Accurate predictions of SARS-CoV-2 infectivity from comprehensive analysis
Figures
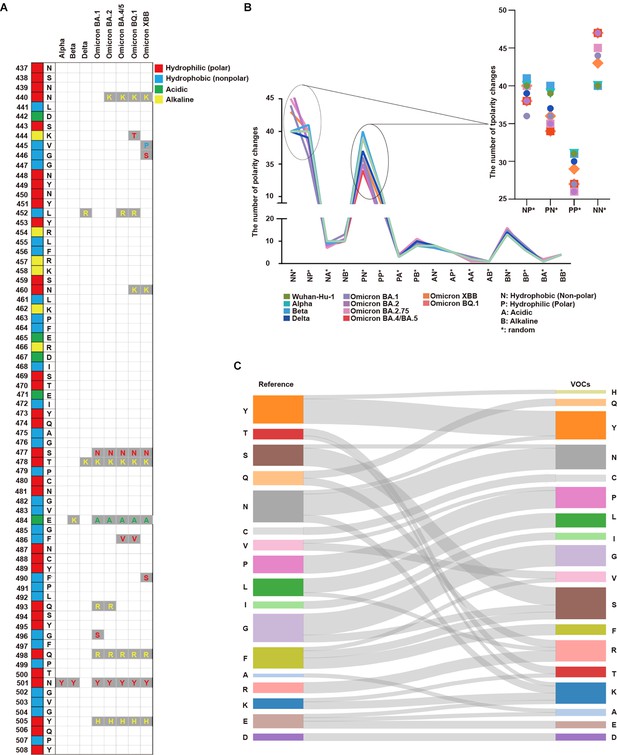
Analysis of protein properties discovered in the SARS-CoV-2 amino acid sequence.
(A) The SARS-CoV-2 amino acid sequence between positions 437 and 508 in the receptor binding motif (RBM) is displayed with the corresponding amino acids in the original positions. Amino acid substitutions are shown for Alpha, Beta, Delta, Omicron BA.1, Omicron BA.2, Omicron BA.4/BA.5, Omicron BQ.1, and Omicron XBB. Hydrophilic (polar) amino acids are displayed in red, hydrophobic (non-polar) in blue, acidic in green, and alkaline (positively charged) in yellow. (B) The number of polarity changes [N: hydrophobic (nonpolar), P: hydrophilic (polar), A: acidic, and B: alkaline (basic)] in the receptor binding domain (RBD) region is displayed. Wuhan-Hu-1, Alpha, Beta, Delta, Omicron (BA.1), Omicron (BA.2), Omicron (BA.2.75), Omicron (BA.4/BA.5), Omicron (XBB), and Omicron (BQ.1) are presented on the graph with each lineage color-coded. The polarity change count for NN*, NP*, PN*, and PP* are shown in more detail. PN* (Wuhan-Hu-1: 39, Alpha: 39, Beta: 40, Delta: 37, Omicron BA.1: 36, Omicron BA.2: 35, Omicron BA.2.75: 35, Omicron BA.4/BA.5: 34, Omicron XBB: 36, Omicron BQ.1: 34) PP* (Wuhan-Hu-1: 31, Alpha: 31, Beta: 31, Delta: 30, Omicron BA.1: 27, Omicron BA.2: 26, Omicron BA.2.75: 26, Omicron BA.4/BA.5: 27, Omicron XBB: 29, Omicron BQ.1: 27). Overall, polarity decreased from PN* to PP* across all SARS-CoV-2 lineages. (C) The amino acid substitutions in the RBM region from the reference to VOCs are displayed. The seventeen amino acids in the reference list are tyrosine (Y), threonine (T), serine (S), glutamine (Q), asparagine (N), cysteine (C), valine (V), proline (P), leucine (L), isoleucine (I), glycine (G), phenylalanine (F), alanine (A), arginine (R), lysine (K), glutamic acid (E), and aspartic acid (D). For variants of concerns (VOCs), the amino acid substitutions are indicated by gray lines. There was a more than twofold increase in lysine (K) and arginine (R) in VOCs compared with the reference.
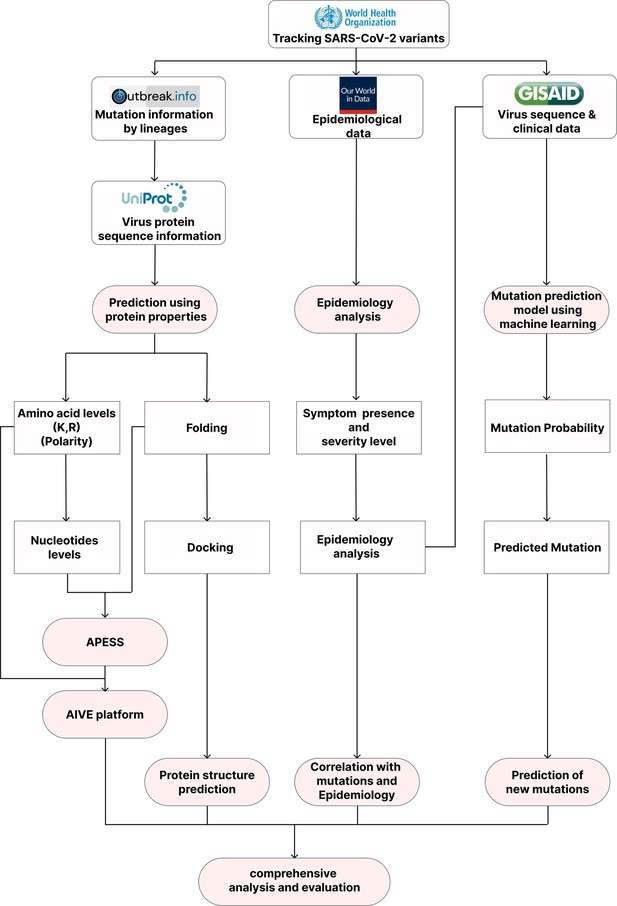
Research Overview.
AIVE can perform comprehensive analyses of SARS-CoV-2 mutations entered by the User. From the World Health Organization (WHO), we obtained data from variants of concern (VOCs) and variants under monitoring (VUMs) and lineage mutation data from outbreak.info. Epidemiological data was obtained from Our World in Data (OWID) to provide the number of infections, deaths, vaccinations, and reproduction rate. From Global Initiative on Sharing All Influenza Data (GISAID), virus sequence data and epidemiological data were collected. Through comprehensive analysis of databases, mutation prediction, evaluation, and epidemiological analysis based on mutation properties was carried out, and a web-based platform (AIVE) was created.
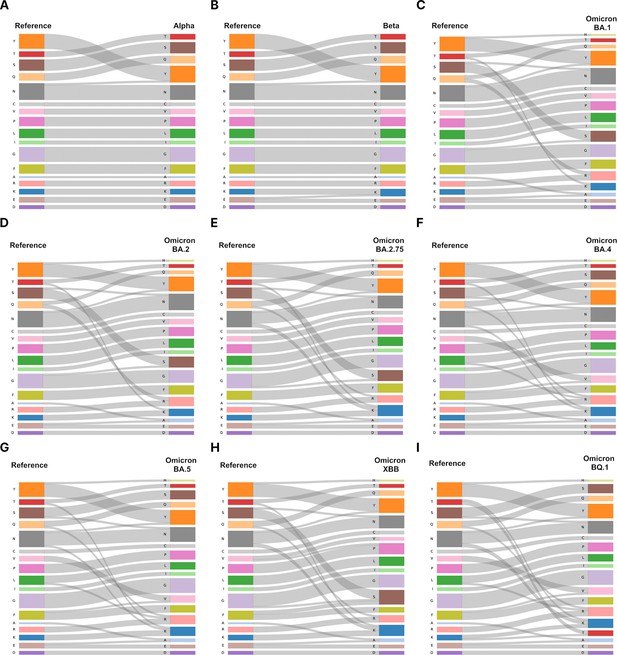
Amino acid substitutions in spike proteins from variants of concerns (VOCs).
To show the changes of mutations according to evolution from Alpha to Omicron, we provide the Sankey diagram of each lineage. In VOCs (Alpha, Beta, Omicron BA.1, BA.2, BA.2.75, BA.4, BA.5, XBB, BQ.1), differences in amino acid substitutions were observed for each lineage compared to the reference. For Omicron sublineages, tyrosine (Y) is substituted for histidine (H). Also, there is an increase in lysine (K) and arginine (R) compared to the reference. The title of each lineage is as follows: (A) Alpha, (B) Beta, (C) Omicron BA.1, (D) Omicron BA.2, (E) Omicron BA.2.75, (F) Omicron BA.4, (G) Omicron BA.5, (H) Omicron XBB, and (I) Omicron BQ.1.
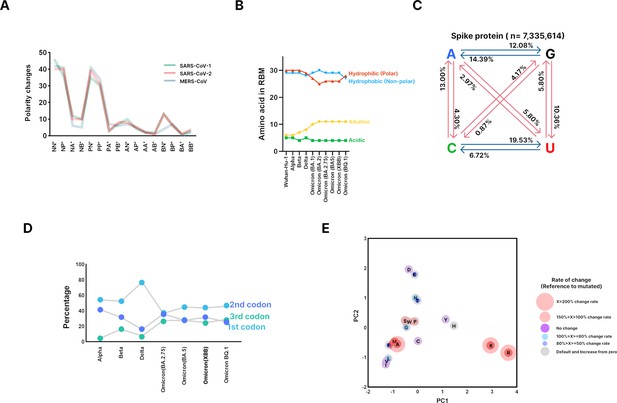
Polarity changes in the spike protein region of coronavirus and characteristics of RNA and amino acid levels of SARS-CoV-2.
(A) The polarity of coronavirus: SARS-CoV-1, MERS-CoV, and SARS-CoV-2 are displayed. hydrophilic (N), hydrophobic (P), acidic (A), and basic (B). (B) The number of amino acids in the receptor binding motif (RBM) region for SARS-CoV-2 lineages (Wuhan-Hu-1, Alpha, Beta, Delta, BA.1, BA.2, BA.2.75, BA.4, XBB, and BQ.1) are displayed. As SARS-CoV-2 lineages progress from Wuhan-Hu-1 to Omicron, hydrophilic (polar) and hydrophobic (non-polar) amino acids are maintained at higher numbers. Meanwhile, there has been a slight increase in basic amino acids. There has not been a significant change in acidic amino acids. (C) Transition and transversion rates for Adenine (A), Guanine (G), Cytosine (C), and Uracil (U) were counted and displayed (Red: transversions and Blue: transitions) for the spike protein of the 7,335,614 samples. (D) We summarized the occurrences of SARS-CoV-2 mutations for the three codon positions comprising amino acids and investigated the mutation rate in the lineages (Alpha, Beta, Delta, Omicron (BA.2.75), Omicron (BA.4/BA.5), Omicron (XBB), Omicron BQ.1). The mutation rate for each codon position showed different values for each lineage. The mutation occurrence rate for the second codon position was over 50% on average for all lineages. In the case of the Delta variant, the mutation rate for the second codon position was the highest among all variants at 76.94%. (E) The amino acid substitutions are displayed, and the size of the circle signifies the rate of change for the mutations against the reference. Lysine (K), arginine (R), and alanine (A) have the highest rate of change at over 200%.
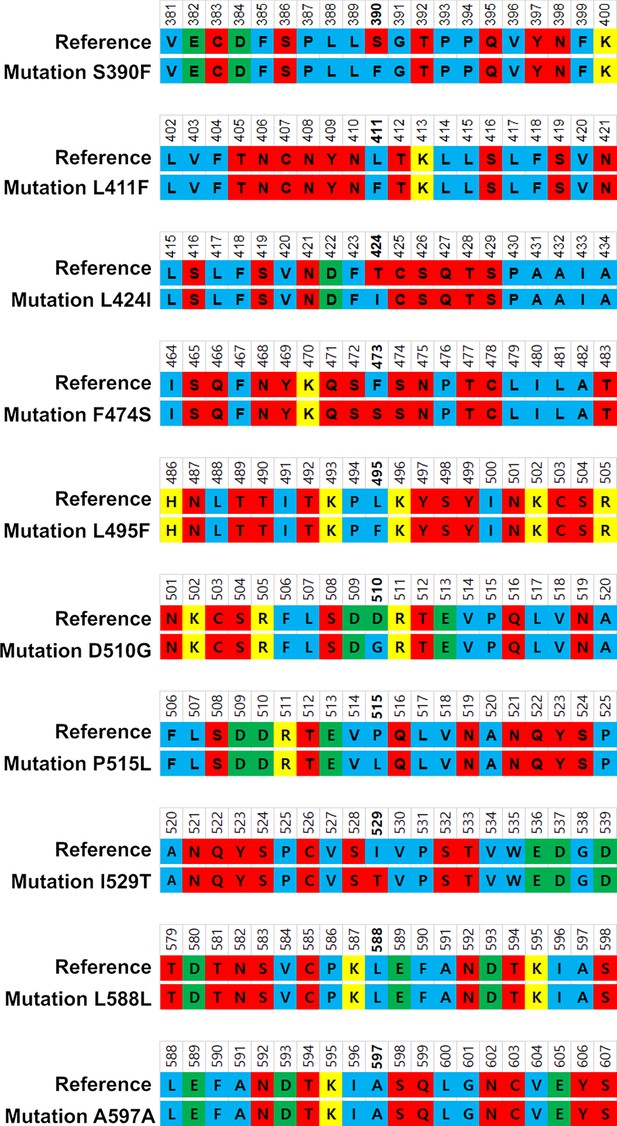
Polarity change analysis due to mutation in the amino acid sequence of MERS-CoV.
The amino acid sequence for MERS-CoV, a known coronavirus like SARS-CoV-2, and the mutations are displayed. The red indicates hydrophilic (polar), the blue indicates hydrophobic (non-polar), the green indicates acidic, and the yellow indicates alkaline (basic) amino acids. In MERS-CoV, a limited number of mutations were found. Notably, the S390F, L411F, T424I, F473S, D510S, P515L, I529T, L588L, and A597A mutations. In characteristics of MERS-CoV mutations, consecutive hydrophilic patterns are found in MERS-CoV, which the mutations do not disrupt.
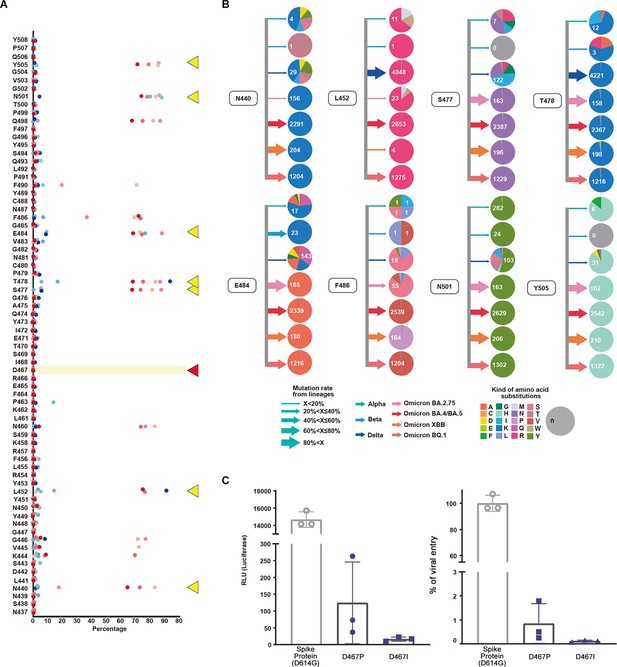
Evaluation and validation of amino acid substitutions in the SARS-CoV-2 receptor binding motif (RBM) region.
(A) Mutations, their occurrence rates as percentages, and the original amino acid at the position are shown. Alpha, Beta, Delta, Omicron (BA.2.75), Omicron (BA.4/BA.5), Omicron (XBB), and Omicron (BQ.1) lineages are displayed with corresponding colors. N440, L452, S477, T478, E484, F486, N501, and Y505 are indicated by yellow triangles while D467 is indicated by a red triangle. (B) For positions N440, L452, S477, T478, E484, F486, N501, and Y505, lineages and amino acid substitutions are displayed. Arrows indicate the mutation rate where width corresponds with the percentage and the colors indicate lineages Alpha, Beta, Delta, Omicron (BA.2.75), Omicron (BA.4/BA.5), Omicron (XBB), and Omicron (BQ.1). The colors in the pie chart indicate amino acids. The mutation rate of Alpha is lower than 20%. At the 501st position in the RBM region, amino acid substitutions from asparagine (N) to tyrosine (Y) (n=282) occurred along with other substitutions. For the Beta variant, the 484th position showed a mutation rate over 60% with E484K. The Delta variant showed a mutation rate of 60% at the 452nd position. L452R and T478K amino acid substitutions along with various mutations were observed. In Omicron, the mutation rate for S477, T478, E484, N501, and Y505 was over 40%. The amino acid substitutions were S477K, T478K, E484A, N501Y, and Y505H. We calculated the mutation rates for the following positions: T478K (99.95%), Q498R (94.14%), N501Y (99.52%), and Y505H (97.66%). (C) The effect of the D467 amino acid substitution on viral infection was evaluated in vitro via luciferase and viral entry assays. Mutagenesis at D467 to hydrophobic amino acids proline (P) and isoleucine (I) was performed. There was a significant decrease in the RLU and viral entry percentages for both D467P and D467I (<0.0001).
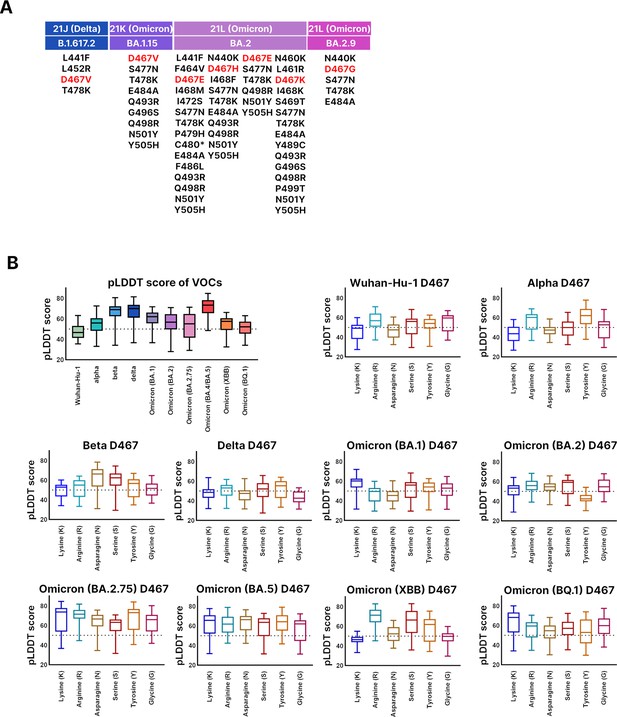
Presence of D467 mutation from Global Initiative on Sharing All Influenza Data (GISAID) and evaluation of protein structure due to D467.
(A) Of the investigated sequences, there are very few instances of D467 amino acid substitutions. In the Delta variant, D467V was observed. In the Omicron BA.1.15 variant, D467V was observed. In the Omicron BA.2 variant, D467E, D467H, D467E, and D467K were observed. In the Omicron BA.2.9 variant, D467G was observed. (B) The pLDDT scores are displayed on the y-axis for the graphs. Protein structure prediction of mutagenesis sequences at D467 showed that there was a general decrease in predicted local distance difference test (pLDDT) score compared to VOCs. For SARS-CoV-2 variants, mutagenesis at D467 to lysine (K), arginine (R), glycine (G), tyrosine (Y), asparagine (N), serine (S) was carried out for Wuhan-Hu-1, Alpha, Beta, Delta, and Omicron (BA.1, BA.2, BA.2.75, BA.4/BA.5, XBB, BQ.1). We compared the pLDDT scores of the D467 mutagenesis variants against VOCs. For Wuhan-Hu-1 (47.96902778), Alpha (53.94541667), Delta (67.10597222), BA.1 (60.06305556), BA.2 (56.23902778), there was a general decrease in pLDDT score compared to variants of concerns (VOCs). For Beta (65.915), BA.5 (70.96763889), BQ.1 (51.05027778), they showed similar pLDDT scores compared to the VOCs. The BA.2.75 (55.45305556) variant showed an increase in pLDDT score. However, for the pLDDT scores of all SARS-CoV-2 variants, the fluctuation of the confidence score was extreme and the predicted aligned error (PAE) score was low as well.
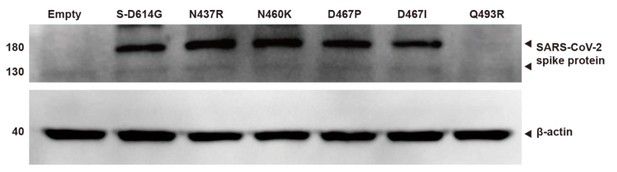
Identification of protein expression using western blotting.
We measured the expression of exogenous spike proteins through western blotting for SARS-CoV-2 spike protein mutations. To compare to the wild-type (S-D614G), mutagenesis was done for N437R, N460K, D467P, D467I, and Q493R. Normalization was done with Beta-actin. The Beta actin bands are observed uniformly across the lanes for the vector, wild-type (D614G), and mutagenesis sequences. The molecular weight of bands are displayed in kDa.
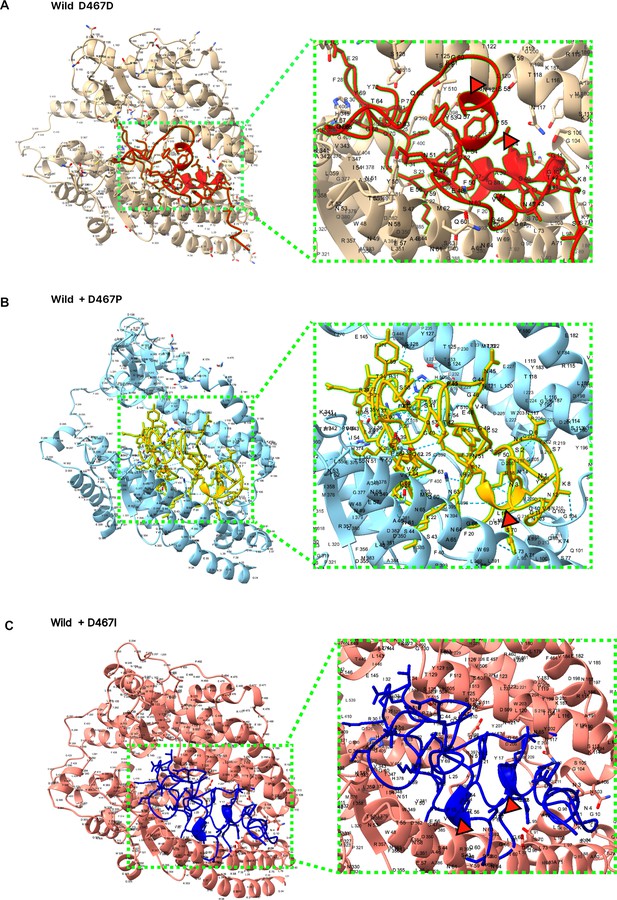
D467 protein 3D structure prediction.
Measuring the viral infectivity in vitro of the SARS-CoV-2 RBM sequence showed the structural importance of D467 (Figure 2C). In silico results of measuring the docking score between SARS-CoV-2 receptor binding motif (RBM) and the host ACE2 receptor showed contradicting results. Visualization of the SARS-CoV-2 folding structure displayed the wild-type D467D with an alpha-helix structure, indicated with a red triangle. Mutagenesis to D467P and D467I to the wild-type backbone resulted in the alpha helix structure turning into a linear structure. It becomes more likely for the protein 3D structure to become flexible.
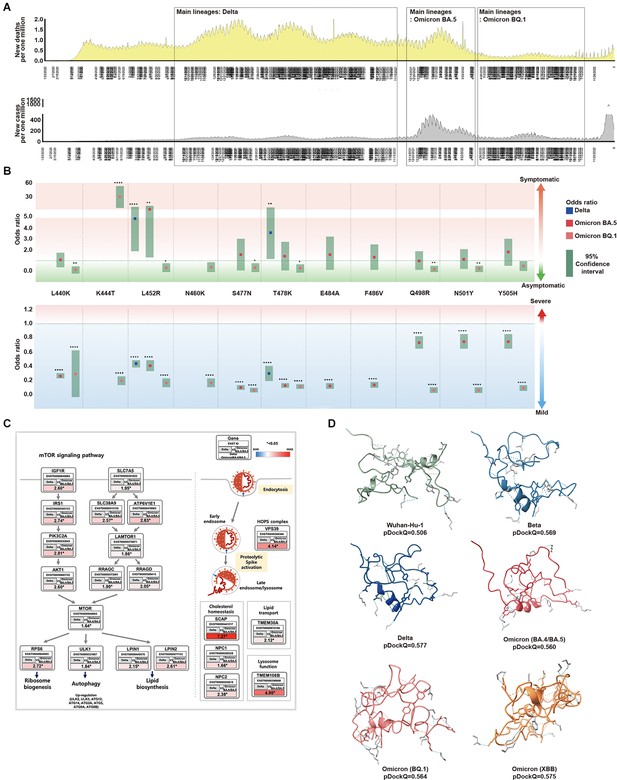
Association between SARS-CoV-2 mutations and epidemiological data.
(A) For each SARS-CoV-2 lineage, the position and distribution of amino acid substitutions were analyzed alongside epidemiological data. The first period, from November 2020 to December 2021, was characterized by low infections and high deaths. Delta was the most prominent during this period, coinciding with worldwide vaccinations. Amino acid substitutions L452R and T478K were observed at the highest frequency while T478K and L452R were independently observed at the highest frequencies. The second period, from January 2022 to April 2022, saw an increase in the new cases and deaths. BA.5 was prominent during this period with various amino acid substitutions observed. The third period, from May 2022 to November 2022, showed a significant decrease in infections and deaths. Omicron, specifically BQ.1 was prominent during this period and worldwide vaccination rates decreased. (B) From the viral sequences of the patients, the association between the primary mutations of Delta, BA.5, and BQ.1, and epidemiological data (symptoms and severity) was analyzed. Odds ratios are displayed for L440K, K444T, L452R, N460K, S477N, T478K, E484A, F486V, Q498R, N501Y, and Y505H. L452R was an indicator of symptomaticity in Delta and BA.5. K477T was associated with symptomaticity in BQ.1. All mutations were associated with mildness. The 95% confidence intervals are shown for Delta, BA.5, and BQ.1. (C) We analyzed the expression of Delta compared to BA.4/BA.5 using the GSE235262 dataset. In Delta, the mTOR pathway was observed to regulate ribosome biogenesis, autophagy, and lipid biosynthesis while also playing a role in viral infection pathways. The expression values were calculated as Delta [log2(TPM +1)] / Omicron BA.4+BA.5 [log2(TPM +1)], with ENST Ensembl Transcript IDs, and * indicating a significance level of p<0.05. (D) The folding structures and pDockQ scores (0.506, 0.569, 0.577, 0.560, 0.564, and 0.575 for Wuhan-Hu-1, Beta, Delta, BA.4/BA.5, BQ.1, and XBB, respectively) were shown.
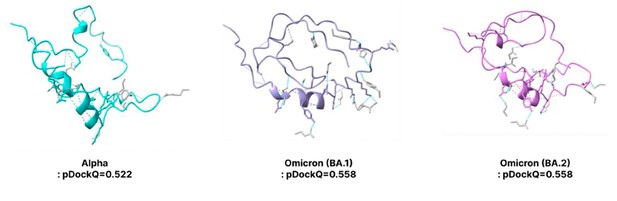
Prediction of spike protein 3D structures from Alpha Omicron BA.1 Omicron BA.2.
The folding structures and pDockQ scores of Alpha, Omicron (BA.1), and Omicron (BA.2) are displayed. The pDockQ scores are 0.522, 0.558, and 0.558, respectively.
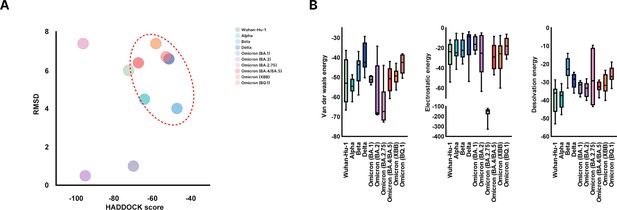
Binding affinity between SARS-CoV-2 and ACE2 receptor using HADDOCK.
(A) The root mean square deviation (RMSD) and HADDOCK scores are displayed for SARS-CoV-2 lineages Wuhan-Hu-1, Alpha, Beta, Delta, Omicron (BA.1), Omicron (BA.2), Omicron (BA.2.75), Omicron (BA.4/BA.5), Omicron (XBB), and Omicron (BQ.1). The SARS-CoV-2 lineages are shown on the graph with corresponding colors. The SARS-CoV-2 lineages with both higher RMSD and HADDOCK scores are indicated with an encompassing red circle. (B) For SARS-CoV-2 lineages Wuhan-Hu-1, Alpha, Beta, Delta, Omicron (BA.1), Omicron (BA.2), Omicron (BA.2.75), Omicron (BA.4/BA.5), Omicron (XBB), and Omicron (BQ.1), the van der waals energy, electrostatic energy, and desolvation energy are displayed in their respective graphs.
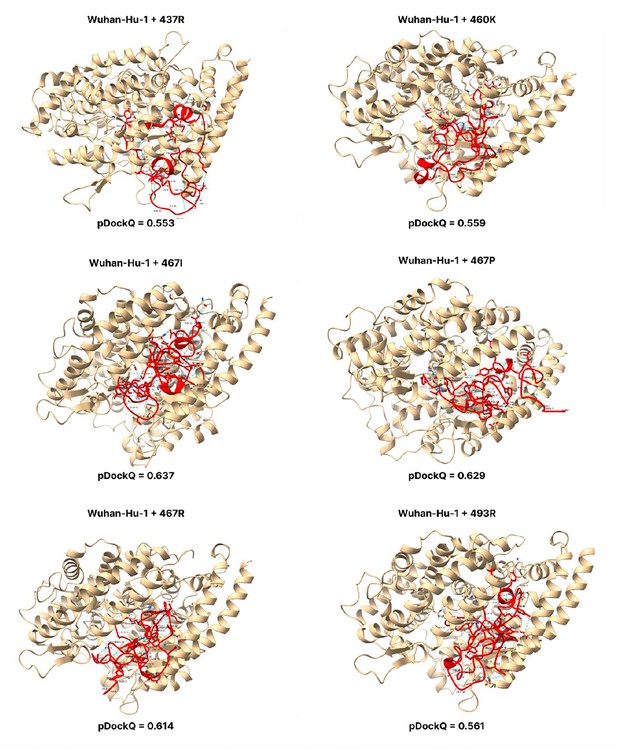
pDockQ scores of Wuhan-Hu-1 mutations.
pDockQ scores of lineages with the Wuhan-Hu-1 as the backbone, with each mutation N437R, N 460 K, D467I, D467P, D467R, and Q493R added.
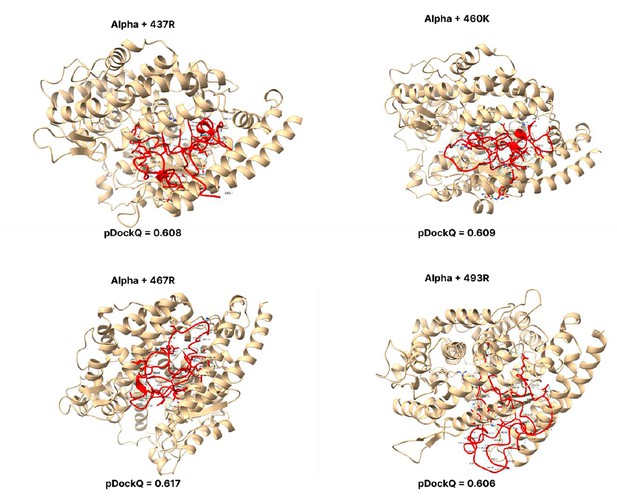
pDockQ scores of Alpha mutations.
pDockQ scores of lineages with the alpha lineages as the backbone, with each mutation N437R, N 460 K, D467R, and Q493R added.
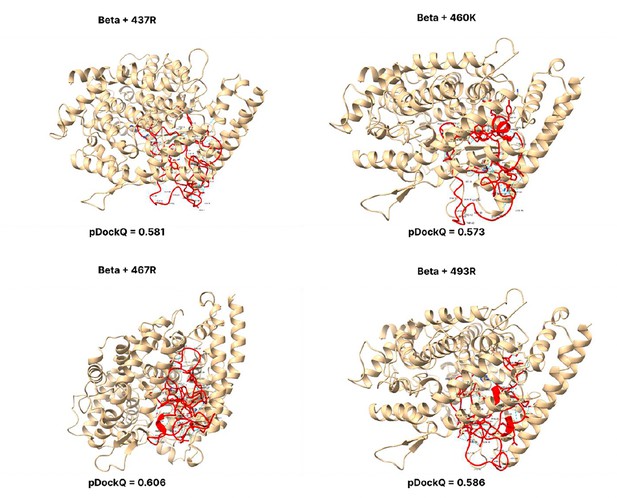
pDockQ scores of Beta mutations.
pDockQ scores of lineages with the beta lineages as the backbone, with each mutation N437R, N 460 K, D467R, and Q493R added.
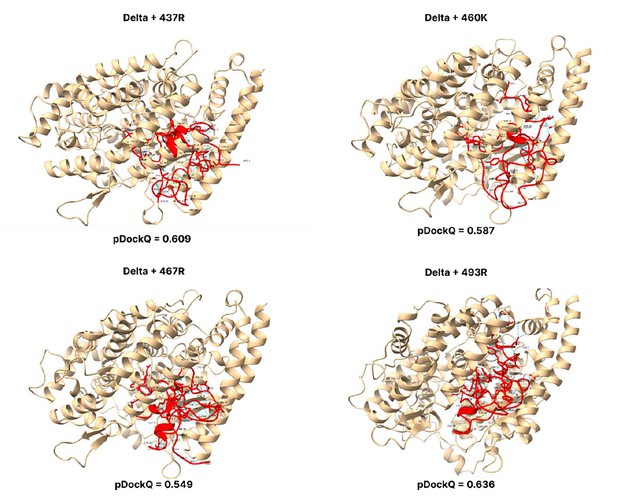
pDockQ scores of Delta mutations.
pDockQ scores of lineages with the delta lineages as the backbone, with each mutation N437R, N 460 K, D467R, and Q493R added.
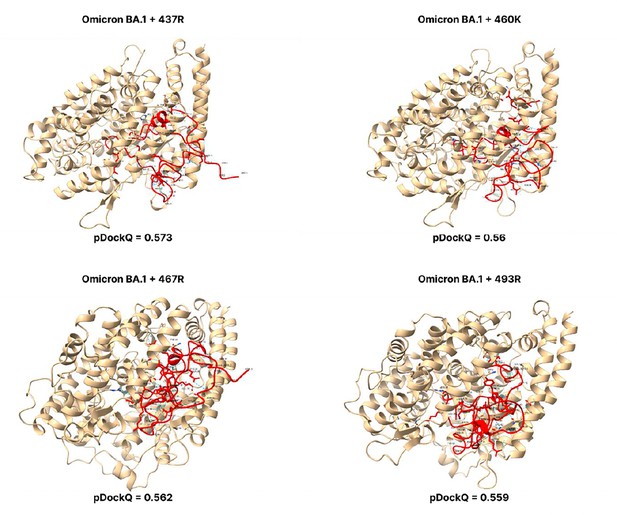
pDockQ scores of BA.1 mutations.
pDockQ scores of lineages with the omicron BA.1 as the backbone, with each mutation N437R, N 460 K, D467R, and Q493R added.
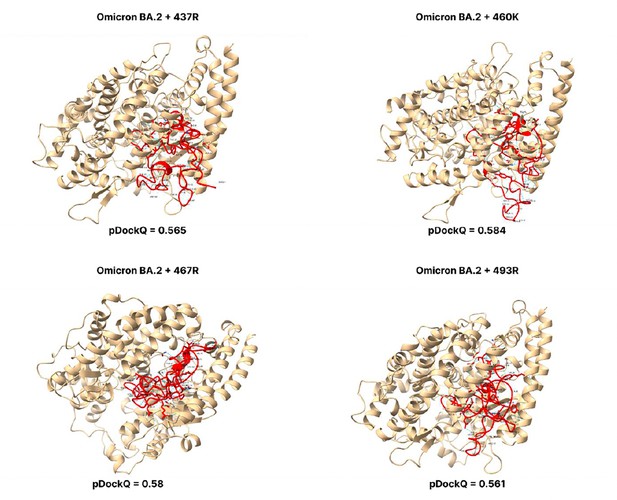
pDockQ scores of BA.2 mutations.
pDockQ scores of lineages with the omicron BA.2 lineages as the backbone, with each mutation N437R, N460K, D467R, and Q493R added.
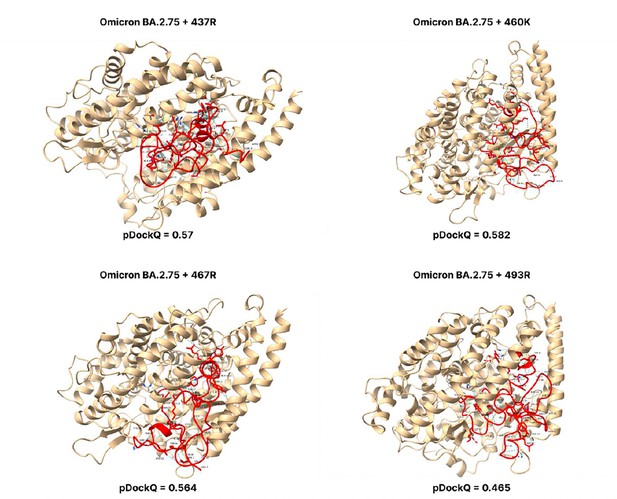
pDockQ scores of BA.2.75 mutations.
pDockQ scores of lineages with the omicron BA.2.75 lineages as the backbone, with each mutation N437R, N460K, D467R, and Q493R added.

pDockQ scores of BA.4 mutations.
pDockQ scores of lineages with the omicron BA.2.75 lineages as the backbone, with each mutation N437R, N460K, D467R, and Q493R added.
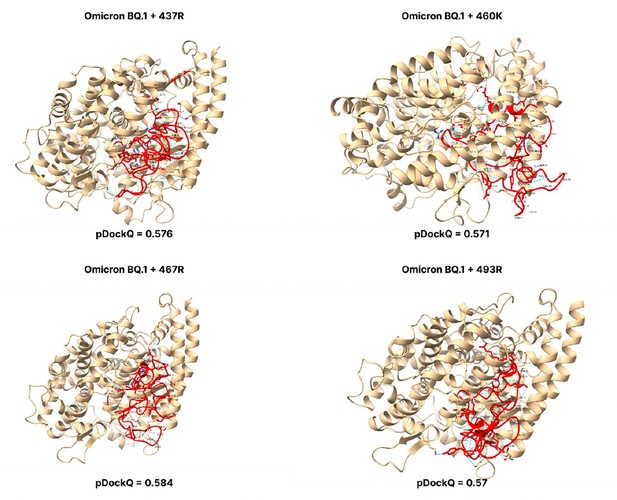
pDockQ scores of BQ.1 mutations.
pDockQ scores of lineages with the omicron BQ1 lineages as the backbone, with each mutation N437R, N460K, D467R, and Q493R added.
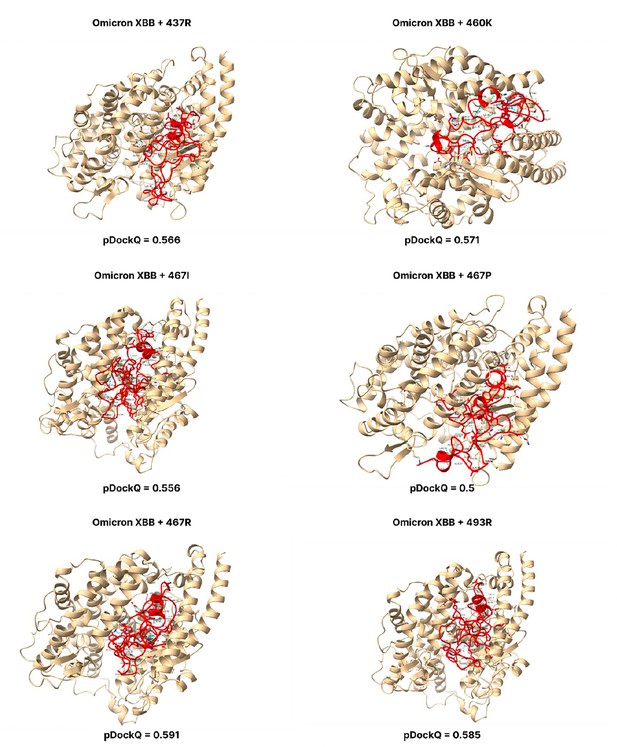
pDockQ scores of XBB mutations.
pDockQ scores of lineages with the omicron BA.2.75 lineages as the backbone, with each mutation N437R, N460K, D467R, and Q493R added.
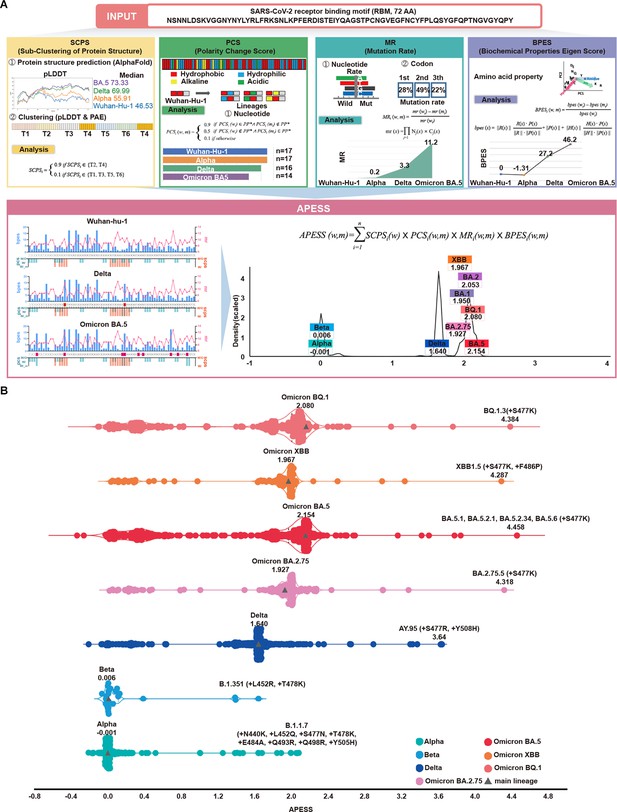
APESS: a comprehensive evaluation model of SARS-CoV-2 mutations.
(A) Amino acid property eigen selection score (APESS), an evaluation model based on the properties discovered in the receptor binding motif (RBM) and the infectivity of SARS-CoV-2, was developed. A 72-amino acid-long RBM sequence of SARS-CoV-2 was used to comprehensively evaluate the sub-clustering of protein structure (SCPS), polarity change score (PCS), mutation rate (MR), and biochemical properties eigen score (BPES). Through comprehensive analysis of each position, the infectivity of the input sequence could be evaluated against preexisting lineages. (B) The APESS scores were calculated for SARS-CoV-2 lineages Alpha, Beta, Delta, and Omicron (BA.2.75, BA.5, XBB, BQ.1), and the data were obtained for sublineages from viral sequences. The original lineages are displayed with a gray triangle and their APESS scores, whereas the sublineages are color-coded differently. The S477K substitution resulted in the highest APESS score.
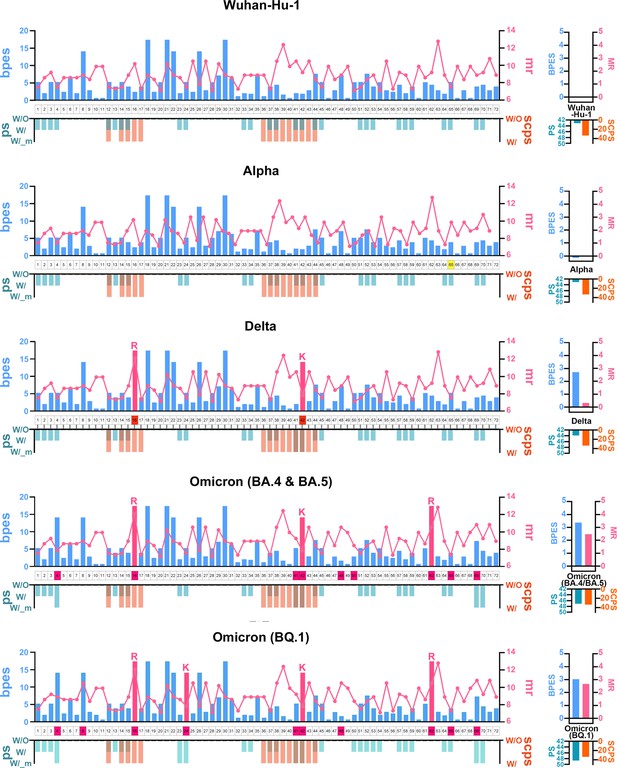
Amino acid property eigen selection score (APESS) evaluation of each section from variants of concerns (VOCs).
For SARS-CoV-2 lineages Wuhan-Hu-1, Alpha, Delta, Omicron (BA.4/BA.5), and Omicron (BQ.1), the sub-clustering of protein structure (SCPS), polarity change score (PCS), mutation rate (MR), and biochemical properties eigen score (BPES) scores are displayed. For SARS-CoV-2 lineages Wuhan-Hu-1, Alpha, Delta, Omicron (BA.4/BA.5), and Omicron (BQ.1), the SCPS, PCS, MR, and BPES scores were calculated. For the RBM 72 positions, a comprehensive evaluation was carried out. The colors in the figure correspond with the following: sky blue: BPES, pink: MR, khaki: PCS, orange: SCPS, and pink box: lysine (K) and arginine (R). As the lineages progressed to Omicron, SCPS, PCS, MR, and BPES scores were increased. Finally, APESS has a higher score in the most infectious lineage.
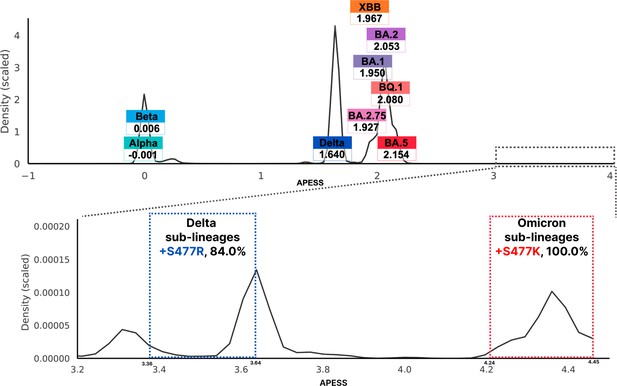
Amino acid property eigen selection score (APESS) distribution from viral sequences and APESS scores of variants of concerns (VOCs) from Global Initiative on Sharing All Influenza Data (GISAID).
We measured the APESS score distribution for a total of 7,335,614 sublineages. The graph was identical to the one created from the randomly selected 30,000 sublineages. We verified four components centered at 0.0236, 1.6062, 1.6404, and 2.0627. Other than the four components, density was very low. High APESS scores were observed within the low-density region, specifically for S477K and S477R mutations. In the Delta variant, amino acid substitution from Serine (S) to Arginine (R) was observed with one nucleotide change at the final codon. In the Omicron variant, amino acid substitution from Serine (S) to Lysine (K) was observed with two nucleotide changes. In other SARS-CoV-2 variants, amino acid substitution from Serine (S) to Aspartic acid (N) with one nucleotide change at the middle codon.
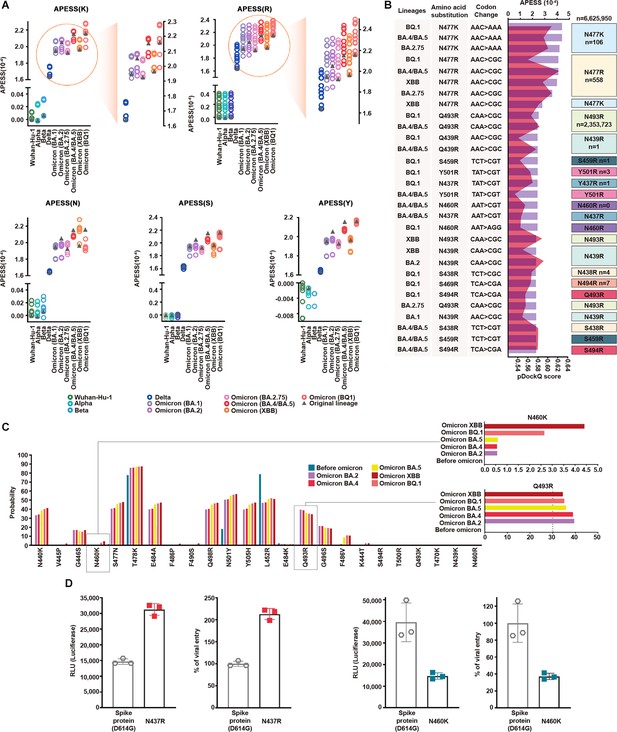
Multifaceted evaluation of SARS-CoV-2: evaluation model, machine learning, and in vitro assay.
(A) Mutagenesis sequences containing consecutive hydrophilic amino acids were evaluated with amino acid property eigen selection score (APESS). They were based on Wuhan-Hu-1, Alpha, Beta, Delta, BA.1, BA.2, BA.2.75, BA.4/BA.5, XBB, and BQ.1, as indicated by colors and gray triangles. APESS values for the K, R, N, S, and Y mutated sequences of the lineages are displayed. Mutagenesis of lysine (K) and arginine (R) in Omicron sublineages resulted in increased APESS scores, whereas mutagenesis of asparagine (N), serine (S), and tyrosine (Y) resulted in decreased APESS scores. Specific regions for K and R are magnified to show the distribution of the APESS scores of the mutagenesis sequences in more detail. (B) To predict mutations with high infectivity using APESS, mutagenesis was performed using Wuhan-Hu-1, Alpha, Beta, Delta, BA.1, BA.2, BA.2.75, BA.4/BA.5, XBB, and BQ.1 as the backbone. The presence of these amino acid substitutions was verified using the viral sequence data from GISAID. For each lineage, the amino acid substitutions resulted in 280 mutagenic sequences. Thirty sequences with the highest APESS and pDockQ scores are displayed. N460R and S469R have not been observed naturally, whereas N439R, S459R, N437R, Y501R, S438R, and S494R have been observed in ten people or less. (C) For mutations occurring in lineages and mutations evaluated through APESS, AI learning models (Random Forest, LightGBM, XGBoost, Ensemble, and deep learning) were used to investigate the probability. For N460K, there was a ninefold increase in the probability of XBB compared to prior Omicron lineages. Q493R is not found in XBB but still has a high probability of occurrence. (D) The effects of N437 and N460 amino acid substitutions on viral infection were evaluated in vitro using luciferase and viral entry assays. There was a significant increase in the Relative Light Units and viral entry percentage for N437R, and vice versa for N460K.
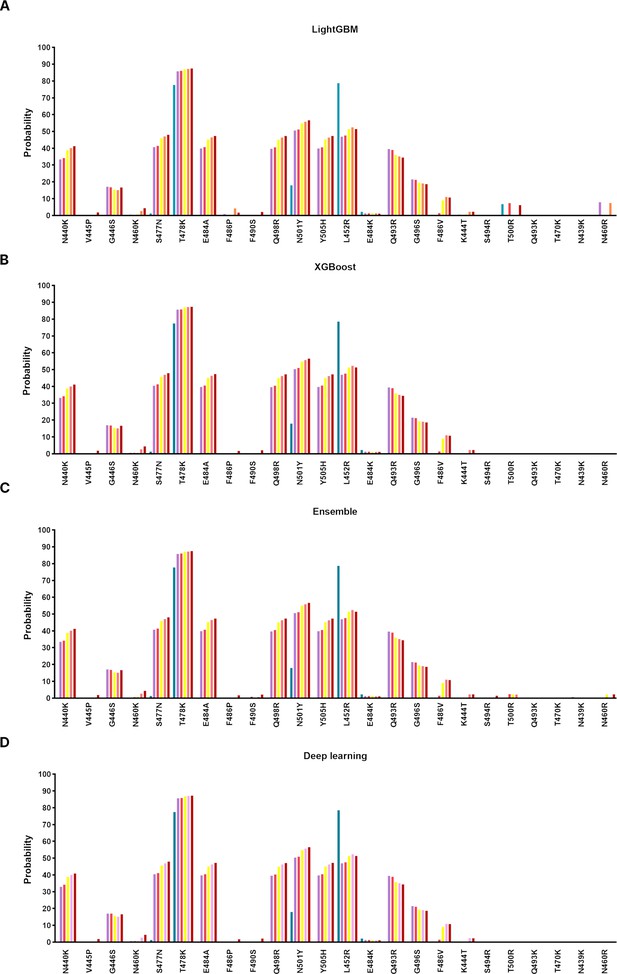
Prediction results of notable mutations in SARS-CoV-2 using ML/DL.
For mutations occurring in SARS-CoV-2 lineages (N440K, V445P, G446S, N460K, S477N, T478K, E484A, F486P, F490S, Q498R, N501Y, Y505H, L452R, E484K, Q493R, G496S, F486V, K444T) and mutations evaluated through APESS (N477R, N477K, N439R, Y501R, N437R, S438R, S459R, S469R, S494R, T470R, T500R, Q493K, T470K, T500K, S469K, S494K, Y501K, N437K, N439K, N460R, S438K, S459K, N460K, Q493R), artificial intelligence learning models were used to evaluate probability. LightGBM, XGBoost, Ensemble, and deep learning methods showed similar results to Random Forest.
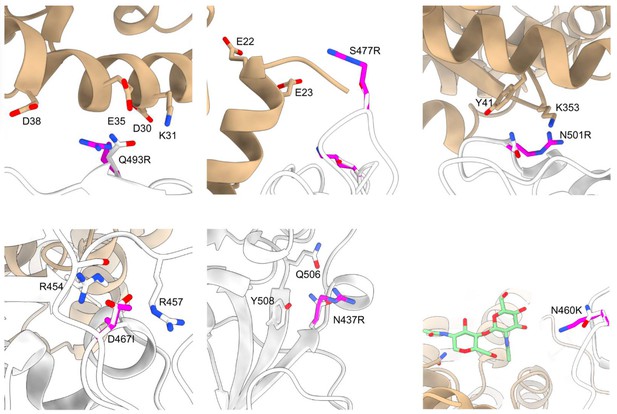
Protein structure evaluation of D467.
Close-up views of the mutated receptor binding domain (RBD) residues and their adjacent residues. ACE2 and RBD are color-coded in wheat and silver cartoons, while the original and mutated RBD residues are represented as white and magenta sticks. Mutagenesis was carried out using PyMol, and all figure images were constructed using ChimeraX-1.5.
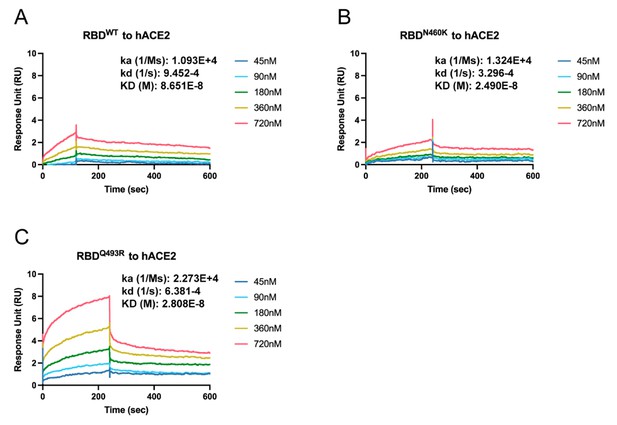
Binding affinity measurement of N460K and Q493R receptor binding domain (RBD) variants.
Surface plasmon resonance results show binding affinity between RBD wild-type (A), N460K (B), and Q493 (C) toward human ACE2. The hACE2 protein was used as a ligand and all experiments were conducted on the same sensor chip.

Flowchart for predicting mutation occurrences using artificial intelligence.
We used machine learning and deep learning methods to predict the likelihood of mutations occurring. ① We began by filtering the information and samples from the Global Initiative on Sharing All Influenza Data (GISAID) data. Unnecessary samples were removed depending on the presence of nonsense mutations, the host species, and the data format of the date column. Then, target mutations in the receptor binding motif (RBM) region were converted into binary variables. Finally, filtering is completed by removing unused features and samples with missing data, excluding the clade, date, and binary target mutation columns. ② In order to utilize the filtered data for training, we preprocessed the data. We replaced the date information in the YYYY-MM-DD format with integer values indicating how many days had passed since the earliest recorded date, 2019-12-23. Then, the values were normalized to [0–1]. We then created preprocessed data by one-hot encoding the clade data. ③ We created a training model and then used the trained model to predict the likelihood of the target mutations. Five different training models were involved in this process. ③–1 A prediction model using LightGBM, ③–2 a model using XGBoost, ③–3 sklearn library’s Random Forest model, and then an ensemble model of the previous three models were used. Finally, ③–4 keras was used to create a multi-output neural network model for training and predicting the likelihood of the target mutations.
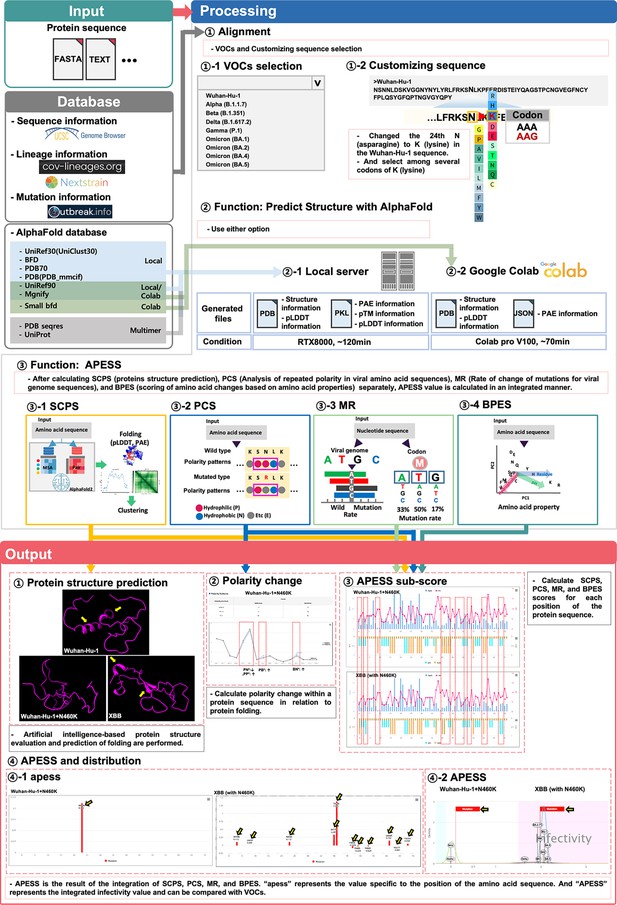
Prediction of potential SARS-CoV-2 mutations through integrated evaluation and prediction.
(Input). This figure consists of three steps: ‘Input,’ ‘Processing,’ and ‘Output.’ Users can select a custom sequence from the entire SARS-CoV-2 sequence, choose variants of concerns (VOCs), or create customized sequences for analysis. Depending on the user’s system environment, analysis can be done through local prediction (server) or Google Colab. (Processing) Three types of analyses are performed. First, the protein 3D structure prediction is analyzed. This includes protein 3D structure, predicted local distance difference test (pLDDT), and predicted aligned error (PAE). Second, the infectivity is evaluated using APESS (2.12). For each position, the structural difference graph for biochemical properties eigen score (BPES), mutation rate (MR), polarity change score (PCS), and sub-clustering of protein structure (SCPS) is visualized. The APESS distribution is visualized for known VOCs and created variants. Third, polarity changes are visualized in sequences. (Output) Four results comparing Wuhan-Hu-1+N460 K and XBB (with N460K) are output and visualized. First, through protein structure prediction, secondary structures can be confirmed in XBB (with N460K) compared to Wuhan-Hu-1+N460 K (Yellow arrow). Second, the comparison of polarity changes through the mutation of Wuhan-Hu-1+N460 K (red dotted line) is done. Third, in XBB (with N460K), which has more mutations than Wuhan-Hu-1+N460 K, the difference in values at each position in the protein sequence of SCPS, PCS, MR, and BPES is displayed (red dotted line). Fourth, the distribution of APESS, which represents the comprehensive value of SCPS, PCS, MR, and BPES, is shown. ‘apess’ indicates the score for each position in the customized protein sequence. In the case of XBB (with N460K), which has more mutations than Wuhan-Hu-1+N460 K, an apess score distribution of XBB (with N460K) has values from –0.079–1.385 is shown (Yellow arrow). X-axis presents the position in RBM (72aa) and Y-axis presents APESS score, respectively (④–1). APESS is the summed value of each position and can evaluate infectivity. A region including XBB (with N460K) shows infectivity due to many mutations, and also shows an increase in APESS score due to the N460K mutation. X-axis presents APESS score and Y-axis presents a density, respectively (④–2). AIVE comprehensively evaluates viral infectivity, protein structure, amino acid substitutions, and polarity changes in preexisting and potential SARS-CoV-2 sequences.
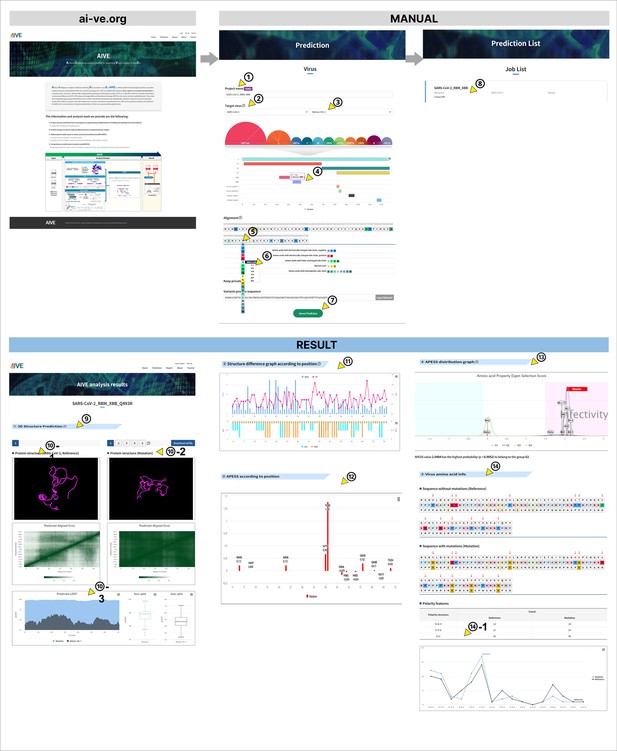
The user interface user experience (UI/UX) of the AIVE system.
An instruction manual for accessing the AIVE website (ai-ve.org) and using its prediction was shown. ① The project name is entered by the user. ② The target virus is selected. In the demonstration, SARS-CoV-2 is selected. ③ The specific variant of concern (VOC) can be selected. In the demonstration, Wuhan-Hu-1 is selected. ④ Click on the receptor binding domain (RBD) region. ⑤ The amino acid can be selected in the position in the sequence. ⑥ The codon can be selected for the amino acid. ⑦ Server prediction is started. ⑧ For Prediction List, it shows the current job list with ongoing projects and how much time is left for analysis. ⑨ The 3D Structure Prediction results are shown. ⑩–1 The 3D Protein structure (SARS-CoV-2, Reference) ⑩–2 The 3D Protein structure (Mutation). ⑩–3 The Predicted LDDT score. ⑪ Scores of sub-clustering of protein structure (SCPS), polarity change score (PCS), mutation rate (MR), and biochemical properties eigen score (BPES) by amino acid position. ⑫ Scores of amino acid property eigen selection score (APESS) by amino acid position. ⑬ Distribution of APESS score of SARS-CoV-2 VOCs Alpha, Beta, Delta, and Omicron (BA.2.75, BA.5, XBB, BQ.1) and location of APESS range as the input. ⑭ Comparison of polarity change between input and reference. ⑭–1. Number of NN*, NP*, NA*, NB*, PN*, PP*, PA*, PB*, AN*, AP*, AA*, AB*, BN*, BP*, BA*, BB* between input and reference.
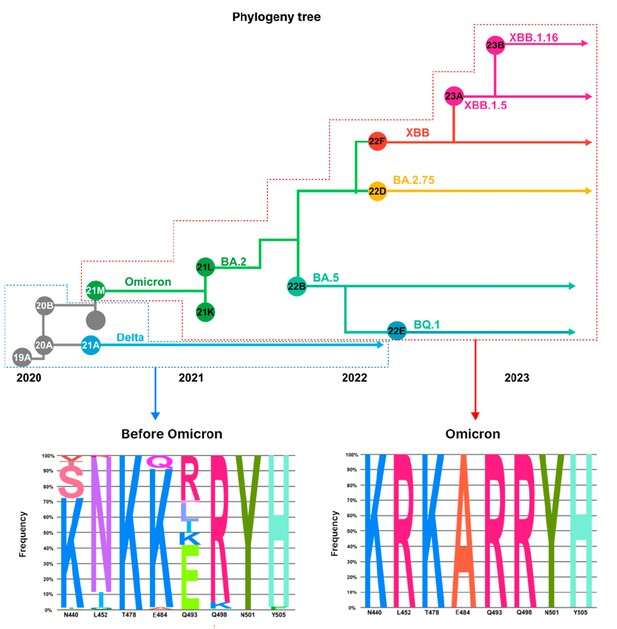
Frequencies of amino acid substitutions of major variants within clades.
Phylogenetic tree showing the evolution of SARS-CoV-2 lineages and their sub-lineages. Before Omicron, at various positions N440, L452, T478, T484, Q493, Q498, N501, Y505, the frequency of amino acids is displayed. There are different types of amino acid substitutions present with the different positions showing varied percentages. In Omicron lineages, the frequency of amino acid substitutions of N440K, L452R, T478K, T484A, Q493R, Q498R, N501Y, and Y505H approach 100%, showing that amino acid substitutions become fixed.
Tables
Statistical values of the Superposition of Gaussian Densities for SCPS weight 0.9/0.1.
Component | mean | variance | se | weight |
---|---|---|---|---|
G1 | 2.0642 | 0.0034 | 0.058309519 | 0.4104 |
G2 | 1.8725 | 0.0778 | 0.278926514 | 0.0388 |
G3 | 0.0263 | 0.0056 | 0.074833148 | 0.2024 |
G4 | 1.6404 | 0 | 0 | 0.3484 |
Statistical values of the Superposition of Gaussian Densities for SCPS weight 0.8/0.2.
Component | mean | variance | se | weight |
---|---|---|---|---|
G1 | 0 | 1.00E-06 | 0.001 | 0.191166653 |
G2 | 8.434051839 | 0.004907285 | 0.070052018 | 0.226271799 |
G3 | 4.210249767 | 1.00E-06 | 0.001 | 0.353961013 |
G4 | 6.758534282 | 2.463725537 | 1.569625923 | 0.228600536 |
Statistical values of the Superposition of Gaussian Densities for SCPS weight 0.7/0.3.
Component | mean | variance | se | weight |
---|---|---|---|---|
G1 | 0 | 1.00E-06 | 0.001 | 0.19116666 |
G2 | 7.306207524 | 2.700755873 | 1.643397661 | 0.229009764 |
G3 | 3.683968546 | 1.00E-06 | 0.001 | 0.353987719 |
G4 | 9.478264495 | 0.011051658 | 0.105126865 | 0.225835857 |
Additional files
-
Supplementary file 1
Supplementary tables.
(a) The number of polarity changes in the receptor binding motif (RBM). (b) The number of polarity changes in the spike protein. (c) Configuration of polarity changes caused by variants of concern (VOCs) in the RBM. (d) The number of amino acids in VOCs. (e) The number of transitions and transversions in the spike protein from viral sequences. (f) Epidemiological information (cases and deaths per million population) from Our World in Data (OWID). (g) Amino acid substitution of sublineages. (h) Symptomatic information (symptomatic and asymptomatic) from Global Initiative on Sharing All Influenza Data (GISAID). (i) Severity information (severe and mild) from GISAID. (j) Gene expression data between the Delta variant and Omicron (BA.4/5) variant from Gene Expression Omnibus (GEO) dataset. (k) Binding affinity between proteins in protein 3D structure using pDockQ. (i) Evaluation of hydrogen bonds (H-bond) through protein 3D structure prediction of SARS-CoV-2. (m) Binding affinity between proteins in protein 3D structure using HADDOCK. (n) The number of mutations per codon in the RBM. (o) The number of mutations per codon in the receptor binding domain (RBD). (p) The number of mutations per codon in the spike protein. (q) Amino acid property eigen selection score (APESS) score for each mutation with VOCs as the backbone. (r) Prediction of mutation probability using lightGBM. (s) Prediction of mutation probability using XGBoost. (t) Prediction of mutation probability using Random Forest. (u) Prediction of mutation probability using ensemble. (v) Prediction of mutation probability using deep learning. (w) The number of samples by clade in global SARS-CoV-2 analysis data. (x) Amino acid property values utilizing principal component analysis (PCA) clustering.
- https://cdn.elifesciences.org/articles/99833/elife-99833-supp1-v2.xlsx
-
MDAR checklist
- https://cdn.elifesciences.org/articles/99833/elife-99833-mdarchecklist1-v2.docx
-
Supplementary file 2
AIVE usage guide.
- https://cdn.elifesciences.org/articles/99833/elife-99833-supp2-v2.pdf