Pervasive transcription read-through promotes aberrant expression of oncogenes and RNA chimeras in renal carcinoma
Figures
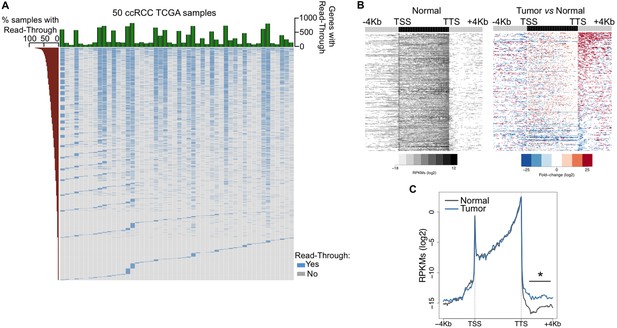
Transcription read-through is prevalent in ccRCC.
(A) Top graph depicts the number of genes with transcription read-through per ccRCC sample. The heatmap illustrates the genes with (blue) and without (grey) transcription read-through. The left graph indicates the percentage of samples on which read-through is observed for each individual gene. (n = 50 tumor/matched normal ccRCC TCGA samples). (B) Heatmap representation of the RNA-seq profile distribution and fold change after the TTS region of genes with transcription read-through in one representative TCGA ccRCC sample (patient barcode TCGA-CZ-5465) of a total of 50 tumor and matched pairs analysed. The gene body region was scaled to 60 equally sized bins and ± 4 Kb gene-flanking regions were averaged in 100-bp windows. The left panel shows the read counts (log2 RPKMs) of the matched normal tissue in all genes with read-through and the right panel shows the fold-change (log2) of read counts between the tumor and the matched normal tissue. Genes are ordered according to the read-through length. Scales and colour keys for each panel are depicted in the bottom. (C) Metagene analysis of RNA-seq profiles for tumor and matched normal tissue from one ccRCC patient. *p<0.05 by Student’s T-test.
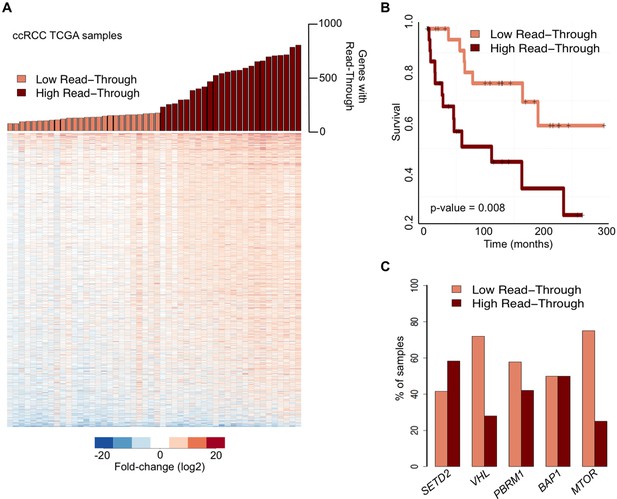
Transcription read-through correlates with ccRCC survival rates.
(A) The top graph indicates the number of genes with transcription read-through on each ccRCC patient sample. Samples were split in two groups according to the number of genes with transcription read-through (low or high), using 200 genes as a cut-off. The heatmap represents the RNA-seq tumor/matched normal fold change 4 Kb after the TTS region of genes with transcription read-through. (B) Kaplan-Meier plot comparing the survival of patients separated into ‘high read-through’ and ‘low read-through’ subsets as defined in A. (C) Proportion of ccRCC patient samples with low and high transcription read-through. Results are shown for samples containing any of the most recurrently mutated genes in ccRCC: SETD2, VHL, PBRM1, BAP1 and MTOR. Proportions of high and low read-through were significantly different between samples carrying mutation in SETD2 and in any of the remaining genes (Fisher’s Exact Test p<0.05).
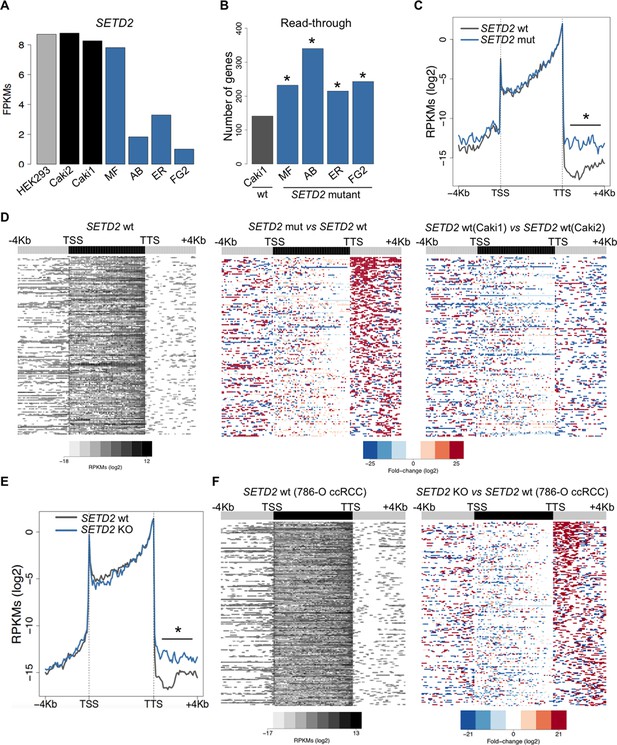
SETD2 mutations promote transcription read-through in ccRCC.
(A) SETD2 expression levels (FPKMs) in HEK293 and ccRCC cell lines. (B) Number of genes with transcription read-through up to 4 Kb downstream the TTS. *p<0.05 by Fisher’s Exact Test after comparing each SETD2 mutant cell line with the SETD2 wt cell line (Caki1); (C) Metagene analysis of genes showing transcription read-through in SETD2 mutant and wt ccRCC cell lines. The gene body region was scaled to 60 equally sized bins and ± 4 Kb gene-flanking regions were averaged in 100-bp windows. *p<0.05 by Student’s T-test; (D) Heatmap representation of RNA-seq profile distribution and fold change after the TTS region of genes showing transcription read-through. Genes were scaled and averaged as in C. The left panel shows the read counts (log2 RPKMs) of the SETD2 wt cell line (Caki2) in all genes with read-through. The two right panels show the fold-change (log2) between each ccRCC cell line and the reference SETD2 wt ccRCC cell line (Caki2). Genes are ordered according to the read-through length. Scales and colour keys for each panel are depicted at the bottom of the panel. (E) Metagene analysis (as detailed in C) of genes showing transcription read-through in SETD2 KO and wt 786-O ccRCC cells. *p<0.05 by Student’s T-test. (F) Analysis as described in D of RNA-seq data from SETD2 KO and control 786-O ccRCC cell lines.
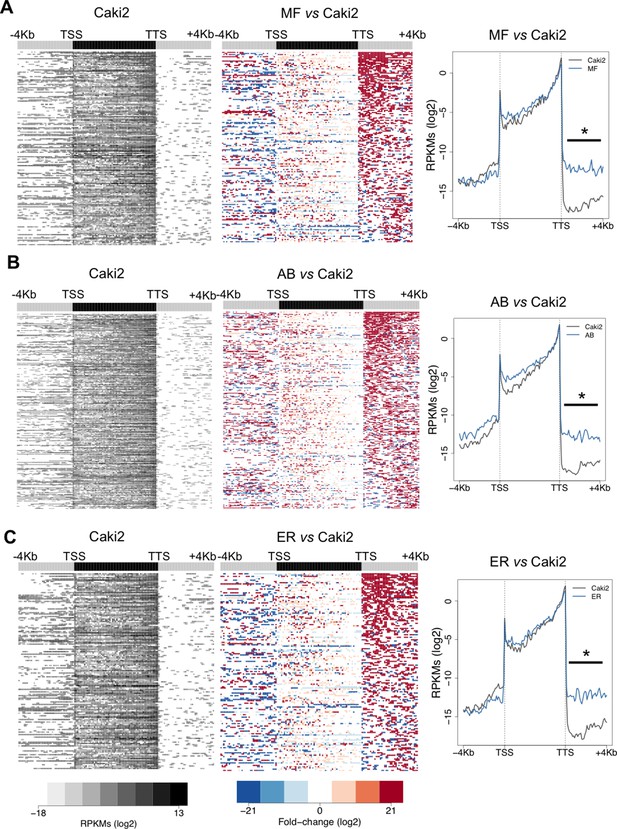
SETD2 mutations in ccRCC cells promote transcription read-through.
Heatmap and metagene of RNA-seq profiles for the following SETD2 mutant ccRCC cell lines: MF (A), AB (B) and ER (C). Heatmap representation of RNA-seq profile distribution and fold change after the TTS of genes with transcription read-through. Gene body was scaled to 60 equally sized bins and ± 4 Kb gene flanking regions were averaged in 100-bp window. Genes are ordered according to the length of the read-through. Left panel shows read counts (log2 RPKMs) of the SETD2 wt cell line (Caki2) in all genes with read-through. The right panel shows the fold-change (log2) for SETD2 mutant vs SETD2 wt (Caki2) ccRCC cell lines. Metagene of RNA-seq profiles for SETD2 mutant and wt ccRCC cell line of genes showing transcription read-through. *p-value <0.05 by Student’s T-test. Scales and color keys for each panel are depicted at the bottom of the panel.
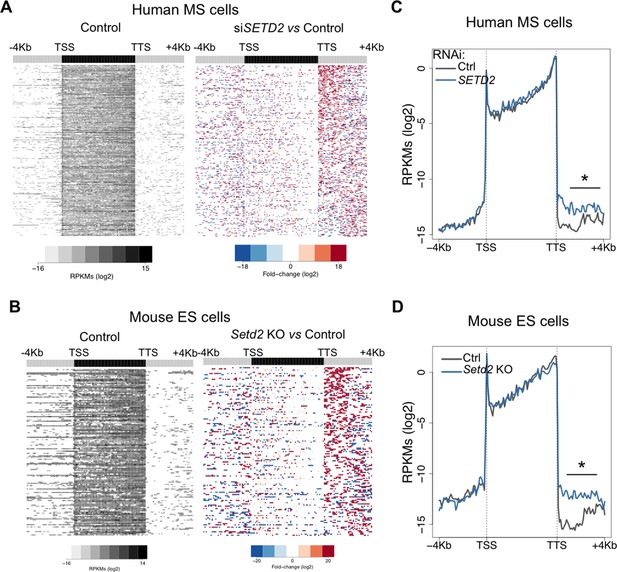
SETD2-depletion impairs transcription termination.
(A,B) Heatmap representation of RNA-seq profile distribution and fold change after the TTS region of genes showing transcription read-through. The left panel shows the read counts (log2 RPKMs) of all genes with read-through in control cells. The two right panels show the fold-change (log2) between SETD2-depleted and control human MS cells (A) and Setd2 KO and wt mouse ES cells (B). Genes are ordered according to the read-through length. Scales and colour keys for each panel are depicted at the bottom of the panel. (C,D) Metagene analyses of RNA-seq profiles of control and SETD2-depleted human MS cells (C) and of Setd2 KO and wt mouse ES cells (D). *p-value <0.05 by Student’s T-test.
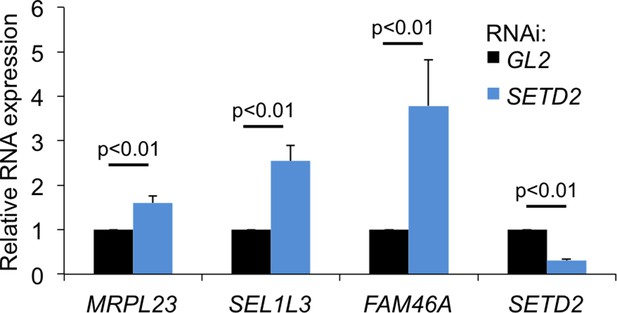
SETD2-depletion impairs transcription termination in ccRCC cells.
Caki2 cells were transfected with siRNA oligos against SETD2 or the firefly luciferase (GL2) (control) 48 hr before RNA extraction. RT-qPCR was carried out with primers designed to detect transcription read-through in MRPL23, SEL1L3 and FAM46A. The levels of SETD2 mRNA expression are also shown. The amount of PCR product estimated by RT-qPCR was normalized to the levels of U6 snRNA.
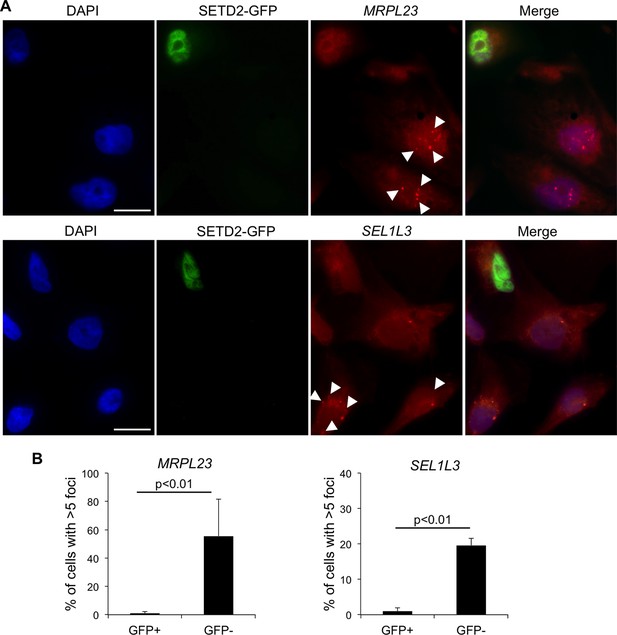
SETD2 rescues the transcription termination defects of SETD2 mutant ccRCC cells.
(A) RNA FISH experiments on a SETD2-mutant ccRCC cell line (FG2) transiently expressing wt SETD2-GFP. Quasar570-labeled probes were designed against a region downstream the termination sites of MRPL23 and SEL1L3. The arrows indicate single RNA transcripts generated by a transcription read-through event. (B) Quantification of the number of GFP-negative and GFP-positive cells containing more than 5 RNA FISH foci. Means and standard deviations from at least 50 cells from four individual experiments are shown. Scale bars: 10 μm. p<0.01 by Student’s T-test.
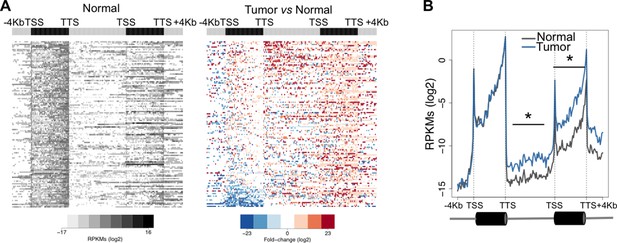
Transcription read-through overruns and interferes with the expression of neighbouring genes.
Heatmap (A) and metagene (B) profiles of the intergenic region and of the gene located downstream of a read-through event in one representative ccRCC TCGA sample (patient barcode TCGA-CZ-5465). Genes were scaled and averaged as in Figure 1. *p<0.05 by Student’s T-test.
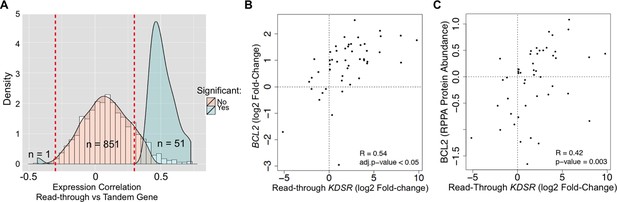
Transcription read-through of the KDSR gene correlates with the expression of the BCL2 oncogene.
(A) Distribution of the correlation between the read-through and the expression levels of the downstream tandem genes. Significant correlation values (Benjamini-Hochberg adjusted p<0.05) are represented in blue. (B) Correlation between the expression levels of BCL2 and the read-through of the upstream KDSR gene. The graph depicts the fold-change of read counts for each tumor and matched normal pair. (C) Correlation between BCL2 protein levels and the KDSR read-through.
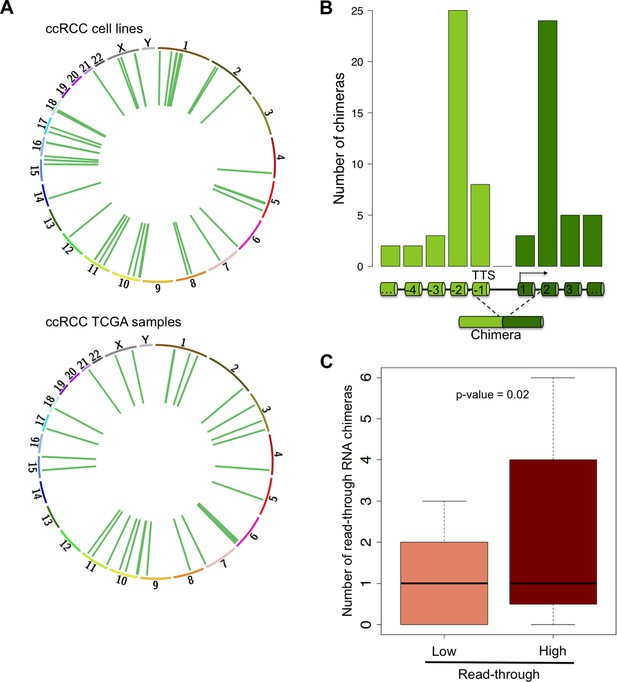
Read-through RNA chimeras are prevalent in ccRCC.
(A) Circos plots showing the location of genes forming each RNA chimera detected on human ccRCC cell lines and TCGA samples. Chimeras are represented by curves inside the Circos. (B) Number of read-through RNA chimeras formed by intergenic splicing between the represented exons. (C) Number of read-through RNA chimeras in the low and high read-through sample subsets defined in Figure 2A.
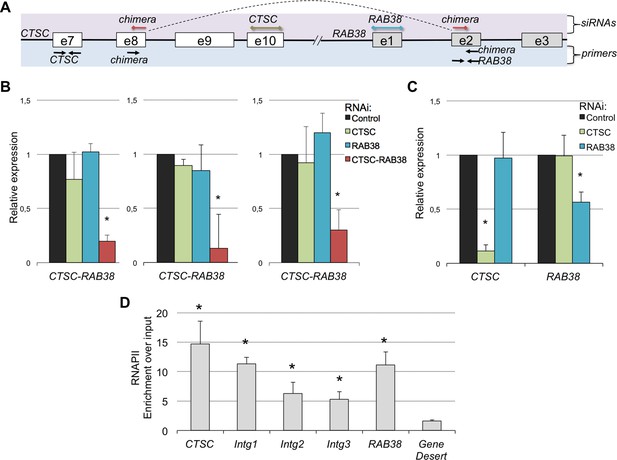
The CTSC-RAB38 chimera is recurrently detected in ccRCC.
(A) Schematic illustration of the CTSC-RAB38 locus depicting the position of the primers used to measure the transcripts levels by RT-qPCR shown in (B) and (C) and the position of the siRNAs targeting each of the three transcripts (CTSC, RAB38 and the CTSC-RAB38 chimera). The dashed curve in the scheme illustrates the splicing pattern of the chimeric transcript. (B) Relative expression of the CTSC-RAB38 chimeric transcript after depletion of the indicated transcripts by RNAi in three distinct ccRCC cell lines (FG2, MF, ER). (C) Relative expression of CTSC and RAB38 upon depletion of the indicated transcripts by RNAi in FG2 cells. Similar results were obtained with the other ccRCC cell lines. *p<0.05 by Student’s T-test compared to controls. (D) RNAPII ChIP along the CTSC-RAB38 locus in FG2 cells. Means and standard deviations from five independent experiments are shown. *p<0.05 by Student’s T-test compared to the gene desert.
Additional files
-
Supplementary file 1
(A) Genes with transcription read-through identified in human ccRCC TCGA samples. (B) Genes involved in transcription termination and pre-mRNA processing with mutations in TCGA samples. (C) Genes with transcription read-through identified in human ccRCC cell lines. (D) Genes with transcription read-through identified in human 786-O ccRCC cells. (E) Genes with transcription read-through identified in human mesenchymal stem cells. (F) Genes with transcription read-through identified in mouse embryonic stem cells. (G) Genes with transcription read-through identified in human ccRCC TCGA samples, showing significant correlation between read-through levels and expression of downstream gene. (H). Read-Through RNA chimeras identified in human ccRCC TCGA samples and ccRCC cell lines. (I). siRNAs and Primer Sequences. (J) Sequences of RNA FISH probes.
- https://doi.org/10.7554/eLife.09214.014