Semantical and geometrical protein encoding toward enhanced bioactivity and thermostability
Figures
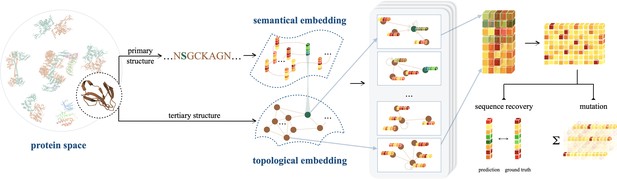
An illustration of ProtSSN that extracts the semantical and geometrical characteristics of a protein from its sequentially ordered global construction and spatially gathered local contacts with protein language models and equivariant graph neural networks.
The encoded hidden representation can be used for downstream tasks such as variants effect prediction that recognizes the impact of mutating a few sites of a protein on its functionality.

Number of trainable parameters and Spearman’s correlation on DTm, DDG, and ProteinGym v1, with the medium value located by the dashed lines.
Dot, cross, and diamond markers represent sequence-based, structure-based, and sequence-structure models, respectively.

Ablation study on ProteinGym v0 with different modular settings of ProtSSN.
Each record represents the average Spearman’s correlation of all assays. (a) Performance using different structure encoders: Equivariant Graph Neural Networks (EGNN) (orange) versus GCN/GAT (purple). (b) Performance using different node attributes: ESM2-embedded hidden representation (orange) versus one-hot encoding (purple). (c) Performance with varying numbers of EGNN layers. (d) Performance with different versions of ESM2 for sequence encoding. (e) Performance using different amino acid perturbation strategies during pre-training.
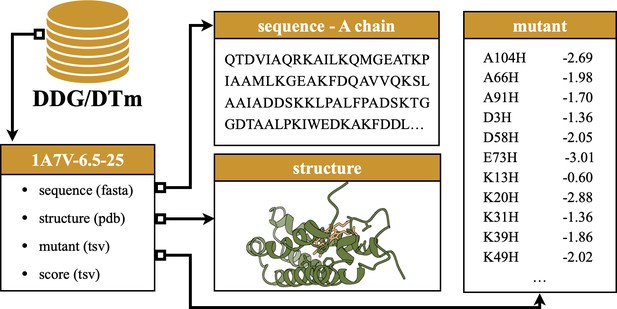
An example source record of the mutation assay.
The record is derived from DDG for the A chain of protein 1A7V, experimented at pH = 6.5 and degree at 25°C.
Tables
Spearman’s correlation of variant effect prediction by with zero-shot methods on DTm, DDG, and ProteinGym v1.
Model | Version | # Params | DTm | DDG | ProteinGym v1 | |||||
---|---|---|---|---|---|---|---|---|---|---|
(million) | Activity | Binding | Expression | Organismal | Stability | Overall fitness | ||||
Sequence encoder | ||||||||||
RITA | Small | 30 | 0.122 | 0.143 | 0.294 | 0.275 | 0.337 | 0.327 | 0.289 | 0.304 |
Medium | 300 | 0.131 | 0.188 | 0.352 | 0.274 | 0.406 | 0.371 | 0.348 | 0.350 | |
Large | 680 | 0.213 | 0.236 | 0.359 | 0.291 | 0.422 | 0.374 | 0.383 | 0.366 | |
xlarge | 1,200 | 0.221 | 0.264 | 0.402 | 0.302 | 0.423 | 0.387 | 0.445 | 0.373 | |
ProGen2 | Small | 151 | 0.135 | 0.194 | 0.333 | 0.275 | 0.384 | 0.337 | 0.349 | 0.336 |
Medium | 764 | 0.226 | 0.214 | 0.393 | 0.296 | 0.436 | 0.381 | 0.396 | 0.380 | |
Base | 764 | 0.197 | 0.253 | 0.396 | 0.294 | 0.444 | 0.379 | 0.383 | 0.379 | |
Large | 2700 | 0.181 | 0.226 | 0.406 | 0.294 | 0.429 | 0.379 | 0.396 | 0.381 | |
xlarge | 6400 | 0.232 | 0.270 | 0.402 | 0.302 | 0.423 | 0.387 | 0.445 | 0.392 | |
ProtTrans | bert | 420 | 0.268 | 0.313 | - | - | - | - | - | - |
bert_bfd | 420 | 0.217 | 0.293 | - | - | - | - | - | - | |
t5_xl_uniref50 | 3000 | 0.310 | 0.365 | - | - | - | - | - | - | |
t5_xl_bfd | 3000 | 0.239 | 0.334 | - | - | - | - | - | - | |
Tranception | Small | 85 | 0.119 | 0.169 | 0.287 | 0.349 | 0.319 | 0.270 | 0.258 | 0.288 |
Medium | 300 | 0.189 | 0.256 | 0.349 | 0.285 | 0.409 | 0.362 | 0.342 | 0.349 | |
Large | 700 | 0.197 | 0.284 | 0.401 | 0.289 | 0.415 | 0.389 | 0.381 | 0.375 | |
ESM-1v | - | 650 | 0.279 | 0.266 | 0.390 | 0.268 | 0.431 | 0.362 | 0.476 | 0.385 |
ESM-1b | - | 650 | 0.271 | 0.343 | 0.428 | 0.289 | 0.427 | 0.351 | 0.500 | 0.399 |
ESM2 | t12 | 35 | 0.214 | 0.216 | 0.314 | 0.292 | 0.364 | 0.218 | 0.439 | 0.325 |
t30 | 150 | 0.288 | 0.317 | 0.391 | 0.328 | 0.425 | 0.305 | 0.510 | 0.392 | |
t33 | 650 | 0.330 | 0.392 | 0.425 | 0.339 | 0.415 | 0.338 | 0.523 | 0.419 | |
t36 | 3000 | 0.327 | 0.351 | 0.417 | 0.322 | 0.425 | 0.379 | 0.509 | 0.410 | |
t48 | 15,000 | 0.311 | 0.252 | 0.405 | 0.318 | 0.425 | 0.388 | 0.488 | 0.405 | |
CARP | - | 640 | 0.288 | 0.333 | 0.395 | 0.274 | 0.419 | 0.364 | 0.414 | 0.373 |
Structure encoder | ||||||||||
ESM-if1 | - | 142 | 0.395 | 0.409 | 0.368 | 0.392 | 0.403 | 0.324 | 0.624 | 0.422 |
Sequence + structure encoder | ||||||||||
MIF-ST | - | 643 | 0.400 | 0.406 | 0.390 | 0.323 | 0.432 | 0.373 | 0.486 | 0.401 |
SaProt | Masked | 650 | 0.382 | - | 0.459 | 0.382 | 0.485 | 0.371 | 0.583 | 0.456 |
Unmasked | 650 | 0.376 | 0.359 | 0.450 | 0.376 | 0.460 | 0.372 | 0.577 | 0.447 | |
ProtSSN (ours) | k20_h512 | 148 | 0.419 | 0.442 | 0.458 | 0.371 | 0.436 | 0.387 | 0.566 | 0.444 |
Ensemble | 1467 | 0.425 | 0.440 | 0.466 | 0.371 | 0.451 | 0.398 | 0.568 | 0.451 |
-
The top three are highlighted by first, second, and third.
Influence of folding strategies (AlphaFold2 and ESMFold) on prediction performance for structure-involved models.
DTm | DDG | ProteinGym-interaction | ProteinGym-catalysis | |||||||||
---|---|---|---|---|---|---|---|---|---|---|---|---|
AlphaFold2 | ESMFold | diff ↓ | AlphaFold2 | ESMFold | diff↓ | AlphaFold2 | ESMFold | diff↓ | AlphaFold2 | ESMFold | diff↓ | |
Avg. pLDDT | 90.86 | 83.22 | - | 95.19 | 86.03 | - | 82.86 | 65.69 | - | 85.80 | 73.45 | - |
ESM-if1 | 0.395 | 0.371 | 0.024 | 0.457 | 0.488 | –0.031 | 0.351 | 0.259 | 0.092 | 0.386 | 0.368 | 0.018 |
MIF-ST | 0.400 | 0.378 | 0.022 | 0.438 | 0.423 | –0.015 | 0.390 | 0.327 | 0.063 | 0.408 | 0.388 | 0.020 |
k30_h1280 | 0.384 | 0.370 | 0.014 | 0.396 | 0.390 | 0.006 | 0.398 | 0.373 | 0.025 | 0.443 | 0.439 | 0.004 |
k30_h768 | 0.359 | 0.356 | 0.003 | 0.378 | 0.366 | 0.012 | 0.400 | 0.374 | 0.026 | 0.442 | 0.436 | 0.006 |
k30_h512 | 0.413 | 0.399 | 0.014 | 0.408 | 0.394 | 0.014 | 0.400 | 0.372 | 0.028 | 0.447 | 0.442 | 0.005 |
k20_h1280 | 0.415 | 0.391 | 0.024 | 0.429 | 0.410 | 0.019 | 0.399 | 0.365 | 0.034 | 0.446 | 0.441 | 0.005 |
k20_h768 | 0.415 | 0.403 | 0.012 | 0.419 | 0.397 | 0.022 | 0.401 | 0.370 | 0.031 | 0.449 | 0.442 | 0.007 |
k20_h512 | 0.419 | 0.395 | 0.024 | 0.441 | 0.432 | 0.009 | 0.406 | 0.371 | 0.035 | 0.449 | 0.439 | 0.010 |
k10_h1280 | 0.406 | 0.391 | 0.015 | 0.426 | 0.411 | 0.015 | 0.396 | 0.365 | 0.031 | 0.440 | 0.434 | 0.006 |
k10_h768 | 0.400 | 0.391 | 0.009 | 0.414 | 0.400 | 0.014 | 0.379 | 0.349 | 0.030 | 0.431 | 0.421 | 0.010 |
k10_h512 | 0.383 | 0.364 | 0.019 | 0.424 | 0.414 | 0.010 | 0.389 | 0.364 | 0.025 | 0.440 | 0.432 | 0.008 |
-
The top three are highlighted by first, second, and third.
Variant effect prediction on ProteinGym v0 with both zero-shot and few-shot methods.
Results are retrieved from Notin et al., 2022a.
Model | Type | # Params | (by mutation depth) | (by MSA depth) | (by taxon) | |||||||
---|---|---|---|---|---|---|---|---|---|---|---|---|
(million) | Single | Double | All | Low | Medium | High | Prokaryote | Human | Eukaryote | Virus | ||
Few-shot methods | ||||||||||||
SiteIndep | Single | - | 0.378 | 0.322 | 0.378 | 0.431 | 0.375 | 0.342 | 0.343 | 0.375 | 0.401 | 0.406 |
EVmutation | Single | - | 0.423 | 0.401 | 0.423 | 0.406 | 0.403 | 0.484 | 0.499 | 0.396 | 0.429 | 0.381 |
Wavenet | Single | - | 0.399 | 0.344 | 0.400 | 0.286 | 0.404 | 0.489 | 0.492 | 0.373 | 0.442 | 0.321 |
GEMME | Single | - | 0.460 | 0.397 | 0.463 | 0.444 | 0.446 | 0.520 | 0.505 | 0.436 | 0.479 | 0.451 |
DeepSequence | Single | - | 0.411 | 0.358 | 0.416 | 0.386 | 0.391 | 0.505 | 0.497 | 0.396 | 0.461 | 0.332 |
Ensemble | - | 0.433 | 0.394 | 0.435 | 0.386 | 0.411 | 0.534 | 0.522 | 0.405 | 0.480 | 0.361 | |
EVE | Single | - | 0.451 | 0.406 | 0.452 | 0.417 | 0.434 | 0.525 | 0.518 | 0.411 | 0.469 | 0.436 |
Ensemble | - | 0.459 | 0.409 | 0.459 | 0.424 | 0.441 | 0.532 | 0.526 | 0.419 | 0.481 | 0.437 | |
MSA-Transfomer | Single | 100 | 0.405 | 0.358 | 0.426 | 0.372 | 0.415 | 0.500 | 0.506 | 0.387 | 0.468 | 0.379 |
Ensemble | 500 | 0.440 | 0.374 | 0.440 | 0.387 | 0.428 | 0.513 | 0.517 | 0.398 | 0.467 | 0.406 | |
Tranception-R | Single | 700 | 0.450 | 0.427 | 0.453 | 0.442 | 0.438 | 0.500 | 0.495 | 0.424 | 0.485 | 0.434 |
TranceptEVE | Single | 700 | 0.481 | 0.445 | 0.481 | 0.454 | 0.465 | 0.542 | 0.539 | 0.447 | 0.498 | 0.461 |
Zero-shot methods | ||||||||||||
RITA | Ensemble | 2,210 | 0.393 | 0.236 | 0.399 | 0.350 | 0.414 | 0.407 | 0.391 | 0.391 | 0.405 | 0.417 |
ESM-1v | Ensemble | 3,250 | 0.416 | 0.309 | 0.417 | 0.390 | 0.378 | 0.536 | 0.521 | 0.439 | 0.423 | 0.268 |
ProGen2 | Ensemble | 10,779 | 0.421 | 0.312 | 0.423 | 0.384 | 0.421 | 0.467 | 0.497 | 0.412 | 0.459 | 0.373 |
ProtTrans | Ensemble | 6,840 | 0.417 | 0.360 | 0.413 | 0.372 | 0.395 | 0.492 | 0.498 | 0.419 | 0.400 | 0.322 |
ESM2 | Ensemble | 18,843 | 0.415 | 0.316 | 0.413 | 0.391 | 0.381 | 0.509 | 0.508 | 0.456 | 0.461 | 0.213 |
SaProt | Ensemble | 1,285 | 0.447 | 0.368 | 0.450 | 0.456 | 0.410 | 0.544 | 0.534 | 0.464 | 0.460 | 0.334 |
ProtSSN | Ensemble | 1,476 | 0.433 | 0.381 | 0.433 | 0.406 | 0.402 | 0.532 | 0.530 | 0.436 | 0.491 | 0.290 |
-
The top three are highlighted by first, second, and third.
Average Spearman’s correlation of variant effect prediction on DTm and DDG for zero-shot methods with model ensemble.
The values within () indicate the standard deviation of bootstrapping.
Model | # Params (million) | DTm | DDG |
---|---|---|---|
RITA | 2210 | 0,195(0.045) | 0.255(0.061) |
ESM-1v | 3250 | 0.300(0.036) | 0.310(0.054) |
Tranception | 1085 | 0.202(0.039) | 0.277(0.062) |
ProGen2 | 10,779 | 0.293(0.042) | 0.282(0.063) |
ProtTrans | 6840 | 0.323(0.039) | 0.389(0.059) |
ESM2 | 18,843 | 0.346(0.035) | 0.383(0.55) |
SaProt | 1285 | 0.392(0.040) | 0.415(0.061) |
ProtSSN | 1476 | 0.425(0.033) | 0.440(0.057) |
Statistical summary of DTm and DDG.
pH range | DTm | DDG | ||||
---|---|---|---|---|---|---|
# Assays | Avg. # mut | Avg. AA | # Assays | Avg. # mut | Avg.AA | |
Acid (pH < 7) | 29 | 29.1 | 272.8 | 21 | 22.6 | 125.3 |
Neutral (pH = 7) | 14 | 37.4 | 221.2 | 10 | 23.1 | 78.3 |
Alkaline (pH > 7) | 23 | 50.1 | 233.3 | 5 | 24.6 | 101.4 |
Sum | 66 | 38.2 | 221.2 | 36 | 23.0 | 108.8 |
Details of zero-shot baseline models.
Model | Type | Description | Source code | |
---|---|---|---|---|
Seq | Struct | |||
RITA (Hesslow et al., 2022) | ✓ | A generative protein language model with billion-level parameters | https://github.com/lightonai/RITA (Hesslow and Poli, 2023) | |
ProGen2 (Nijkamp et al., 2023) | ✓ | A generative protein language model with billion-level parameters | https://github.com/salesforce/progen (Nijkamp, 2022) | |
ProtTrans (Elnaggar et al., 2021) | ✓ | Transformer-based models trained on large protein sequence corpus | https://github.com/agemagician/ProtTrans (Elnaggar and Heinzinger, 2025) | |
Tranception (Notin et al., 2022a) | ✓ | An autoregressive model for variant effect prediction with retrieve machine | https://github.com/OATML-Markslab/Tranception (Notin, 2023) | |
CARP (Yang et al., 2024) | ✓ | Pretrained CNN protein sequence masked language models of various sizes | https://github.com/microsoft/protein-sequence-models (microsoft, 2024) | |
ESM-1b (Rives et al., 2021) | ✓ | A masked language model-based pre-train method with various pre-training dataset and positional embedding strategies | https://github.com/facebookresearch/esm (facebookresearch, 2023) | |
ESM-1v (Meier et al., 2021) | ✓ | |||
ESM2 (Lin et al., 2023) | ✓ | |||
ESM-if1 (Hsu et al., 2022) | ✓ | An inverse folding method with mask language modeling and geometric vector perception (Jing et al., 2020) | ||
MIF-ST (Yang et al., 2023) | ✓ | ✓ | Pretrained masked inverse folding models with sequence pretraining transfer | https://github.com/microsoft/protein-sequence-models |
SaProt (Su et al., 2023) | ✓ | ✓ | Structure-aware vocabulary for protein language modeling with FoldSeek | https://github.com/westlake-repl/SaProt (Su and fajieyuan, 2025) |
Spearmar’s score of the number of MSA sequences and the model’s performance.
Model Name | Model Type | Spearmanr's score |
---|---|---|
EVE (ensemble) | Alignment-based model | 0.239 |
GEMME | Alignment-based model | 0.207 |
TranceptEVE L | Hybrid - Alignment & PLM | 0.237 |
MSA Transformer (ensemble) | Hybrid - Alignment & PLM | 0.262 |
ESM-IF1 | Inverse folding model | 0.346 |
ESM2 (650M) | Protein language model | 0.297 |
ESM-1v (ensemble) | Protein language model | 0.372 |
SaProt (650M) | Hybrid - Structure & PLM | 0.260 |
ProtSSN (ensemble) | Hybrid - Structure & PLM | 0.217 |
Additional files
-
MDAR checklist
- https://cdn.elifesciences.org/articles/98033/elife-98033-mdarchecklist1-v1.pdf
-
Source code 1
The source code .ZIP file contains the complete implementation of model training and evaluation, the associated processed datasets, and the .README document which provides a general instruction.
- https://cdn.elifesciences.org/articles/98033/elife-98033-code1-v1.zip