Tripartite organization of brain state dynamics underlying spoken narrative comprehension
Figures
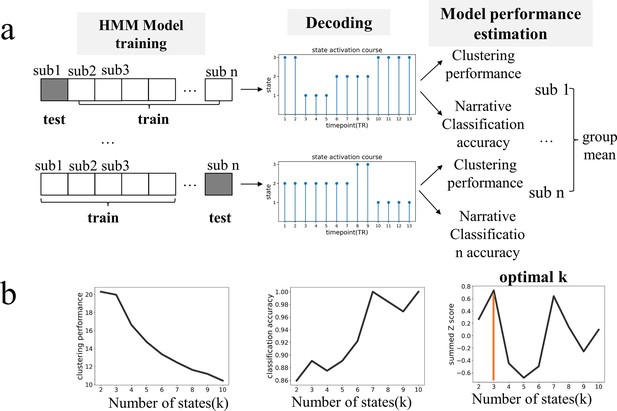
Identifying the optimal number of brain latent states based on the criterion of statistical robustness and cognitive sensibility.
(a) For each candidate K ranging from 2 to 10, we trained a Hidden Markov Modeling (HMM) model on n-1 subjects and applied it to decode the time course of state expression for the test subject. The decoded time course was then used to compute a Calinski-Harabasz score, with a larger value indicating better clustering performance, and to decipher which narrative (out of three) was heard by the subject. The two measurements were first assessed at the individual level and then averaged across participants. (b) Model performance as a function of K. With the increase of K, the model’s clustering performance tended to decline while the ability to decipher narrative contents tended to improve. We combined the two indices by converting them independently to z scores and summed them up. Notably, at K = 3, the summed z-score reached its highest point, therefore, it was set as the optimal number of latent states. See Figure 1—figure supplement 2 for the replication of optimal K identification using a different whole-brain parcellation scheme and on a dataset of older adults.
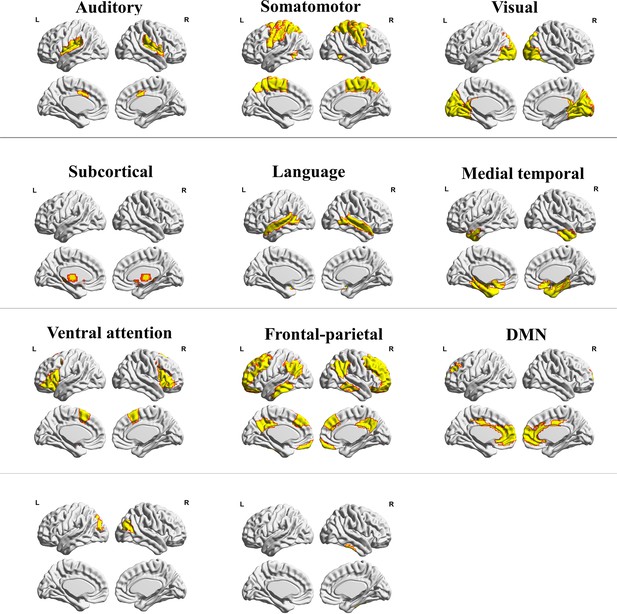
Spatial maps of the nine networks derived from whole-brain parcellation using data from 64 participants engaged in narrative comprehension.
The two unlabeled networks consisted of only one or two nodes each and were, therefore, excluded from further analyses.
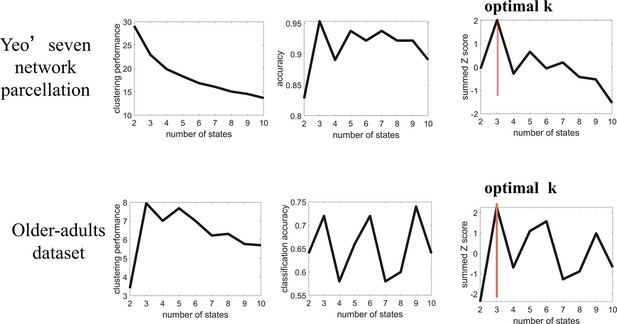
Replicating the identification of the optimal number of states using a different scheme of brain network parcellation and on a dataset of older adults.
We re-analyzed the main dataset using Yeo’s 7-network atlas to parcellate brain networks. Consistently, the model with K = 3 achieved the overall best performance in terms of clustering and accuracy in classifying three narratives. Additionally, we tested a different dataset consisting of older adults who listened to two narratives differing in content and length from the main dataset. In this dataset as well, the model with three states was identified as optimal.
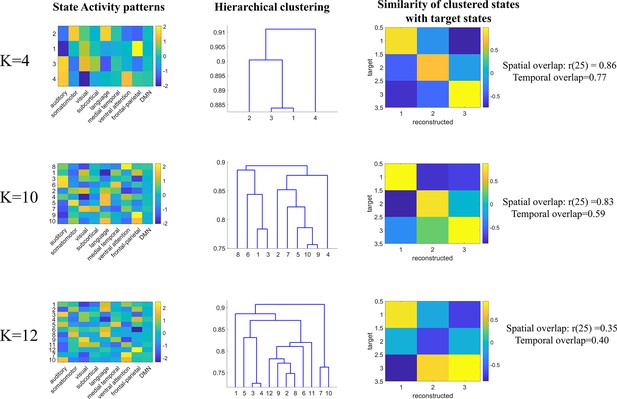
Reconstructing the tripartite-state space from smaller states.
Left and middle panels: States inferred by Hidden Markov Modeling (HMM) with K = 4,10, and 12 were hierarchically grouped into three clusters based on between-states transition probability matrix. Right panels: The reconstructed states (clusters) from the 4- and 10-state models show high and exclusive similarity to the three states (targets) inferred by HMM with K = 3.
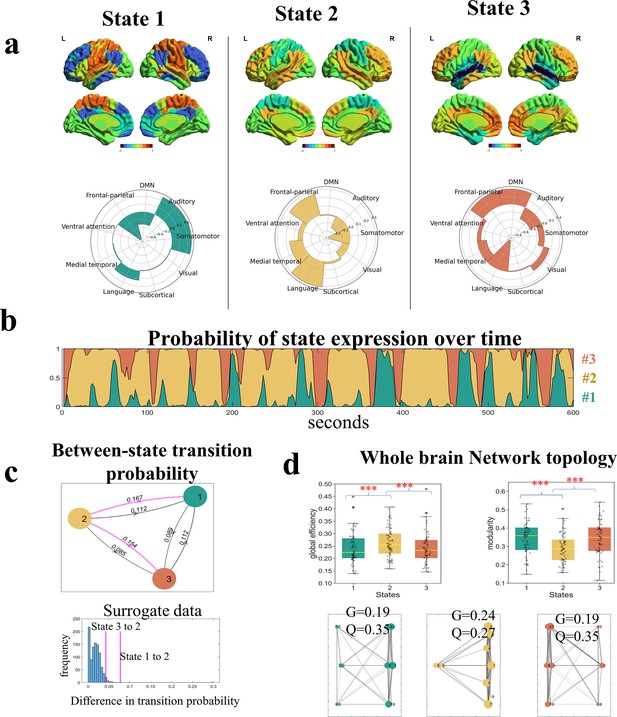
Spatial and temporal features of latent states revealed by Hidden Markov Modeling (HMM).
(a) The activity loadings of each state on the nine networks. These activity values preserved the scale of the original BOLD time courses which were z-score normalized. (b) The ebb and flow of state expression over the time course of narrative comprehension, plotted using data from a representative participant. The curves of the three states are stacked showing the relative strength of activation probability at each time interval. (c) Between-state transition probabilities. Both State #1 and State #3 were more likely to switch to State #2 (denoted by the pink lines) than switching directly to each other. The differences in transition probabilities were larger than most of the instances from surrogate data. (d). Topological properties of whole-brain networks when occupied by each of the three states. Brain occupied by State #2 demonstrated the highest global efficiency (G) and the lowest modularity (Q). The upper panel shows the results of graph constructed using state-specific time series extracted from individual participants (N=64). The lower panel shows results of graph constructed using functional connectivity (FC) matrix derived from the HMM. The error bars indicate standard error of the mean. *p < 0.05, **p < 0.01, ***p < 0.005 for t-tests. See Figure 2—figure supplements 1 and 2 for the replication with a different brain parcellation scheme and with the dataset of older adults.
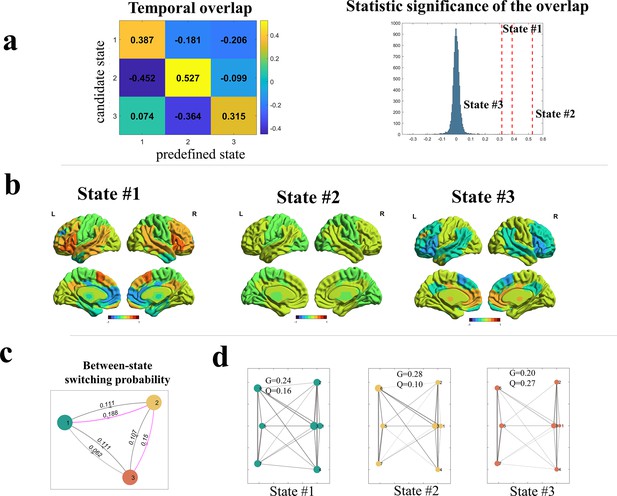
Replicating the spatial and temporal features of tripartite states using Yeo’s 7-network atlas for brain network parcellation.
(a) Establishing State correspondence between parcellation schemes. Each of the three states from the 7-network scheme (treated as candidate states) was best matched to State #1, State #2, and State #3 from the 9-network scheme (treated as predefined states), respectively. The degree of actual overlap for the three states exceeded 100%, 100%, and 99.97% of the permutation instances. (b) Activity patterns of latent states. The network activity values preserved the scale of the original BOLD time courses which were z-scored normalized. (c) Between-state switching probabilities. State #2 was the transitional hub. (d) Topological properties of whole brain networks when occupied by each state. At State #2, the brain exhibited the highest global efficiency and lowest modularity.
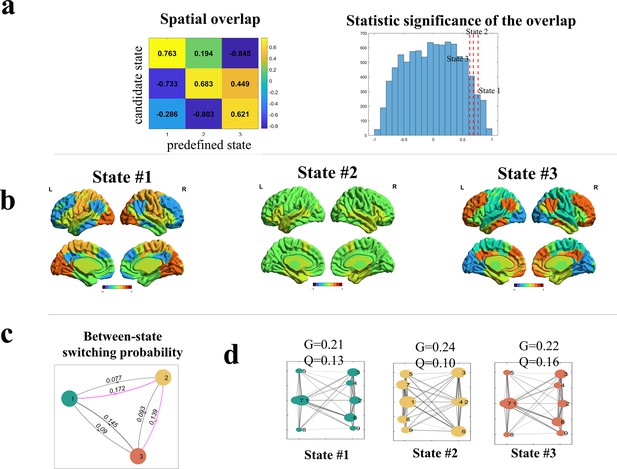
Replicating the spatial and temporal features of tripartite states on the dataset of older adults.
(a) Establishing State correspondence between datasets. Each of the three states from the older-adult dataset (treated as candidate states) was best matched to State #1, State #2, and State #3 from the young-adult dataset (treated as predefined states), respectively. The degree of actual overlap for the three states exceeded 95.7%, 93.04%, and 90.30% of the permutation instances. (b) Activity patterns of latent states. The network activity values preserved the scale of the original BOLD time courses which were z-scored normalized (c) Between-state switching probabilities. State #2 was the transitional hub. (d) Topological properties of whole brain networks when occupied by each state. At State #2, the brain exhibited the highest global efficiency and lowest modularity.
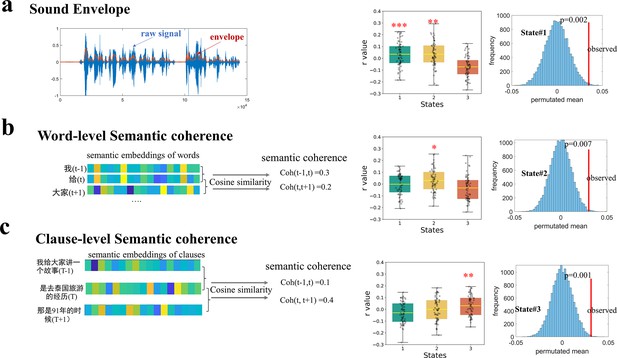
Selective modulation of state expression by different narrative features.
(a) The expression probability of State #1, as well as of State #2, was positively modulated by the temporal envelope of speech. The blue shaded area represents the original amplitude of a speech signal clip, while the orange line shows the smoothed, low-frequency contour of amplitude changes over time (i.e. the sound envelope) (b) Only the expression probability of State #2 was modulated by word-level semantic coherence. (c) Only the expression probability of State #3 was modulated by clause-level semantic coherence. Semantic coherence was measured by cosine similarity between the embeddings (obtained by BERT) of each word (or clause) and the word (clause) immediately before it. Those effects were greater than most instances from permutation where the time courses of state expression were randomly shuffled in a circular manner 5000 times. The error bars indicate standard error of the mean. *p < 0.05, **p < 0.01, ***p < 0.005 for two-tailed t-tests (N=64). See Figure 3—figure supplement 1 for the replication of the modulation effects with a different brain parcellation scheme and with the dataset of older adults.
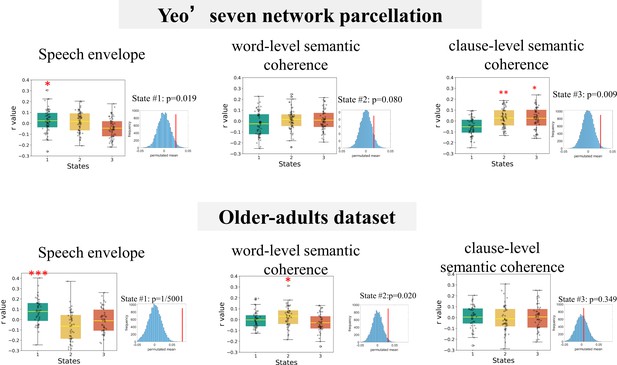
Replicating the modulation effects using a different scheme of brain network parcellation and on a dataset of older adults.
The modulation effects were largely reproducible when applying the Yeo’s 7-network atlas to parcellate the whole brain for the main dataset. Speech envelope significantly modulated the expression of State #1 (t(63) = 1.90, one-tailed p = 0.031). Word-level semantic coherence modulated the expression of State #2 with marginal significance (t(63) = 1.38, one-tailed p = 0.086). Clause-level semantic coherence significantly modulated the expression of both State #2 (t(63) = 2.54, one-tailed p = 0.007) and State #3 (t(63) = 2.02, one-tailed p = 0.023). In the older-adults dataset, speech envelope significantly modulated the expression of State #1 (t(49) = 4.43, one-tailed p < 10-4) . Word-level semantic coherence significantly modulated the expression of State #2 (t(49) = 2.33, one-tailed p = 0.012). However, no significant modulation effect was found for clause-level semantic coherence (all p values > 0.36). The error bars indicate standard error of the mean. *p < 0.05, **p < 0.01, ***p < 0.005githubgithub
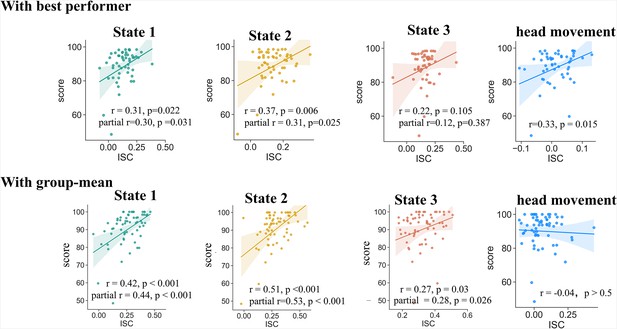
Correlation of state expression with behavior.
Participants' alignment with both the best performer(s) and the group mean in terms of brain state expression predicted their narrative comprehension scores. The alignment with the best performer in head movement trajectory, which probably reflected inter-subject similarity in the fluctuation of task engagement or attention, also correlated with narrative comprehension. After adjusting this effect using partial correlation, the significant correlations between inter-subject alignment in states expression and narrative comprehension still existed. The results were replicated with a different brain parcellation scheme (Figure 4—figure supplement 1), but irreproducible in the older-adults dataset, probably due to substantial heterogeneity among them (Figure 4—figure supplement 2).
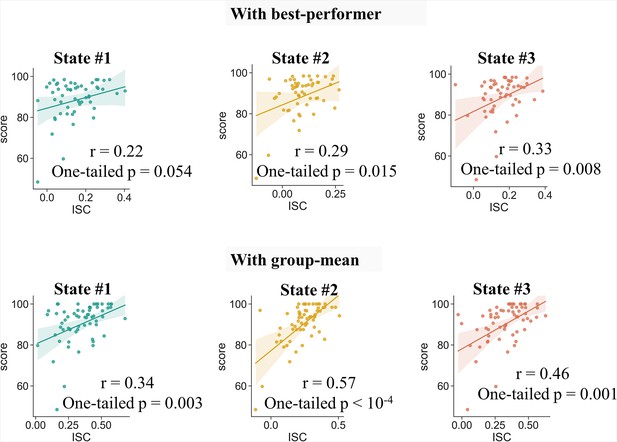
Replicating brain-behavior correlation using the Yeo’s 7-network parcellation scheme.
Consistent with the main results, participants' alignments with both the best performer(s) and the group-mean in brain state dynamics were significantly correlated with their comprehension scores.
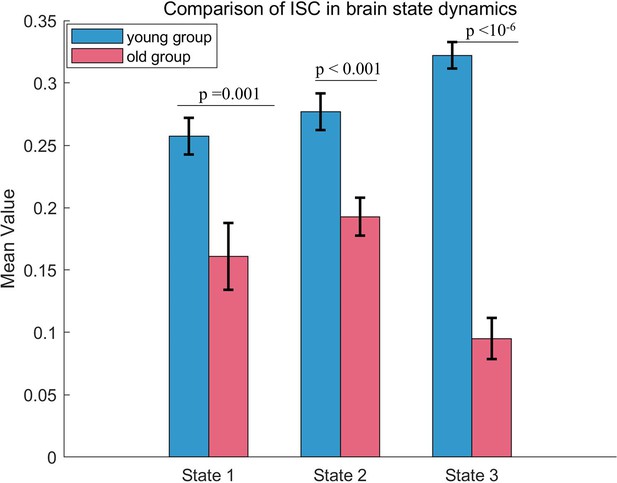
Comparison in inter-subject correlation (ISC) in brain state dynamics between the young and older age groups.
Unlike the young adults, in older adults, we did not find a significant correlation between ISC and speech comprehension. This may be attributed to substantial heterogeneity among older adults arising from task-unrelated factors, which we tested by comparing the ISC between the two datasets. The ISC was assessed by iteratively leaving out one participant at a time. For each left-out participant, we calculated the Pearson correlation between their time course of state expression probabilities and the average time course of the remaining participants, all of whom were all engaged in the same narrative. For all three states, the ISCs were significantly higher among the young adults than that among the older adults. For State #1: t(63, 49) = 3.32, p = 0.001; for State #2: t(63, 49) = 3.94, p < 0.001; and for State #3: t(63, 49) = 11.96, p < 10–6. The error bars indicate standard error of the mean.
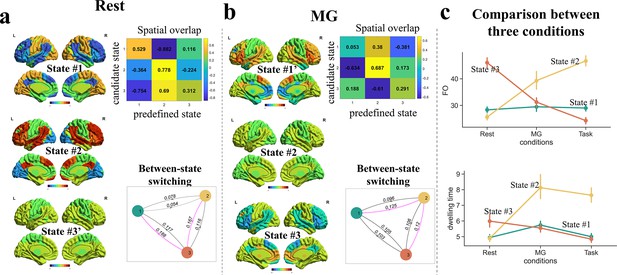
Comparisons of brain states across conditions.
(a) During the rest period, the activity patterns of latent states were similar to those during narrative comprehension, but State #3 became the transitional hub. (b) When listening to the unintelligible narrative (in Mongolian, MG), the activity patterns of latent states varied substantially from that during narrative comprehension, but State #2 was still the transitional hub. (c) The fractional occupation of State#2 increased with greater involvement in linguistic computations, while that of State#3 decreased. A similar pattern was found on the dwelling time of states. The error bars indicate standard error of the mean.