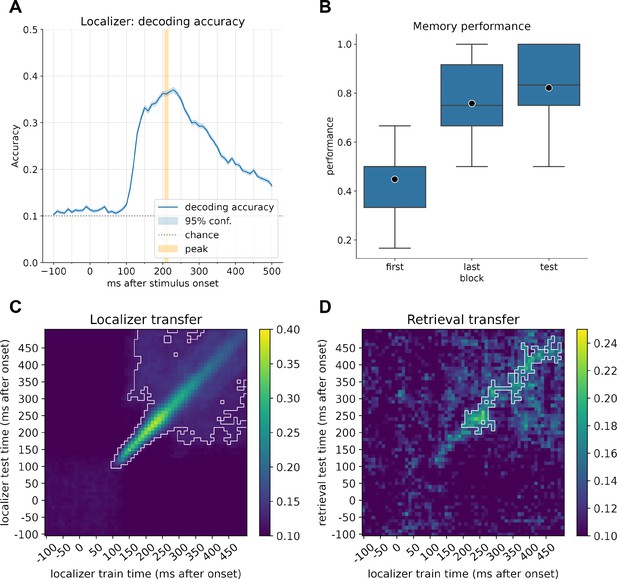
Decoder accuracy and learning performance.
(A) Decoding accuracy of the currently displayed item during the localizer task for participants with a decoding accuracy higher than 30% (n = 21). The mean peak time point across all participants corresponded to 210 ms, with an average decoding peak decoding accuracy of 42% (n = 21). Note that the displayed graph combines accuracies across participants, where peak values were computed on an individual level and then averaged. Therefore, the indicated individual mean peak does not match the average at a group level. (B) Memory performance of participants after completing the first block of learning, the last block (blocks 2–6, depending on the speed of learning), and the retrieval performance. (C) Classifier transfer within the localizer when trained and tested at different time points determined by cross-validation. (D) Classifier transfer from the localizer session to the retrieval session when trained at different time points during training and tested at different time points during cue presentation of the first (predecessor) image cue during retrieval. For (B) and (C), within the white outline, classification was significantly above chance level (cluster permutation testing, α < 0.05).
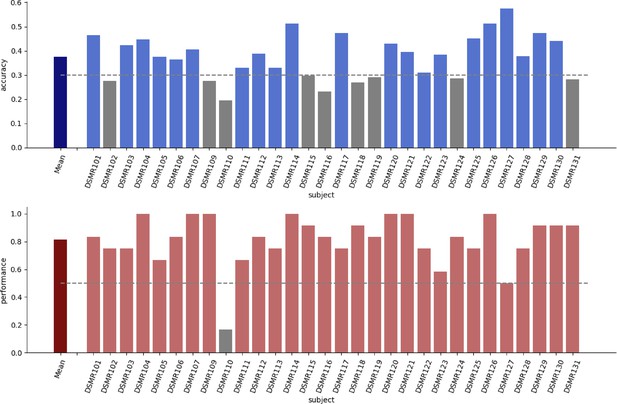
Excluded participants based on decoding accuracy and memory performance during retrieval.
Peak decoding accuracy was determined by a leave-one-per-class-our cross-validation across time for each participant. Memory performance was the percentage of correct responses during the 12 retrieval trials.
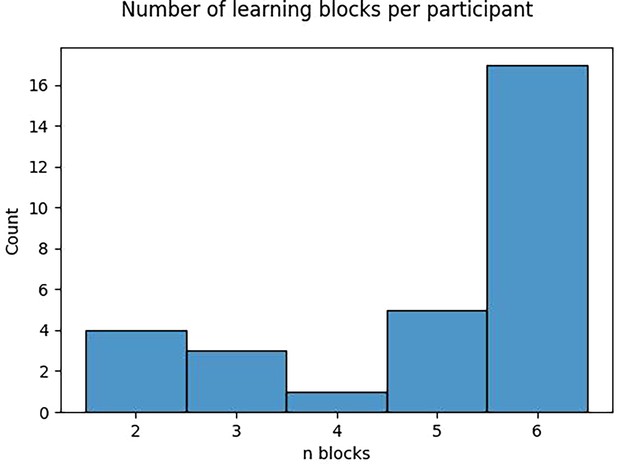
Number of learning blocks that each participant completed.
The number of learning blocks was adapted to the speed of the participant such that each participant had a similar performance at their last block. Learning was stopped if participants reached at least 80% memory performance in a block or if they reached six blocks. A minimum of two blocks are shown, even if participants reached above 80% in their first block (by chance).
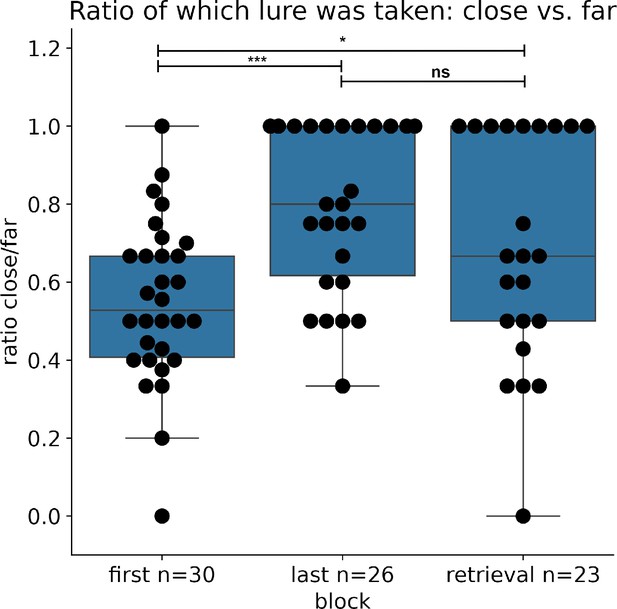
During the learning and retrieval blocks, participants were presented two lures next to the correct answer to complete the triplet, one of which was closer to the target and one further away on the graph.
To show that participants indeed learned the graph structure and not just triplets, the figure shows the ratio of close lures chosen vs lures that were further away on the graph. In the first learning block, the chosen lure is random as participants have not learned the graph structure yet. On the last learning block, many participants exclusively choose the closer lure, indicating that they are aware of approximate distances of the presented stimuli. Note, however, that the analysis relies solely on trials with incorrect responses. Therefore, the apparent (nonsignificant) drop of the ratio from the last block to the retrieval block can be attributed to participants reaching ceiling performance. Additionally, the number of blocks was determined by the learning speed of the participant (with a minimum of two learning blocks), making it hard to compare between participants with different numbers of learning blocks. Therefore, we have decided to plot the first, last, and retrieval blocks as they were defined for each participant. An ANOVA indicated that the three blocks were significantly different (F = 7.5, p=0.001), a post hoc t-test indicated a significant difference between the first and last (t = −4.3, p<0.0001) and the first and retrieval session (t = −2.0, p=0.046) and no difference between the last and retrieval block (t = 1.4, p=0.16).
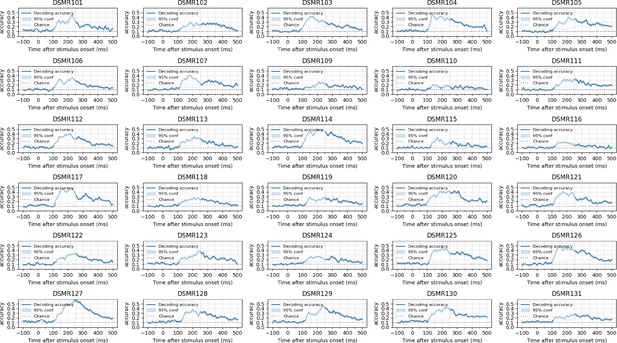
Decoding accuracy across time determined by a leave-one-per-class-out cross-validation per participant.
For details on decoder training, see ‘Methods’.
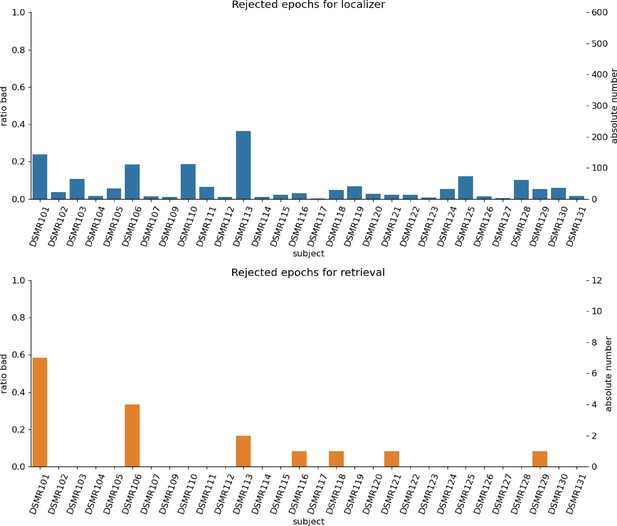
Percentage of rejected trials for each participant.
Artifacts were detected automatically using AutoReject. If possible, channels were interpolated for the affected time span, else the trial was rejected. The figure displays the ratios as well as the absolute number of rejected epochs for each participant in the study. The analysis is based on the remaining nonrejected epochs. For the retrieval, on average, 11.5 epochs were available, in total 252 across the study.
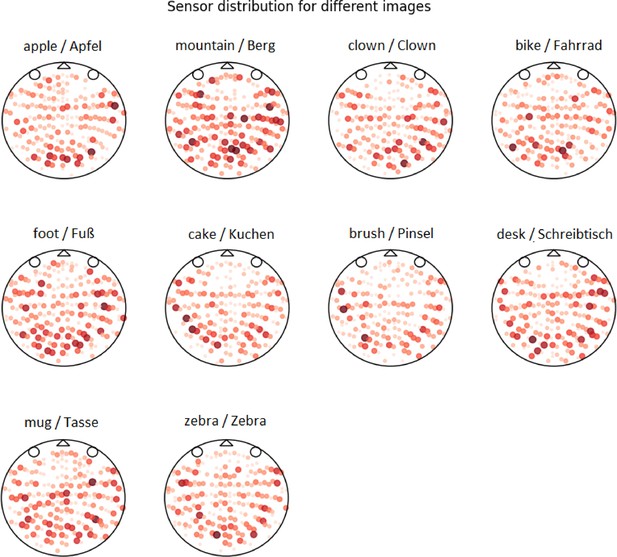
Percentage of sensors relevant for each image across all participants (beta weight of sensor location unequal to zero).
Larger/darker dots indicate more participants' decoders' used information from this sensor. LASSO/L1 regularization forces individual regressor values of the classifier belonging to a specific sensor to 0, such that only a sparse number of sensors contribute information to the decision process. The plot shows the average ratio that a sensor was included across participants, giving a rough estimate for the location of stimulus processing. The largest dot indicates that this sensor was used for all participants for this image. The smallest/lightest dot indicates that almost no participant’s decoder used information from this sensor. Please note that the magnetoencephalographic (MEG) head positioning was not aligned between participants such that the average dots do not indicate a specific location but only a broad region.
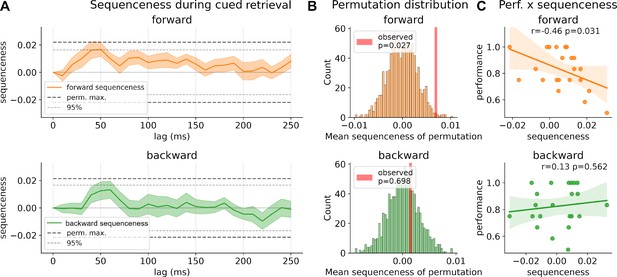
Sequenceness during retrieval.
(A) Strength of forward and backward sequenceness across different time lags up to 250 ms during the 1500 ms window after cue onset. Two significance thresholds are shown: conservative threshold of the maximum of 1000 permutations of classification labels across all time lags and the 95% percentiles (see ‘Methods’ for details). (B) Permutation distribution of mean sequenceness values across 1000 state permutations. Observed mean sequenceness is indicated with a red line. (C) Association between memory performance and mean sequenceness value computed across all trials, and time lags, for each participant.
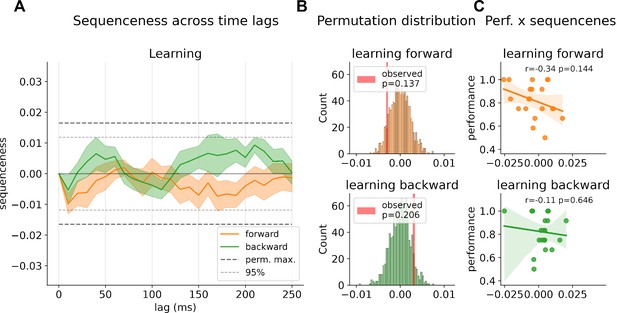
Sequential replay for all learning blocks.
(A) Strength of forward and backward sequenceness across different time lags (see ‘Methods’ and Figure 4 for details). (B) Permutation distribution of mean sequenceness values across 1000 state permutations. (C) Association between memory performance and mean sequenceness value computed across all trials, and time lags, for each participant. Note: as the paradigm applied criteria learning, participants had different amount of blocks and hence different exposure at different time points (see Figure 2—figure supplement 1), making a block-wise comparison between participants conceptually difficult. Therefore, to alleviate the bias of different learning speeds, we combined all trials of the learning blocks.
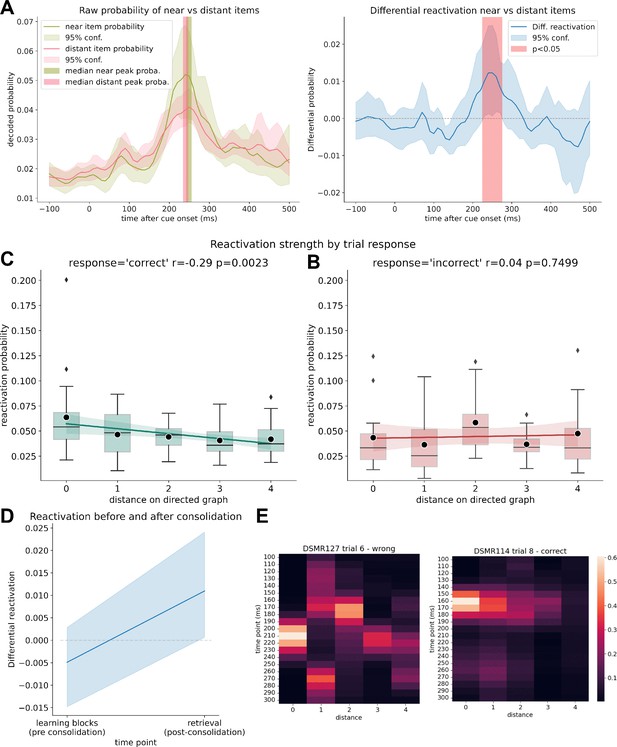
Clustered reactivation during retrieval.
(A) Decoded raw probabilities for off-screen items that were up to two steps ahead of the current stimulus cue (‘near’) vs. distant items that were more than two steps away on the graph, on trials with correct answers. The median peak decoded probability for near and distant items was at the same time point for both probability categories. Note that the displayed lines reflect the average probability while, to eliminate the influence of outliers, the peak displays the median. (B) Differential reactivation probability between off-screen items that were up to two steps ahead of the current stimulus cue vs. distant items that were more than two steps away on the graph for trials with correct answers. Between 220 and 260 ms, the next items are simultaneously reactivated significantly more than the items that are further away (p<0.05; permutation test with 10,000 shuffles). (C) Reactivation strength of items after retrieval cue onset by distance of items to the currently on-screen stimulus subdivided into trials in which participants answered correctly (left) and in which participants did not know the correct answer (right). A correlation between reactivation strength and distance can only be seen in case of successful retrieval (but see also limitations for a discussion of the low trial and participant number in this sub-analysis). Mean probability values are marked by black dots. (D) Mean differential reactivation at peak time point (220–260 ms) during all learning trials (before consolidation) compared to retrieval trials. (E) Example activations of a successful retrieval (left) and a failed retrieval (right), sorted by distance to current cue. Colors indicate probability estimates of the decoders.
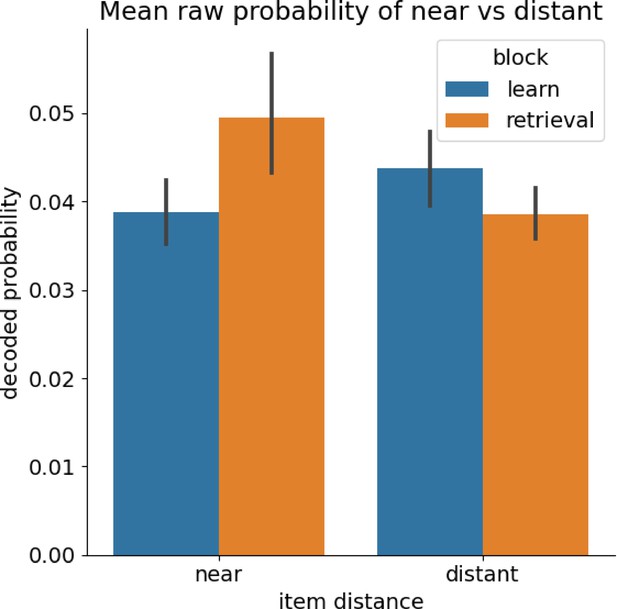
Mean raw probabilities of near vs far item reactivation at peak time point (210–240 ms, see Figure 3B) during learning and retrieval blocks.
Reactivation markers increased for near items while it slightly decreased for far items. However, all interactions are nonsignificant. Note that direct comparison of raw probabilities between different recording session parts might be difficult to interpret due to baseline probability shifts (e.g. due to sensor distance or head position changes).
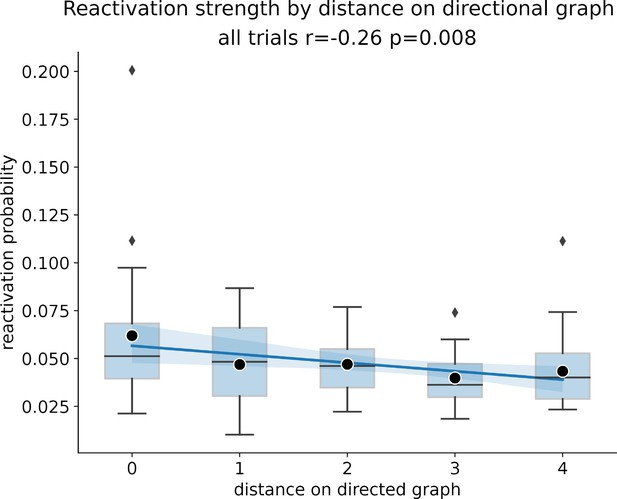
Reactivation strength of items after retrieval cue onset by distance of items to the currently on-screen stimulus.
A significant negative correlation between distance on a directional graph and reactivation strength can be seen (p=0.008). The correlation is shown for both correct and incorrect answers. For a sub-analysis of correct and incorrect analysis, see Figure 3C.
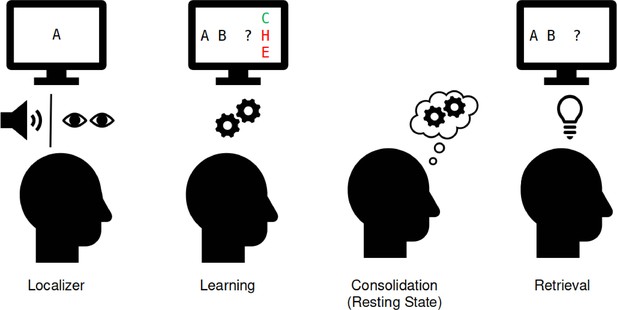
Experimental procedure in the magnetoencephalographic (MEG).
Localizer task: the 10 individual items were repeatedly presented to the participant auditorily and visually to extract multisensory activity patterns. Learning: participants learned pseudo-randomly generated triplets of the 10 items by trial and error. These triplets were determined by an underlying graph structure. Participants were unaware of the exact structure and graph layout. Consolidation: 8 min of resting state activity were recorded. Retrieval: participants’ recall was tested by cueing triplets from a sequence. The letters in the pictograms are placeholders for individual images.
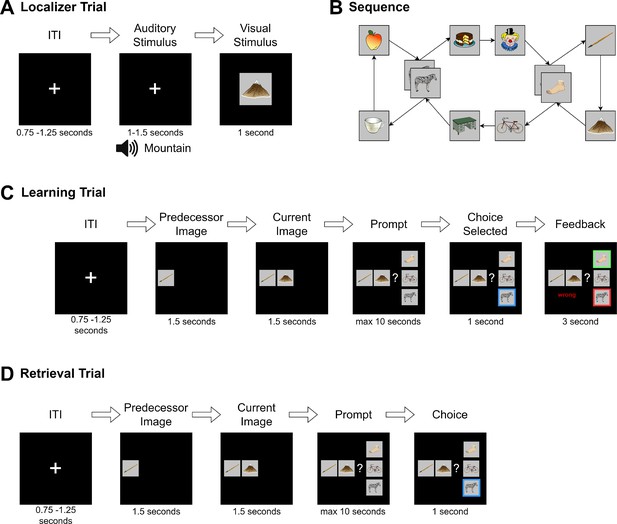
Task structure.
(A) During the localizer task, a word describing the stimulus was played via headphones and the corresponding visual item was then shown to the participant. In 4% of trials, the audio and visual cue did not match, and in this case, participants were instructed to press a button on detection (attention check). (B) Graph layout of the task. Two elements could appear in two different triplets. The graph was directed such that each tuple had exactly one successor (e.g., apple→zebra could only be followed by cake and not mug), but individual items could have different successors (zebra alone could be followed by mug or cake). Participants never saw the illustrated birds-eye view. (C) During learning, in each trial one node was randomly chosen as the current node. First, its predecessor node was shown, followed by the current node with the participant then given a choice of three items. They were then required to choose the node that followed the displayed cue tuple. Feedback was then provided to the participant. This process was repeated until the participant reached 80% accuracy for any block or reached a maximum of six blocks of learning. (D) The retrieval followed the same structure as the learning task, except that no feedback was given.