Derivation and internal validation of prediction models for pulmonary hypertension risk assessment in a cohort inhabiting Tibet, China
Figures
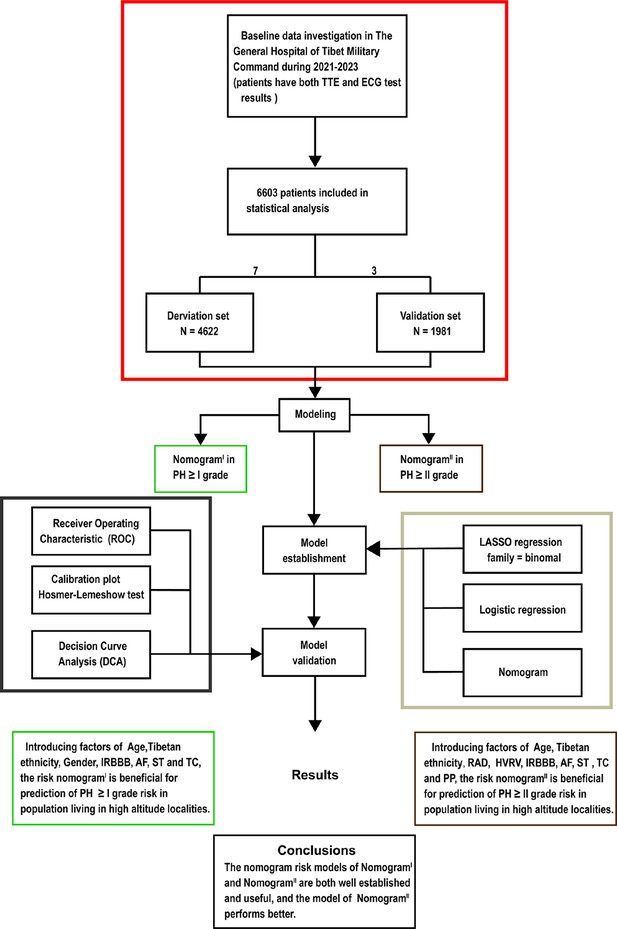
Flow diagram.
Based on the exclusion and inclusion criteria, 6603 patients were included in this study. Patients were divided into a validation set and a derivation set randomly following a 7:3 ratio. Pulmonary hypertension, PH; right axis deviation, RAD; high voltage in the right ventricle, HVRV; incomplete right bundle branch block, IRBBB; atrial fibrillation, AF; sinus tachycardia, ST; T wave changes, TC; pulmonary P waves, PP.
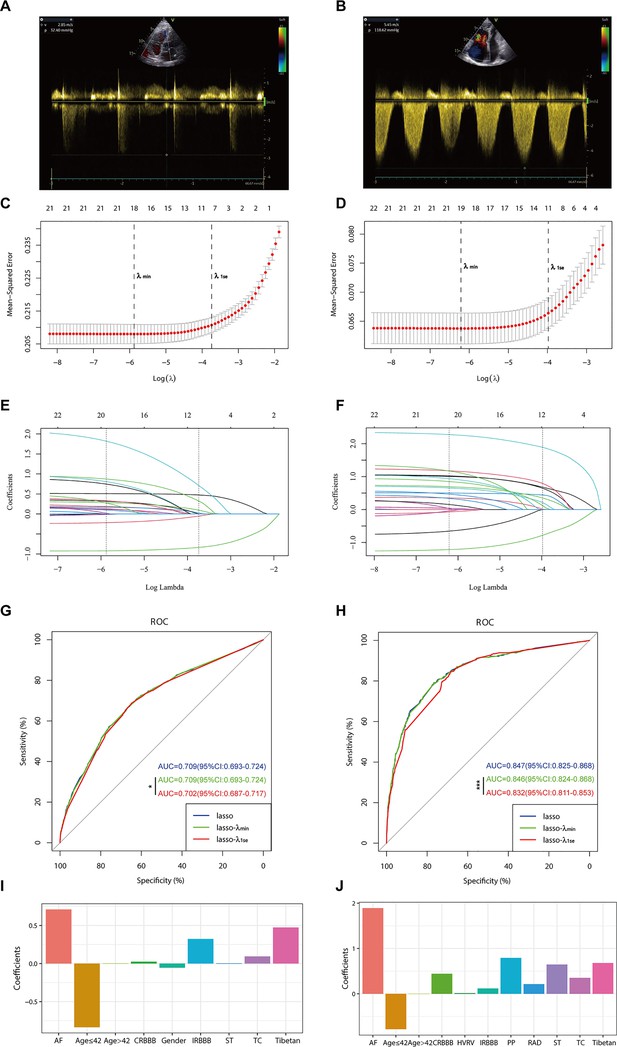
Illustrates the optimal predictive variables as determined by the least absolute shrinkage and selection operator (LASSO) binary logistic regression model.
Panels A and B depict the measurement of tricuspid regurgitation spectra via transthoracic echocardiography in patients with Grade I pulmonary hypertension (PH) (A) and Grade III PH (B). Panels C to J demonstrate the identification of the optimal penalisation coefficient lambda (λ) in the LASSO model using 10-fold cross-validation for the PH ≥ I grade group (C) and the PH ≥ II grade group (D). The dotted line on the left (λ_min) represents the value of the harmonic parameter log(λ) at which the model’s error is minimised, and the dotted line on the right (λ_1se) indicates the value of the harmonic parameter log(λ) at which the model’s error is minimal minus 1 standard deviation. The LASSO coefficient profiles of 22 predictive factors for the PH ≥ I grade group (E) and the PH ≥ II grade group (F) show that as the value of λ decreased, the degree of model compression increased, enhancing the model’s ability to select significant variables. Receiver operating characteristic (ROC) curves were constructed for three models (LASSO, LASSO-λ_min, and LASSO-λ_1se) in both the PH ≥ I grade group (G) and the PH ≥ II grade group (H). Histograms depict the final variables selected according to λ_1se and their coefficients for the PH ≥ I grade group (I) and the PH ≥ II grade group (J). Asterisks denote levels of statistical significance: *p < 0.05, **p < 0.01, ***p < 0.001.
-
Figure 2—source data 1
Raw data of Figure 2.
- https://cdn.elifesciences.org/articles/98169/elife-98169-fig2-data1-v1.zip
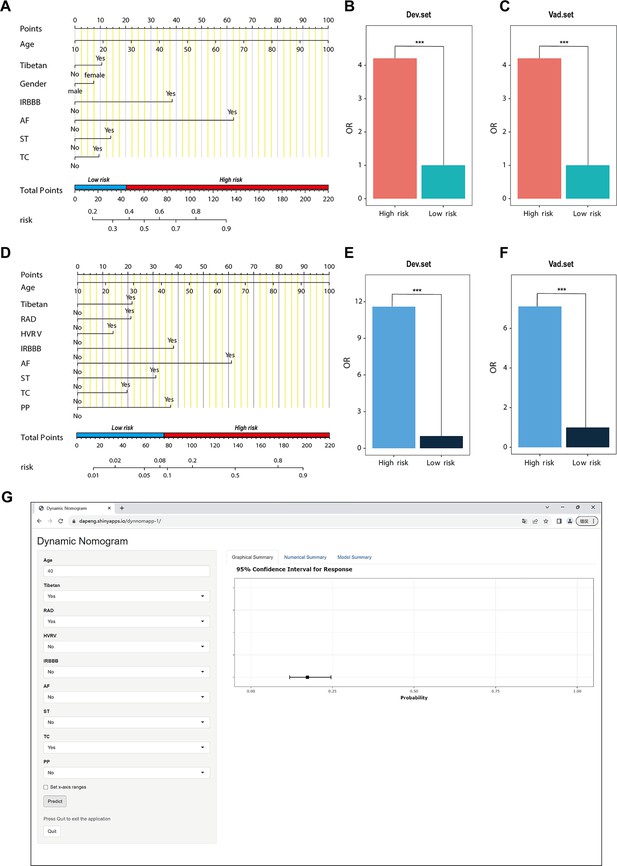
Nomogram for predicting pulmonary hypertension (PH) and risk stratification based on total score.
(A–C) NomogramI for the prediction of PH ≥ I grade in the PH ≥ I grade group. Points for each independent factor are summed to calculate total points, determining the corresponding ‘risk’ level. Patients were divided into ‘High-risk’ and ‘Low-risk’ subgroups according to the cut-off of the total points (A). Histograms illustrate the odds ratio (OR) comparing the ‘High-risk’ group to the ‘Low-risk’ group in the derivation set (B) and validation set (C). (D–F) NomogramII for predicting PH ≥ II grade within the PH ≥ II grade group: Similarly, points from each independent factor are totalled, and the corresponding ‘risk’ level is ascertained. Patients are divided into ‘High-risk’ and ‘Low-risk’ groups based on the cut-off value of the total points (D). Histograms display the OR for the ‘High-risk’ group compared to the ‘Low-risk’ group in the derivation (E) and validation set (F). ***p < 0.001. (G) Screenshot of dynamic NomogramII’s web page.
-
Figure 3—source data 1
The raw data and R software code of Figure 3.
- https://cdn.elifesciences.org/articles/98169/elife-98169-fig3-data1-v1.zip
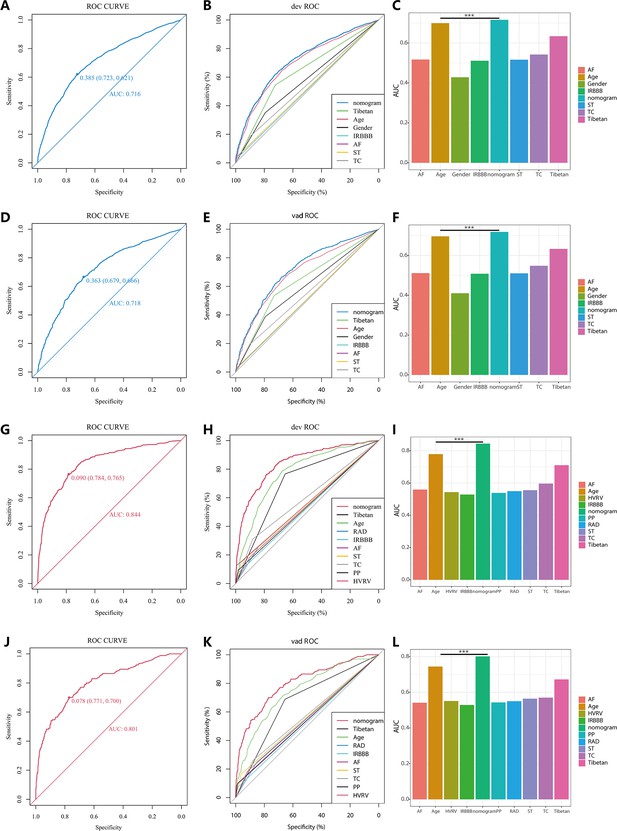
Receiver operating characteristic (ROC) curves and area under the curve (AUC) for NomogramI in pulmonary hypertension (PH) ≥ I and NomogramII in PH ≥ II grade groups.
In the PH ≥ I grade group, the ROC and corresponding AUC of NomogramI and independent factors in the derivation set (A–C) and validation set (D–F). In the PH ≥ II grade group, the ROC and corresponding AUC of NomogramII and independent factors in the derivation set (G–I) and validation set (J–L).
-
Figure 4—source data 1
The raw data and R software code of Figure 4.
- https://cdn.elifesciences.org/articles/98169/elife-98169-fig4-data1-v1.zip
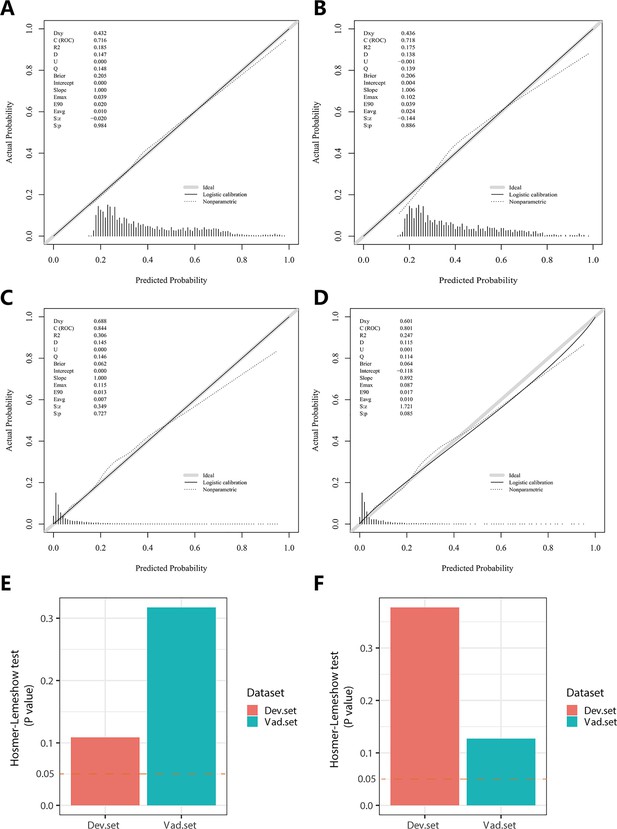
Calibration plots and Hosmer–Lemeshow test results for NomogramI in pulmonary hypertension (PH) ≥ I and NomogramII in PH ≥ II grade groups.
In the PH ≥ I grade group, the calibration plots of NomogramI in the derivation set (A) and the validation set (B). In the PH ≥ II grade group, the calibration plots of NomogramII in the derivation set (C) and the validation set (D). (E) In the PH ≥ I grade group, Hosmer–Lemeshow test results for NomogramI in the derivation set and the validation set. (F) In the PH ≥ II grade group, Hosmer–Lemeshow test results for NomogramII in the derivation set and the validation set.
-
Figure 5—source data 1
The raw data and R software code of Figure 5.
- https://cdn.elifesciences.org/articles/98169/elife-98169-fig5-data1-v1.zip
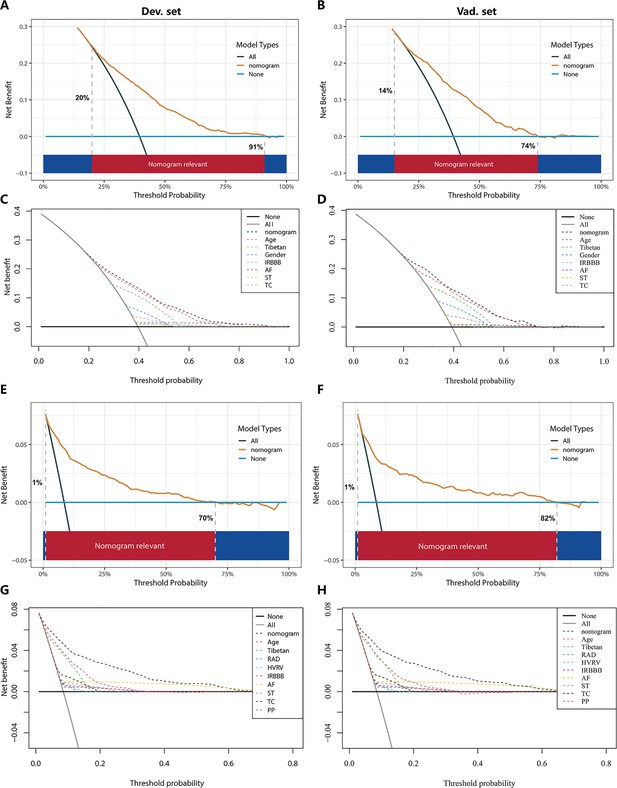
Decision curve analysis (DCA) for NomogramI in the pulmonary hypertension (PH) ≥ I grade and NomogramII in the PH ≥ II grade group.
In the PH ≥ I grade group, the DCAs of NomogramI and independent factors in the derivation (A, C) and validation set (B, D). In the PH ≥ II grade group, the DCAs of NomogramII and independent factors in the derivation (E, G) and validation set (F, H).
-
Figure 6—source data 1
The raw data and R software code of Figure 6.
- https://cdn.elifesciences.org/articles/98169/elife-98169-fig6-data1-v1.zip
Tables
Baseline characteristics of individuals in the derivation and validation sets.
Variable | Derivation set (n = 4622) | Validation set (n = 1981) | p | ||
---|---|---|---|---|---|
Age Total (mean ± SD) | 42.43 ± 16.93 | 42.05 ± 16.41 | 0.390 | ||
Age ≤42, n (%) | 2619 (56.66) | 1135 (57.29) | |||
Age >42, n (%) | 2003 (43.34) | 846 (42.71) | 0.635 | ||
Tibetan, n (%) | 0.538 | ||||
No | 2856 (61.79) | 1240 (62.59) | |||
Yes | 1766 (38.21) | 741 (37.41) | |||
Gender, n (%) | 0.260 | ||||
Female | 1219 (26.37) | 549 (27.71) | |||
Male | 3403 (73.63) | 1432 (72.29) | |||
RAD, n (%) | 0.141 | ||||
No | 3833 (82.93) | 1672 (84.40) | |||
Yes | 789 (17.07) | 309 (15.60) | |||
CR, n (%) | 0.387 | ||||
No | 4000 (86.54) | 1730 (87.33) | |||
Yes | 622 (13.46) | 251 (12.67) | |||
CCR, n (%) | 0.402 | ||||
No | 3994 (86.41) | 1727 (87.18) | |||
Yes | 628 (13.59) | 254 (12.82) | |||
HVRV, n (%) | 0.102 | ||||
No | 4151 (89.81) | 1805 (91.12) | |||
Yes | 471 (10.19) | 176 (8.88) | |||
IRBBB, n (%) | 0.573 | ||||
No | 4547 (98.38) | 1945 (98.18) | |||
Yes | 75 (1.62) | 36 (1.82) | |||
CRBBB, n (%) | 0.945 | ||||
No | 4444 (96.15) | 1904 (96.11) | |||
Yes | 178 (3.85) | 77 (3.89) | |||
AF, n (%) | 0.594 | ||||
No | 4551 (98.46) | 1954 (98.64) | |||
Yes | 71 (1.54) | 27 (1.36) | |||
SA, n (%) | 0.243 | ||||
No | 4247 (91.89) | 1837 (92.73) | |||
Yes | 375 (8.11) | 144 (7.27) | |||
ST, n (%) | 0.910 | ||||
No | 4395 (95.09) | 1885 (95.15) | |||
Yes | 227 (4.91) | 96 (4.85) | |||
SB, n (%) | 0.345 | ||||
No | 4245 (91.84) | 1833 (92.53) | |||
Yes | 377 (8.16) | 148 (7.47) | |||
TC, n (%) | 0.769 | ||||
No | 4003 (86.61) | 1721 (86.88) | |||
Yes | 619 (13.39) | 260 (13.12) | |||
STC, n (%) | 0.415 | ||||
No | 4399 (95.18) | 1876 (94.70) | |||
Yes | 223 (4.82) | 105 (5.30) | |||
APB, n (%) | 0.219 | ||||
No | 4587 (99.24) | 1960 (98.94) | |||
Yes | 35 (0.76) | 21 (1.06) | |||
JPB, n (%) | 0.425 | ||||
No | 4603 (99.59) | 1970 (99.44) | |||
Yes | 19 (0.41) | 11 (0.56) | |||
VPB, n (%) | 0.844 | ||||
No | 4580 (99.09) | 1962 (99.04) | |||
Yes | 42 (0.91) | 19 (0.96) | |||
PP, n (%) | 0.439 | ||||
No | 4507 (97.51) | 1938 (97.83) | |||
Yes | 115 (2.49) | 43 (2.17) | |||
CLBBB, n (%) | 0.757 | ||||
No | 4610 (99.74) | 1975 (99.70) | |||
Yes | 12 (0.26) | 6 (0.30) | |||
IAB, n (%) | 0.910 | ||||
No | 4556 (98.57) | 1952 (98.54) | |||
Yes | 66 (1.43) | 29 (1.46) | |||
PH ≥ I grade, n (%) | 0.820 | ||||
No | 2793 (60.43) | 1203 (60.73) | |||
Yes | 1829 (39.57) | 778 (39.27) | |||
PH ≥ II grade, n (%) | 0.962 | ||||
No | 4227 (91.45) | 1811 (91.42) | |||
Yes | 395 (8.55) | 170 (8.58) |
-
Table 1—source data 1
The raw data of Table 1.
- https://cdn.elifesciences.org/articles/98169/elife-98169-table1-data1-v1.zip
Risk factors for pulmonary hypertension (PH) ≥ I grade in the derivation set.
Variable | β-Coefficient | OR (95% CI) | p | |
---|---|---|---|---|
Tibetan | 0.34 | 1.40 (1.23–1.60) | <0.001 | |
Gender | −0.3 | 0.74 (0.65–0.84) | <0.001 | |
Age | 0.034 | 1.03 (1.03–1.04) | <0.001 | |
IRBBB | 1.106 | 3.02 (1.96–4.67) | <0.001 | |
AF | 1.431 | 4.18 (2.19–7.97) | <0.001 | |
ST | 0.369 | 1.45 (1.14–1.84) | 0.003 | |
TC | 0.306 | 1.36 (1.16–1.59) | <0.001 |
-
Table 2—source data 1
Raw data of Table 2.
- https://cdn.elifesciences.org/articles/98169/elife-98169-table2-data1-v1.zip
Risk factors for pulmonary hypertension (PH) ≥ II grade in the derivation set.
Variable | β-Coefficient | OR (95% CI) | p | |
---|---|---|---|---|
Tibetan | 0.689 | 1.99 (1.55–2.57) | <0.001 | |
Age | 0.042 | 1.04 (1.03–1.05) | <0.001 | |
RAD | 0.751 | 2.12 (1.56–2.88) | <0.001 | |
HVRV | 0.486 | 1.63 (1.14–2.31) | 0.007 | |
IRBBB | 1.512 | 4.53 (2.77–7.42) | <0.001 | |
AF | 2.102 | 8.18 (5.13–13.05) | <0.001 | |
ST | 1.247 | 3.48 (2.58–4.70) | <0.001 | |
TC | 0.592 | 1.81 (1.44–2.27) | <0.001 | |
PP | 1.486 | 4.42 (2.96–6.61) | <0.001 |
-
Table 3—source data 1
The raw data of Table 3.
- https://cdn.elifesciences.org/articles/98169/elife-98169-table3-data1-v1.zip