CausalXtract, a flexible pipeline to extract causal effects from live-cell time-lapse imaging data
Figures
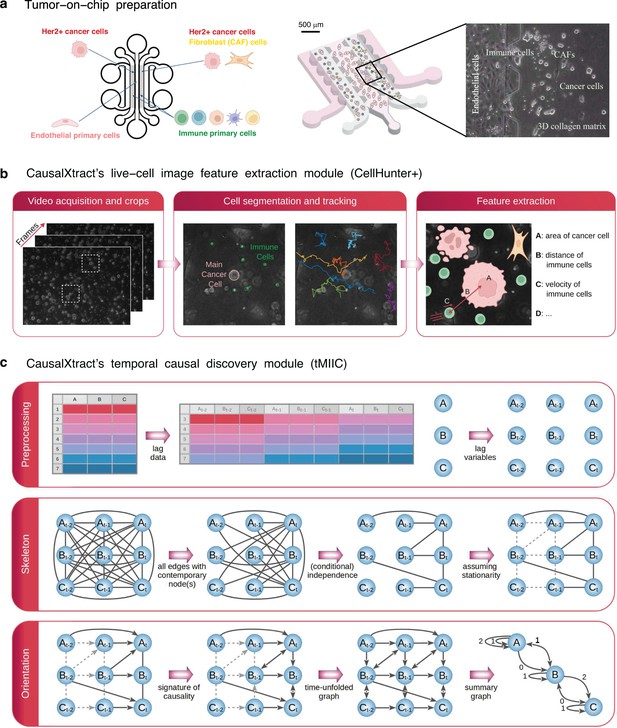
CausalXtract pipeline.
(a) Live-cell tumor ecosystem reconstituted ex vivo (Nguyen et al., 2018) using the tumor-on-chip technology (‘Materials and methods’). (b) CausalXtract’s live-cell image feature extraction module (CellHunter+). The tracking of cancer and immune cells and of their mutual interactions is illustrated in Videos 1–3, in the absence or presence of cell division and apoptosis event. Examples of time series of extracted cellular features are shown in Figure 1—figure supplement 1. (c) CausalXtract’s temporal causal discovery module (tMIIC) learns a temporal causal network from the features extracted in (b). See ‘Materials and methods’ for CausalXtract’s implementation details and theoretical foundations. A step-by-step notebook of CausalXtract pipeline is provided with the source code.
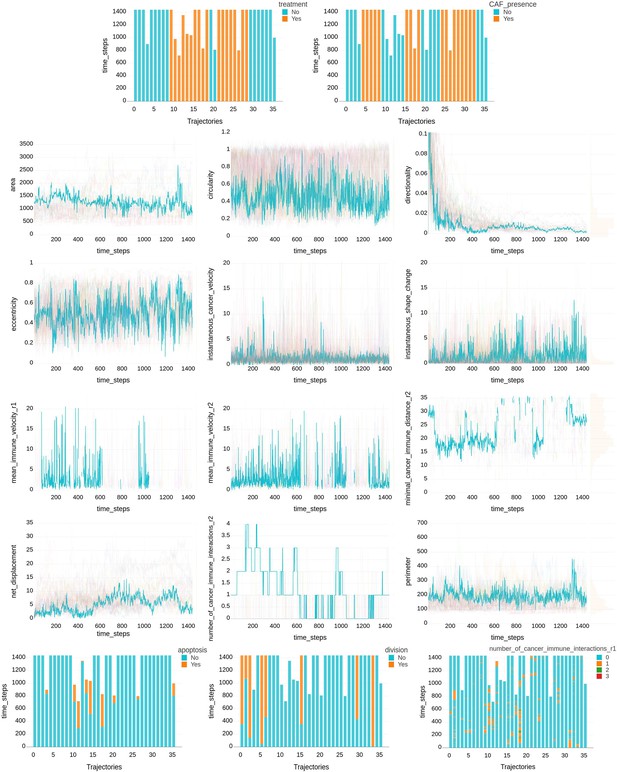
Time series of cellular features extracted from the tumor ecosystems.
Example of time series of cellular features extracted by CausalXtract’s feature extraction module (CellHunter+) from the tumor ecosystems analyzed in this study (Figure 1a). It includes two experimental control parameters (i.e., treatment and CAF presence) and 15 cellular features extracted every 2 min over a period of 2 days. Continuous features are highlighted for one trajectory (traj.18), while categorical features are shown for all trajectories.
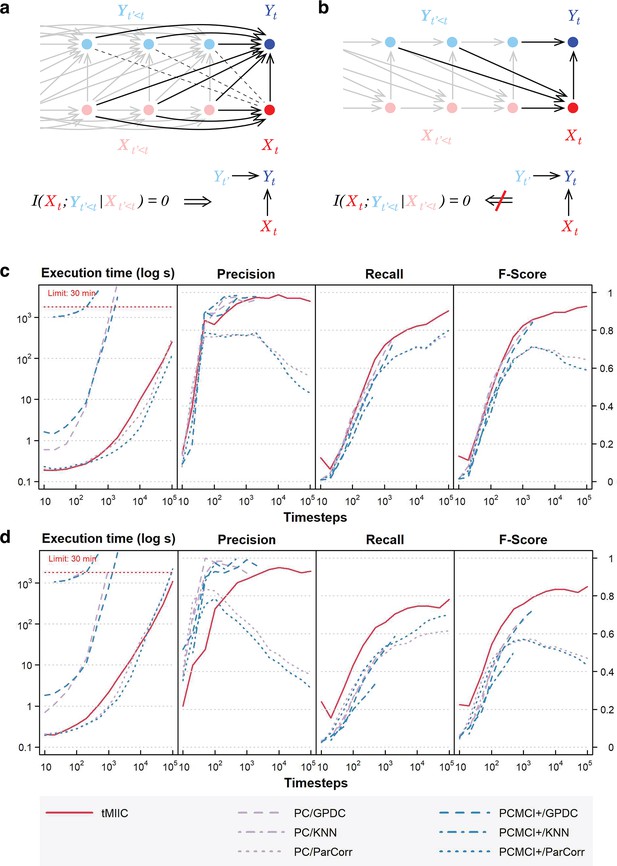
Relation to Granger–Schreiber temporal causality and tMIIC benchmarking against PC and PCMCI+.
(a) The signature of Granger–Schreiber temporal causality is a vanishing Transfer Entropy, that is, (‘Materials and methods’). In the time-unfolded causal network framework, it implies (i) the absence of (dashed) edge between and any , with , and (ii) if is adjacent to , the presence of temporal (2-variable+time) v-structures, , for all adjacent to , with (‘Materials and methods’, Theorem 1). (b) By contrast, the presence of a temporal (2-variable+time) v-structure, does not imply a vanishing Transfer Entropy as long as there remains an edge between any and . It implies that Granger–Schreiber temporal causality is in fact too restrictive and may overlook actual causal effects, which can be uncovered by graph-based causal discovery methods. Hence, tMIIC’s time-unfolded network framework, combining graph-based and information-based approaches, sheds light on the common foundations of the seemingly unrelated graph-based causality and Granger–Schreiber temporal causality, while clarifying their actual differences and limitations. (c) Benchmarking of tMIIC on synthetic time-series datasets generated from 15-node causal networks based on linear combinations of contributions, Appendix 1 and Figure 2—figure supplements 1–3. (d) Benchmarking with more complex 15-node time-series datasets based on nonlinear combinations of contributions, Appendix 2 and Figure 2—figure supplement 4. Running times and scores (Precision, Recall, Fscore) are averaged over 10 datasets and compared to PC and PCMCI+ methods using different kernels (GPDC, KNN, ParCorr).
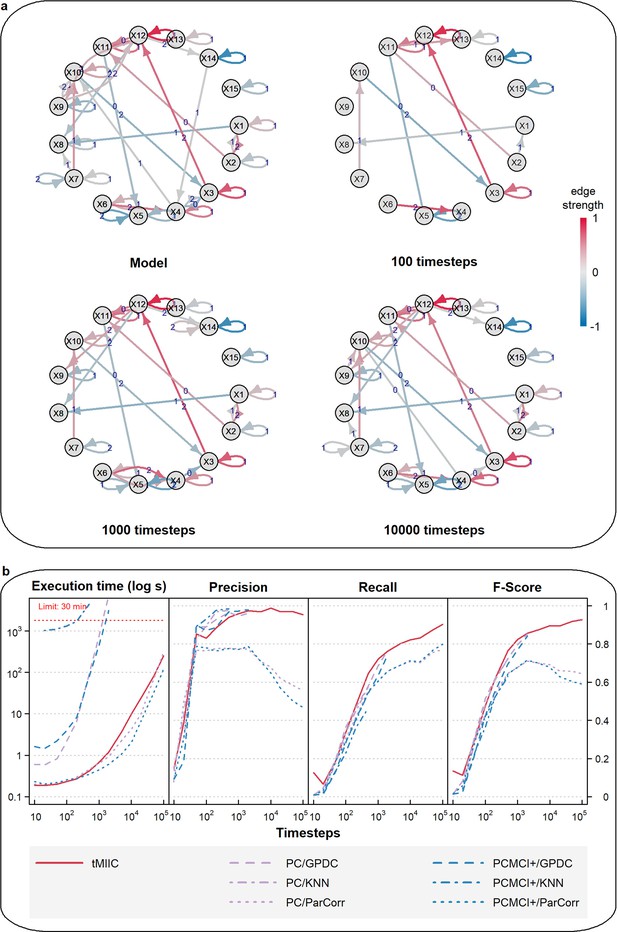
Benchmark assessment of CausalXtract’s causal discovery module (tMIIC) using generated time-series datasets.
(a) Example of a 15-node causal network to generate benchmark time-series datasets based on linear combinations of contributions (Appendix 1). Examples of temporal causal networks reconstructed by tMIIC based on 100, 1000, or 10,000 simulated time steps. (b) Running times and scores (Precision, Recall, Fscore) averaged over 10 datasets and compared to PC and PCMCI+ methods using different kernels (GPDC, KNN, ParCorr); tMIIC is at par with PC and PCMCI+ scores using GPDC and KNN kernels but runs orders of magnitude faster. Only ParCorr kernel matches tMIIC running speed but with significantly lower scores at large sample size; see ‘Materials and methods’.
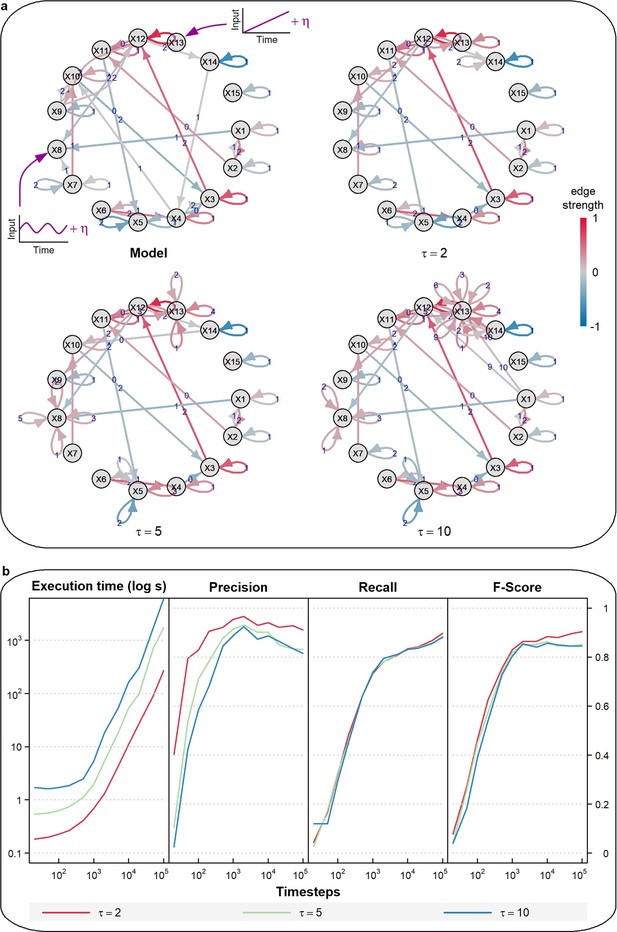
CausalXtract sensitivity to non-stationary variables.
(a) Example of a temporal causal network model () with a low-frequency periodic input () applied to X8 and a time-linear trend applied to X13. Corresponding temporal causal networks inferred by tMIIC from 1000 time step time series (Appendix 1) including non-stationary inputs to X8 and X13. Increasing the maximum lag from to or 10 leads to the appearance of multiple self-loops, which result from the non-stationary dynamics of X8 and X13, whilst the rest of the network remains largely unaffected. (b) Running times and scores (Precision, Recall, Fscore ignoring X8 and X13 self-loops) of tMIIC causal network reconstructions for or 10 averaged over 10 time series of 10 to 105 time steps.
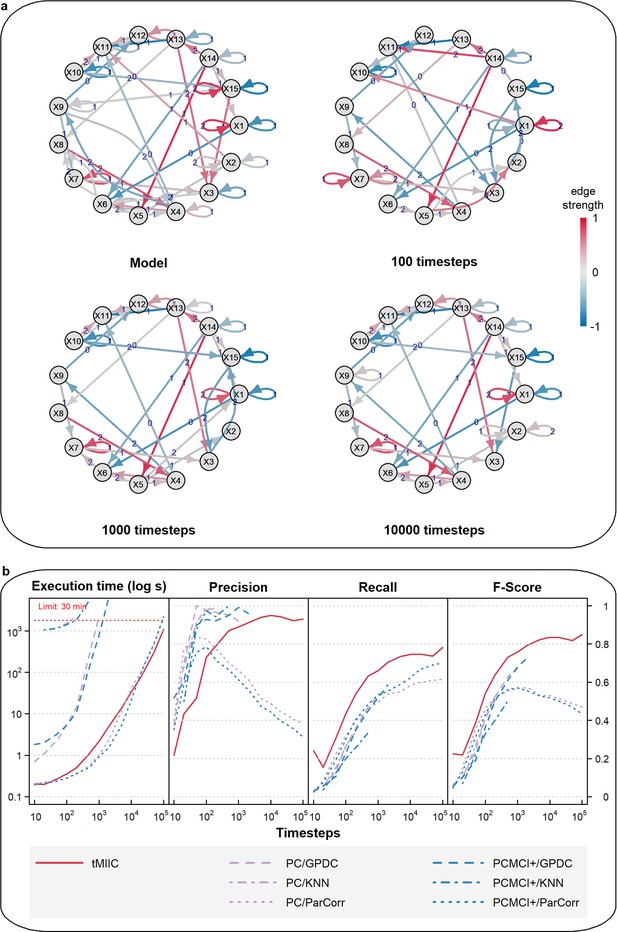
Benchmark assessment of CausalXtract’s causal discovery module (tMIIC) using more complex time-series datasets.
(a) Example of a 15-node causal network to generate more complex benchmark time-series datasets based on nonlinear combinations of contributions (Appendix 2). Examples of temporal causal networks reconstructed by tMIIC based on 100, 1000, or 10,000 simulated time steps. (b) Running times and scores (Precision, Recall, Fscore) averaged over 10 datasets and compared to PC and PCMCI+ methods using different kernels (GPDC, KNN, ParCorr); tMIIC outperforms both PC and PCMCI+, in terms of Recall and Fscores, while running orders of magnitude faster, except for the ParCorr kernel, which leads, however, to significantly lower scores at large sample size.
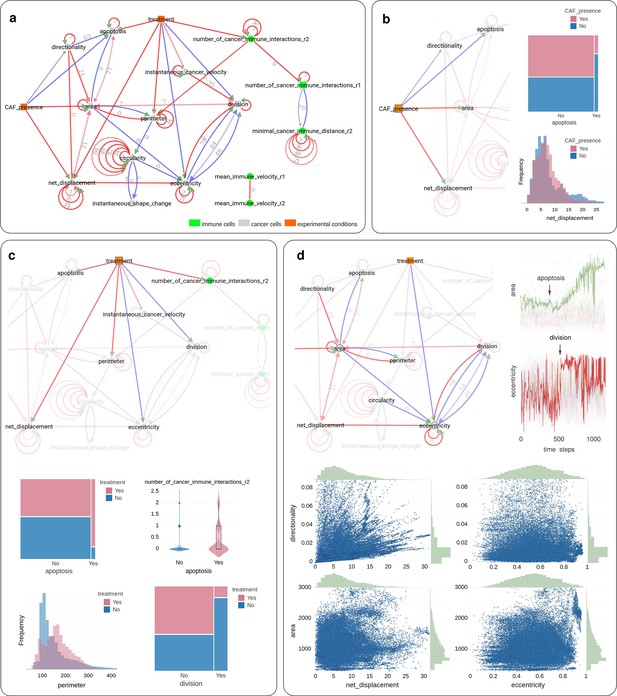
Application of CausalXtract to time-lapse images of tumor ecosystems reconstituted ex vivo.
(a) Summary causal network inferred by CausalXtract. The underlying time-unfolded causal network is shown in Figure 3—figure supplement 1. Red (resp. blue) edges correspond to positive (resp. negative) associations. Bidirected dashed edges represent the effect of unobserved (latent) common causes. Annotations on edges correspond to time delays in time steps (1 ts = 2 min). The inferred network is largely robust to variations in sampling rate () and maximum lag (), Figure 3—figure supplement 2. Here, ts and ts are chosen automatically by CausalXtract. (b) The CAF presence subnetwork highlighting the direct causal effects of CAFs on cancer cells. In particular, CausalXtract uncovers that CAFs directly inhibit cancer cell apoptosis independently from treatment, which has not been reported so far. (c) The treatment subnetwork highlighting the direct causal effects of treatment on cancer cells. In particular, CausalXtract uncovers that treatment increases cancer cell perimeter, which has not been reported either. (d) The eccentricity-area subnetwork highlighting multiple direct and possibly antagonistic time-lagged effects, notably, between cell division and eccentricity and between cell apoptosis and area, as discussed in the main text.
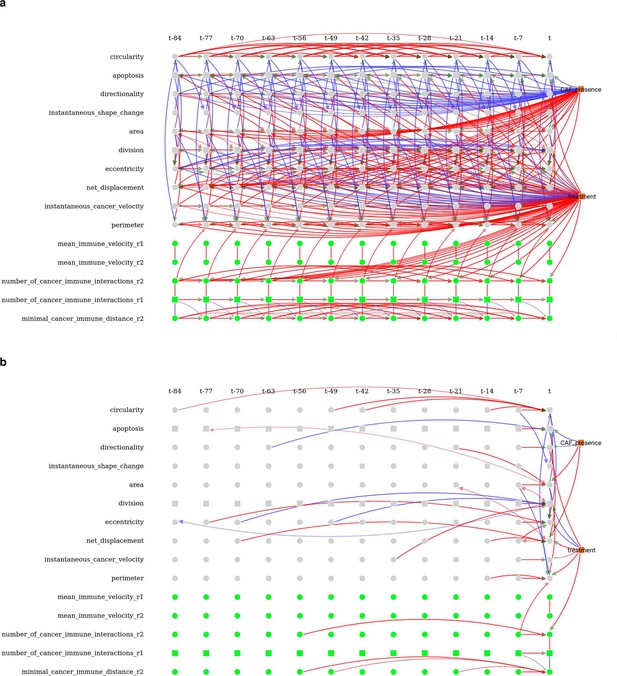
Time-unfolded causal network inferred by CausalXtract.
(a) Time-unfolded causal network assuming stationary dynamics of cellular ecosystems implying translational time invariance of the inferred causal network. (b) Only edges involving at least one contemporaneous variables (i.e., at time t) need to be tested for conditional independence by tMIIC and the remaining edges are then duplicated at all previous time steps before assigning orientations when time-lagged latent variables are taken into account (Figure 1c). Variables retaining multiple self-loops with different time delays correspond to non-stationary variables in Figure 1—figure supplement 1, in agreement with benchmarks from simulated data including non-stationary variables (Figure 2—figure supplement 3).
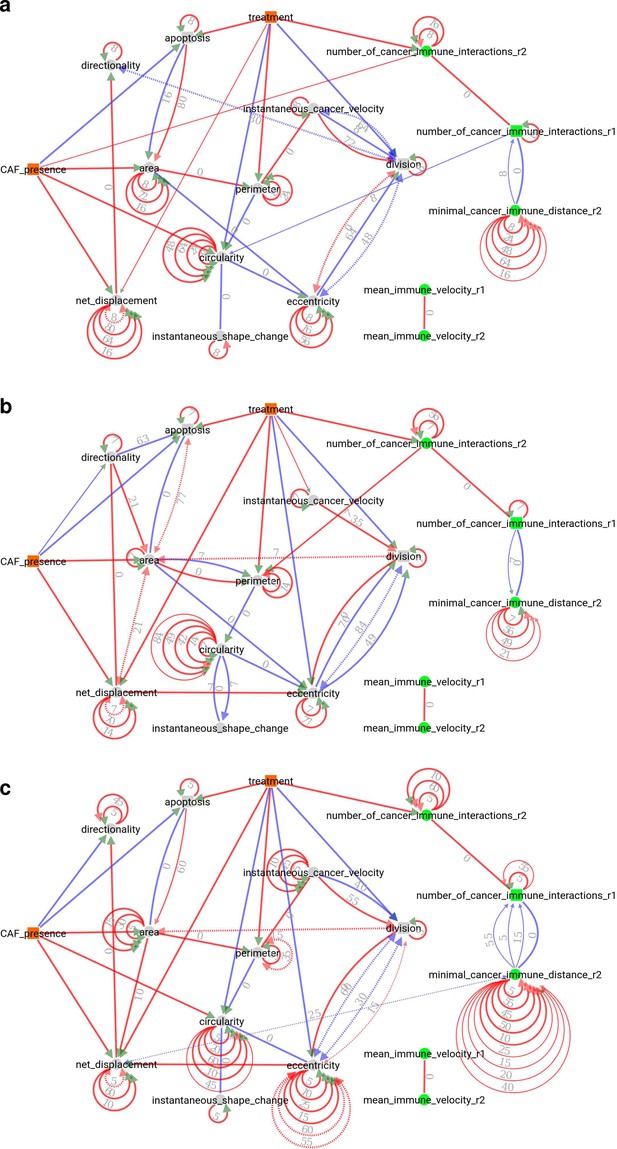
Robustness of CausalXtract’s temporal causal networks to variations in sampling rate.
Summary causal networks inferred by CausalXtract using different sampling rates (). (a) ts and ts, in time step units (1 ts = 2 min). (b) ts, and ts, as chosen automatically by CausalXtract based on the average relaxation time across the 15 monitored variables, ts, which defines a maximum lag ts. Given a total number of (time-lagged and -unlagged) nodes, chosen to be around 200 nodes for computational efficiency, it leads to 13 temporal layers () and a lag increment ts. This summary causal network corresponds to Figure 3a. (c) ts and ts, corresponding to with temporal layers, as in (b).