Decoding the physics of observed actions in the human brain
Figures
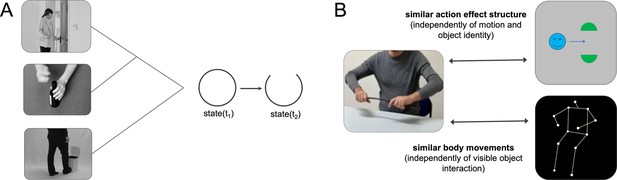
Schematic illustration of action effect structures and cross-decoding approach.
(A) Simplified schematic illustration of the action effect structure of ‘opening’. Action effect structures encode the specific interplay of temporospatial object relations that are characteristic for an action type independently of the concrete object (e.g. a state change from closed to open). (B) Cross-decoding approach to isolate representations of action effect structures and body movements. Action effect structure representations were isolated by training a classifier to discriminate neural activation patterns associated with actions (e.g. ‘breaking a stick’) and testing the classifier on its ability to discriminate activation patterns associated with corresponding abstract action animations (e.g. ‘dividing’). Body movement representations were isolated by testing the classifier trained with actions on activation patterns of corresponding point-light-display (PLD) stick figures.
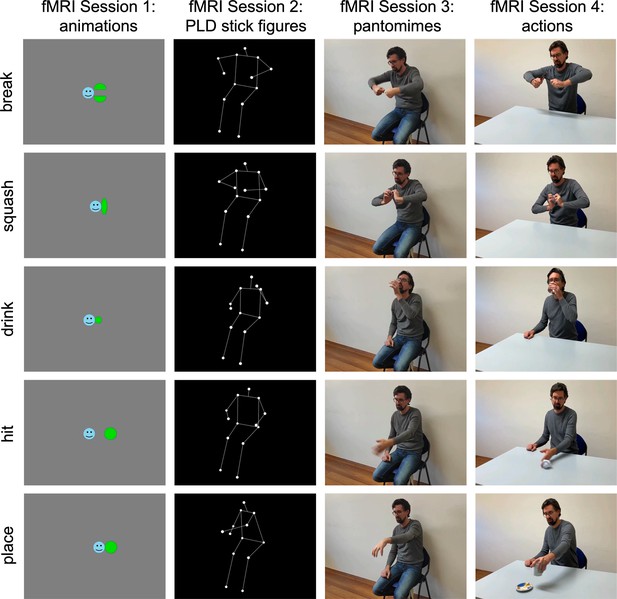
Experimental design.
In four fMRI sessions, participants observed 2-s-long videos of five actions and corresponding animations, point-light-display (PLD) stick figures, and pantomimes. For each stimulus type, eight perceptually variable exemplars were used (e.g. different geometric shapes, persons, viewing angles, and left–right flipped versions of the videos). A fixed order of sessions from abstract animations to naturalistic actions was used to minimize memory and imagery effects.
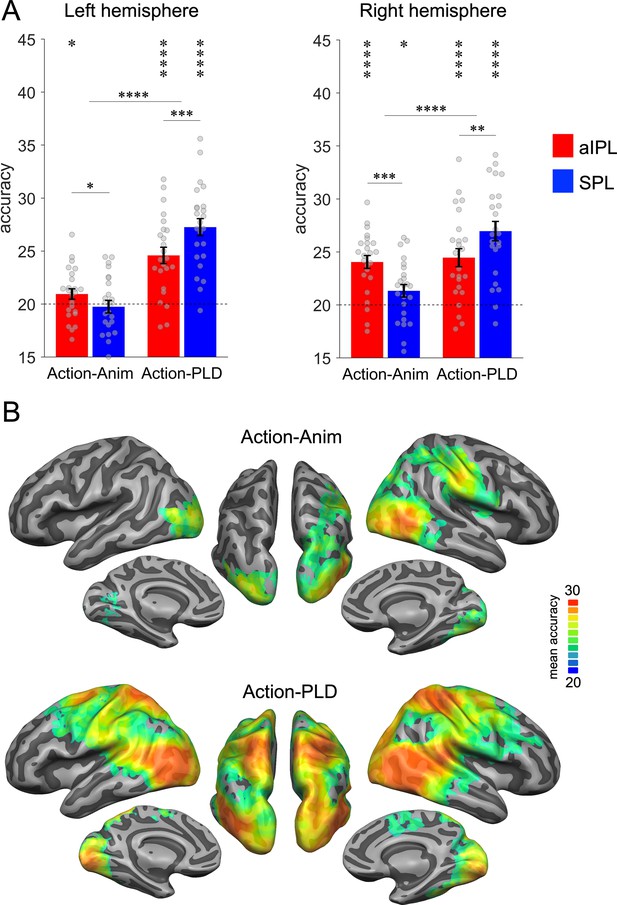
Cross-decoding of action effect structures (action-animation) and body movements (action-PLD).
(A) Region of interest (ROI) analysis in left and right anterior inferior parietal lobe (aIPL) and superior parietal lobe (SPL) (Brodmann areas 40 and 7, respectively; see Methods for details). Decoding of action effect structures (action-animation cross-decoding) is stronger in aIPL than in SPL, whereas decoding of body movements (action-PLD cross-decoding) is stronger in SPL than in aIPL. Asterisks indicate FDR-corrected significant decoding accuracies above chance (*p < 0.05, **p < 0.01, ***p < 0.001, ****p < 0.0001). Error bars indicate SEM (N=24). (B) Mean accuracy whole-brain maps thresholded using Monte-Carlo correction for multiple comparisons (voxel threshold p = 0.001, corrected cluster threshold p = 0.05). Action-animation cross-decoding is stronger in the right hemisphere and reveals additional representations of action effect structures in lateral occipitotemporal cortex (LOTC).
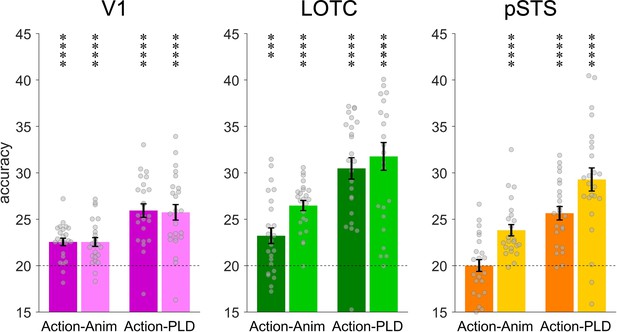
Cross-decoding of action effect structures (action-animation) and body movements (action-PLD) in left and right LOTC, pSTS, and V1 (respectively; see Methods for details on region of interest [ROI] definition).
Dark tones show effects in left ROIs, light tones show effects in right ROIs. Asterisks indicate FDR-corrected significant decoding accuracies above chance (***p < 0.001, ****p < 0.0001). Error bars indicate SEM (N=24).
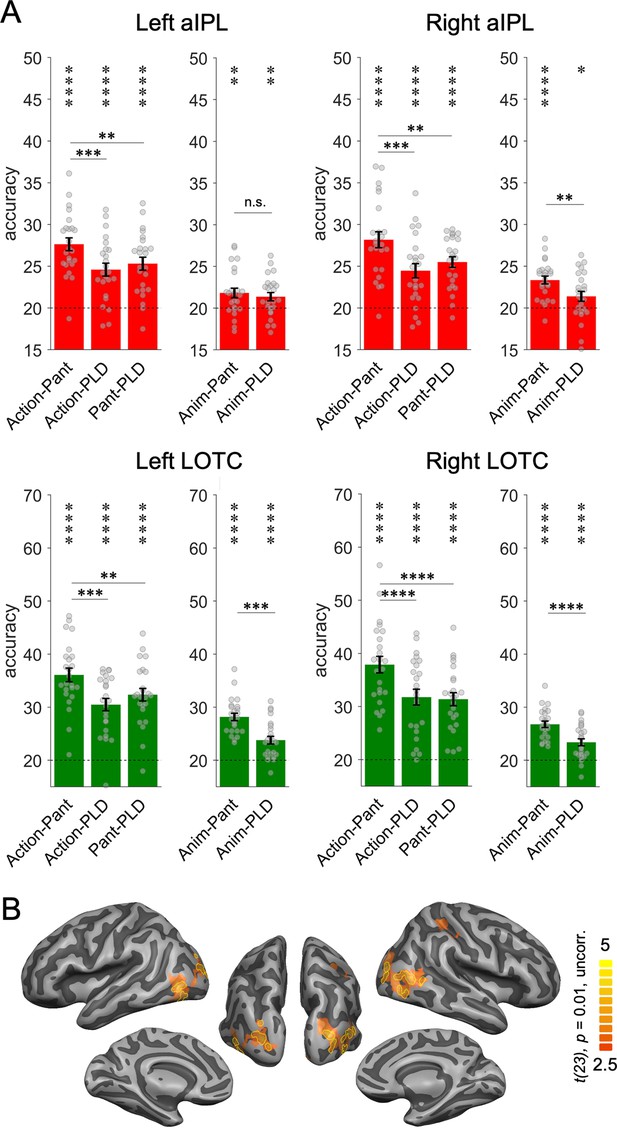
Cross-decoding of implied action effect structures.
(A) Region of interest (ROI) analysis. Cross-decoding schemes involving pantomimes but not point-light-displays (PLDs) (action-pantomime, animation-pantomime) reveal stronger effects in right anterior inferior parietal lobe (aIPL) than cross-decoding schemes involving PLDs (action-PLD, pantomime-PLD, animation-PLD), suggesting that action effect structure representations in right aIPL respond to implied object manipulations in pantomime irrespective of visuospatial processing of observable object state changes. Same conventions as in Figure 3. (B) Conjunction of the contrasts action-pantomime versus action-PLD, action-pantomime versus pantomime-PLD, and animation-pantomime versus animation-PLD. Uncorrected t-map thresholded at p = 0.01; yellow outlines indicate clusters surviving Monte-Carlo correction for multiple comparisons (voxel threshold p = 0.001, corrected cluster threshold p = 0.05).
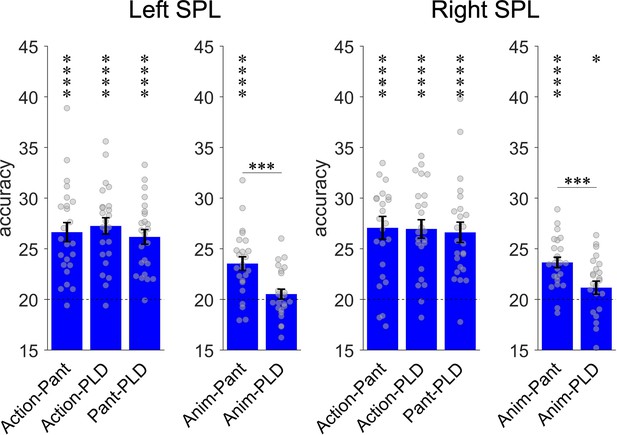
Cross-decoding of implied action effect structures in superior parietal lobe (SPL).
Implied action effect structures should reveal higher decoding accuracies in cross-decoding schemes involving pantomimes but not point-light-displays (PLDs) (action-pantomime, animation-pantomime) as compared to cross-decoding schemes involving PLDs (action-PLD, pantomime-PLD, animation-PLD). In both left and right SPL, there were no differences for the comparisons of Action-Pant with Action-PLD and Pant-PLD, whereas there was stronger decoding for Anim-Pant versus Anim-PLD. This pattern of results is not straightforward to explain: First, the equally strong decoding for Action-Pant, Action-PLD, and Pant-PLD suggests that SPL is not substantially sensitive to body part details. Rather, the decoding relied on the coarse body part movements, independently of the specific stimulus type (action, pantomime, PLD). However, the stronger difference between Anim-Pant and Anim-PLD suggests that SPL is also sensitive to implied AES. This appears unlikely, because no effects (in left anterior inferior parietal lobe [aIPL]) or only weak effects (in right SPL) were found for the more canonical Action-Anim cross-decoding. The Anim-Pant cross-decoding was even stronger than the Action-Anim cross-decoding, which is counterintuitive because naturalistic actions contain more information than pantomimes, specifically with regard to action effect structures. How can this pattern of results be interpreted? Perhaps, for pantomimes and animations, not only aIPL and lateral occipitotemporal cortex (LOTC) but also SPL is involved in inferring (implied) action effect structures. However, for this conclusion, also differences for the comparison of Action-Pant with Action-PLD and for Action-Pant with Pant-PLD should be found. Another non-mutually exclusive interpretation is related to the fact that both animations and pantomimes are more ambiguous in terms of the specific action, as opposed to naturalistic actions. For example, the ‘squashing’ animation and pantomime are both ambiguous in terms of what is squashed/compressed, which might require additional load to infer both the action and the induced effect. The increased activation of action-related information might in turn increase the chance for a match between neural activation patterns of animations and pantomimes. In any case, these additional results in SPL do not question the effects reported in the main text, that is, disproportionate sensitivity for action effect structures in right aIPL and LOTC and for body movements in SPL and other AON regions. The evidence for implied action effect structures representation in SPL is mixed and should be interpreted with caution. Asterisks indicate FDR-corrected significant decoding accuracies above chance (*p < 0.05, ***p < 0.001, ****p < 0.0001). Error bars indicate SEM (N=24).
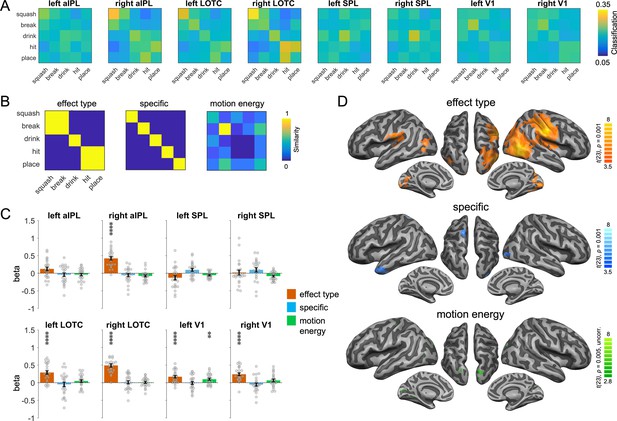
Representational similarity analysis (RSA) for the action-animation representations.
(A) Classification matrices of regions of interest (ROIs). (B) Similarity models used in the RSA. (C) Multiple regression RSA ROI analysis. Asterisks indicate FDR-corrected significant decoding accuracies above chance (**p < 0.01, ****p < 0.0001). Error bars indicate SEM (N=24). (D) Multiple regression RSA searchlight analysis. T-maps are thresholded using Monte-Carlo correction for multiple comparisons (voxel threshold p = 0.001, corrected cluster threshold p = 0.05) except for the motion energy model (p = 0.005, uncorrected).
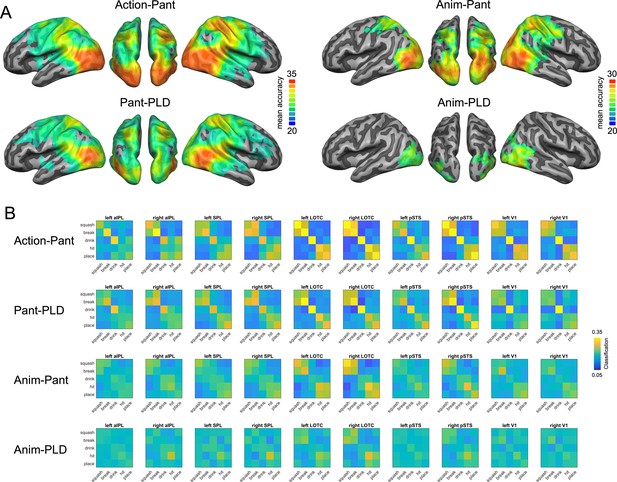
Cross-decoding results for Action-Pantomime, Pantomime-PLD, Animation-Pantomime, and Animation-PLD.
(A) Cross-decoding maps for Action-Pantomime, Pantomime-PLD, Animation-Pantomime, and Animation-PLD (Monte-Carlo corrected for multiple comparisons; voxel threshold p = 0.001, corrected cluster threshold p = 0.05). (B) Classification matrices extracted from the cross-decoding maps.
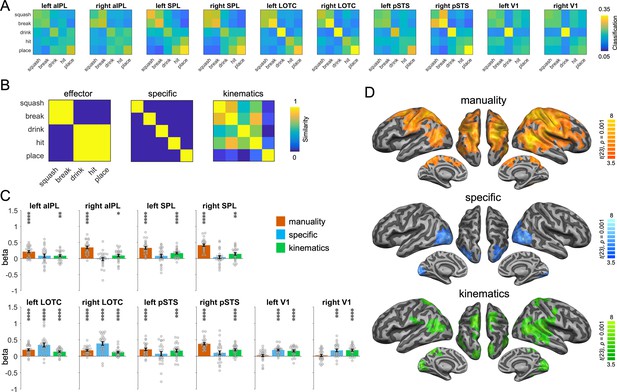
Representational similarity analysis (RSA) for the action-PLD representations.
(A) Classification matrices of regions of interest (ROIs). (B) Similarity models used in the RSA. (C) Multiple regression RSA ROI analysis. (D) Multiple regression RSA searchlight analysis. Same figure conventions as in Figure 5.

Region of interest (ROI) similarity for action-animation and action-PLD representations.
(A) Correlation matrix for the action-animation and action-PLD decoding and all ROIs. (B) Multidimensional scaling. (C) Dendrogram plot.
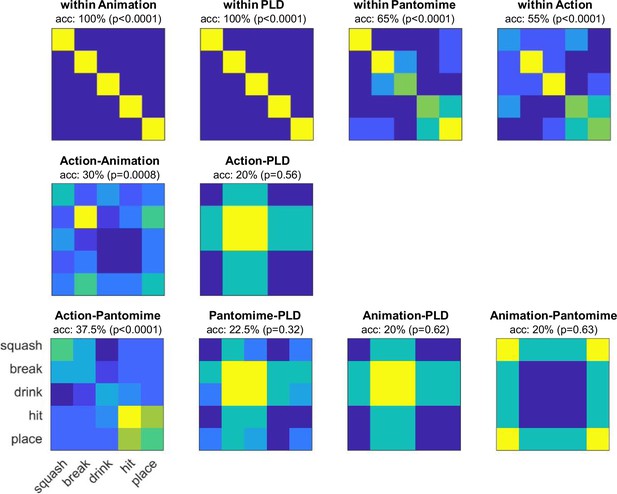
Stimulus-based cross-decoding.
For each video, motion energy features were extracted as described in Nishimoto et al., 2011 using Matlab code from GitHub (Nishimoto and Lescroart, 2018). To reduce the number of features for the subsequent MVPA, we used the motion energy model with 2139 channels. The resulting motion energy features were averaged across time and entered into within- and across-decoding schemes as described for the fMRI-based decoding. For the decoding within stimulus type, we used leave-one-exemplar-out cross-validation, that is, the classifier was train on seven of the eight exemplars for each action and tested on the remaining exemplar, etc. For the cross-decoding, the classifier was trained on all eight exemplars of stimulus type A and tested on all eight exemplars of stimulus type B (and vice versa). Significance was determined using a permutation test: For each decoding scheme, 10,000 decoding tests with shuffled class labels were performed to create a null distribution. p-values were computed by counting the number of values in the null distribution that were greater or as great as the observed decoding accuracy. The within-stimulus-type decoding served as a control analysis and revealed highly significant decoding accuracies for each stimulus type (animations: 100%, PLDs: 100%, pantomimes: 65%, actions: 55%), which suggests that the motion energy data generally contains information that can be detected by a classifier. The cross-decoding between stimulus types was significantly above chance for action-animation and action-pantomime, but not significantly different from chance for the remaining decoding schemes. Interestingly, all cross-decoding schemes with PLDs did not perform well and revealed similar classification matrices (systematically confusing squash, hit, and place with break and drink). This might be due to different feature complexity and motion information at different spatial frequencies for PLDs, which do not generalize to the other stimulus types. We also tested whether the different stimulus types can be cross-decoded using other visual features. To test for pixelwise similarities, we averaged the video frames of each video, vectorized and z-scored them, and entered them into the decoding schemes as described above. We found above chance decoding for all within-stimulus type schemes, but not for the cross-decoding schemes (animations: 55%, PLDs: 80%, pantomimes: 40%, actions: 30%, action-anim: 15%, action-PLD: 20%, action-pant: 20%, pant-PLD: 12.5%, anim-PLD: 20%, anim-pant: 22.5%). To test for local motion similarities, we computed frame-to-frame optical flow vectors (using Matlab’s opticalFlowHS function) for each video, averaged the resulting optical flow values across frames, vectorized and z-scored them, and entered them into the decoding schemes as described above. We found above chance decoding for all within-stimulus type schemes and the action-pantomime cross-decoding, but not for the other cross-decoding schemes (animations: 75%, PLDs: 100%, pantomimes: 65%, actions: 40%, action-anim: 15%, action-PLD: 18.7%, action-pant: 38.7%, pant-PLD: 26.2%, anim-PLD: 22.5%, anim-pant: 21.2%).
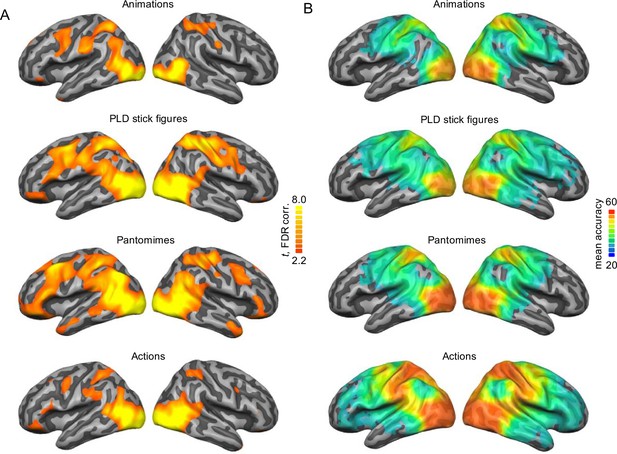
Univariate baseline and within-session decoding maps.
(A) Univariate activation maps for each session (all five actions vs. Baseline; FDR-corrected at p = 0.05) and (B) within-session decoding maps (Monte-Carlo corrected for multiple comparisons; voxel threshold p = 0.001, corrected cluster threshold p = 0.05).
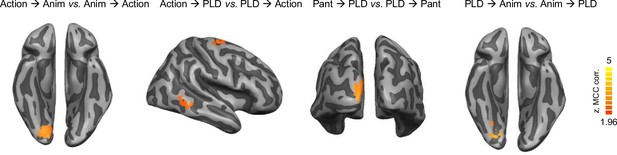
Direction-specific cross-decoding effects.
To test whether there were differences between the two directions in the cross-decoding analyses, we ran, for each of the six across-session decoding schemes, two-tailed paired samples t-tests between the decoding maps of one direction (e.g. action → animation) versus the other direction (animation → action). Direction effects were observed in left early visual cortex for the directions action → animation, PLD → animation, and pantomime → PLD, as well in right middle temporal gyrus and dorsal premotor cortex for action → PLD. These effects might be due to noise differences between stimulus types (van den Hurk and Op de Beeck, 2019) and do not affect the interpretation of direction-averaged cross-decoding effects in the main text. Monte-Carlo corrected for multiple comparisons; voxel threshold p = 0.001, corrected cluster threshold p = 0.05.
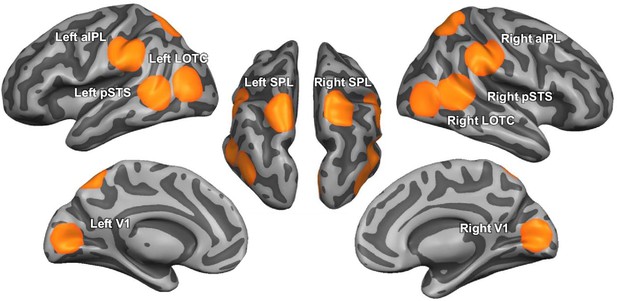
ROIs used in the study.
Spherical ROIs were in volume space (12 mm radius); here, we projected them on the cortical surface for a better comparison with the whole-brain maps in the main article.
Additional files
-
Supplementary file 1
Results of behavioral pilot experiment for abstract animations.
Verbal descriptions of each participant and mean confidence ratings (from 1 = not at all to 10 = very much ± standard deviations).
- https://cdn.elifesciences.org/articles/98521/elife-98521-supp1-v1.docx
-
Supplementary file 2
Results of behavioral pilot experiment for PLD stick figures.
Verbal descriptions of each participant and mean confidence ratings (from 1 = not at all to 10 = very much ± standard deviations).
- https://cdn.elifesciences.org/articles/98521/elife-98521-supp2-v1.docx
-
Supplementary file 3
Results of behavioral pilot experiment for pantomimes.
Verbal descriptions of each participant and mean confidence ratings (from 1 = not at all to 10 = very much ± standard deviations).
- https://cdn.elifesciences.org/articles/98521/elife-98521-supp3-v1.docx
-
MDAR checklist
- https://cdn.elifesciences.org/articles/98521/elife-98521-mdarchecklist1-v1.pdf