Cell assemblies at multiple time scales with arbitrary lag constellations
Figures
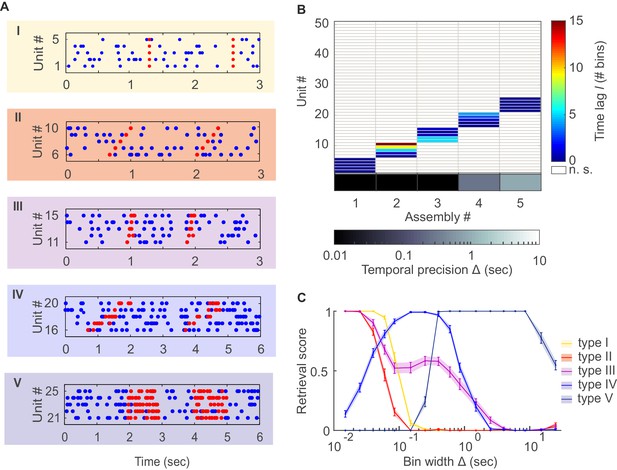
Detection of assemblies defined by different degrees of temporal precision, scale, and internal structure.
(A) Different assembly types in simulated non-stationary spike trains: I –highly precise lag-0 synchronization; II – precise sequential pattern; III – precise spike-time pattern without clear sequential structure; IV – rate pattern with temporal structure; V – simultaneous rate increase. (B) Assembly-assignment matrix, showing how the 50 simulated units were grouped into assemblies, at which lags to the leading unit they were so (color-coded), and at which bin widths Δ the corresponding assemblies were detected (sorted along abscissa). (C) Assembly retrieval score (fraction of correctly assigned units) as a function of bin width for the different assembly types, averaged across 70 independent runs. Error bars = SEM.
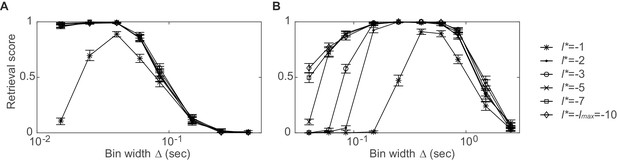
Dependence of synchronous pattern detection on reference lag.
Assembly retrieval score as a function of bin width for different choices of reference lag . Synchronous assembly patterns in the form of (A) sharp spike time coincidences (type I assembly, spike times jittered with ) and (B) common rate modulations (type V assembly, duration: ) in spike time series of length , averaged across 45 independent runs. Error bars = SEM. Throughout this manuscript, has been used for testing . Note that at the detection rates start to decline, while larger lags diminish the temporal sensitivity (for a given, non-confounded assembly, however, the most appropriate timescale may still be indicated through the p-levels associated with each ; cf. sect. ‘Recursive assembly agglomeration algorithm’).
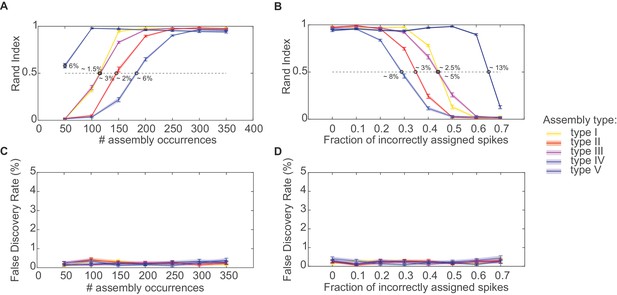
Performance evaluation of assembly detection algorithm.
(A) Rand index , Equation 14, as a function of the total number of occurrences of the assembly pattern in spike time series of length =1400 s, averaged across 50 independent runs, for all five types of assemblies from Figure 1 (as indicated in the inset legend). Percentages at the half-width points of the -curves indicate the proportion of spikes in the time series contributed by the assembly structures at these points. (B) Rand index for all assembly types as a function of the fraction of incorrectly assigned spikes (‘sorting errors’). (C) Fraction of units incorrectly assigned to an assembly across a range of assembly occurrence rates. (D) Fraction of units incorrectly assigned to an assembly as a function of the fraction of misattributed spikes. Error bars = SEM.
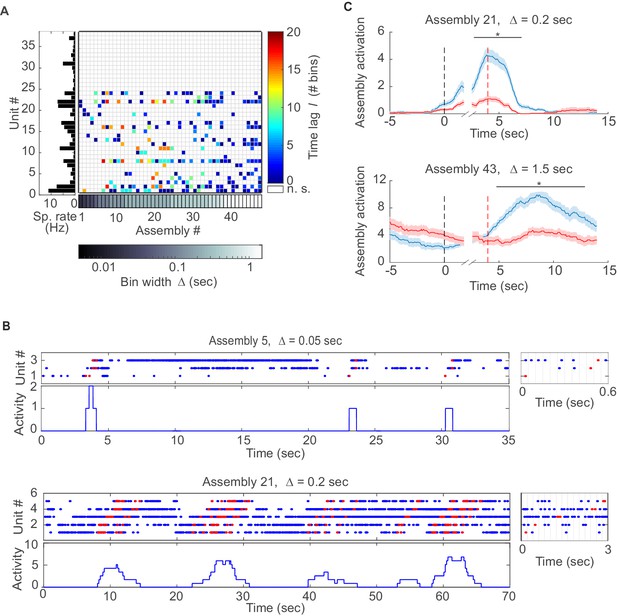
Assemblies in recordings from anterior cingulate cortex (ACC) during delayed alternation.
(A) Assembly-assignment matrix for one ACC data set, with the average firing rate of units indicated on the left. (B) Examples of detected assembly patterns at relatively precise (top; 50 ms) and broader (bottom; 200 ms) time scales. Insets on the right zoom in on detected assemblies with optimal binning Δ indicated by vertical lines. See Material and methods for computation of assembly activation scores (‘activity’) as shown in the lower panels. (C) Two examples of selective assembly activity discriminating between left (blue curves, n=39) and right (red curves, n=34) lever presses during actual lever press (top) or during delay (bottom). Times of lever press and nose poke are indicated by vertical red and black dashed lines, respectively. Periods of significant differentiation indicated by black bars above curves (two-tailed, paired t-test, *p<0.05, Bonferroni-corrected for number of bins tested, Figure 3—source data 1). Shaded areas = SEM.
-
Figure 3—source data 1
Assembly activation in different trials relative to left/right lever press for assemblies n. 21 and n. 43.
- https://doi.org/10.7554/eLife.19428.006
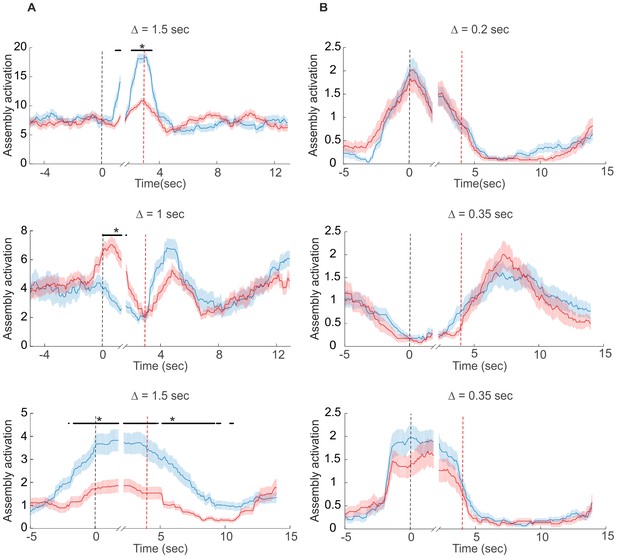
Examples of assembly activation in a delayed alternation task.
Times of lever press and nose poke are indicated by vertical red and black dashed lines, respectively. (A) Examples of assemblies with right (red) vs. left (blue) lever-selectivity around the time of the lever press itself (top; right: n=14, left: n=14), during both nose poke and reward consumption, but with a change in preference (center; right: n=35, left: n=28), and during the whole nose poke - lever press - delay phase (bottom panel; right: n=34, left: n=39). (B) Examples of non-selective assemblies with preferential activation during specific task epochs (right: n=34, left: n=39). Periods of significant differentiation indicated by black bars above curves (two-tailed, paired t-test, *p<0.05, Bonferroni-corrected for number of bins tested). Shaded areas = SEM.
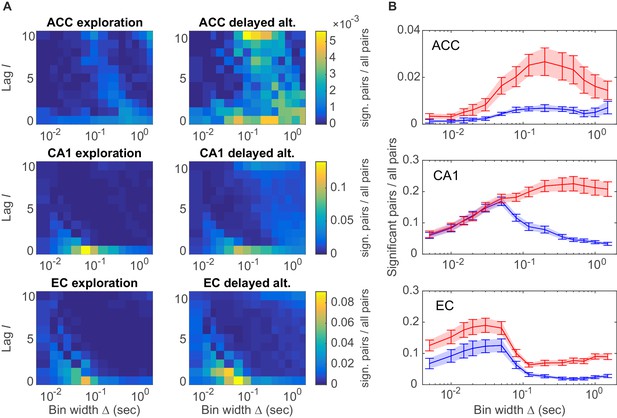
Assembly structure in different brain areas and behavioral tasks.
(A) Relative frequency histograms (color-coded) of all significant unit pairs, pooled across all detected assemblies, as a function of characteristic time scale s and lag , for the anterior cingulate cortex (ACC, top), CA1 region (center), and entorhinal cortex (EC, bottom) during environmental exploration (left; total numbers of pairs tested: 9316 [ACC], 7597 [CA1], 5024 [EC]) and delayed alternation (right; total numbers of pairs tested: 4090 [ACC], 9847 [CA1], 4183 [EC]). Note that the aggregation at larger lags for ACC is partly due to the fact that the algorithm considered lags up to , such that significant pairs with optimal lag have been assigned to . All tests are Bonferroni-corrected for numbers of pairs and lags tested. (B) Marginal distributions of significant assembly-unit pairs across temporal scales Δ for ACC (top), CA1 (center), and EC (bottom). Blue curves = environmental exploration. Red curves = delayed alternation. Shaded areas = SEM.
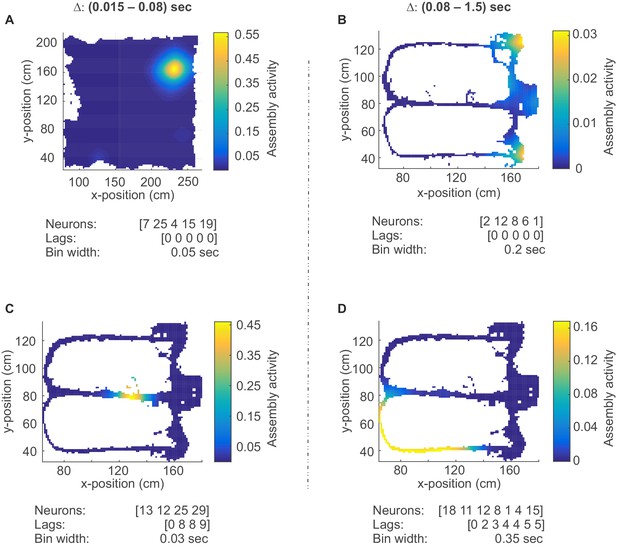
Assembly coding at different time scales in CA1.
Color-coded activity maps for four CA1 assemblies during an environmental exploration task (A) and a delayed alternation task (B, C, D). Below x-axis in each panel: Identities of neurons assigned to the assembly, associated time lags within an assembly, and temporal scale of assembly.
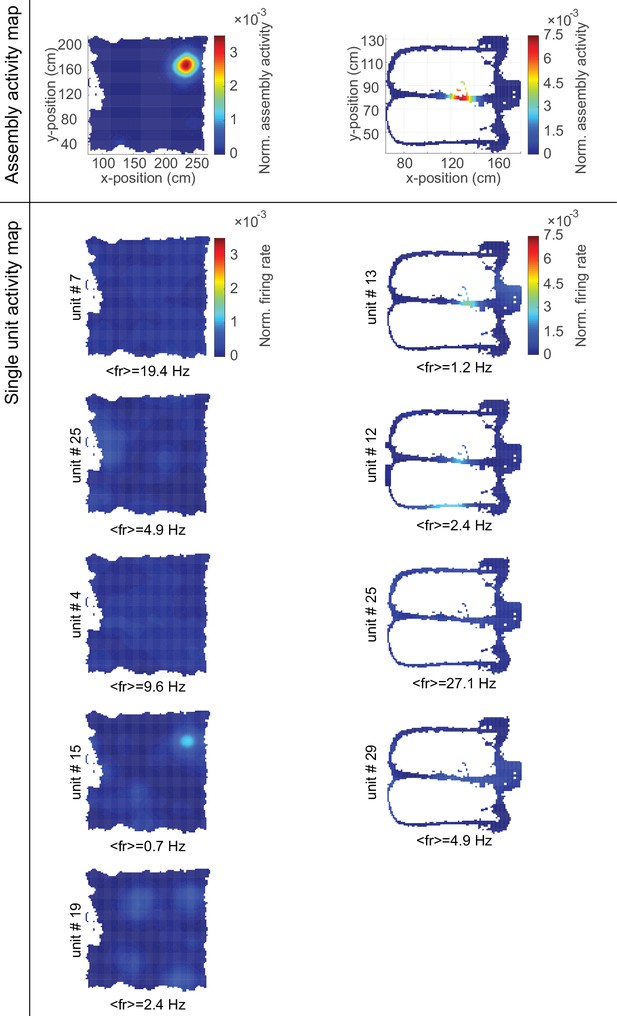
Single-unit composition of cell assemblies.
Color-coded normalized (to area under curve) activity maps for two assemblies (top row, Figure 5A and C) and their constituent single neurons (rows below) within an environmental exploration task (left) and a delayed alternation task (right). Average firing rates of the constituent single units indicated below each map.
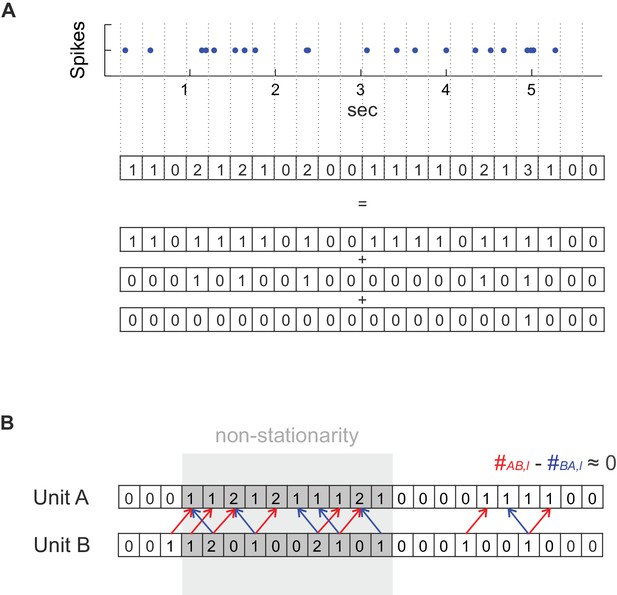
Method details.
(A) For deriving a statistical test that works with any temporal bin width the spike count series were separated into an overlay of several (dependent) binary sub-processes. See Materials and methods for further explanation. (B) Dealing with non-stationarity in the spike trains. In the case of non-stationarity in the form of a common rate increase in two units A and B (highlighted in gray), some spike co-occurrences caused by the rate increase might be incorrectly attributed to coupled activity (mutual dependence) at the finer timescale (bin width) at which coupling is investigated (at a lag of one in the illustrated example), even if there is not really any such coupling as assumed in this example. This corruption by non-stationarity may be removed by considering the difference count , in which spurious excess coincidences in one direction (: red arrows) would cancel out with those in the reverse direction (: blue arrows). It is important to note that if, on the other hand, the rate increase is on the timescale of interest, as it is the case for the ‘rate assemblies’ of type IV or V in Figure 1), subtracting off the reverse-lag count would not prevent assembly detection on that time scale.
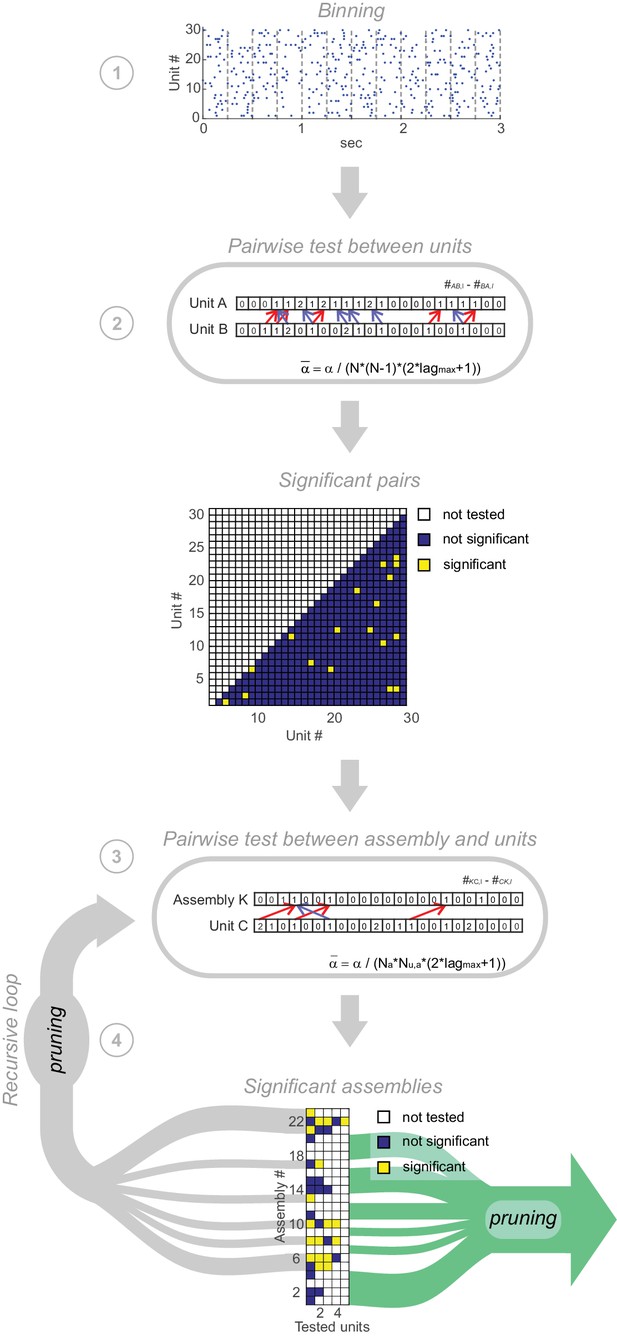
Pipeline of assembly agglomeration algorithm.
(1) Binning: Spike trains are binned at some time scale of interest. (2) Detection of pairwise interactions at chosen scale : For all unit pairs, test for significance, with . Significant unit pairs form elementary assemblies fed into the agglomerative recursion loop. (3) Assembly agglomeration: Test pairwise combinations of previously formed assemblies (with time stamps centered on first activated assembly unit) and single units for significance, and add latter to assembly set if significant. For this step, only units are considered which already are significantly related to at least one assembly member. (4) Recursive loop and pruning: All assemblies extended in the previous iteration are fed back into step 3. After each iteration, from all assemblies consisting of the same set of units but with different patterns of time lags only the one with the lowest p-value is retained. The loop is terminated if no units have been added to existing assemblies in the previous iteration. In a final pruning step all assemblies which are true subsets of larger assemblies are removed.
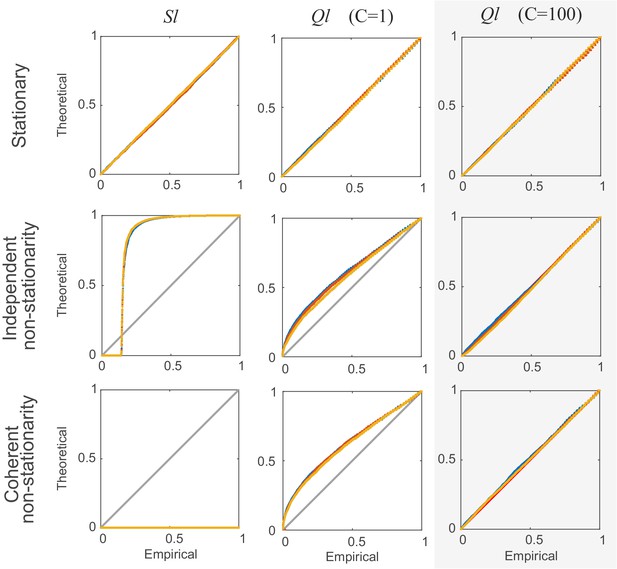
Comparison of non-corrected () and stationarity-corrected () pairwise test statistics.
Percentile-percentile plots showing agreement between the theoretical distributions for different test statistics considered in the text (, with =1, and with =100 segments) and the distributions empirically obtained, for the truly stationary case (top row), independent non-stationarity (center row), and non-stationarity coupled among the two units A and B (bottom row). Overlaid are distributions derived from 4000 simulation runs with spike time series analyzed for the three different lags = 0 (blue curves), 5 (yellow) and 10 (red). =100 in all cases. Simulations are with non-stationarity implemented as step-type rate-changes (see Materials and methods) with =1 and =75000. Identity line (bisectrix) is marked in gray. Results for test statistic used for all data analyses in this work highlighted by light-gray box.
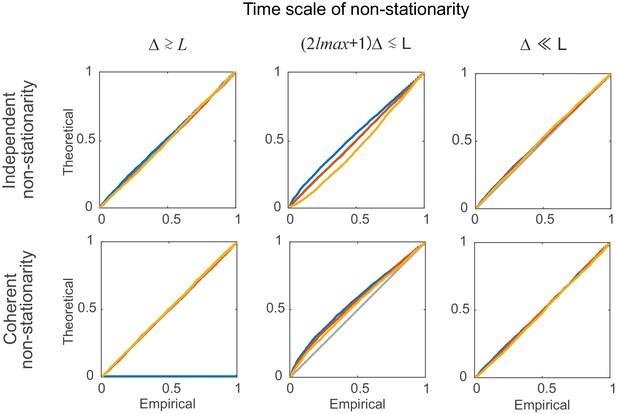
Statistical testing under non-stationarity on different time scales: step-like rate change.
Percentile-percentile plots showing agreement between the theoretical and empirical distributions (computed based on segments of length =100 bins, see Supplemental Experimental Procedures). Non-stationarity is implemented here via step-type rate changes with parameters as given in Materials and methods. Overlaid are distributions derived from 4000 simulation experiments with spike time series analyzed for the three different lags = 0 (blue), 5 (red), and 10 (yellow), and =3. Identity line (bisectrix) is marked in gray.
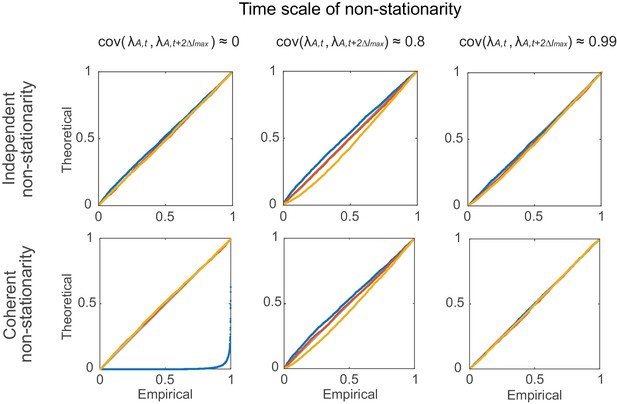
Statistical testing under non-stationarity on different time scales: rate covariation.
Agreement in theoretical vs. empirical (=100) distributions (P-P-plots as in Figure 7—figure supplement 1) under non-stationary conditions implemented through autoregressive processes (Equations 12–13). Denoting by the coupling matrix of the auto-regressive process, parameters were: =0 (top row) and =0.4, 0.997, 0.99999 (from left to right); =0.4, =0.5 (bottom-left), =0.995, =0.003 (bottom-center), =0.9996, =0.0004 (bottom-right). Overlaid are distributions derived from 4000 simulation experiments with spike time series analyzed for the three different lags = 0 (blue), 5 (red), and 10 (yellow), and =3. Identity line (bisectrix) is marked in gray.
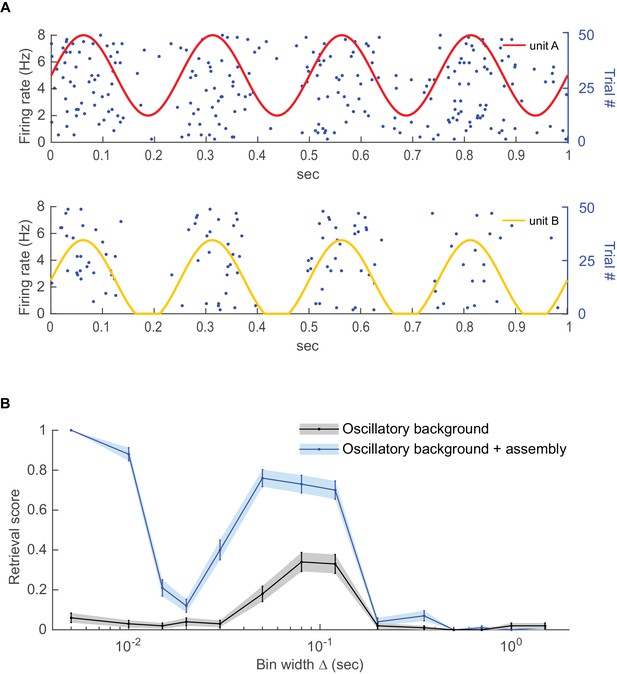
Detecting coupling among oscillating units.
Two Poisson units with different mean rates were subjected to a common oscillatory drive. (A) Illustration of the two units’ mean spike rates together with various spike trains drawn from this same process. (B) Detected fraction (from 0 to 1) of significant couplings among the two units as a function of bin width for the case where the units were just driven by the same oscillation but otherwise independent (gray curve) vs. the case where the units exhibited finer-time scale spike interactions on top (blue curve), averaged across n=100 independent runs. For the independent case, coupling is only detected at the time scale of the common oscillation, but not at finer scales. Error bars = SEM.
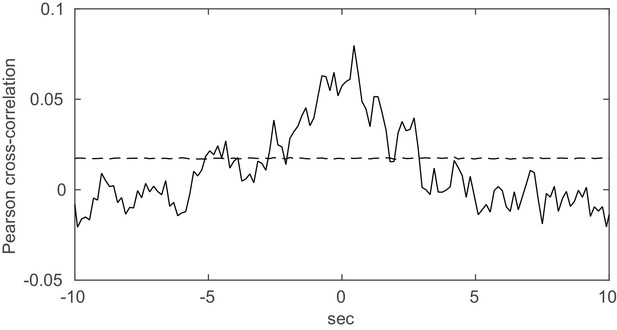
Spurious peaks in the cross-correlation function due to non-stationarity.
Two units with Poisson spike trains (=1900 s) and step-like rate variations of length =0.5 s (as, e.g., induced by a stimulus) were simulated. The onsets of the rate steps were drawn independently for the two units from normal distributions centered at randomly selected time points (with s). The Pearson cross-correlation was computed (binning Δ = 0.15 s) and tested for significance using inter-spike-interval shuffling (3000 repetitions). Dashed line indicates 2 standard deviations from mean. For this same simulation setup, our method correctly indicated the absence of true spike time dependencies when applied with the same bin width as used for the cross-correlogram.
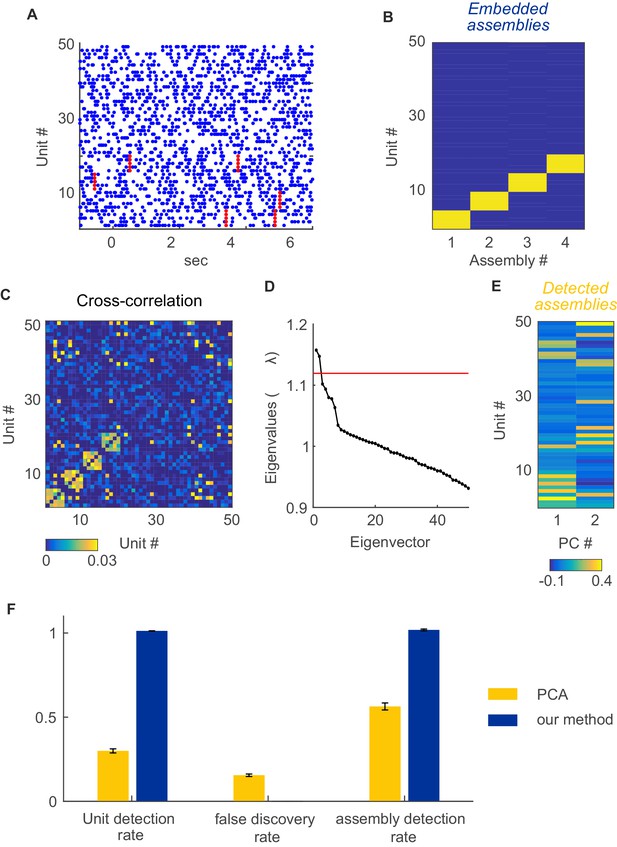
Assembly detection with PCA under non-stationarity.
For comparison with PCA-based assembly detection methods, simulations were performed with 50 non-stationary Poisson spike trains with four embedded, disjoint assemblies. Assemblies were defined as synchronous spike events (i.e., 'type I', cf. Figure 1A; 250 activations in total) occurring at random times within a set of five units. Non-stationary events were implemented as step-like changes shared among 4 groups of 5 units each, randomly chosen from the 50 units simulated, at random timings as described in sect. “Limitations of parametric testing under non-stationarity” (parameters used here were =0.02 s, =250, =1 s, =1950 s, baseline rate=5 Hz, up-state rate=10 Hz). For assembly detection by PCA, based on the cross-correlation matrix indicated in C, we followed the procedure described in Lopes-dos-Santos et al. (Lopes-dos-Santos et al., 2011) using code made publically available by the authors. (A) Examples of spike trains with assembly occurrences marked in red. (B) True unit-assembly assignment matrix. (C) Cross-correlation matrix with diagonal set to zero for better visualization. (D) Eigenvalue spectrum. Red line marks the upper limit of the Marčenko-Pastur distribution. (E) ‘Loadings’ of units on the two only significant principal components, indicating the assignment of units to the two assemblies detected this way. (F) Fraction of correctly detected assembly units (left), fraction of units falsely assigned to an assembly (center), and fraction of correctly detected assemblies (right) for PCA (yellow bars) and our method (blue bars). Error bars = SEM, based on 50 independent simulation runs.
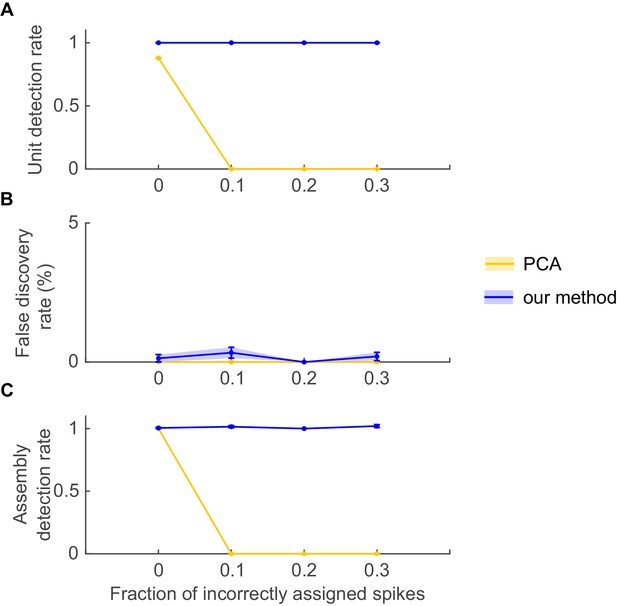
Stability of PCA solutions to degradation in assembly patterns.
Even under fully stationary conditions, PCA may completely fail to detect assembly patterns if degraded by spike assignment noise. Simulation setup was as in Appendix 1—figure 2, with the exception that no non-stationary step changes were included, i.e. spike trains were completely stationary. PCA methods were implemented as in Appendix 1—figure 2 (see ref. [Euston et al., 2007]). Noise in the form of spike misattributions or spike failures was introduced by randomly removing a fraction of assembly spikes from each spike train. (A) Fraction of correctly detected assembly units as a function of the proportion of spike assignment errors, (B) fraction of units incorrectly assigned to an assembly (false discovery rate), and (C) fraction of correctly detected assemblies (out of the total number of embedded assemblies), for PCA (yellow curves) and our method (blue curves). Error bars = SEM, based on 50 independent simulation runs.