Proteogenomic analysis of air-pollution-associated lung cancer reveals prevention and therapeutic opportunities
Figures
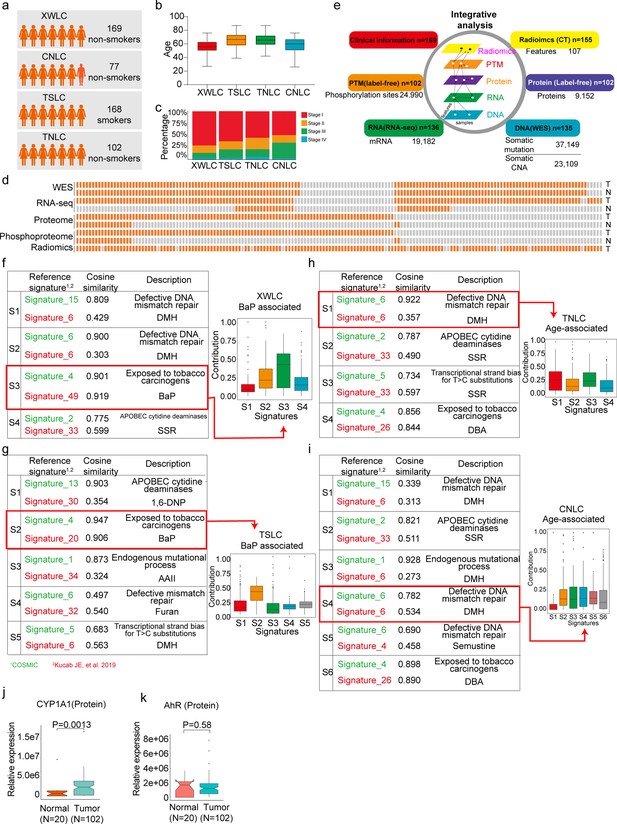
Proteogenomic profiling and mutational signatures in Xuanwei lung cancer (XWLC).
(a) Four cohort datasets used in this study: XWLC (Lung adenocarcinoma from non-smoking females in Xuanwei area), CNLC (subset of lung adenocarcinoma from non-smoking patients in Chinese Human Proteome Project), TSLC (subset of lung adenocarcinoma from smoking females in TCGA-LUAD project), TNLC (subset of lung adenocarcinoma from non-smoking females in TCGA-LUAD project); (b) Age distribution of patients at the time of operation in the four cohorts; (c) Distribution of tumor stages across the cohorts; (d) Data availability for the XWLC datasets. Each bar represents a sample, with orange bars indicating data availability and gray bars indicating data unavailability. T, tumor sample. N, Normal tissue; (e) Summary of data generated from the XWLC cohort; (f–i) Mutational signatures were identified in XWLC (f), TSLC (g), TNLC (h), and CNLC (i) cohorts. Cosine similarity analysis of the signatures compared to well-established COMIC signatures (in green) and Kucab et al. signatures (in red). Contribution of signatures in each cohort is provided on the right; (j–k) Protein abundance of CYP1A1 (j) and AhR (k) in tumor and normal samples within the XWLC cohort; Two-tailed Wilcoxon rank sum test used to calculate p-values in (j–k).
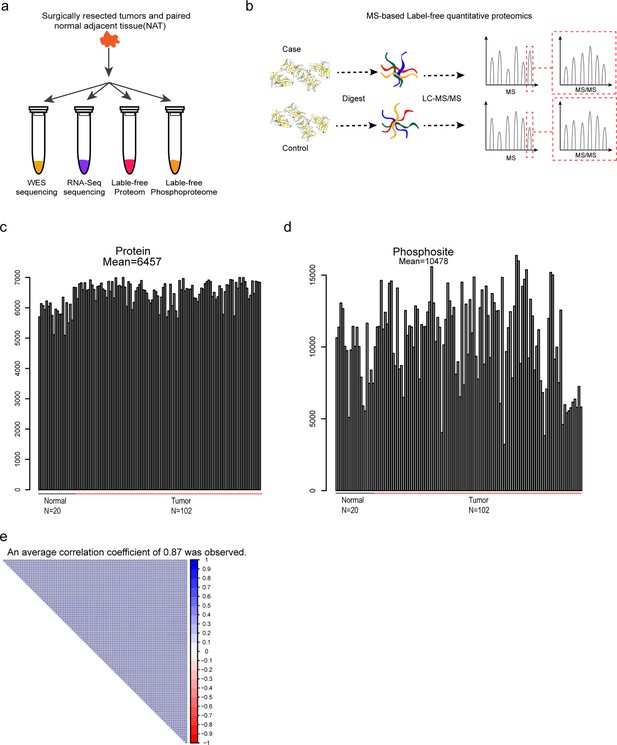
Experimental workflow and data quality metrics.
(a) Schematic representation showing sample processing steps. Tumors and their matched normal-adjacent tissues (NATs) were divided for genomics and proteomics analyses; (b) Schematic representation of the workflows used for proteome and phosphoproteome analyses. MS-based label-free quantification was used to determine the relative amount of proteins or phosphoproteins; (c–d) Bar plot showing consistent numbers of identified and quantified proteins (c) and phosphosites (d) in 102 tumors and 20 NATs; (e) Correlation matrix of 102 tumor proteomes (Pearson’s correlation coefficients).
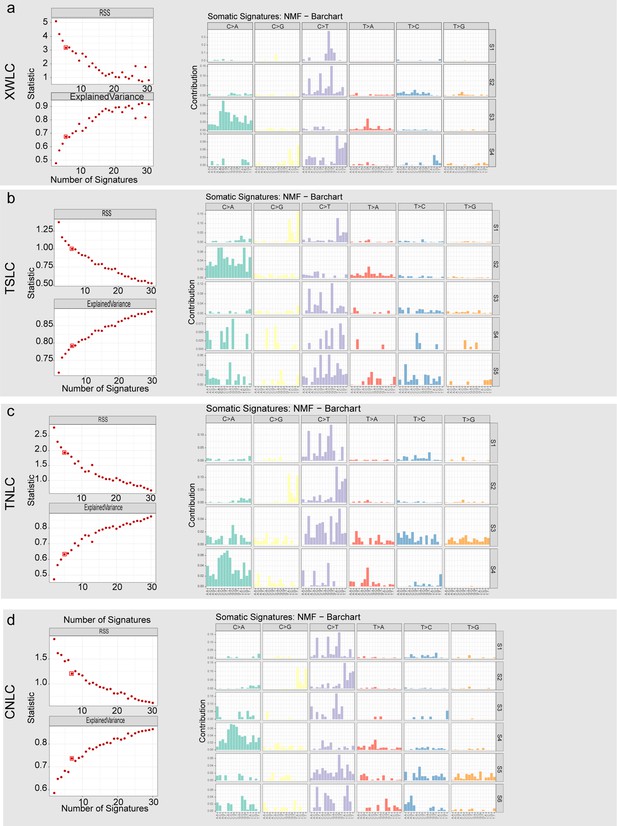
Identification and profile of de novo mutational signatures in each cohort.
(a–d) Left, residual sum of square (RSS) and percentage of variance explained of different signature number selections in each cohort. Number of signatures was determined by choosing the reflection point in the curves of the residual sum of squares (RSS) and explained variance. Right, mutation signatures detected in each cohort.
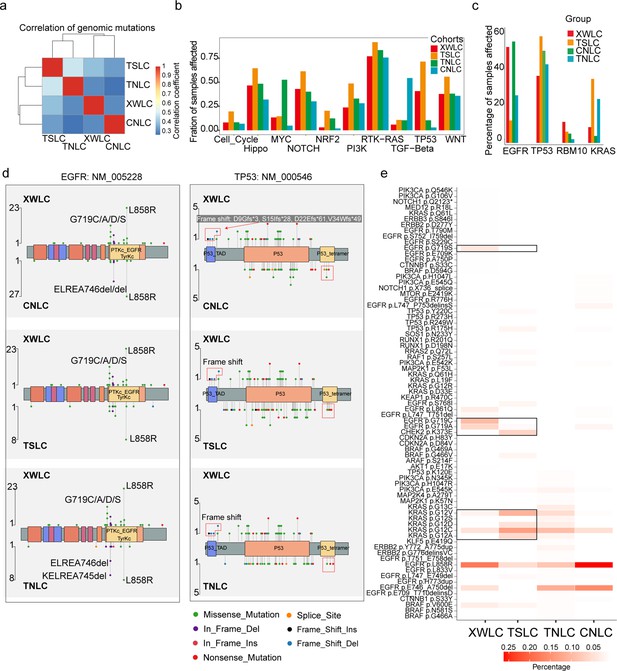
Genomic and genetic features in the Xuanwei lung cancer (XWLC) cohort.
(a) Correlation of genomic mutations among cohorts, determined using the Pearson correlation coefficient; (b) Comparison of oncogenic pathways affected by mutations in each cohort; (c) Comparison of mutation frequency of four key genes across cohorts; (d) Lollipop plot illustrating differences in mutational sites within EGFR (left) and TP53 (right) across XWLC/CNLC, XWLC/TSLC, and XWLC/TNLC pairs; (e) Analysis of the percentage of samples with actionable alterations, with a focus on significant variations between XWLC and TSLC cohorts, highlighted by black boxes.
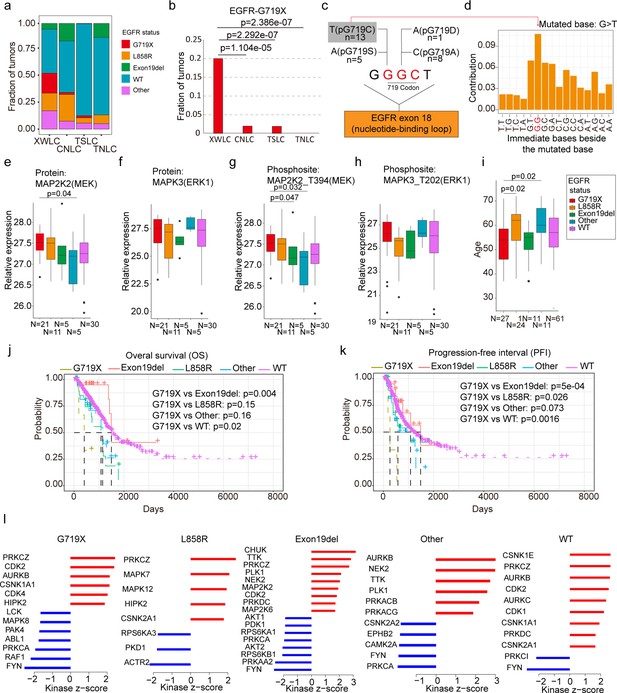
EGFR-G719X in the Xuanwei lung cancer (XWLC) cohort.
(a) Distribution of different EGFR mutation statuses across the four cohorts; (b) Comparison of the fraction of G719X mutations across the four cohorts. Two-sided Fisher’s test was used to calculate p-values; (c) Detailed information on pG719X (pG719/A/D/C/S) mutations in the XWLC cohort. The number of each mutation type is labeled; (d) Distribution of nucleotide pairs surrounding the most common G>T transversion site in the XWLC cohort. The x-axis represents the immediate bases surrounding the mutated base. For Benzo[a]pyrene (BaP), the tallest G>T peak occurs at GpGpG; (e–h) Comparison of activation levels of key components in the MAPK pathway across different EGFR mutation statuses in the XWLC cohor. N, number of tumor samples containing corresponding EGFR mutation; (i) Comparison of patient ages across different EGFR mutation statuses in the XWLC cohort, N, number of tumor samples containing corresponding EGFR mutation; (j–k) Presentation of overall survival (OS), (j) and progression-free interval (PFI), (k) analysis across different EGFR mutation statuses in the TCGA-LUAD cohort, Logrank test was used to calculate p-values; (l) Evaluation of kinase activities by KSEA in tumors across different EGFR mutation statuses in the XWLC cohort. The two-tailed Wilcoxon rank sum test was used to calculate p-values in panels (e–i).
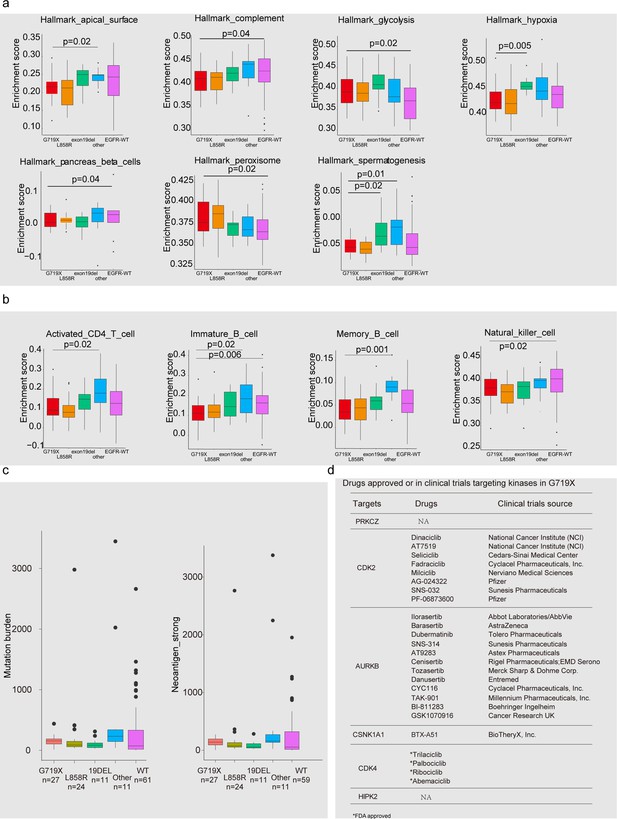
Comparison of hallmark capability, immune cell infiltration, and mutation burden across different EGFR mutation status.
(a) Hallmark capabilities; (b) Immune cell infiltration; (c) Mutation burden and neoantigens; (d) FDA-approved drugs targeting activated kinases in tumors with G719X mutation.
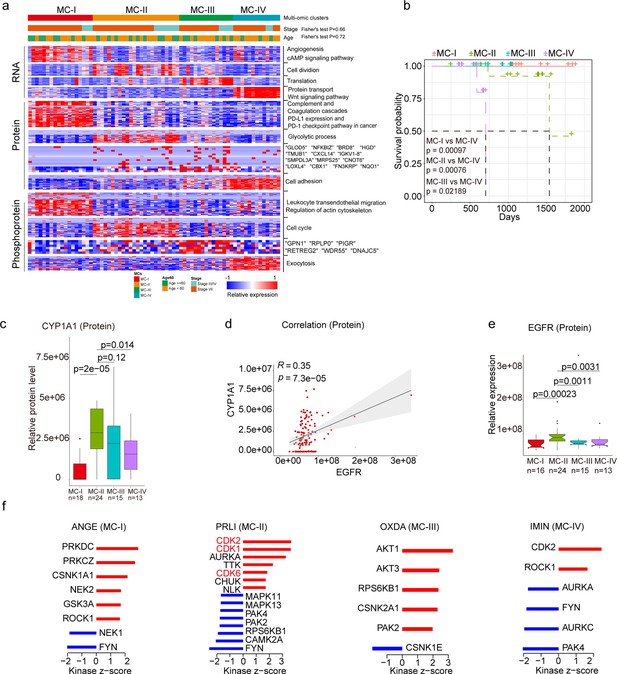
Subtyping of Xuanwei lung cancer (XWLC).
(a) Integrative classification of tumor samples into four ConsensusClusterPlus-derived clusters (MC-I to MC-IV). The heatmap displays the top 50 features, including mRNA transcripts, proteins, and phosphoproteins, for each multi-omic cluster. The features are annotated with representative pathways or genes. If a cluster has fewer than 50 features, all features are shown. If no significant GO biological processes are associated with cluster features, all features are displayed; (b) Comparison of overall survival between MC-IV and the other three subtypes; (c) Protein abundance comparison of CYCP1A1 across subtypes; (d) Protein-level correlation between CYCP1A1 and EGFR; (e) Protein-level comparison of EGFR across subtypes; (f) Evaluation of kinase activities by Kinase-Substrate Enrichment Analysis (KSEA) in tumors across subtypes in the XWLC cohort. The two-tailed Wilcoxon rank sum test was used to calculate p-values in panels (c) and (e).

ConsensusClusterPlus clustering in Xuanwei lung cancer (XWLC).
(a) Delta area plot showing the relative change in area under the CDF curve comparing k and k-1. This plot allows to determine the relative increase in consensus and determine k at which there is no appreciable increase. (b) Consensus cumulative distribution function (CDF) Plot shows the cumulative distribution functions of the consensus matrix for each k (indicated by colors), estimated by a histogram of 100 bins. This figure allows to determine at what number of clusters, k, the CDF reaches an approximate maximum, thus consensus and cluster confidence is at a maximum at this k. (c) Heatmaps of the consensus matrices for k=4.
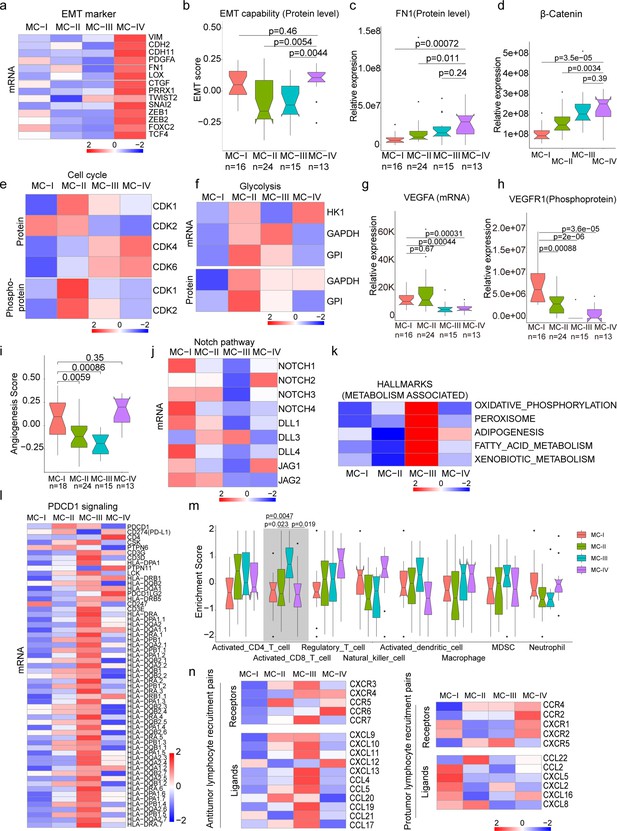
Biological and immune features across MC subtypes.
(a) Relative expression of epithelial-mesenchymal transition (EMT) markers across subtypes; (b) EMT scores across subtypes using a gene set derived from MsigDB (M5930); (c–d) Protein abundance comparison of FN1 (c) and β-Catenin (d) across subtypes; (e) Protein and phosphoprotein levels of key cell cycle kinases across subtypes; (f) Expression of mRNA and protein levels of glycolysis-associated enzymes; (g) mRNA expression of VEGFA across subtypes; (h) Phosphoprotein abundance of VEGFR1 across subtypes; (i) Angiogenesis score across subtypes using a gene set derived from MsigDB (Systematic name M5944); (j) Expression comparison of key regulators of the Notch pathway across subtypes; (k) Metabolism-associated hallmarks across subtypes. Gene sets for oxidative phosphorylation, peroxisome, adipogenesis, fatty acid metabolism, and xenobiotic metabolism were derived from MsigDB hallmark gene sets; (l) Expression of PD-1 signaling-associated genes across subtypes. PD-1 signaling-associated genes were derived from MsigDB (Systematic name M18810); (m) Immune cell infiltration across subtypes. Gene sets for each immune cell type were derived from a previous study (Charoentong et al., 2017); (n) Expression of anti-tumor/pro-tumor lymphocyte receptors and ligands across subtypes. The two-tailed Wilcoxon rank sum test was used to calculate p-values in panels b, c, d, g, h, i, and m.
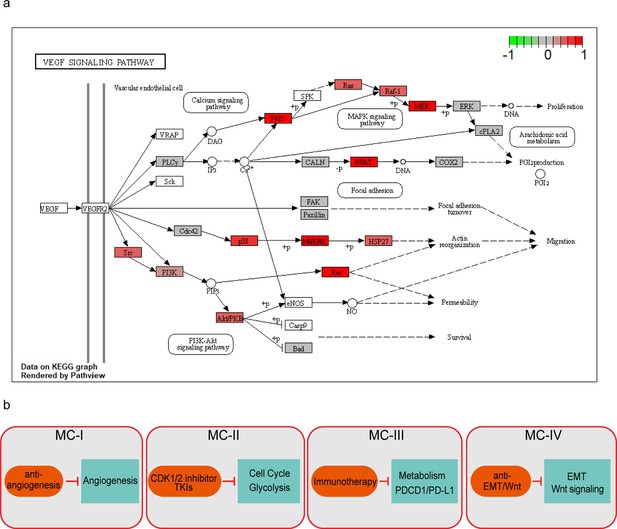
KEGG pathview showing the difference across MC subtypes.
KEGG pathview showing the protein change between tumor vs normal in MC-I subtype (a) and therapeutic strategies for each subtype (b).
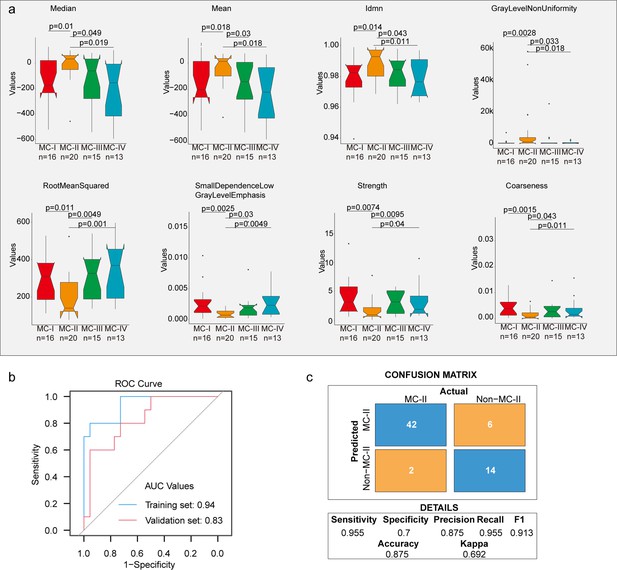
Radiomic features across subtypes.
(a) Eight features showing significant differences between MC-II and the other three subtypes. The Wilcoxon rank sum test was used to calculate the p-values; (b) A receiver operating characteristic (ROC) curve was used to evaluate the performance of the radiomic signature in distinguishing MC-II from the other three subtypes; (c) Confusion matrix allows visualization of the performance of the algorithm in separating MC-II from other subtypes.
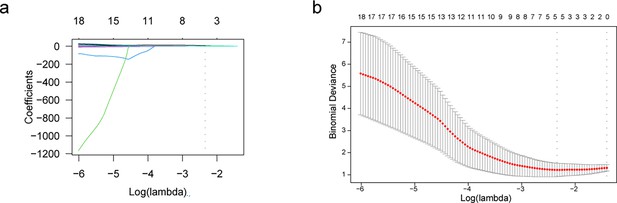
Determination of number of signature features.
(a) LASSO regression coefficient varies with change trend of tuning parameters (λ). The upper horizontal axis is the number of non-zero coefficients in the model. The dotted line is the λ value with the smallest mean squared error. (b) λ selected in the LASSO model applied tenfold cross-validation via the minimum criteria. The upper horizontal axis is the number of non-zero coefficients in the model. The left dotted line is the λ value with the smallest mean squared error; The right dotted line is the λ value when the mean squared error is the minimum mean square error plus 1 standard error.
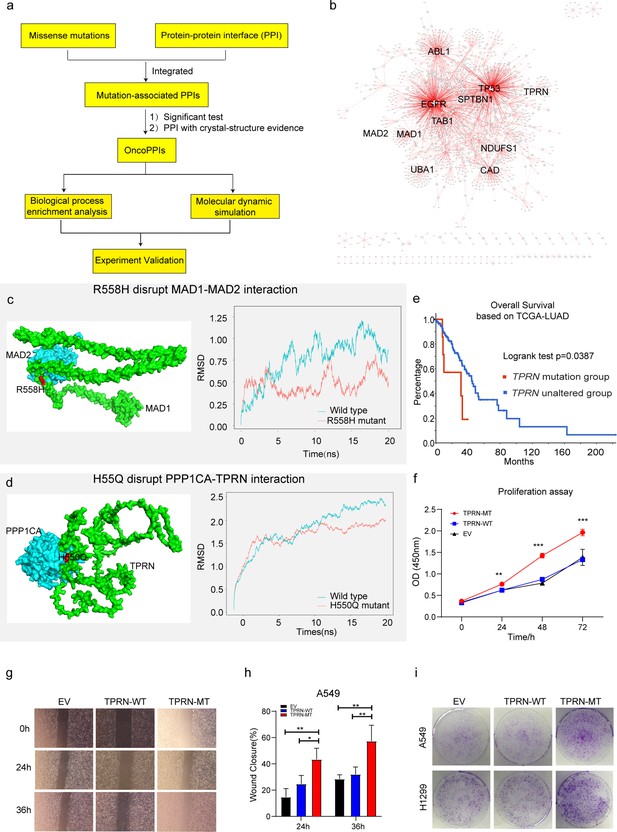
Identification of novel targets in Xuanwei lung cancer (XWLC).
(a) Flow chart showing the integration of mutation-informed PPI analysis, molecular dynamic simulation, and experiment validation to identify novel targets; (b) Network visualization of XWLC_oncoPPIs. Edge thickness represents the number of missense mutations at the protein-protein interaction (PPI) interface, while node size indicates connectivity; (c) MAD1-MAD2 interaction model and the p.Arg558His mutation at the interface (left). The complex model was generated using Zdock protein docking simulation. The right distribution showing root-mean-squared deviation (RMSD) during a 20 ns molecular dynamics simulation of MAD1 wild-type vs. MAD1 p.Arg558His in the complex; (d) Model showing the p.His550Gln alteration within the TPRN-PPP1CA complex (left). The right distribution showing root-mean-squared deviation (RMSD) during a 20 ns molecular dynamics simulation for TPRN wild-type vs. TPRN p.His550Gln (H550Q) in the complex; (e) Survival analysis of the TPRN mutation group and an unaltered group derived from cbioportal using TCGA-LUAD cohort (https://www.cbioportal.org/); (f) CCK8 assay for empty vector (EV), TPRN-WT, and TPRN-MT cell lines in A549 cells which was transfected by EV, TPRN-WT, and TPRN-MT, respectively. (g) Transwell assay for EV, TPRN-WT, and TPRN-MT after 24 hr and 36 hr in A549 cells. Magnification was set to 40 x; (h) Bar chart showing the statistical results of transwell assay; i. Cell colony assay for EV, TPRN-WT, and TPRN-MT in A549 and H1299 cell lines. All samples were run in triplicate; The two-tailed Wilcoxon rank sum test was used to calculate p-values in f and h. *p<0.05; **p<0.01; ***p<0.001.
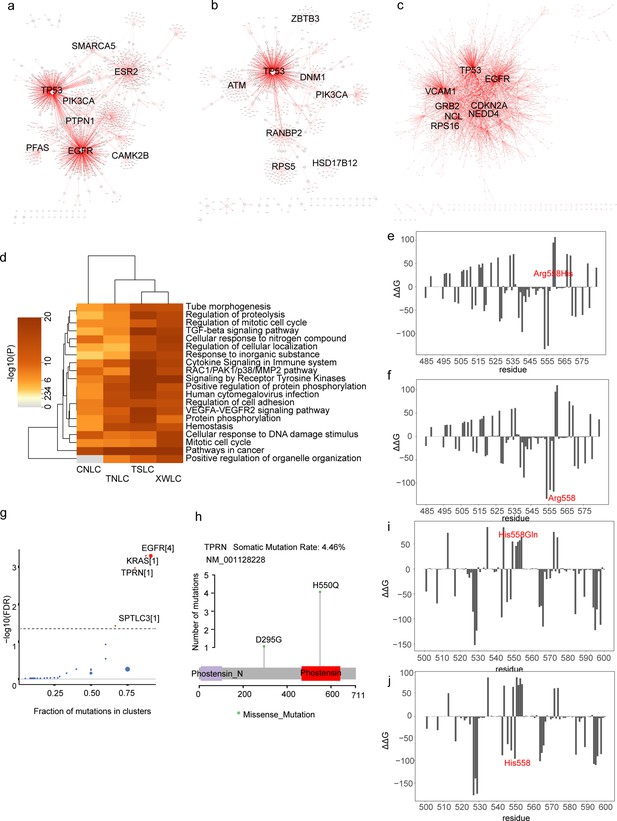
Networks from oncoPPIs and energy distribution.
(a–c) Networks for CNLC_oncoPPIs (a), TNLC_oncoPPIs (b) and TSLC_oncoPPIs (c). Edge thickness is proportioned to the number of missense mutations at the PPIs interface. Node size is measured by connectivity; (d) Clustered heatmap showing the top 20 enriched terms derived from oncoPPIs across CNLC, TSLC, TNLC, and LCCS cohorts. Colors are scaled by p-values; (e–f) Distribution of binding affinity (ΔG) at amino acid residue for MAD1-MAD2 complex in mutant p.Arg558His (e) and wild-type (f) on MAD1; (g) Cancer driver genes detected in LCCS based on positional clustering (Tamborero et al., 2013). Dot size is proportional to the number of clusters found in the gene. The x-axis shows the number of mutations (or fraction of mutations) observed in these clusters; (h) Lollipop plots for amino acid changes in TPRN in LCCS cohort; (i–j) Distribution of binding affinity (ΔG) at amino acid residue for TPRN-PPP1CA in p.His550Gln (i) and wild-type (j) on TPRN.
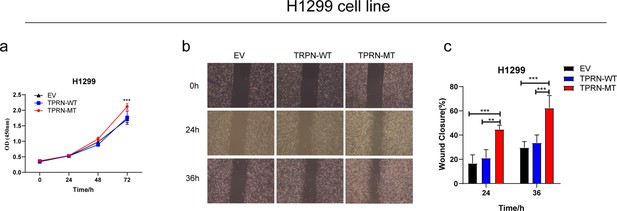
TPRN p.His550Gln promotes tumor progression in the H1299 cell line.
(a) CCK8 assay for TPRN-MT, TPRN-WT, and EV cell lines in H1299 cells. (b) Transwell assay for TPRN-MT, TPRN-WT, and EV after 24 hr and 36 hr in H1299 cells. Magnification was set to 40 x; (c) Bar chart showing the statistical results of transwell assay; All samples were run in triplicate; The two-tailed Wilcoxon rank sum test was used to calculate p-values in a and c. *p<0.05; **p<0.01; ***p<0.001.
Tables
Liquid chromatography elution gradient table.
Time (min) | Flow rate (nL/min) | Mobile phase A (%) | Mobile phase B (%) |
---|---|---|---|
0 | 600 | 94 | 6 |
2 | 600 | 90 | 10 |
45 | 600 | 70 | 30 |
48 | 600 | 65 | 35 |
50 | 600 | 50 | 50 |
51 | 600 | 0 | 100 |
60.5 | 600 | 95 | 5 |
61.5 | 600 | 95 | 5 |
62 | 600 | 5 | 95 |
67 | 600 | 5 | 95 |
70 | 600 | 95 | 5 |
Liquid chromatography elution gradient table.
Time(min) | Flow rate (nL/min) | Mobile phase A (%) | Mobile phase B (%) |
---|---|---|---|
0 | 600 | 95 | 5 |
2 | 600 | 90 | 10 |
112 | 600 | 70 | 30 |
117 | 600 | 50 | 50 |
118 | 600 | 5 | 95 |
123 | 600 | 5 | 95 |
Additional files
-
Supplementary file 1
Clinical information for TSLC, TNLC, CNLC, and XWLC cohorts.
(a) Clinical data for TSLC and TNLC cohorts. (b) Clinical data for CNLC cohort. (c) Clinical data for XWLC cohort.
- https://cdn.elifesciences.org/articles/95453/elife-95453-supp1-v1.xlsx
-
Supplementary file 2
Somatic mutation profile in XWLC.
(a) Somatic mutation profile in XWLC. (b) Mutation frequency of cancer driver genes XWLC cohort.
- https://cdn.elifesciences.org/articles/95453/elife-95453-supp2-v1.xlsx
-
Supplementary file 3
Copy number variation results in XWLC.
- https://cdn.elifesciences.org/articles/95453/elife-95453-supp3-v1.xlsx
-
Supplementary file 4
Normalized mRNA expression (CPM values derived from EdgeR) in XWLC.
- https://cdn.elifesciences.org/articles/95453/elife-95453-supp4-v1.xlsx
-
Supplementary file 5
Proteomic expression results normalized by column (patient) median.
- https://cdn.elifesciences.org/articles/95453/elife-95453-supp5-v1.xlsx
-
Supplementary file 6
Quantitative phosphoproteomic data at phosphosite level normalized by column (patient) median.
- https://cdn.elifesciences.org/articles/95453/elife-95453-supp6-v1.xlsx
-
Supplementary file 7
Estimation of Immune cell infiltration with ssGSEA method.
- https://cdn.elifesciences.org/articles/95453/elife-95453-supp7-v1.xlsx
-
Supplementary file 8
Radiomics features in XWLC cohort.
- https://cdn.elifesciences.org/articles/95453/elife-95453-supp8-v1.xlsx
-
Supplementary file 9
Onco_PPIs identified in four cohorts.
(a) onco_PPIs in XWLC cohort; (b) onco_PPIs in CNLC cohort; (c) onco_PPIs in TNLCF cohort; (d) onco_PPIs in TSLC cohort.
- https://cdn.elifesciences.org/articles/95453/elife-95453-supp9-v1.xlsx
-
MDAR checklist
- https://cdn.elifesciences.org/articles/95453/elife-95453-mdarchecklist1-v1.docx