Layer-specific chromatin accessibility landscapes reveal regulatory networks in adult mouse visual cortex
Figures
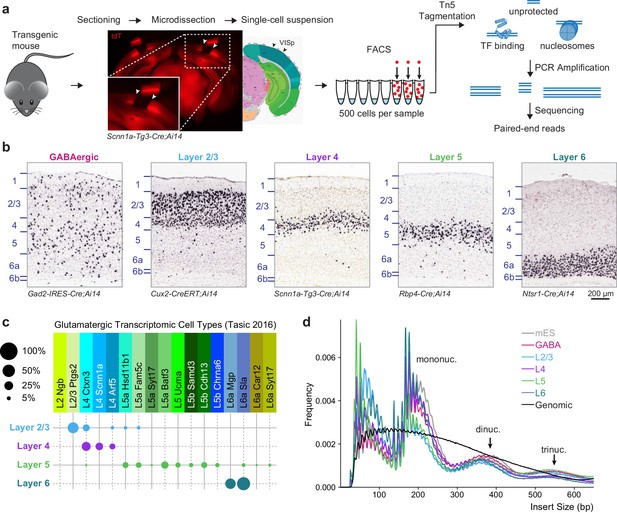
Overview of 500 cell ATAC-seq.
(a) Mouse visual cortex was isolated from transgenic mice by brain sectioning and microdissection, and dissociated into single-cell suspension. 500 fluorescently labeled cells were isolated from the suspension by FACS, tagmented by Tn5, indexed and amplified by PCR, and sequenced on an Illumina platform. (b) Chromogenic RNA in situ hybridization (ISH) for tdTomato mRNA in Cre lines used for this study. Scale bar below Layer 6 applies to all panels. (c) Cell-type specificity of the glutamatergic Cre lines based on scRNA-seq profiling. Each Cre line labels at least two related transcriptomic types, with minimal overlap between Cre lines. Disc sizes are scaled by area to represent the percent of cells from each Cre line that were identified as each transcriptomic cell type. (d) Insert size frequency of ATAC-seq fragments from primary neurons reveals protection of DNA by individual nucleosomes and nucleosome multimers that is absent from purified genomic DNA sample (black line).
-
Figure 1—source data 1
Cre-line cell type composition table, as plotted in Figure 1C.
- https://doi.org/10.7554/eLife.21883.003
-
Figure 1—source data 2
Fragment size frequencies for single replicates of each cell class.
- https://doi.org/10.7554/eLife.21883.004
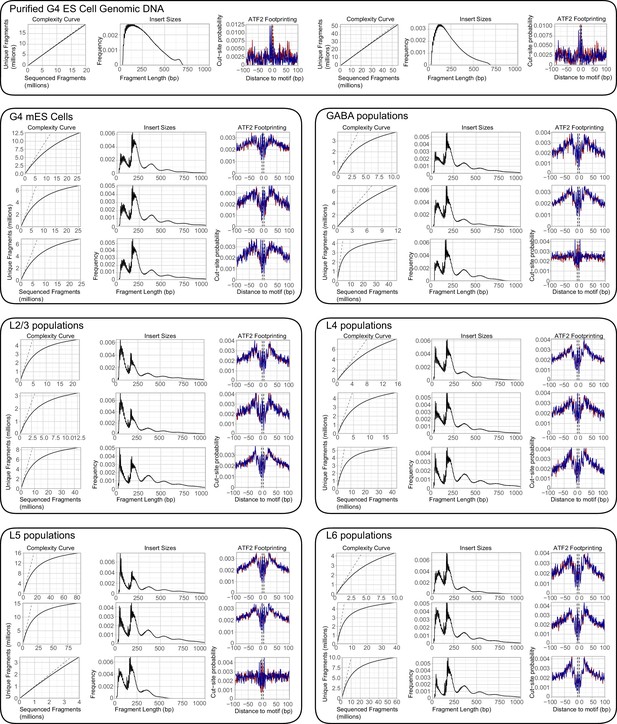
Quality control plots for ATAC-seq libraries.
Each library is composed of DNA from 500 cells. For each library, we plotted the complexity curve derived from preseq output, the insert sizes derived using Picard Tools, and ATF2 footprinting from CENTIPEDE (Materials and methods). We note that GABAergic replicate three and L5 replicate three display a weaker ATF2 footprint than the other ATAC-seq libraries. However, these footprints are qualitatively different from those derived from purified ES cell genomic DNA (note y-axes), and these samples cluster with other replicates from the same cell class (see Figure 3A). Thus, they were retained for downstream analyses.
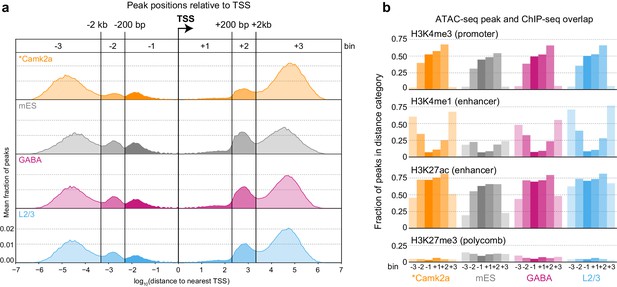
Peak locations relative to TSS and histone modifications.
(a) Histogram of peak positions relative to the nearest TSS location. Distance to nearest TSS was used to group peaks into three upstream categories (−3, –2, and −1) and three downstream categories (+1, +2, and +3). (b) Fractions of ATAC-seq peaks in each distance category that overlap ChIP-seq peaks derived from Camk2a-Cre neurons show similar patterns of enrichment in excitatory types (Camk2a and L2/3), but reduced enhancer overlaps and increased polycomb-repressed region overlaps in mES and GABAergic cells. *, Camk2a data were from a previous study (Mo et al., 2015).
-
Figure 2—source data 1
Distributions of peak locations relative to TSS, used for Figure 2A.
- https://doi.org/10.7554/eLife.21883.007
-
Figure 2—source data 2
Histone modification frequencies for peaks by cell class and distance bin, used for Figure 2B.
- https://doi.org/10.7554/eLife.21883.008
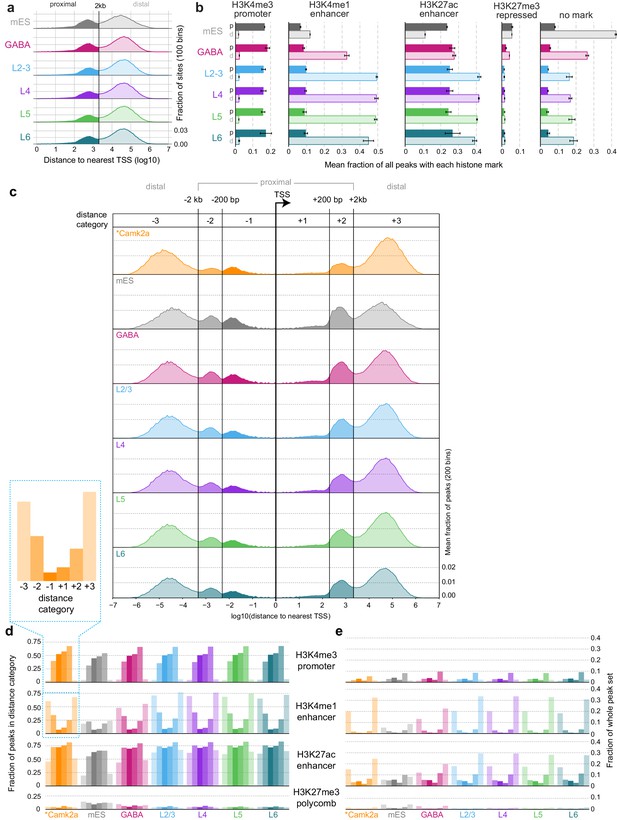
Comparisons of ATAC-seq peaks to TSS locations and Camk2a-Cre-derived histone ChIP-seq data.
(a) Absolute distances from peaks to the nearest TSS. Peaks separate into two clear populations when plotted on a log10 distance scale: p, proximal (<2 kb from a TSS); d, distal (>2 kb from TSS). (b) The fraction of peaks in each dataset that overlapped histone modification ChIP-seq from Camk2a-Cre cells (Mo et al., 2015). Bars represent mean values of 3 replicates; Error bars are standard deviations. (c) As for (a), but plotted 5’ and 3’ of relative to the TSS positions. In this case, peaks fall into six distinct groups, as described in the legend of Figure 2A. (d) Overlaps between peaks in each distance category for all cell classesand peaks of histone modifications defined by ChIP-seq on Camk2a-Cre-labeled glutamatergic cells, plotted as a fraction of peaks in each distance category. (e) As for (d), but plotted as a fraction of all HotSpot peaks identified for each cell class. Bars in (d) and (e) represent means of three replicates. *, Camk2a data is derived from a previous study (Mo et al., 2015).
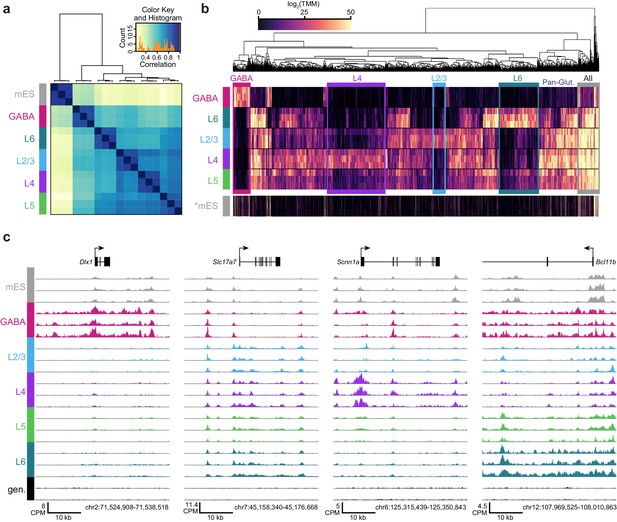
ATAC-seq samples cluster by cell class and reveal class-specific chromatin accessibility.
(a) Correlation between each sample pair was based on the number of overlapping HotSpot regions weighted by normalized accessibility scores for each sample. The pairwise correlation scores were then used for hierarchical clustering (DiffBind). (b) Hierarchical clustering by complete linkage of the 7500 most statistically significant differentially accessible peaks as defined by DiffBind. Boxes highlight peak clusters with high cell-class specificity. *, Peak values from mES cells were arranged based on clustering of neural cell classes, but were not used for clustering. (c) Genomic regions near four marker genes show differences in accessibility across different cell classes: Dlx1, distal-less homeobox 1, is expressed in GABAergic cells; Slc17a7, solute carrier family 17 (sodium-dependent inorganic phosphate cotransporter), member 7, is expressed in glutamatergic cells; Scnn1a, sodium channel, nonvoltage-gated 1 alpha, is primarily expressed in L4 glutamatergic cells; Bcl11b, B-cell leukemia/lymphoma 11B, is strongly expressed in L5 and L6 cells, as well as in a subset of GABAergic cells. CPM, counts of overlapping fragments per million; gen, purified genomic DNA control.
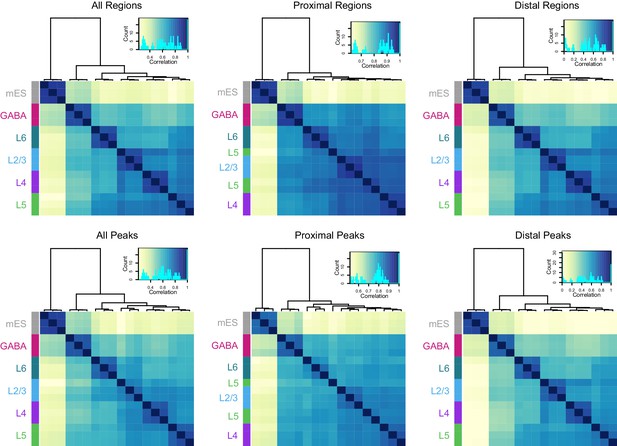
Hierarchical clustering of samples based on TSS-proximal or TSS-distal HotSpot results.
Top and bottom row, clustering of samples based on their HotSpot regions and peaks, respectively. HotSpot regions vary in length from 10 bp to 7.7 kb (median = 312 bp), while peaks are uniformly 150 bp. Distal region and peak sets (> 2kb away from TSS) appear to more stronly segregate cell classes compared to proximal sets (< 2kb away from TSS). The top-left, ‘All Regions’ plot corresponds to Figure 3A.
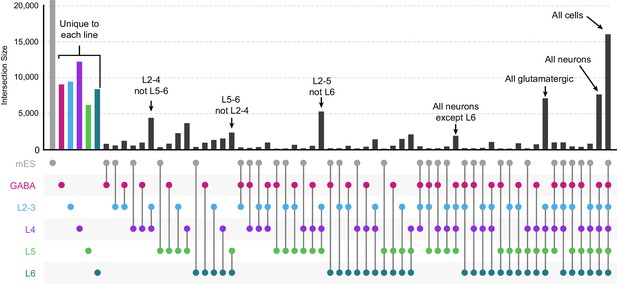
Intersections among peak sets derived from different cell classes.
We first derived a merged peak set by Diffbind for all peaks identified in all cell classes. We counted how often each merged peak overlapped peaks called for all 3 replicates of each cell class, then calculated the frequency with which each peak was found in every combination of cell classes. We plotted these frequencies using the UpSetR package for R. We find many genomic regions that are unique to each cell class, and others that are shared among various cell classes.
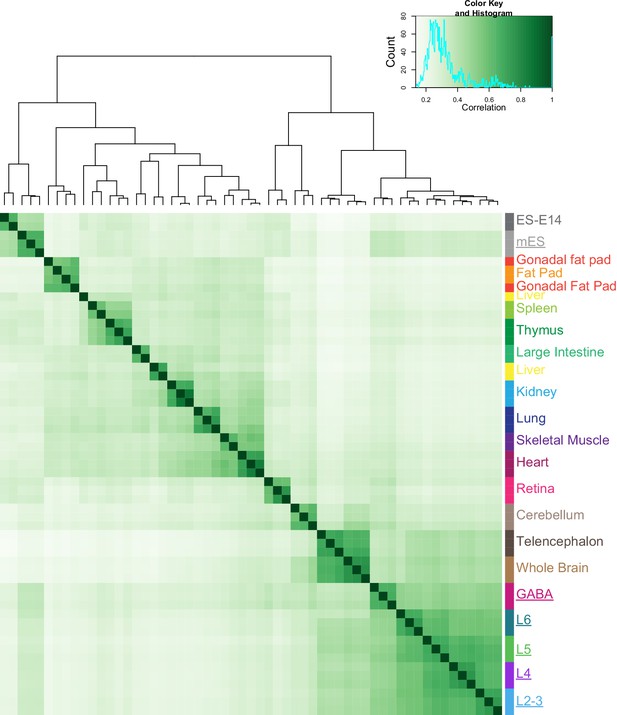
Correlation of HotSpot peak sets from this study and ENCODE tissue DNase-seq.
To test if our datasets agree with previously published brain-derived chromatin accessibility datasets, we obtained HotSpot peaks derived from DNase-seq of 15 tissues from the Mouse ENCODE database. We then performed peak overlap analysis using DiffBind to assess the similarity of our data (underlined names) to data from other mouse tissues. As expected, our neuron-derived datasets were found to be most similar to Whole Brain and Telencephalon tissue-derived DNase-seq data, while our mES-derived datasets clustered with ES-E14 cells.
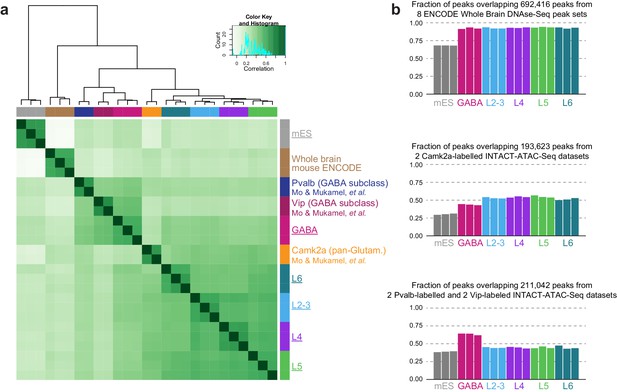
Correlation of brain-derived DNase-seq and ATAC-seq datasets.
(a) To test if our data showed similar patterns of chromatin accessibility as previously published mouse cortical ATAC-seq datasets, we performed DiffBind analysis of our datasets (underlined), ENCODE whole brain DNase-seq samples, and Pvalb, Vip, and Camk2a ATAC-seq samples from whole mouse cortex (Mo et al., 2015). For this purpose, Pvalb, Vip, and Camk2a data were downsampled to 3.2 M pairs of reads, and peaks were called using HotSpot with the same settings for 500 cell ATAC-seq samples. (b) We calculated the overlap between our ATAC-seq HotSpot peaks and merged sets of peaks from previously published chromatin accessibility datasets: 8 ENCODE Whole Brain DNase-seq datasets (top), 2 Camk2a-Cre ATAC-seq samples (middle), and 2 Pvalb-IRES-Cre and 2 Vip-IRES-Cre interneuron-derived ATAC-seq datasets (bottom).
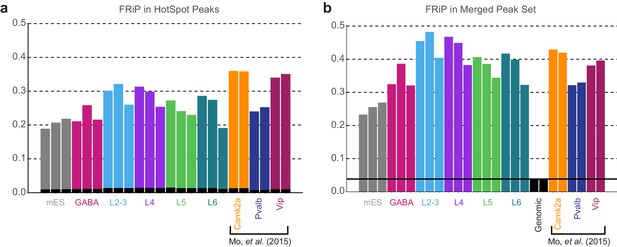
Fraction of Reads in Peaks.
(a) Fraction of reads in peaks (FRiP) scores were calculated for each sample by counting the number of downsampled BAM reads (3.2M per sample) that overlapped the corresponding HotSpot peaks in that sample. Black bars are the average FRiP scores from the two genomic control replicates. (b) FRiP scores for each downsampled BAM file calculated based on the set of merged peaks generated by DiffBind. The black line is the average FRiP for the two genomic samples. Camk2a, Pvalb, and Vip cell classes are from (Mo et al., 2015), and were not used to generate the merged peak set.
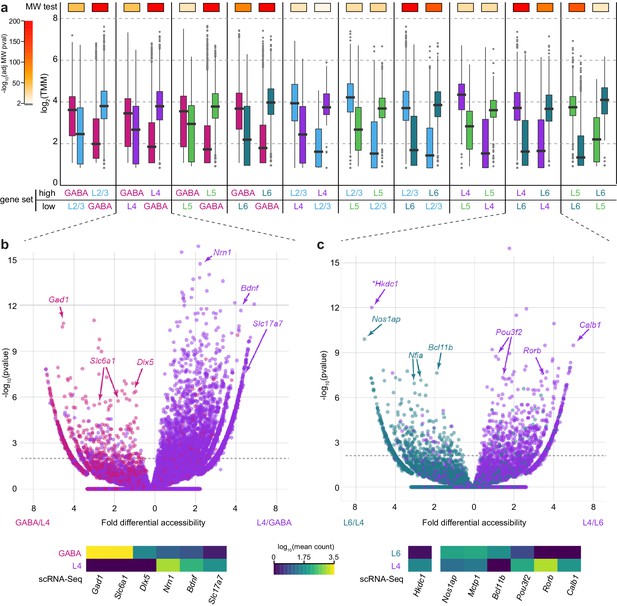
Chromatin accessibility corresponds to cell class-specific transcription.
(a) For each pair of cell classes, we identified differentially expressed genes (adjusted p-value < 0.05 and fold change > 2), then separated genes into two groups based on the class with higher expression. We then asked if the peaks that are positionally associated with each group of genes had higher accessibility in the class with higher gene expression. For each peak set, box plots show the median accessibility (black bar), quartiles (boxes), 1.5 × interquartile range (whiskers), and outliers (points). The two distributions of peak accessibility scores for each gene set were compared using a Mann-Whitney U test (MW test). Adjusted p-values are displayed using heatmap boxes above each gene set. (b, c) Two example volcano plots for pairwise comparisons between GABAergic and L4 cells (b) and L4 and L6 cells (c) showing all peaks associated with differentially-expressed genes (adjusted p-value < 1×10–6) . Peaks associated with select marker genes are labeled, and the corresponding average gene expression for these genes from single-cell RNA-seq data for each cell class is shown below. *, Hkdc1-associated peak is more accessible in L6 cell class, although expression of Hkdc1 is greater in L4 class.
-
Figure 4—source data 1
Peak accessibility scores (TMM) for peaks associated with gene sets in Figure 4A.
- https://doi.org/10.7554/eLife.21883.017
-
Figure 4—source data 2
Mann-Whitney test results for each comparison in Figure 4A.
- https://doi.org/10.7554/eLife.21883.018
-
Figure 4—source data 3
Gene expression data for the heatmap at the bottom of Figure 4B.
- https://doi.org/10.7554/eLife.21883.019
-
Figure 4—source data 4
Differential accessibility and –log10(pvalue) scores used to generate the volcano plot in Figure 4B.
- https://doi.org/10.7554/eLife.21883.020
-
Figure 4—source data 5
Gene expression data for the heatmap at the bottom of Figure 4C.
- https://doi.org/10.7554/eLife.21883.021
-
Figure 4—source data 6
Differential accessibility and –log10(pvalue) scores used to generate the volcano plot in Figure 4C.
- https://doi.org/10.7554/eLife.21883.022
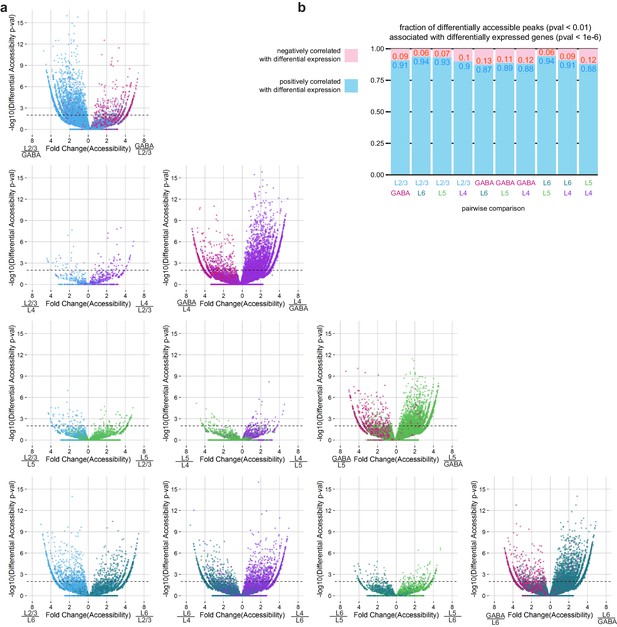
Pairwise comparisons of peak accessibility for peaks associated with differentially-expressed genes.
(a) To assess the overall correspondence between differentially expressed genes and differentially accessible chromatin, we plotted the statistical significance for each peak that was positionally associated with a differentially expressed gene (adjusted p-value < 1×10–6) as a function of the fold change in accessibility for that peak for each pair of neural cell classes. p-value <(b) We calculated the fraction of differentially accessible peaks positionally associated with differentially expressed genes that were positively or negatively correlated with gene expression. For this analysis, we used the mean fold change of 3 replicates.
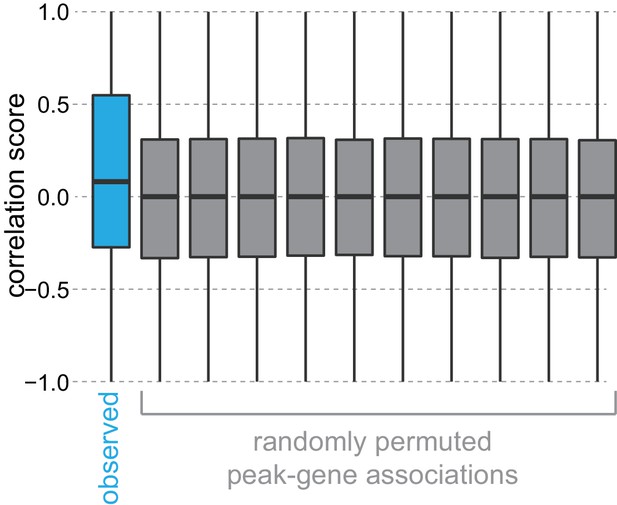
Permutation of peak-gene associations.
To test the correlation between gene expression and nearby peak accessibility, we calculated mean peak accessibility scores for each peak and mean gene expression scores for each gene across neuronal cell classes, then scaled these scores per peak and per gene between 0 and 1. These scaled values were used to compute Pearson correlation scores between each peak and the nearest (positionally associated) gene. The distribution of observed correlations between peak accessibility and gene expression of positionally associated genes are shown in blue, compared to randomly permuted associations between peaks and genes, shown in gray. Boxplots show medians (middle bar), quartiles (boxes), and 1.5 × interquartile ranges (whiskers). A Kolmogorov–Smirnov test between observed correlations and a randomly-permuted correlation set had a p-value of 0, while a comparison between two randomly-permuted correlation sets had a p-value of 0.334.
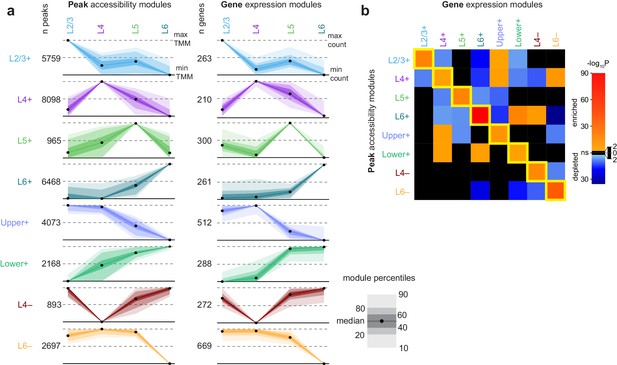
Clustering of peaks and genes reveals common patterns of chromatin accessibility and gene expression.
(a) Scaled module profiles derived from k-means clustering of peaks and genes (Materials and methods). Points represent median values, and shaded areas represent percentiles as shown in the legend. (b) We calculated how frequently peaks in each peak module were positionally-associated with genes in each gene module, then computed enrichment or depletion using Fisher's exact tests for enrichment. The heatmap represents the log-transformed, adjusted p-values from Fisher’s exact tests, with enrichment (odds ratio > 1) in red and depletion (odds ratio < 1) in blue. Black indicates non-significant enrichment or depletion (adjusted p-value > 0.01).
-
Figure 5—source data 1
Fisher’s exact test result values presented in Figure 5B.
- https://doi.org/10.7554/eLife.21883.026
-
Figure 5—source data 2
Quantile values for gene clusters presented in Figure 5A.
- https://doi.org/10.7554/eLife.21883.027
-
Figure 5—source data 3
Quantile values for peak clusters presented in Figure 5A.
- https://doi.org/10.7554/eLife.21883.028
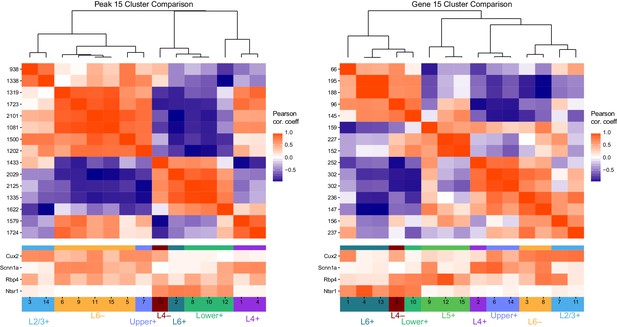
Example instance of initial k-means clustering of differentially accessible peaks and differentially expressed genes.
We first performed k-means clustering on peaks (left) and genes (right) with k = 15. This resulted in overclustering, as many clusters are extremely similar to each other. Top heatmaps show Pearson correlation coefficients for comparisons between cluster centers. Bottom heatmaps show the scaled values of the cluster centers (between 0 and 1) for each glutamatergic class, with colored tags corresponding to the final cluster patterns we selected. We note that, at this level of clustering, we did not identify a clear Layer 5+ cluster among peaks, though it was found among genes.
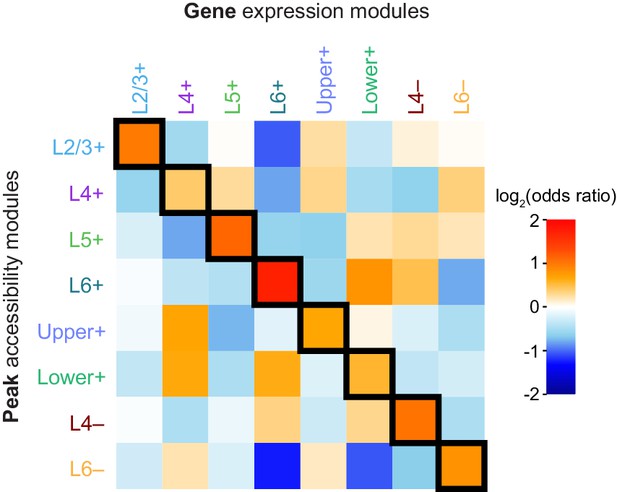
Log-odds ratios for tests of Peak-Gene module association.
Log-odds ratios for Fisher's exact tests of enrichment between each pair of peak and gene modules, corresponding to the p-values reported in (Figure 5).
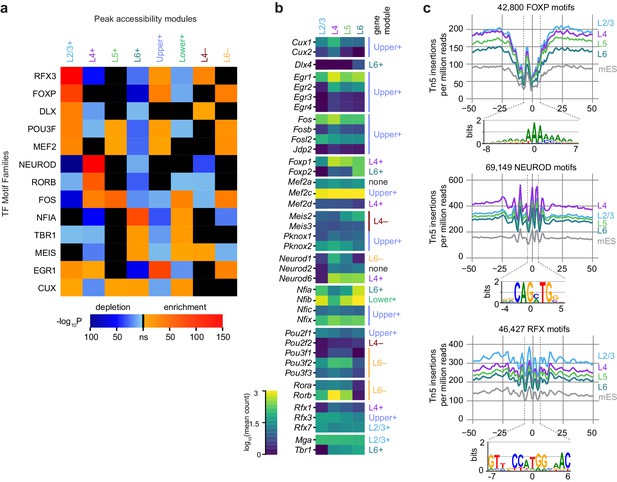
Peak module analysis reveals layer-specific enrichment of transcription factor motifs.
(a) Select TF motif families are significantly enriched or depleted in specific peak modules. Enrichment and depletion were calculated relative to unrelated modules (Figure 6—figure supplement 1) using AME. (b) Expression of genes belonging to TF families identified by Treefam and other criteria (Materials and methods). Heatmap shows mean gene expression counts within each cell class based on single-cell RNA-seq data (Materials and methods). For each gene, the most strongly correlated gene module is listed to the right of the heatmap. (c) Tn5 footprinting for select motif families in each glutamatergic cell class and mES cells. The cut site frequency was calculated at each base position relative to the center of each corresponding motif . Frequencies are shown as the number of Tn5 insertions (locations of 5’ ends of fragments) per million reads.
-
Figure 6—source data 1
AME result p-values, as plotted in Figure 6A.
- https://doi.org/10.7554/eLife.21883.032
-
Figure 6—source data 2
Gene expression values used for Figure 6B.
- https://doi.org/10.7554/eLife.21883.033
-
Figure 6—source data 3
FOXP motif Tn5 insertion frequency data.
- https://doi.org/10.7554/eLife.21883.034
-
Figure 6—source data 4
NEUROD motif Tn5 insertion frequency data.
- https://doi.org/10.7554/eLife.21883.035
-
Figure 6—source data 5
RFX motif Tn5 insertion frequency data.
- https://doi.org/10.7554/eLife.21883.036
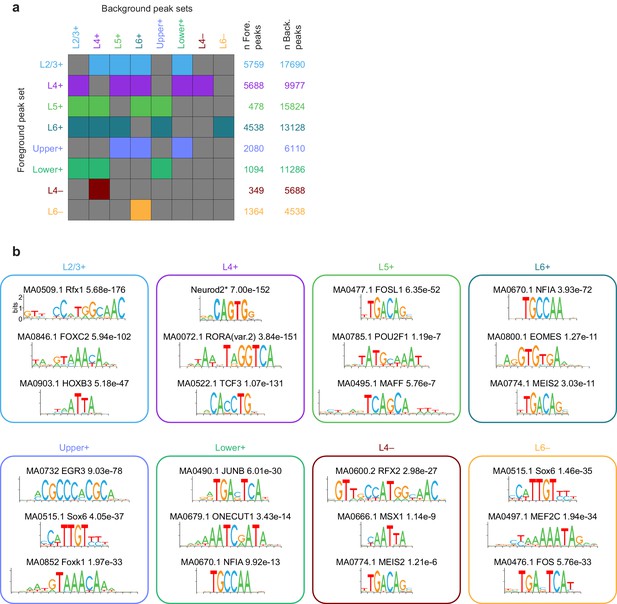
Background set selection for AME and top significant AME results for each peak module.
(a) For each peak module (rows), the background modules are represented as colored boxes. These sets of foreground and background peaks were used for AME analysis. (b) Representative motif LOGOS for the top three enriched motifs from AME analysis of each gene module. To select the top 3, we excluded extremely similar motifs found in the AME results. A more complete set of significant results is available in Supplementary file 2A. *, the Neurod2 motif was not included in the JASPAR database, but was added based on (Fong et al., 2015).
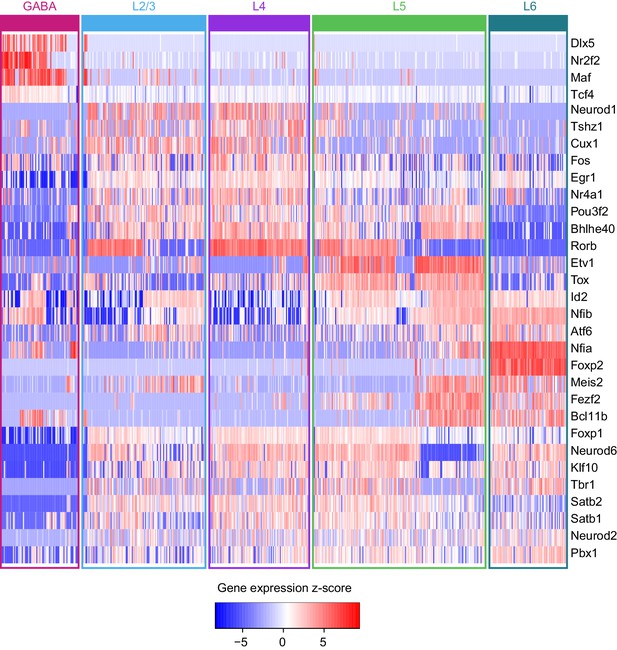
Transcription factor gene expression in individual cells arranged by cell class.
https://doi.org/10.7554/eLife.21883.038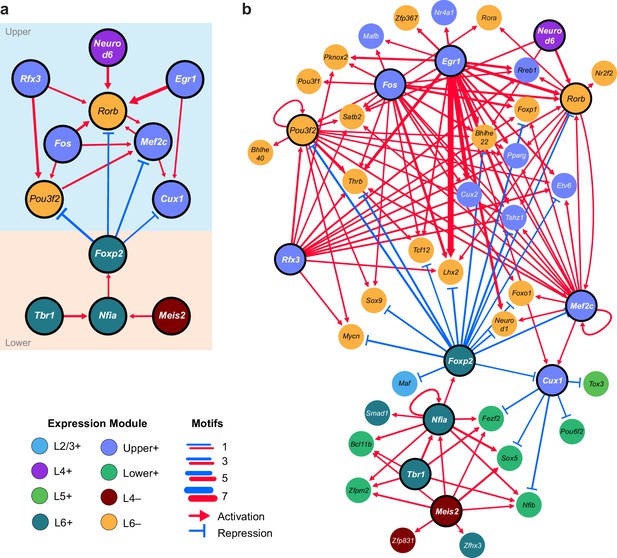
Putative regulatory interactions that govern layer-specific chromatin and transcriptomic state in glutamatergic cell classes.
(a) Putative regulatory interactions between key TFs. (b) Putative regulatory interactions between key TFs and other differentially expressed TFs in glutamatergic cell classes. Key TFs have bold outlines, whereas targets have no outline. Each TF node is colored according to the most strongly correlated gene module. Edges of the network represent differentially-accessible motifs, and are weighted based on the number of motifs observed near the target gene.
-
Figure 7—source data 1
Data used to build the network presented in Figure 7B and Figure 8.
- https://doi.org/10.7554/eLife.21883.040
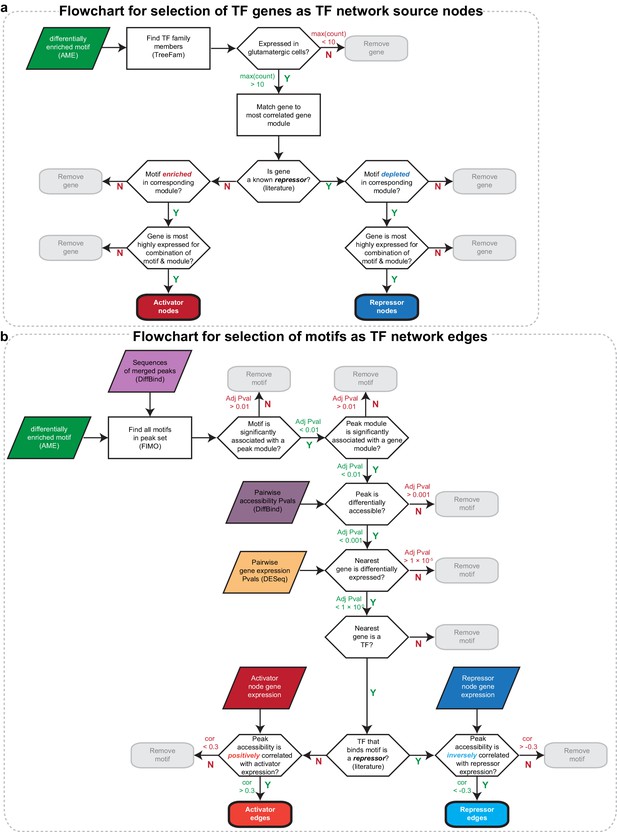
Flowcharts for selecting TFs and TF targets to construct a network of regulatory interactions.
(a) Flowchart for selecting key TFs that act as activators or repressors by binding differentially enriched TF motifs. These genes were used as nodes in our network diagram. (b) Flowchart for selecting differentially accessible motifs present in our peak sets that may be responsible for layer-specific transcriptional regulation. These motifs were used as edges in our network diagram.
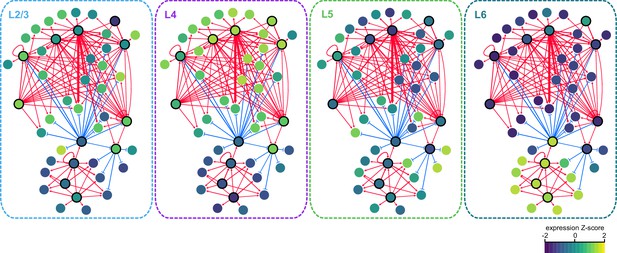
Gene expression patterns of layer-specific transcription factors.
The location and identity of each node is the same as in the regulatory network presented in Figure 7. The color of each node represents the normalized, average gene expression across all cells in each cell class.
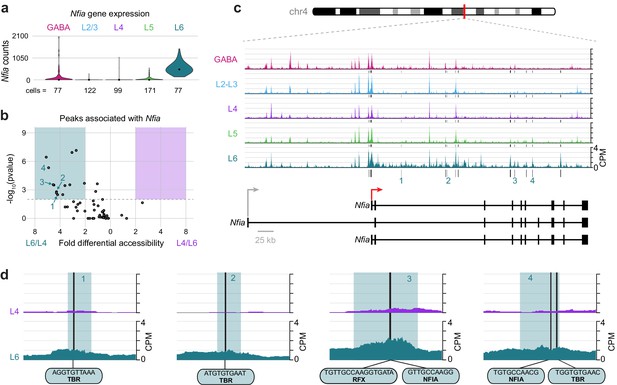
The Layer-specific regulatory landscape of Nfia.
(a) Nfia gene expression distributions based on scRNA-seq in each neuronal cell class. (b) Volcano plot showing all peaks that are positionally associated with Nfia in L6 and L4. Significantly differentially accessible peaks (adjusted p-values < 0.01 and > 2-fold change in accessibility score) are highlighted as L6-specific (green box) or L4-specific (purple box). (c) Chromatin accessibility near the Nfia gene in each cell class (547 kb window; mm10 chr4:97,576,942–98,123,876). Vertical lines below the tracks represent the locations of peaks that are significantly more accessible in L6 compared to L4. (d) 1 kb windows centered on four numbered peaks that contain putative TF binding sites. TF motif locations within each peak are marked by black bars. CPM, counts of overlapping sequenced fragments at each position per million mapped reads for each cell class. All fragment overlap panels are plotted on the same scale (0 to 4 CPM).
-
Figure 9—source data 1
Nfia expression values used to generate the plot in Figure 9A.
- https://doi.org/10.7554/eLife.21883.044
-
Figure 9—source data 2
Peak statistics for peaks positionally associated with Nfia, used to generate Figure 9B.
- https://doi.org/10.7554/eLife.21883.045
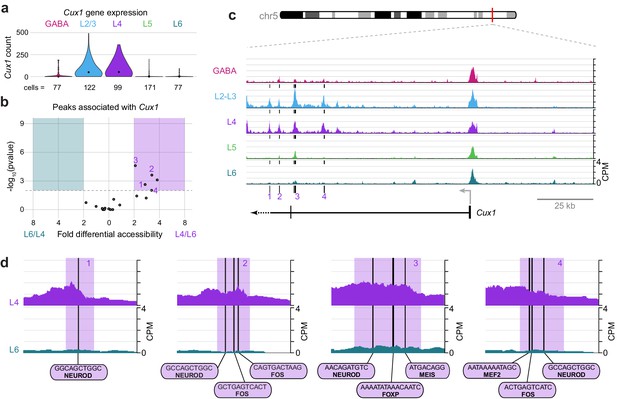
Cell class-specific regulatory domains downstream of the Cux1 TSS.
(a) Cux1 gene expression distributions based on scRNA-seq in each neuronal cell class. (b) Volcano plot shoing all peaks that are positionally associated with Cux1 in a pairwise comparison between L6 and L4 cell classes. Significantly differentially accessible peaks (adjusted p-values < 0.01 and > 2 -fold change in accessibility score) are highlighted as L6-specific (green box) or L4-specific (purple box). (c) Chromatin accessibility near the Cux1 gene in each cell class (155 kb window; mm10 chr5:136,465,981–136,620,981). Vertical lines below the tracks represent the locations of peaks that are significantly more accessible in L4 compared to L6. (d) 1 kb windows centered on four numbered peaks that contain putative TF binding sites. TF motif locations are marked by black bars. CPM, counts of overlapping sequenced fragments at each position per million mapped reads for each cell class. All fragment overlap panels are plotted on the same scale (0 to 4 CPM).
-
Figure 10—source data 1
Cux1 expression values used to generate the plot in Figure 10A.
- https://doi.org/10.7554/eLife.21883.047
-
Figure 10—source data 2
Peak statistics for peaks positionally associated with Cux1, used to generate Figure 10B.
- https://doi.org/10.7554/eLife.21883.048
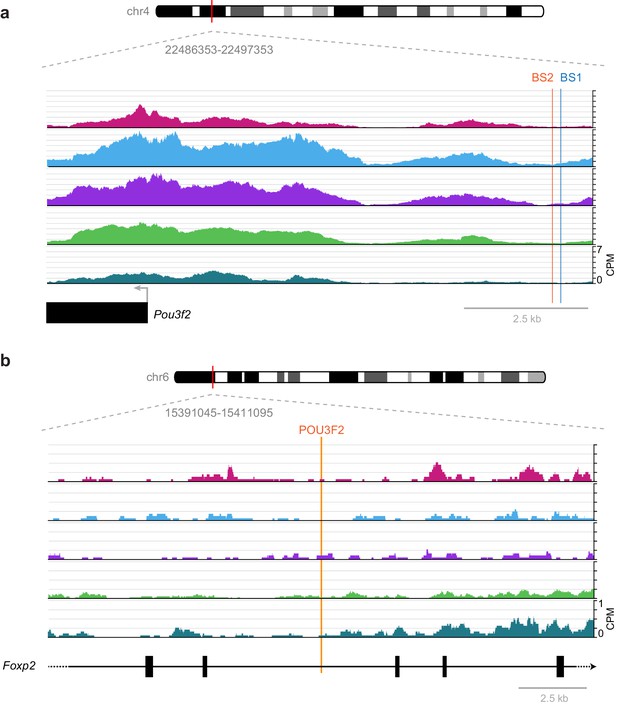
Previously published TF-binding sites near Pou3f2 and Foxp2 observed during development are not accessible in adult mouse cortex.
(a) Previously described RORB-binding sites (BS1 and BS2) near the Pou3f2 TSS are not accessible in any of the adult cell classes we examined. (b) Same as in (a), but for a POU3F2 binding site in the Foxp2 gene. CPM, counts of overlapping sequenced fragments at each position per million mapped reads for each cell class.
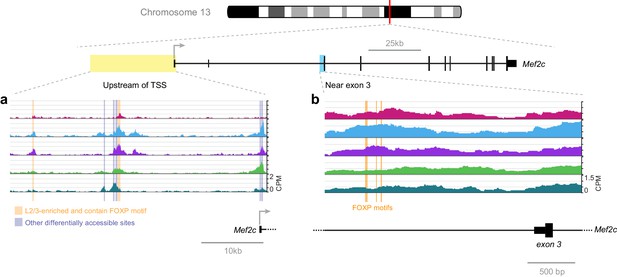
FOXP motif accessibility within or near the Mef2c gene.
(a) Chromatin accessibility upstream of the Mef2c TSS. Orange boxes, putative FOXP binding sites that are significantly less accessible in L6 than in upper layers; Gray boxes, differentially-accessible sites that do not contain FOXP motifs. (b) FOXP motifs identified as direct targets of FOXP2 in a previous study (Chen et al., 2016). CPM, counts of overlapping sequenced fragments at each position per million mapped reads for each cell class.
Additional files
-
Supplementary file 1
Libraries, Peaks, and associated statistics.
(a) Library and alignment statistics. (b) Merged DiffBind peak locations, mean TMM scores per cell class, and pairwise DiffBind p-values. (c) Associations between peaks and nearest genes, average expression scores for associated genes, and pairwise DESeq2 differential expression p-values.
- https://doi.org/10.7554/eLife.21883.051
-
Supplementary file 2
Motif analysis results.
(a) Peak module AME results. (b) FIMO motif search results. (c) Network interaction table.
- https://doi.org/10.7554/eLife.21883.052
-
Supplementary file 3
Downsampled RNA-seq data.
- https://doi.org/10.7554/eLife.21883.053