Disparity in temporal and spatial relationships between resting-state electrophysiological and fMRI signals
Figures
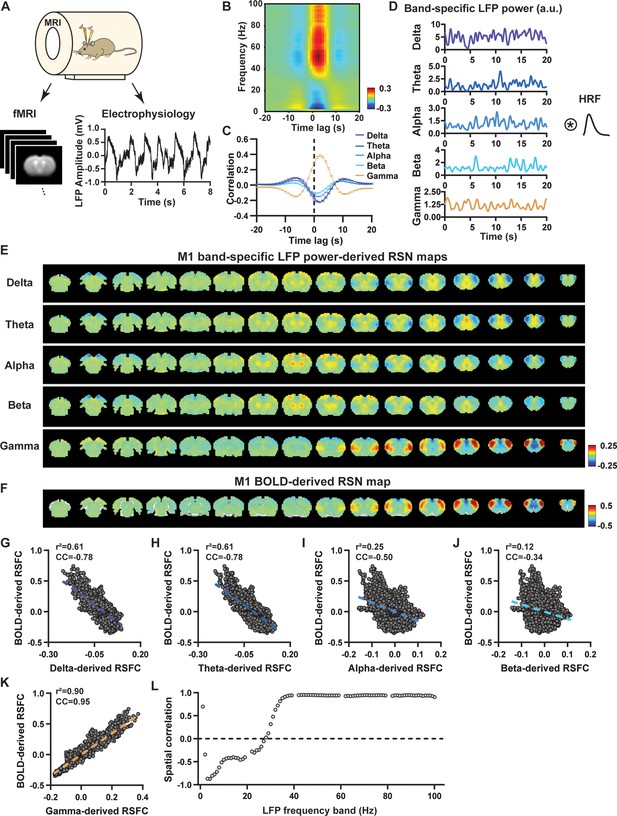
Highly consistent resting-state brain network (RSN) spatial patterns derived from local field potential (LFP) and resting-state functional magnetic resonance imaging (rsfMRI) signals in the primary motor cortex (M1) of lightly sedated rats.
(A) Simultaneous acquisition setup for whole-brain rsfMRI and electrophysiology signals in the M1 and anterior cingulate cortex (ACC). (B) Cross correlations between the rsfMRI signal and LFP power in the M1 across the frequency range of 0.1–100 Hz (band interval: 1 Hz; lag range: –20 to 20 s). (C) Cross correlations between the rsfMRI signal and powers of individual LFP bands in the M1. Error bars: SEM. (D) Exemplar powers of individual LFP bands. Convolving these powers with a rodent-specific hemodynamic response function (HRF) generates the corresponding LFP-predicted blood-oxygen-level-dependent (BOLD) signals. (E) M1 band-specific LFP power-derived RSN maps, obtained by voxel-wise correlating the LFP-predicted BOLD signal for each band with BOLD signals of all brain voxels. (F) M1 BOLD-derived RSN map (i.e. M1 seedmap), obtained by voxel-wise correlating the regionally averaged BOLD time course of the seed (i.e. M1) with BOLD time courses of all brain voxels. (G–K) Spatial similarity between the M1 BOLD-derived RSN map and the M1 LFP-derived RSN map for each band, quantified by their voxel-to-voxel spatial correlations (G: delta, CC = –0.78; H: theta, CC = –0.78; I: alpha, CC = –0.5; J: beta, CC = –0.34; K: gamma, CC = 0.95). (L) Spatial correlations between the M1 BOLD-derived RSN map and RSN maps derived by individual 1-Hz bands across the full LFP spectrum.
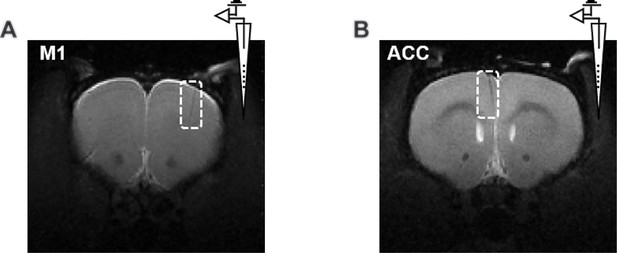
Representative T2-weighted structural images confirming the electrode location in (A) primary motor cortex (M1) and (B) anterior cingulate cortex (ACC).
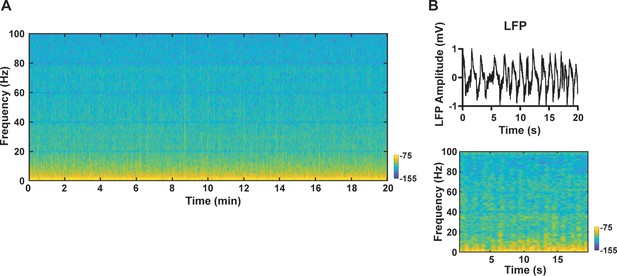
Exemplar denoised local field potential (LFP) signal.
(A) Exemplar LFP power spectrogram from one functional magnetic resonance imaging (fMRI) scan. (B) A ‘zoom-in’ 20 s segment of the LFP signal. Top: LFP time series; bottom: LFP power spectrogram.
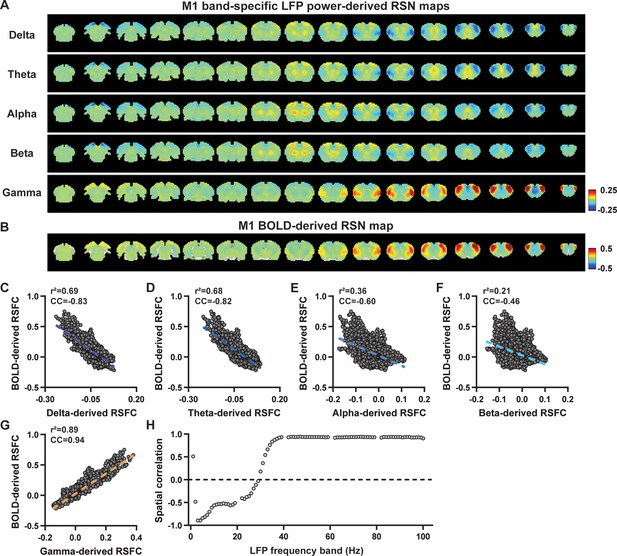
Spatial relationship between primary motor cortex (M1) local field potential (LFP)-derived and M1 blood-oxygen-level-dependent (BOLD)-derived resting-state brain networks (RSNs) without global signal regression in resting-state functional magnetic resonance imaging (rsfMRI) data preprocessing.
(A) RSN maps derived by band-specific LFP powers in the M1. (B) M1 BOLD-derived RSN map (i.e. M1 seedmap). (C–G) Spatial similarity between the M1 BOLD-derived RSN map and the M1 LFP-derived RSN maps for individual bands, quantified by their voxel-to-voxel correlations (C: delta, CC = –0.83; D: theta, CC = –0.82; E: alpha, CC = –0.6; F: beta, CC = –0.46; G: gamma, CC = 0.94). (H) Spatial correlations between the M1 BOLD-derived RSN map and RSN maps derived by all 1-Hz bands in the full LFP spectrum.
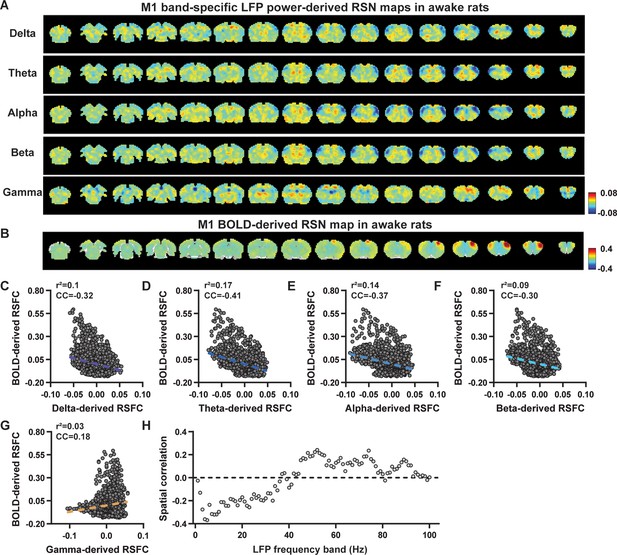
Spatial correlations of primary motor cortex (M1) local field potential (LFP)-derived and blood-oxygen-level-dependent (BOLD)-derived resting-state brain networks (RSNs) in awake animals.
(A) RSN maps derived by band-specific LFP powers in the M1, obtained by voxel-wise correlating the LFP-predicted BOLD signal for each band with BOLD signals of all brain voxels. (B) M1 BOLD-derived RSN map (i.e. M1 seedmap), obtained by voxel-wise correlating the regionally averaged BOLD time course of the seed (i.e. M1) with BOLD time courses of all brain voxels. (C–G) Spatial similarity between the M1 BOLD-derived RSN map and M1 LFP-derived RSN maps of individual bands, quantified by their voxel-to-voxel spatial correlations (C: delta, CC = –0.32; D: theta, CC = –0.41; E: alpha, CC = –0.37; F: beta, CC = –0.30; G: gamma, CC = 0.18). (H) Spatial correlations between the M1 BOLD-derived RSN map and RSN maps derived by individual 1 Hz bands across the full LFP spectrum.
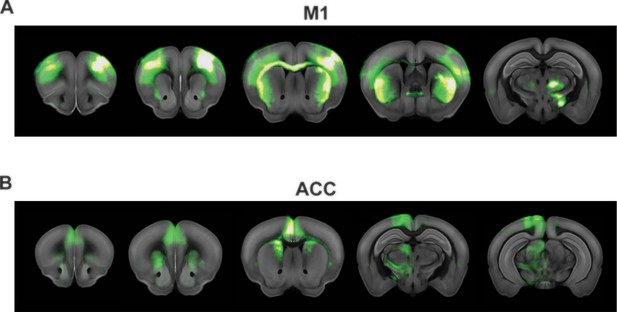
Anatomical connectivity labeled by tracers.
Adopted from the Allen Brain Institute database (Oh et al., 2014).
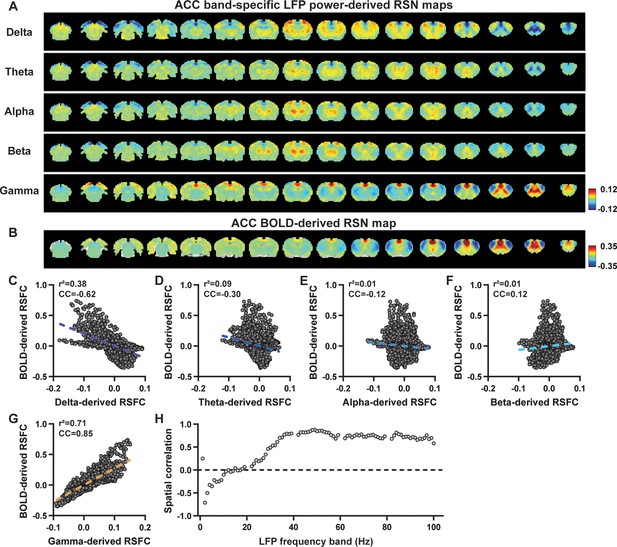
Highly consistent resting-state brain network (RSN) spatial patterns derived from local field potential (LFP) and resting-state functional magnetic resonance imaging (rsfMRI) signals in the anterior cingulate cortex (ACC) of lightly sedated rats.
(A) ACC band-specific LFP power-derived RSN maps, obtained by voxel-wise correlating the LFP-predicted blood-oxygen-level-dependent (BOLD) signal for each band with BOLD signals of all brain voxels. (B) ACC BOLD-derived RSN map (i.e. ACC seedmap), obtained by voxel-wise correlating the regionally averaged BOLD time course of the seed (i.e. ACC) with BOLD time courses of all brain voxels. (C–G) Spatial similarity between the ACC BOLD-derived RSN map and the ACC LFP-derived RSN map for each band, quantified by their voxel-to-voxel spatial correlations (C: delta, CC = –0.62; D: theta, CC = –0.30; E: alpha, CC = –0.12; F: beta, CC = 0.12; G: gamma, CC = 0.85). (H) Spatial correlations between the ACC BOLD-derived RSN map and RSN maps derived by individual 1-Hz bands across the full LFP spectrum.
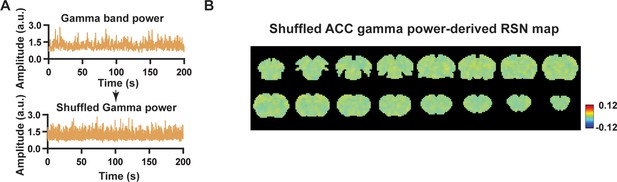
Control analysis for local field potential (LFP)-derived spatial pattern.
(A) Top: Example of gamma-band power in the anterior cingulate cortex (ACC); bottom: shuffled gamma-band power. (B) Resting-state brain network (RSN) map derived by shuffled gamma-band power, obtained by voxel-wise correlating the shuffled gamma-band power convolving with hemodynamic response function (HRF) to blood-oxygen-level-dependent (BOLD) signals of all brain voxels.
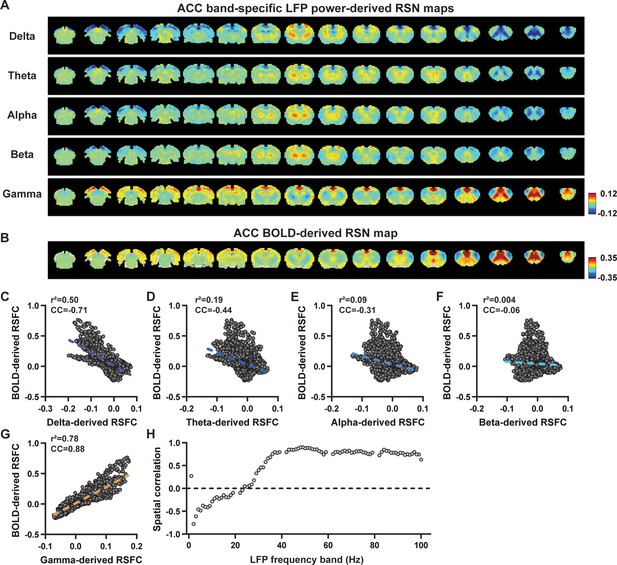
Spatial relationship between anterior cingulate cortex (ACC) local field potential (LFP)-derived and ACC blood-oxygen-level-dependent (BOLD)-derived resting-state brain networks (RSNs) without global signal regression in resting-state functional magnetic resonance imaging (rsfMRI) data preprocessing.
(A) RSN maps derived by band-specific LFP powers in the ACC. (B) ACC BOLD-derived RSN map (i.e. ACC seedmap). (C–G) Spatial similarity between the ACC BOLD-derived RSN map and the ACC LFP-derived RSN maps for individual bands, quantified by their voxel-to-voxel correlations (C: delta, CC = –0.71; D: theta, CC = –0.44; E: alpha, CC = –0.31; F: beta, CC = –0.06; G: gamma, CC = 0.88). (H) Spatial correlations between the ACC BOLD-derived RSN map and RSN maps derived by all 1-Hz bands in the full LFP spectrum.
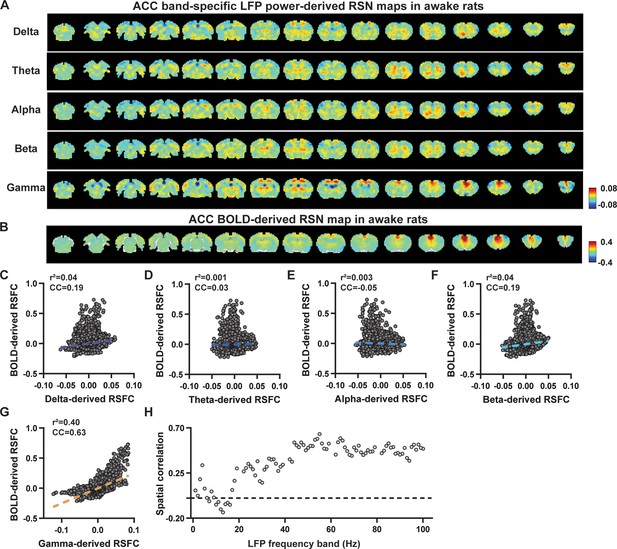
Spatial correlations of anterior cingulate cortex (ACC) local field potential (LFP)-derived and blood-oxygen-level-dependent (BOLD)-derived resting-state brain networks (RSNs) in awake animals.
(A) RSN maps derived by band-specific LFP powers in the ACC, obtained by voxel-wise correlating the LFP-predicted BOLD signal for each band with BOLD signals of all brain voxels. (B) ACC BOLD-derived RSN map (i.e. ACC seedmap), obtained by voxel-wise correlating the regionally averaged BOLD time course of the seed (i.e. ACC) with BOLD time courses of all brain voxels. (C–G) Spatial similarity between the ACC BOLD-derived RSN map and ACC LFP-derived RSN maps of individual bands, quantified by their voxel-to-voxel spatial correlations (C: delta, CC = –0.19; D: theta, CC = 0.03; E: alpha, CC = –0.05; F: beta, CC = 0.19; G: gamma, CC = 0.63). (H) Spatial correlations between the ACC BOLD-derived RSN map and RSN maps derived by all 1 Hz bands across the full LFP spectrum.
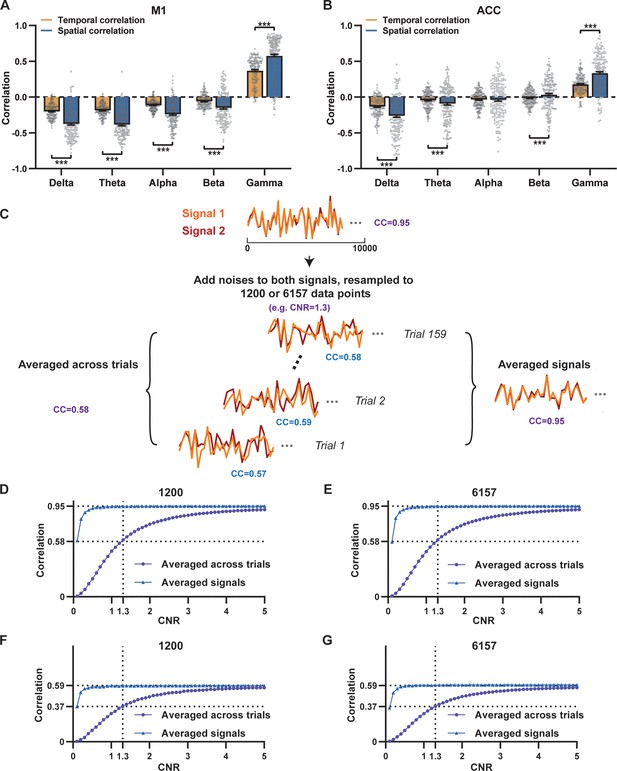
Disparity in spatial and temporal correlations persists after controlling for the noise effect.
(A,B) Comparison of scan-wise spatial and temporal correlations (paired t-tests across individual scans. ***: p<0.005). (C–G) Simulation to evaluate factors affecting apparent correlation values including contrast-to-noise ratio (CNR) and the number of data points. (C) Two fixed signals with a true correlation coefficient (CC) of 0.95 are simulated (10,000 data points) with random noise added at a given CNR level. This process is repeated 159 times (i.e. the number of scans in our study) for each CNR level. At each CNR, the CC is calculated based on either the averaged signals from all 159 trials (i.e. denoised data, triangle dots in D–G), or signals of individual trials (i.e. with-noise data, round dots in D–G) before averaging the resulting correlations across trials. (D) Simulated signals resampled to 1200 data points (equal to the number of time points used to calculate temporal correlations). (E) Simulated signals resampled to 6157 data points (equal to the number of brain voxels used to calculate spatial correlations). Importantly, we can replicate the difference between true (R=0.95) and apparent (R=0.58) correlations obtained from denoised data and with-noise data, respectively, when CNR = 1.3. Therefore, we estimate that the CNR of our blood-oxygen-level-dependent (BOLD) data is ~1.3. (F,G) The same process as C–E with the true correlation of 0.59. This true correlation value is obtained by iteratively setting different true correlation values and searching for the one that provides the trial-wise apparent correlation of 0.37, as measured by the gamma-BOLD temporal correlation in our real data shown in (A), at CNR = 1.3. (F) Simulated signals resampled to 1200 data points. (G) Simulated signals resampled to 6157 data points.
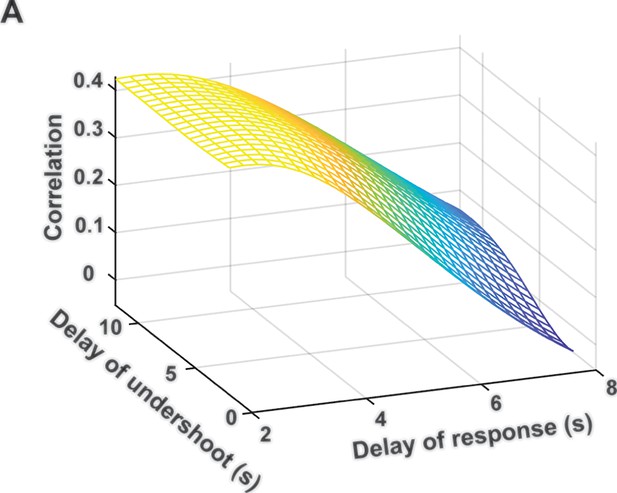
Blood-oxygen-level-dependent (BOLD)-gamma power correlations in the two-dimensional space of hemodynamic response function (HRF) parameters.
The maximal correlation value is close to that obtained with the HRF used in the study.
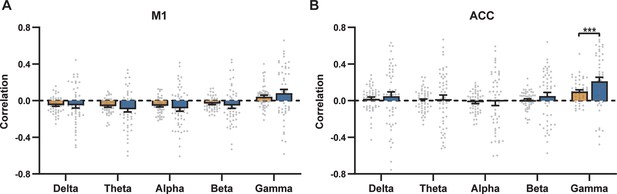
Comparison of scan-wise spatial and temporal correlations for (A) primary motor cortex (M1) and (B) anterior cingulate cortex (ACC) in awake rats.
Paired t-tests across individual scans. # of scans = 50. ***: p<0.005.
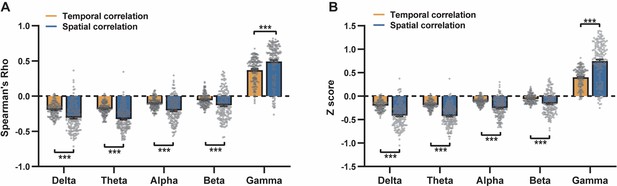
Disparity in spatial and temporal relationships between resting-state local field potential (LFP) and blood-oxygen-level-dependent (BOLD) signals quantified using alternative methods.
(A) Spearman’s rank correlation coefficient. (B) z-Transformation prior to comparison of Pearson correlation coefficient. Paired t-tests across individual scans. ***: p<0.005.
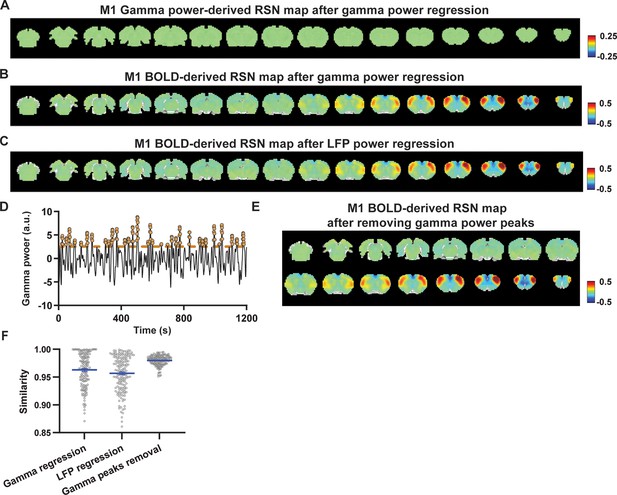
Impact of removing the electrophysiology signal on primary motor cortex (M1) blood-oxygen-level-dependent (BOLD)-derived resting-state brain network (RSN) spatial patterns.
(A) RSN map derived from gamma power after regressing out the hemodynamic response function (HRF)-convolved gamma power in the M1 from resting-state functional magnetic resonance imaging (rsfMRI) signals of all brain voxels. (B) M1 BOLD-derived RSN map (i.e. M1 seedmap) after voxel-wise regression of the HRF-convolved gamma power from rsfMRI signals. (C) M1 BOLD-derived RSN map after voxel-wise regression of all five local field potential (LFP) band powers from rsfMRI signals using soft regression. (D) Peaks of HRF-convolved gamma power in one representative scan. (E) M1 BOLD-derived RSN map after removing 15% of rsfMRI time points corresponding to gamma peaks. (F) Spatial similarity of M1 BOLD-derived RSN maps before and after gamma power regression, regression of all LFP band powers, or gamma peak removal.
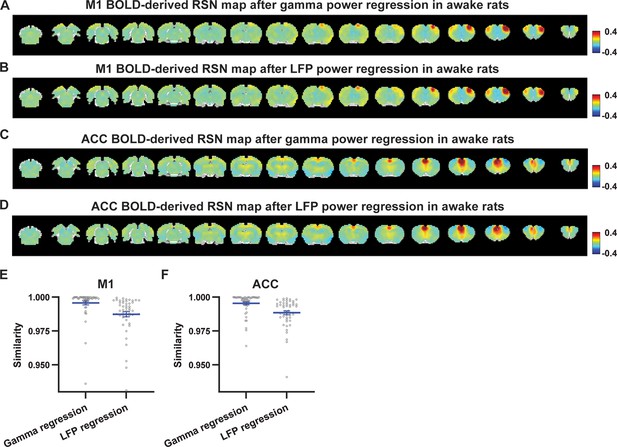
Impact of removing electrophysiology signals on primary motor cortex (M1) and anterior cingulate cortex (ACC) resting-state brain network (RSN) spatial patterns in awake animals.
(A) M1 blood-oxygen-level-dependent (BOLD)-derived RSN map (i.e. M1 seedmap) after the hemodynamic response function (HRF)-convolved gamma power in the M1 is voxel-wise regressed out from resting-state functional magnetic resonance imaging (rsfMRI) signals of all brain voxels. (B) M1 BOLD-derived RSN map after powers of all five local field potential (LFP) bands are voxel-wise regressed out from rsfMRI signals of all brain voxels using soft regression. (C) ACC BOLD-derived RSN map after the HRF-convolved gamma power in the ACC is voxel-wise regressed out from rsfMRI signals. (D) ACC BOLD-derived RSN map after powers of all five LFP bands are voxel-wise regressed out from rsfMRI signals using soft regression. (E) Spatial similarity of M1 BOLD-derived RSN maps before and after gamma power regression or regression of powers of all LFP bands. (F) Spatial similarity of ACC BOLD-derived RSN maps before and after gamma power regression or regression of powers of all LFP bands.
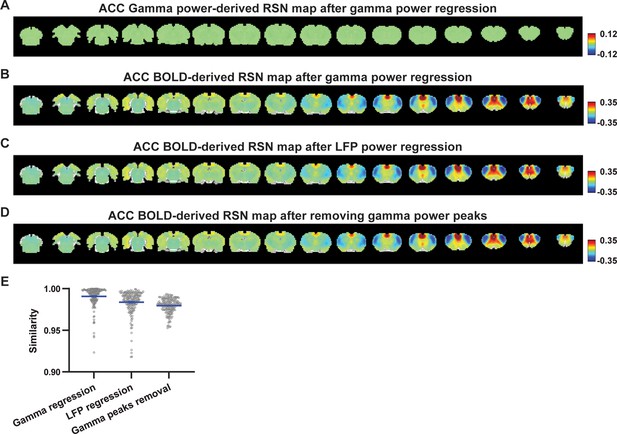
Impact of removing the electrophysiology signal on anterior cingulate cortex (ACC) blood-oxygen-level-dependent (BOLD)-derived resting-state brain network (RSN) spatial patterns.
(A) RSN map derived from gamma power after regressing out the hemodynamic response function (HRF)-convolved gamma power in the ACC from resting-state functional magnetic resonance imaging (rsfMRI) signals of all brain voxels. (B) ACC BOLD-derived RSN map (i.e. ACC seedmap) after voxel-wise regression of the HRF-convolved gamma power from rsfMRI signals. (C) ACC BOLD-derived RSN map after voxel-wise regression of all five local field potential (LFP) band powers from rsfMRI signals using soft regression. (D) ACC BOLD-derived RSN map after removing 15% of rsfMRI time points corresponding to gamma peaks. (E) Spatial similarity of ACC BOLD-derived RSN maps before and after gamma power regression, regression of all LFP band powers, or gamma peak removal.
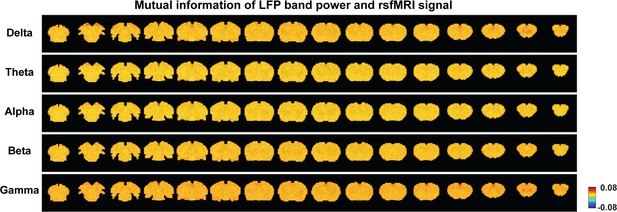
Mutual information between anterior cingulate cortex (ACC) band-limited local field potential (LFP) powers and brain-wide resting-state functional magnetic resonance imaging (rsfMRI) signals.
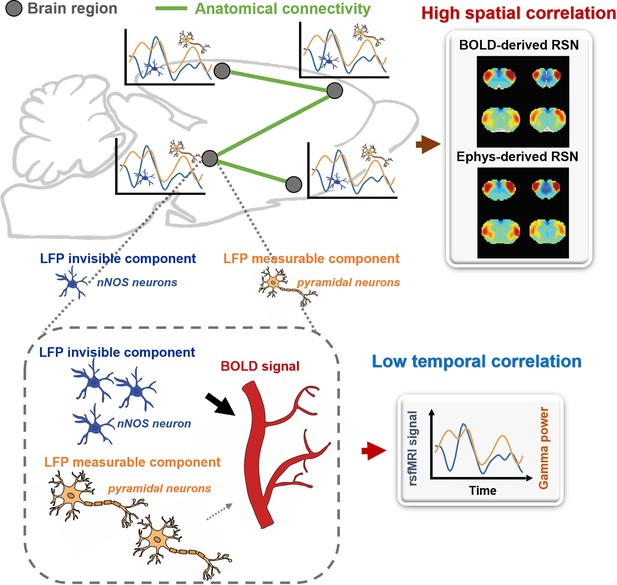
A theoretic model that can explain the disparity in spatial and temporal correlations between resting-state electrophysiology and functional magnetic resonance imaging (fMRI) signals.
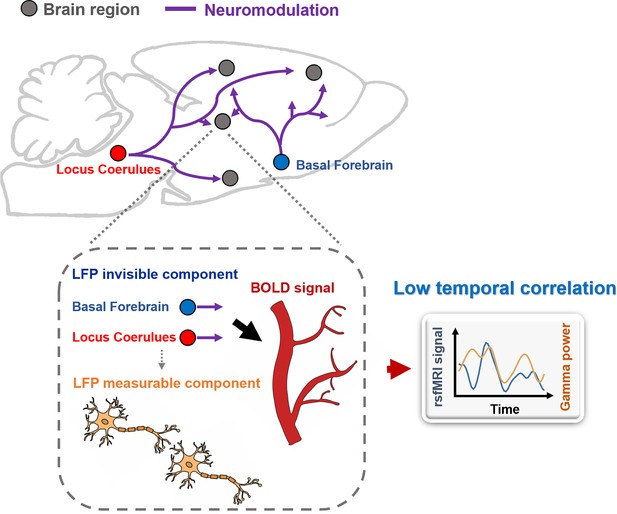
Schematic diagram illustrating that distal neuromodulation, which have strong vasoactive effects, can contribute to low temporal correlations between electrophysiology and resting-state functional magnetic resonance imaging (rsfMRI) signals.
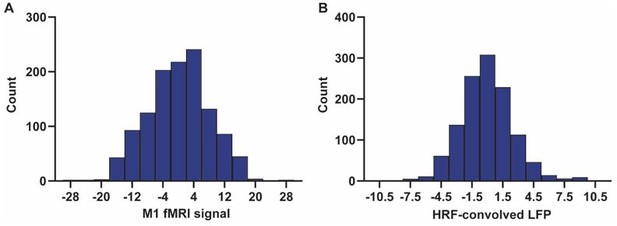