A proteomic chronology of gene expression through the cell cycle in human myeloid leukemia cells
Figures
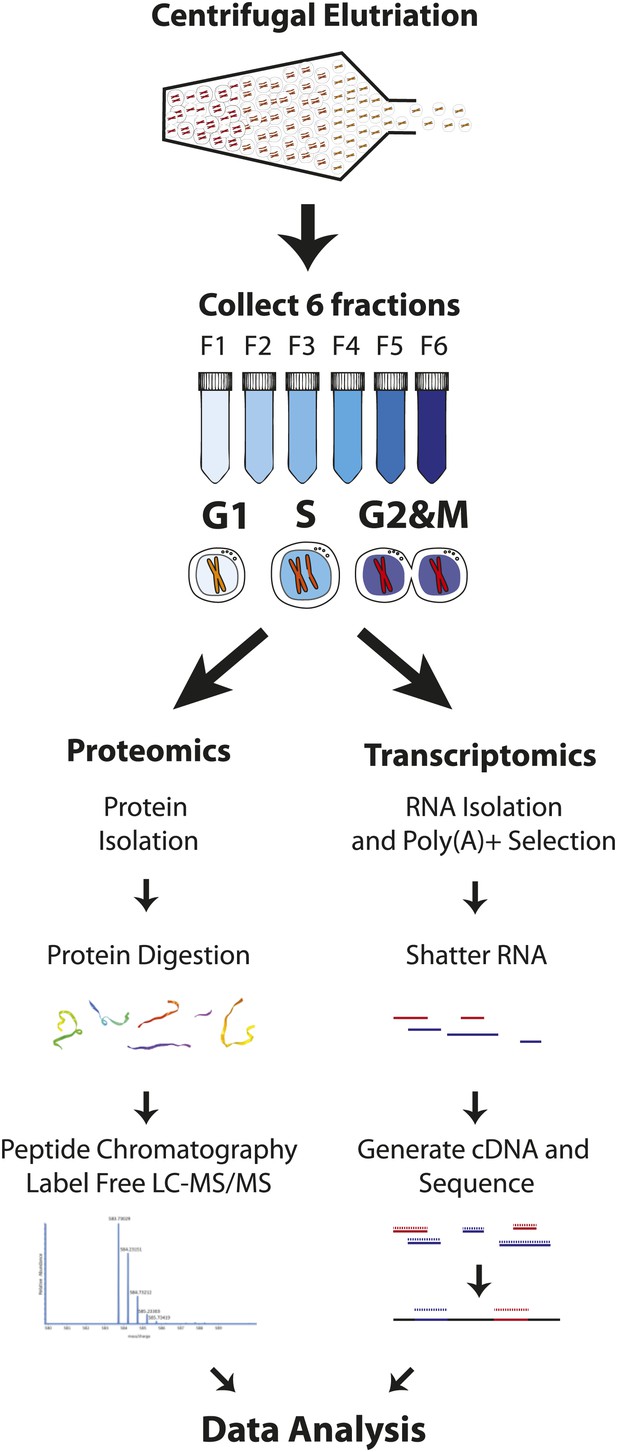
Experimental workflow.
NB4 cells were harvested and fractionated by cell size using centrifugal elutriation. Six fractions were collected and processed separately for transcriptomics and proteomics. For proteomics, cells were lysed and digested with either Lys-C or Lys-C/trypsin. Peptides were then separated by two orthogonal modes of chromatography prior to analysis using an Orbitrap mass spectrometer. Data normalization, peak picking, database searching, peptide and protein identification were performed using the MaxQuant software suite. For transcriptomics, cells from the six fractions were pooled into three (G1, S, and G2&M-enriched fractions). Total RNA was extracted, and subjected to poly(A)+ tail selection. Poly(A)+ transcripts were shattered, reverse transcribed to establish cDNA libraries, which were sequenced using Illumina paired-end sequencing technology. Reads were aligned to the human genome (build hg19) using TopHat, and then used for quantitative gene expression analysis of known protein coding genes using Cufflinks.
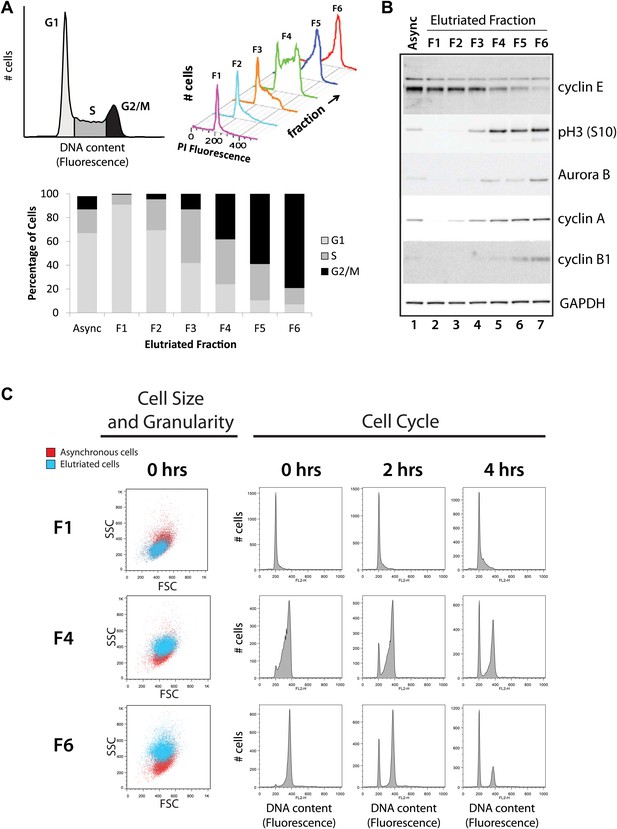
Validation of cell cycle enrichment by centrifugal elutriation.
(A) Cells from asynchronous cells (top left) and each elutriation fraction (top right) were stained with a DNA-binding fluorescent dye and analyzed with flow cytometry. Proportions of cells in each cell cycle phase (bottom) were estimated using the Watson model. Fractions 1 and 2 (F1 and F2) are enriched in G1, fractions 3 and 4 (F3 and F4) are enriched in S, and fractions 5 and 6 (F5 and F6) are enriched in G2 and M phase (G2&M). (B) Immunoblot analyses of the protein lysates for known cell cycle phase-specific markers (cyclin E, phospho-Histone H3 S10, aurora kinase B, cyclin A, and cyclin B1) are consistent with previous literature and the enrichment profiles in (A). (C) Forward scatter and side scatter plots (first column) and cell cycle distributions (remaining columns) for three representative fractions post inoculation (F1, F4, and F6). The forward and side scatter plots for each elutriated fraction are shown in cyan, and are directly compared with the same plot for asynchronous cells, which is shown in red. Cell cycle distributions of the three fractions are measured directly after elutriation (0 hr), and 2 and 4 hr after inoculation into tissue culture medium. Note that the cell cycle distributions shown includes cells with <2N DNA content.
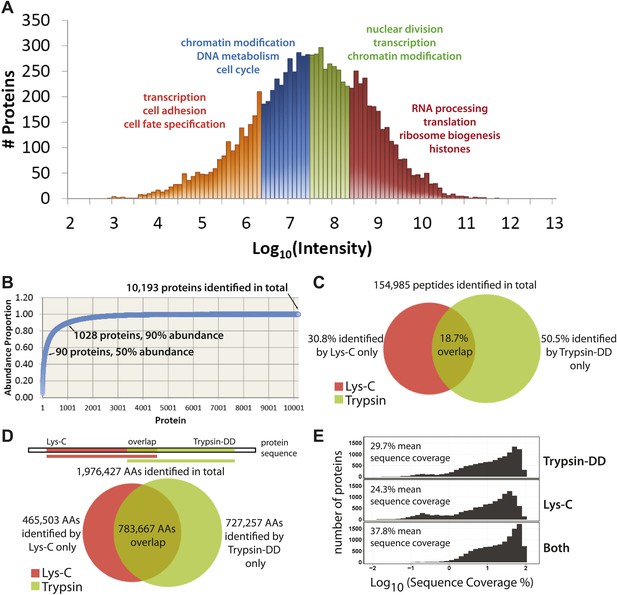
Quantitative, in-depth characterization of a myeloid leukemia proteome.
(A) A histogram of log-transformed protein abundance (iBAQ-scaled protein intensities). Quartile regions are shown in different colors, and enriched gene ontology terms (p<0.01) are shown above each region. (B) A cumulative plot of protein abundance, as estimated using iBAQ-scaled intensities. In total, 10,193 proteins were identified with at least two supporting peptides per protein. Protein abundances follow an exponential increase, with 90 proteins (0.9%) constituting 50% of the bulk protein mass, and 1028 proteins (10%) constituting 90% of the bulk protein mass. The remaining protein identifications (9075 or 89.1%) comprise less than 10% of the bulk protein mass in NB4 cells. (C and D) Venn diagrams showing the total number of sequence-unique peptides (154,985) and amino acid coverage (1,976,427) split by digestion method. Lys-C increases the number of peptides identified by 44% relative to Trypsin-DD. Amino acid coverage was calculated by mapping sequences back to an assembled proteome. Over 30% of the amino acids detected using Lys-C digestion reside in sections of protein sequences that are complementary to Trypsin. In summary, complementary digestion methods substantially increase the overall sequence coverage, as shown in (E). Combining data from both methods boosts the mean sequence coverage to 37.8% with comprehensive proteome depth of over 10,000 proteins.
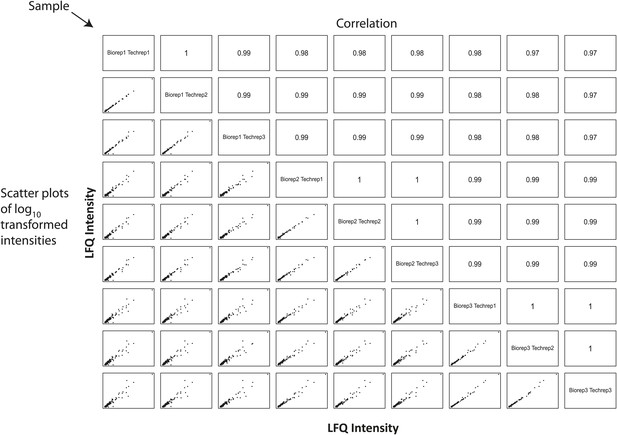
Estimation of technical and biological variances among replicates indicates highly reproducible protein quantitation.
A correlation matrix showing pairwise comparisons between biological and technical replicates of the SingleShot proteomics workflow is shown. Sample identifiers are shown along the diagonal. Log-transformed label-free quantitation (LFQ) intensities are shown along the bottom left corner, and the associated Pearson correlation coefficients are shown along the top right corner. All pairwise comparisons reveal high correlation (>0.95) between replicates, indicating high biological and technical reproducibility.
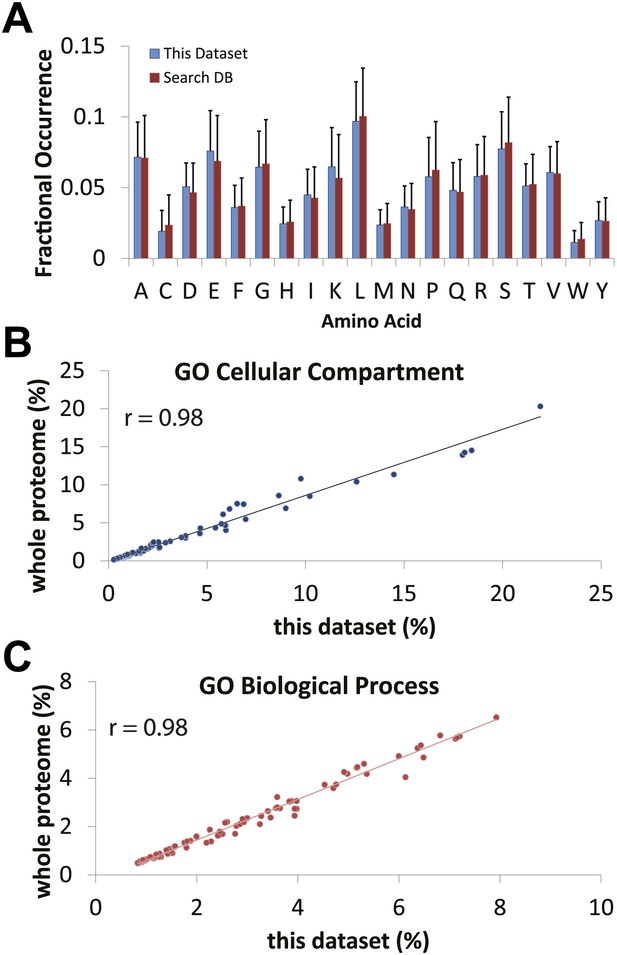
Comparison of expected versus observed amino acid and gene ontology frequencies reveals no major detection bias in the proteomics data set.
(A) The amino acid frequency of identified proteins using the hSAX workflow was compared against the search database (the UniProt Complete Human Reference Proteome). Cellular compartment (B) and biological process (C) gene ontology term frequencies were calculated for the identified data set and the search database. High correlation between the expected frequencies from the search database and the observed frequencies in the identified proteome suggests that the data set is not obviously biased against or for a particular cellular compartment or biological process.
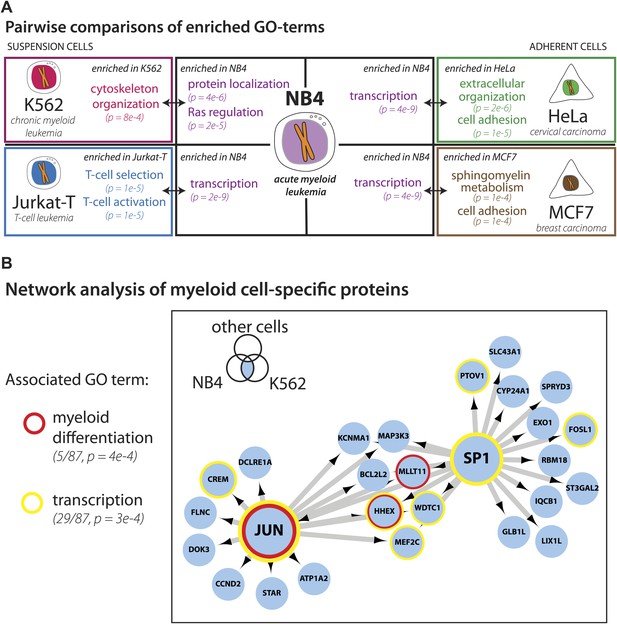
Identification of myeloid-specific factors in the NB4 proteome.
(A) Pairwise comparisons between the NB4 proteome (this study, acute myeloid leukemia) and K562 (chronic myeloid leukemia), Jurkat-T (T-cell leukemia), HeLa (cervical carcinoma), and MCF7 (breast carcinoma) proteomes published by Geiger et al. (2012). Enriched gene ontology terms and the enrichment p-values are shown for each pairwise comparison. The observed cell-line specific gene ontology enrichments are consistent with the developmental origins of the cell lines (immune vs epithelial), and culturing conditions (suspension vs adherent). The NB4 proteome is highly enriched in transcription factors when compared to cell lines that are not in the myeloid lineage (Jurkat-T, HeLa, and MCF7), implying that there is set of shared transcription factors between NB4 and K562 that may be myeloid-specific. (B) A transcriptional regulatory network analysis of proteins identified in myeloid cells (NB4 and K562). Arrows connect transcription factors with their predicted gene substrates (MSigDB). JUN and SP1 appear to be regulatory hubs that can regulate the expression of numerous NB4- and K562-specific genes (Friedman, 2002). Together, these data highlight a protein group that may have important transcriptional regulatory activity in myeloid cells. Circles indicate genes that are annotated as being involved in myeloid differentiation (red) or transcription (yellow).
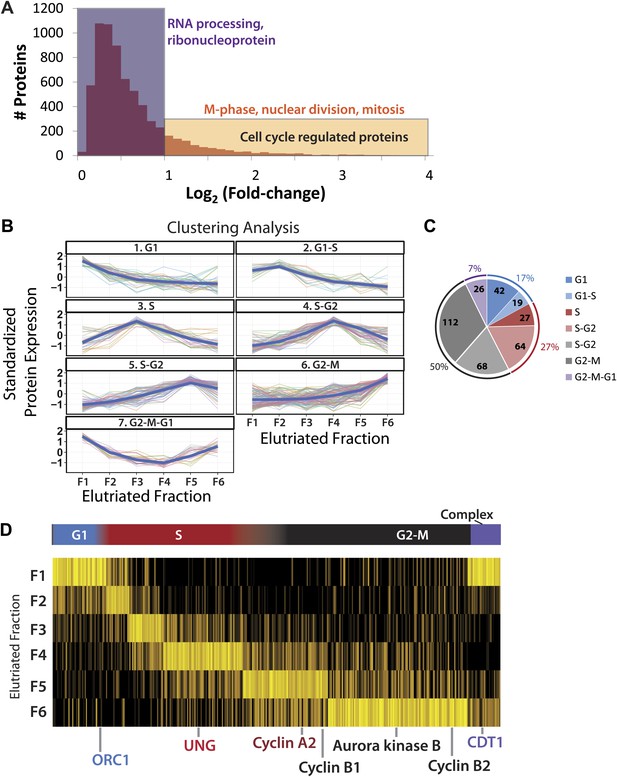
Identification of cell cycle-regulated proteins.
(A) The fold change in label free intensities between any two fractions are shown as a histogram. To identify cell cycle-regulated proteins, an arbitrary fold change cutoff of 2.0 (1.0 in the log2-transformed axis) was set, as indicated by the border between the orange and blue boxes. Highly significant enriched gene ontology terms (p<<0.01) are shown above each group. A twofold change is sufficient to enrich for cell cycle related gene ontology terms, such as M-phase, nuclear division, and mitosis. (B) Clustering of the 358 cell cycle-regulated proteins identified in (A). Scaled protein intensity profiles were clustered by the phase of maximum expression, with the exception of a small minority of proteins that peaked in multiple phases. Graph titles indicate the phase that is enriched in that fraction. (C) The number of cell cycle-regulated proteins split by cluster. Half of the cell cycle-regulated proteins are maximally expressed in the G2&M phase of the cell cycle. (D) Scaled intensities are depicted as a heat map. Each vertical line represents a cell cycle-regulated protein, and the shading indicates the intensity (bright yellow being the most intense). Cell cycle regulators established in the literature are highlighted along the bottom of the heat map, and include cyclins A2, B1, B2, and CDT1.
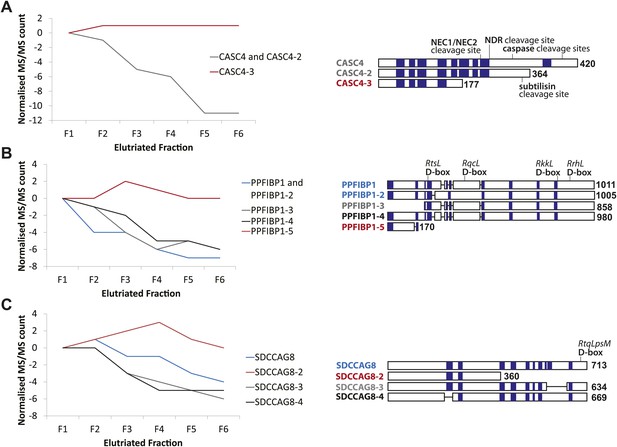
CASC4, PPFIBP1, and SDCCAG8 are examples of ORFs that encode multiple splice isoforms that behave differently across the cell cycle.
Protein spectral count profiles across the six elutriated fractions for three open reading frames (ORFs) showing protein-level isoform-specific cell cycle variation: CASC4 (A), PPFIBP1 (B), and SDCCAG8 (C). Isoform sequences are shown schematically above each graph. Sequence regions for which direct peptide evidence was detected are shaded in blue, and sequence motifs known to be important in post-translational regulation are indicated.
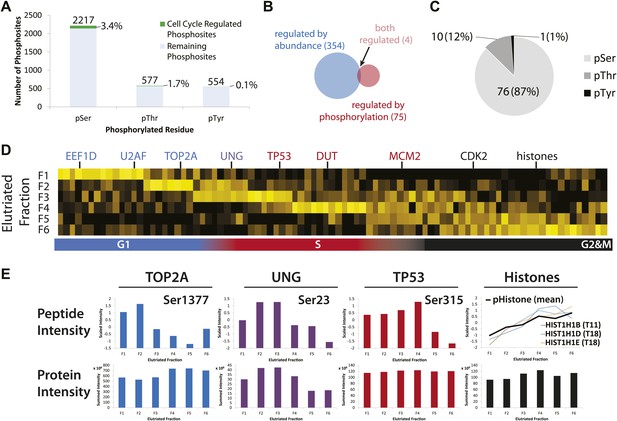
Identification of cell cycle-regulated phosphopeptides.
(A) A total of 2761 phosphorylation sites were identified without phosphopeptide enrichment, which are shown split by residue (Ser, Thr, and Tyr). Cell cycle regulated phosphosites are shown in green. The numbers on top of each bar indicate the total number of pSer, pThr, and pTyr residues detected, respectively. The proportions of cell cycle-regulated pSer, pThr, and pTyr, relative to the total pSer, pThr, and pTyr sites detected respectively, are shown as percentages. (B) Overlap between proteins whose abundances are cell cycle regulated and proteins whose phosphorylation is cell cycle regulated. (C) A breakdown of cell cycle-regulated phosphosites by residue. The number and the percentage of phosphosites relative to the total number of cell cycle regulated phosphosites are shown. (D) Scaled phosphopeptide intensity profiles plotted as a heatmap. Representative cell cycle-regulated phosphorylations that are established in the literature are shown along the top of the heatmap. (E) Scaled phosphopeptide and summed protein intensity profiles for four cell cycle regulated phosphorylated proteins (TOP2A, UNG, TP53, and histones). The peptide intensity graphs are annotated with the mapped phosphorylation site. For histones, several phosphopeptide profiles (light purple, light blue, and light orange) and the average (black) are shown on the same graph. The total histone intensity is calculated as the sum of all histones identified.
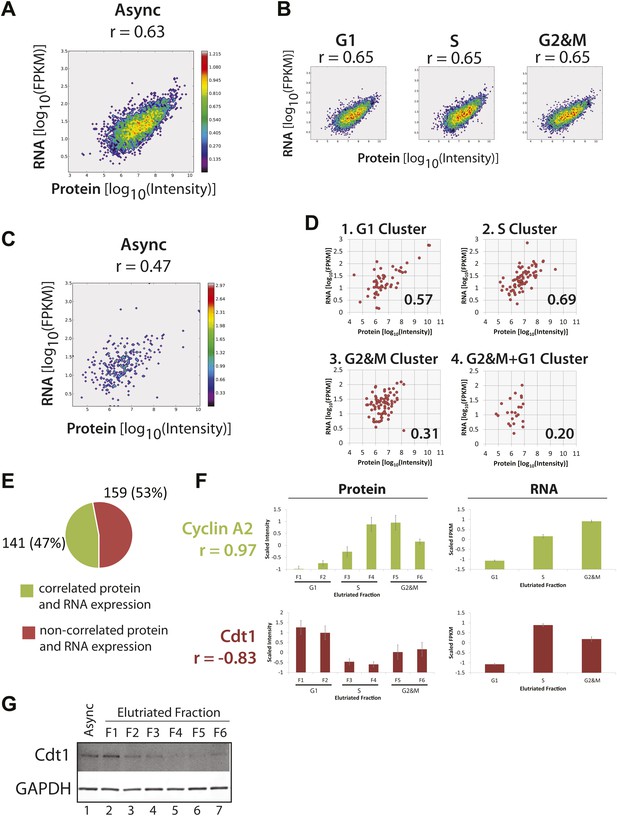
Correlation of protein and RNA levels across the cell cycle.
Log-transformed, iBAQ-scaled protein intensities and log-transformed FPKM values (RNA) from asynchronous cells (A) and cell cycle fractions (B). RNASeq data are expressed as Fragments Per Kilobase of exon per million fragments Mapped (FPKM), which is a proxy for RNA copy number. Histone genes were removed from the analysis due to the absence of poly(A)+ tails in histone-encoding messages. Each graph is annotated with the calculated Spearmen correlation coefficients (r). (C) Correlation of the protein and mRNA abundances in asynchronous cells of the 358 proteins whose abundances are cell cycle regulated (r = 0.45). (D) The same data shown in (C), but split by protein clusters as described in Figure 5. (E) Correlation of the expression profiles of the 358 cell cycle regulated proteins and their associated transcripts. Genes were classified into two groups based on protein and RNA expression correlation (Pearson’s correlation coefficient greater than or equal to 0.5). (F) Cyclin A2 and Cdt1 are examples highlighted from the groups in (E). Protein and RNA abundance standard errors were calculated from the variance in scaled peptide intensities and biological replicates, respectively. (G) Immunoblot analysis of Cdt1 and GAPDH protein expression across asynchronous and elutriated NB4 cells.
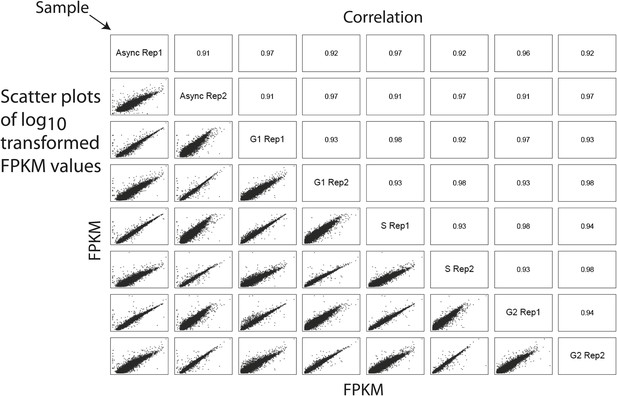
Analysis of technical and biological variance among duplicates reveals highly reproducible RNA quantitation.
A correlation matrix showing pairwise comparisons between biological and technical replicates of the RNASeq transcriptomics workflow. Sample identifiers are shown along the diagonal. Log-transformed FPKM values are shown along the bottom left corner, and the associated Pearson correlation coefficients are shown along the top right corner. All pairwise comparisons reveal high correlation (>0.90) between replicates, indicating high biological and technical reproducibility.
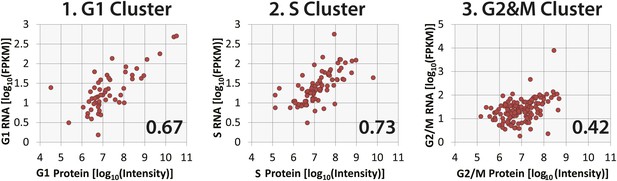
Correlation of protein and RNA abundances of cell cycle-regulated proteins.
Comparison of protein and RNA abundances in G1, S, and G2&M phases of the cell cycle for proteins that peak in G1, S, and G2&M, respectively. Spearman correlation coefficients, which are shown in the inset, follow the same trend as observed in Figure 8D, with G2&M-peaking proteins having the poorest protein and mRNA abundance correlation.
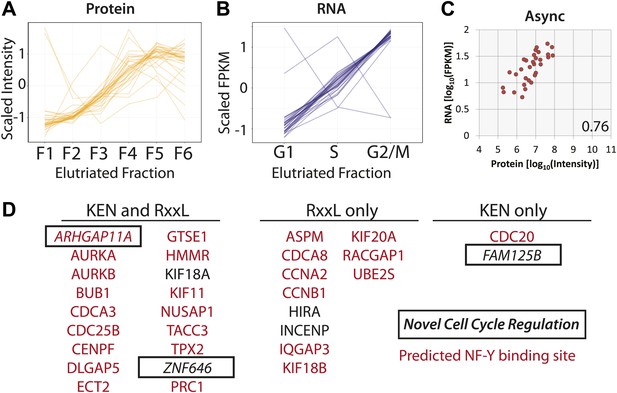
Protein and RNA levels are correlated for the specific subset of cell cycle-regulated proteins whose cognate mRNA change by 1.5-fold.
Of the 358 proteins whose abundances are cell cycle regulated, the cognate mRNA of 31 proteins also changes across the elutriated fractions by more than 1.5-fold. Scaled protein (A) and mRNA expression profiles (B) are shown as line graphs for these 31 genes, respectively. (C) Comparison of protein and mRNA abundances in asynchronous cells reveals a Spearman correlation of 0.76. (D) 27 of the 31 genes have predicted NF-Y binding sites in their promoters, and all 31 encode proteins containing a KEN or D-box sequence degron. KEN-motifs are especially enriched (>eightfold enrichment, compared to 7% expected by random chance). Three genes have not been previously annotated as being cell cycle regulated: FAM125B, ZNF646, ARHGAP11A.
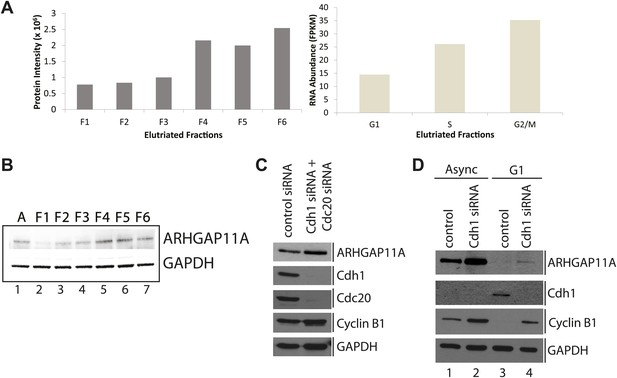
Identification of ARHGAP11A as a cell cycle regulated protein and a substrate of the APC/C.
(A) MS and RNA-Seq quantitation for ARHGAP11A protein (left) and mRNA (right), respectively. (B) Immunoblot analysis of ARHGAP11A (HPA antibody) and GAPDH protein expression across asynchronous and elutriated NB4 cells. (C) Lysates from U2OS cells treated with either a non-targeting control siRNA (lane 1) or siRNAs targeting Cdh1 and Cdc20 (lane 2) were probed for levels of ARHGAP11A, Cdh1, Cdc20, cyclin B1, and GAPDH by immunoblot. (D) Asynchronous or serum-starved RPE-1 cells were treated with either a non-targeting control siRNA or an siRNA against Cdh1. Lysates were then probed with antibodies against ARHGAP11A (Bethyl antibody), Cdh1, cyclin B1, and GAPDH.
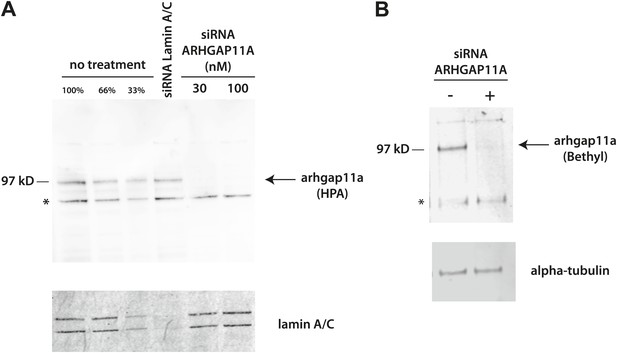
Validation of anti-ARHGAP11A antibodies by siRNA-based depletion of ARHGAP11A protein.
(A) HeLa cells were either mock treated, treated with siRNAs that target lamin A/C, or treated with different concentrations of siRNAs that target ARHGAP11A. Cells were cultured for 48 hr before harvest, lysis, and immunoblot analysis with a Human Protein Atlas (HPA) antibody recognizing ARHGAP11A (top) or an antibody recognizing lamin A/C (bottom). The anti-ARHGAP11A recognizes a band at the correct molecular weight (∼100 kDa), which is significantly decreased upon siRNA depletion of ARHGAP11A protein. Note that lamin A/C levels are not significantly perturbed by treatment of siRNA targeting ARHGAP11A. (B) U2OS cells were either mock treated or treated with siRNAs that target ARHGAP11A, incubated for 48 hr, lysed, and immunoblotted with antibodies recognizing ARHGAP11A (Bethyl, top) or alpha-tubulin (bottom). The anti-ARHGAP11A recognizes a band at the same molecular weight as the HPA antibody that is significantly decreased upon siRNA depletion of ARHGAP11A. Note that alpha-tubulin levels are unchanged +/− ARHGAP11A siRNA. *Non-specific bands.
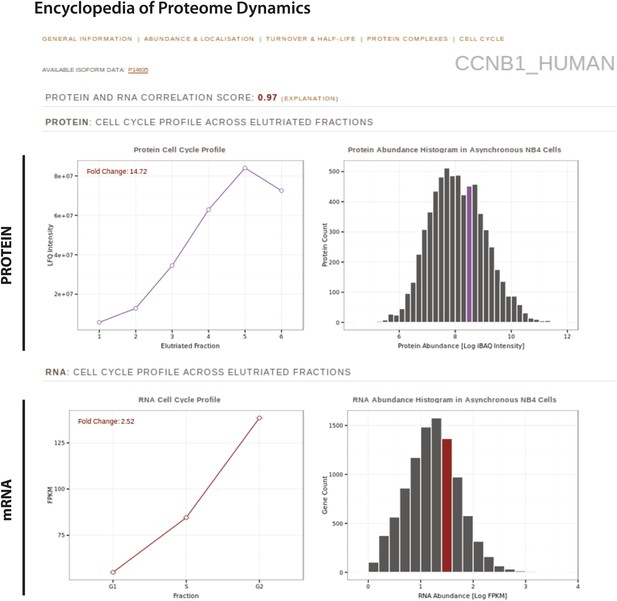
The Encyclopedia of Proteome Dynamics, a fully searchable, open-access online repository of proteome data.
Quantitative protein and RNA data from this study are available through the Encyclopedia of Proteome Dynamics (EPD). A screenshot of the EPD is shown, which displays protein and mRNA expression profiles across the elutriated fractions, the calculated Pearson correlation coefficient between the protein and mRNA profiles, and protein and mRNA abundances in asynchronous cells for cyclin B1 (CCNB1_HUMAN).
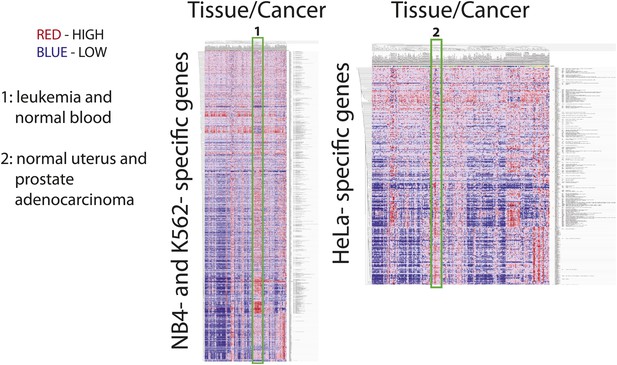
Many cell-line specific genes are overexpressed in tumors and normal tissues that are associated with the developmental origin of the cell line.
mRNA expression heatmaps from the Broad Global Cancer Map for NB4- and K562- specific genes (left) and HeLa-specific genes (right). Each heatmap has tissue along the horizontal axis and gene along the vertical axis. Vertical red streaks indicate that many genes are similarly overexpressed in a particular tissue. Many NB4- and K562-specific genes are overexpressed in lymphoid, leukemia, and normal hematopoietic tissues, whereas HeLa-specific genes are overexpressed in normal uterine tissues and prostate tumors.
Tables
Enriched functional annotations among the cell cycle varying proteins
Peak phase | Functional annotation | Proteins | % of total | p value |
---|---|---|---|---|
G1 (42 proteins) | transcription cofactor activity | 5 | 12% | 0.003 |
transcription factor binding | 5 | 12% | 0.012 | |
S (110 proteins) | phosphoprotein | 82 | 75% | 1.2E-07 |
E2F* | 82 | 75% | 0.002 | |
DNA metabolic process | 10 | 9% | 0.012 | |
positive regulation of gene expression | 8 | 7% | 0.037 | |
cell cycle | 11 | 10% | 0.009 | |
G2/M (180 proteins) | M phase | 26 | 14% | 7.5E-20 |
cell cycle | 41 | 23% | 3.9E-19 | |
phosphoprotein | 94 | 52% | 1.8E-06 | |
NFY* | 93 | 52% | 3.6E-05 | |
Complex (26 proteins) | STAT3* | 20 | 77% | 0.003 |
nucleotide-binding | 7 | 27% | 0.015 |
-
Proteins were partitioned into four categories by peak phase and analyzed for functional annotation enrichment. Functional annotations include gene ontology terms and predicted transcription factor binding sites in the promoter region of the encoding gene. Enriched annotations, their enrichment p values, the number and percentage of proteins with the specified annotation are shown.
-
*
Transcription factor binding sites from the UCSC TFBS database.
Additional files
-
Supplementary file 1
Proteomics data set.
This file summarizes the proteins identified and quantified in asynchronous NB4 cells and in the fractions produced by elutriation, and includes the following data for each protein identification: protein and gene identifiers, protein descriptions, sequence coverage, the number of supporting peptides, the posterior error probabilities (PEPs), the extracted ion chromatogram (XIC) intensities, the LFQ-normalized intensities, the iBAQ-scaled intensities, and mapped transcript FPKM values from the RNA-Seq data.
- https://doi.org/10.7554/eLife.01630.021
-
Supplementary file 2
Cell line proteome meta-analysis.
Comparison of the proteomic data set obtained for NB4, a human promyelocytic leukemia cell line that grows in suspension culture, with other recent examples of in depth proteomic analysis of different human cell lines, most of which are adherent tumor cell lines of either fibroblast or epithelial origin. In total, the meta-analysis included protein data from 14 cell line proteomes: 3 × HeLa, 2 × U2OS, A549, GAMG, HEK293, K562, LnCap, MCF7, RKO, HepG2, and Jurkat-T (Lundberg et al., 2010; Beck et al., 2011; Nagaraj et al., 2011; Geiger et al., 2012), which were consolidated and mapped to Ensembl Genes prior to comparison. The combined data set provides evidence of protein-level expression of over 11,000 genes. Of these, a common set of ∼3000 genes are identified by protein data from all these cell lines, defining a core, shared proteome (Columns D and E), and >1000 genes are uniquely detected in this analysis of NB4 cells (Columns A and B). A focused comparison of NB4, K562, Jurkat-T, HeLa and MCF7 cell line proteomes reveals ∼90 genes that are specifically expressed in myeloid cell lines NB4 and K562 (Columns G and H).
- https://doi.org/10.7554/eLife.01630.022
-
Supplementary file 3
Proteins whose Abundance is cell cycle regulated.
For quantitation, the proteomic data set was filtered to only include proteins that were detected in asynchronous cells and all six elutriation fractions. Of these ∼6500 proteins, 358 (∼5.5%) are proteins whose abundance is cell cycle regulated (i.e., varies in abundance by at least two-fold across the fractions). These proteins vary in expression profile, and cluster into seven distinct groups that differ primarily in peak fraction. Gene and protein identifiers, cluster membership, and motifs that are predicted to modulate post-translational regulation are provided below. Other than the Dbox (R-x-x-L from King et. al, Mol. Biol. Cell 1996, 7, 1343-1357), motif sequences were obtained from the Eukaryotic Linear Motif resource (ELM).
- https://doi.org/10.7554/eLife.01630.023
-
Supplementary file 4
Phosphopeptide dataset.
This file summarizes the ∼2700 phosphopeptides identified and quantified in asynchronous NB4 cells and in the fractions produced by elutriation, and includes the following data for each phosphopeptide identification: protein and gene identifiers, protein descriptions, the phosphopeptide sequence, localization scores and probabilities, posterior error probabilities (PEPs), the Andromeda search scores, the mass error, and the extracted ion chromatogram (XIC) intensity.
- https://doi.org/10.7554/eLife.01630.024
-
Supplementary file 5
Proteins whose phosphorylation is cell cycle regulated.
This file summarizes the cell cycle varying phosphopeptides that were identified without phospho-specific enrichment. These phosphosites were filtered to only include phosphopeptides that were independently identified in asynchronous cells and in all elutriation fractions. A minor fraction of these phosphopeptides (89 phosphopeptides, or 3% of the total phosphopeptides identified in this data set, corresponding to 79 phosphoproteins) vary by at least two-fold across the elutriation fractions. Cell cycle regulated phosphopeptides are listed below with Andromeda database search scores, localization probabilities, posterior error probabilities (PEPs), and intensities in each fraction.
- https://doi.org/10.7554/eLife.01630.025
-
Supplementary file 6
RNA-Seq data set.
This file provides gene identifiers, counts, and data quality markers for protein coding genes identified in any of the elutriated samples. The six elutriated fractions were pooled into three samples (F1+F2, F3+F4, F5+F6). mRNA was then separately extracted from these pooled samples using oligo dT beads, fragmented, then reverse transcribed using random hexamers. The cDNA was then sequenced using paired ends reads at a length of 75 bp. Each sample was run on a single lane of an Illumina HiSeq, to improve coverage of lower abundance transcripts. The paired-end RNA-Seq data were then aligned to the human genome (build hg19), using TopHat, without providing a gene reference (to avoid forced mappings). Following duplicate removal using Picard’s MarkDuplicate (http://picard.sourceforge.net), we quantified the gene expression of known protein coding genes using Cufflinks (Trapnell et al., 2013). Genes with low data quality were removed from subsequent data analysis.
- https://doi.org/10.7554/eLife.01630.026