A unified internal model theory to resolve the paradox of active versus passive self-motion sensation
Figures
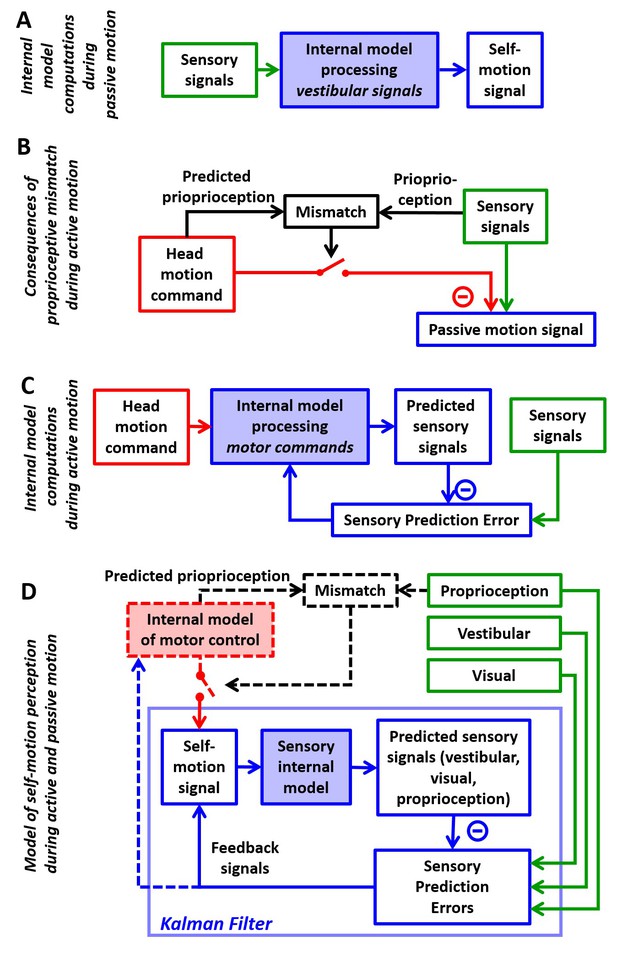
Internal model computations for self-motion estimation.
(A) Previous studies based on passive stimuli have proposed that vestibular sensory signals are processed by an internal model to compute optimal estimates of self-motion. (B) Other studies (Roy and Cullen, 2004) have shown that the cancellation of central vestibular responses during active motion is gated by mismatches between predicted and actual neck proprioceptive signals, and interpreted central vestibular responses as a passive motion signal. (C) Brooks and Cullen (Brooks et al., 2015; Brooks and Cullen, 2013) have proposed that an internal model processes motor commands to compute sensory predictions during active motion and that central vestibular neurons encode sensory prediction errors. (D) Framework proposed in this study, in which the internal models in (A) and (C) are in fact identical and interactions between head motion commands and sensory signals are modeled as a Kalman filter (blue) that computes optimal self-motion estimates during both passive and active motions. For simplicity, we have not included how head motion commands are generated (red), how head movements are executed, as well as the contribution of feedback to error correction and motor learning (dashed blue arrow). In line with B, the proprioceptive gating mechanism in D is shown as a switch controlling the transmission of head motion commands to the Kalman filter. Solid lines in (D): computations modeled as a Kalman filter. Broken lines in (D): additional computations that are only discussed (but not modeled) in the present study. Note that the ‘self-motion signal’ box in D stands for both the predicted motion and final self-motion estimate (Figure 1—figure supplement 2).
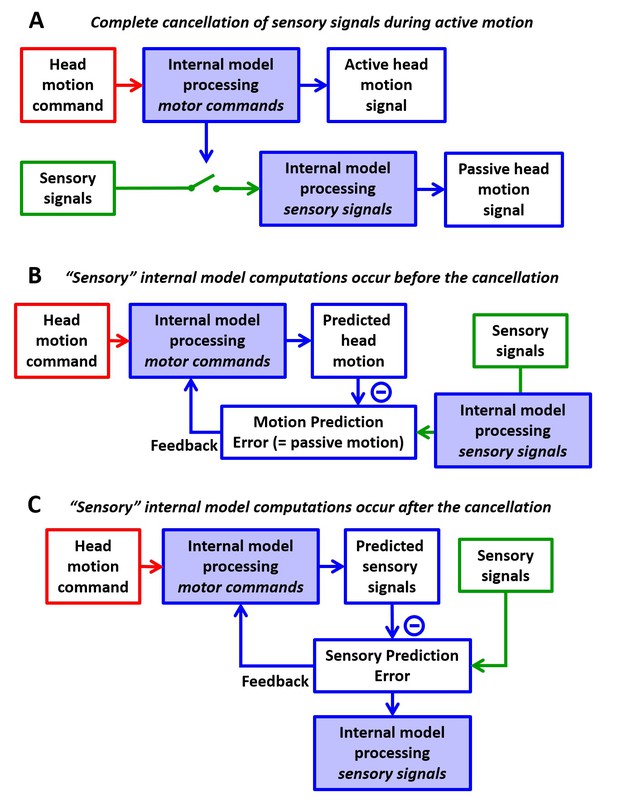
Alternative computational schemes where separate internal models process vestibular signals and motor commands.
(A) Sensory signals may be switched off during active motion. This model will be refuted by simulations in Figure 8. (B,C) Alternatively, the output of the ‘internal model processing motor commands’ (whose nature and properties have never been defined in all previous publications by Cullen and colleagues) may be subtracted from the output (B) or the input (C) of the sensory internal model. In (B), sensory signals would be transformed into head motion signals before being cancelled by a predicted self-motion signal, which implies that central vestibular neurons would encode a motion prediction error (equal to the passive component of head motion), instead of a sensory prediction error. We refute this possibility in Figure 9. In (C), the processing is similar to the Kalman filter. This is because, as demonstrated in this study, the transformation of motor commands into predicted sensory signals requires the same sensory internal model that processes vestibular signals during passive motion, and we collapse these computations into a single internal model in Figure 1D. Note that the Kalman filter could be transformed into the model in panel (B) by (unnecessarily) duplicating this single internal model. We discuss these alternative possibilities in Results: ‘Alternative models of interaction between active and passive motions’.
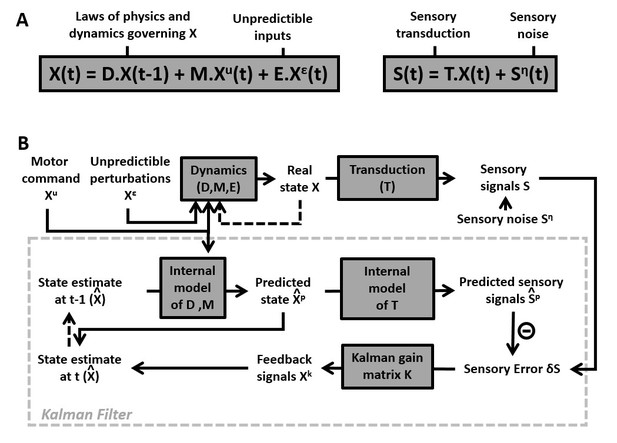
Generic structure of a Kalman Filter.
(A) Equations of the Kalman filter algorithm describing how motor commands and sensory signals are processed for optimal state estimation. Motion variables () are computed as a function of motor commands and unpredictable perturbations . Matrices ,, and encode the system’s dynamics. Sensory inputs are computed as a function of and sensory noise, . (B) Schematic implementing the equations of the Kalman filter algorithm. An internal estimate of motion variables (internal states, ) is computed dynamically as a function of , motor commands and sensory signals . The dashed arrows indicate that the estimate at time is passed to the next time step, where it becomes the estimate at . Sensory errors are transformed into feedback , where is a matrix of feedback gains, whose rank is determined by the dimensionality of both the state variable and the sensory signals . The box defined by dashed gray lines illustrates the Kalman filter computations.
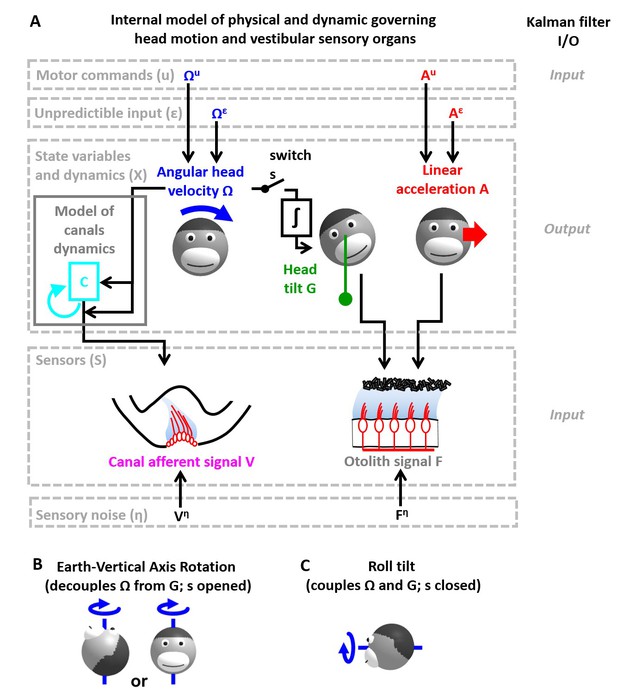
Application of the Kalman filter algorithm into optimal self-motion estimation using an internal model with four state variables and two vestibular sensors.
(A) Schematic diagram of the model. Inputs to the model include motor commands, unexpected perturbations, as well as sensory signals. Motor commands during active movements, that is angular velocity () and translational acceleration (), are known by the brain. Unpredicted internal or external factors such as external (passive) motion are modeled as variables and . The state variable has 4 degrees of freedom: angular velocity , tilt position linear acceleration and a hidden variable used to model the dynamics of the semicircular canals (see Materials and methods). Two sensory signals are considered: semicircular canals (rotation sensors that generate a signal ) and the otoliths organs (linear acceleration sensors that generate a signal ). Sensory noise and is illustrated here but omitted from all simulations for simplicity. (B, C) illustration of rotations around earth-vertical (B) and earth-horizontal (C) axes.
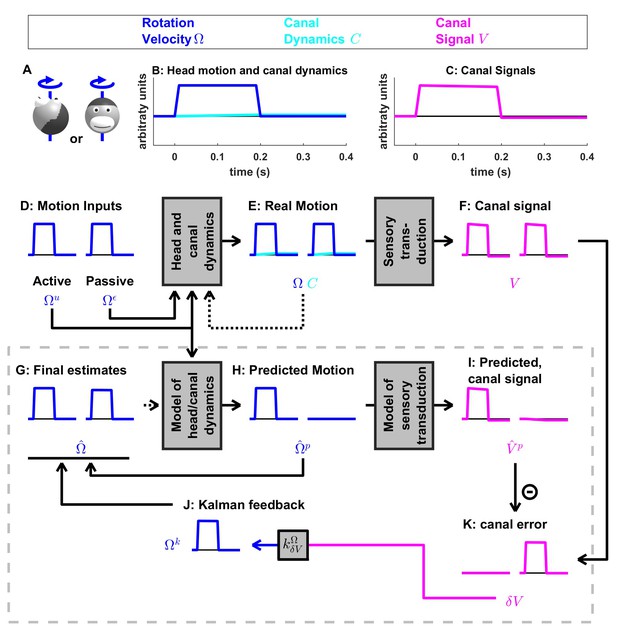
Short duration rotation around an earth-vertical axis (as in Figure 2B).
(A) Illustration of the stimulus lasting, 200 ms. (B,C) Time course of motion variables and sensory (canal) signals. (D–K) Simulated variables during active (left panels) and passive motion (right panels). Only the angular velocity state variable is shown (tilt position and linear acceleration are not considered in this simulation, and the hidden variable is equal to zero). Continuous arrows represent the flow of information during one time step, and broken arrows the transfer of information from one time step to the next. (J) Kalman feedback. For clarity, the Kalman feedback is shown during passive motion only (it is always zero during active movements in the absence of any perturbation and noise). The box defined by dashed gray lines illustrates the Kalman filter computations. For the rest of mathematical notations, see Table 1.
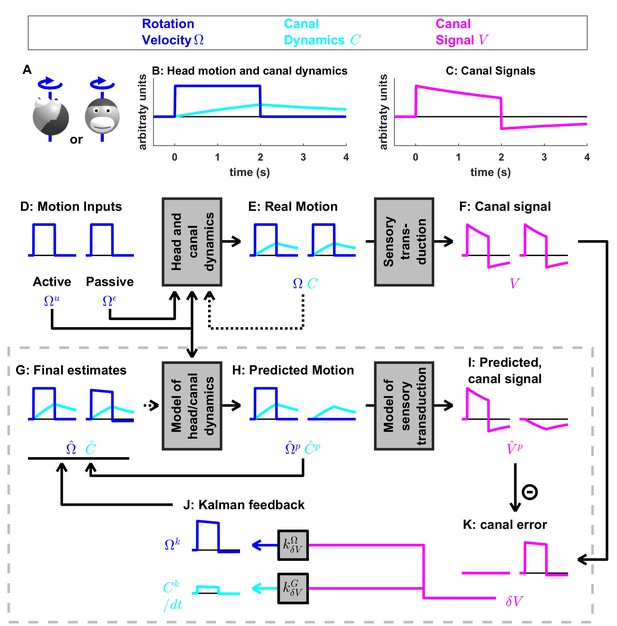
Medium-duration rotation around an earth-vertical axis, demonstrating the role of the internal model of canal dynamics.
(A) Illustration of the stimulus lasting 2 s. (B,C) Time course of motion variables and sensory (canal) signals. (D–K) Simulated variables during active (left panels) and passive motions (right panels). Two state variables are shown: the angular velocity (blue) and canal dynamics (cyan). Continuous arrows represent the flow of information during one time step, and broken arrows the transfer of information from one time step to the next. (J) Kalman feedback. For clarity, the Kalman feedback (reflecting feedback from the canal error signal to the two state variables) is shown during passive motion only (it is always zero during active movements in the absence of any perturbation and noise). All simulations use a canal time constant of 4 s. Note that, because of the integration, the illustrated feedback is scaled by a factor ; see Supplementary methods, ‘Kalman feedback gains’. The box defined by dashed gray lines illustrates the Kalman filter computations. For the rest of mathematical notations, see Table 1.
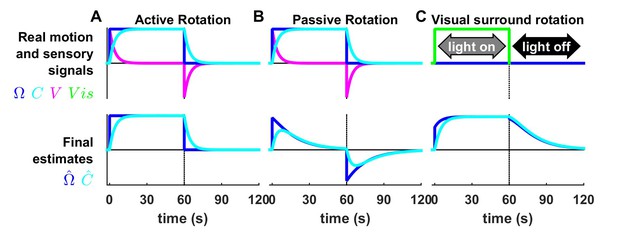
Processing of rotation information during long-duration motion.
(A,B) Simulation of constant velocity rotation lasting 60 s, followed by 60 s where the head doesn’t rotate (top row, , blue). The semicircular canal signal (top row, V, magenta) decreases exponentially (time constant = 4 s) during the rotation ( = –; represented by cyan lines). The deceleration at t = 60 s induces a canal after-effect. During active rotation (A), the final estimates of rotation velocity ( lower row, blue) and canal dynamics (, lower row, cyan) are identical to their real respective values (A, top panel). In contrast, during passive rotation (B), the final estimate of rotation differs from the stimulus: decreases exponentially toward zero, although the decrease is slower (time constant = 16.5 s) than that of the canals. This prolonged estimate arises from the contribution of the internal state variable , which rises at the beginning of rotation as in (A) but reaches a maximum and decreases towards zero. (C) Simulation of optokinetic stimulation, i.e., a visual stimulus rotating at constant velocity (, top row, green) while the head is immobile. The rotation estimate rises immediately to 70% of stimulus velocity at the beginning of the stimulation, and then increases exponentially to 96% of stimulus velocity. rises exponentially to the stimulation velocity but doesn’t exhibit an immediate increase at the beginning of the stimulation. Both and persist at the end of the rotation. The simulated results in B and C match the perceptual (Bertolini et al., 2011) and reflex (Raphan et al., 1979) responses to these stimuli. Furthermore, the rotation estimate is equal to the Kalman feedback signal during passive rotation (B) and visual surround rotation (C). As expected, VN neurons responses follow the same dynamics than in these conditions (Waespe and Henn, 1977; Yakushin et al., 2017). Previous work (Raphan et al., 1979; Laurens and Angelaki, 2011) used an intermediate variable called ‘velocity storage’ to model low-frequency responses to vestibular and visual stimulation. The state variable in the Kalman filter model is identical to the velocity storage. An optimal model based on particle filtering also produced identical results (Laurens and Droulez, 2007; Laurens and Droulez, 2008). The Kalman filter also predicts that rotation perception should last indefinitely during active constant-velocity rotation (A), unlike passive rotation (B), in agreement with experimental findings (Solomon and Cohen, 1992; Guedry and Benson, 1983; Howard et al., 1998; Jürgens et al., 1999). Note, however, that sustained rotation perception during active rotation saturates at about 10°/s in humans (Guedry and Benson, 1983; Howard et al., 1998), possibly related to the saturation of the velocity storage () at velocities of ~20–30°/s in humans (Laurens et al., 2011). Therefore, actively rotating at substantially higher velocities may lead to disorientation and vertigo (as commonly experienced by children and waltz dancers). Note that all Kalman filter simulations do not include this saturation. Sustained rotation perception does not saturate in macaques at velocities up to 200°/s (Solomon and Cohen, 1992).
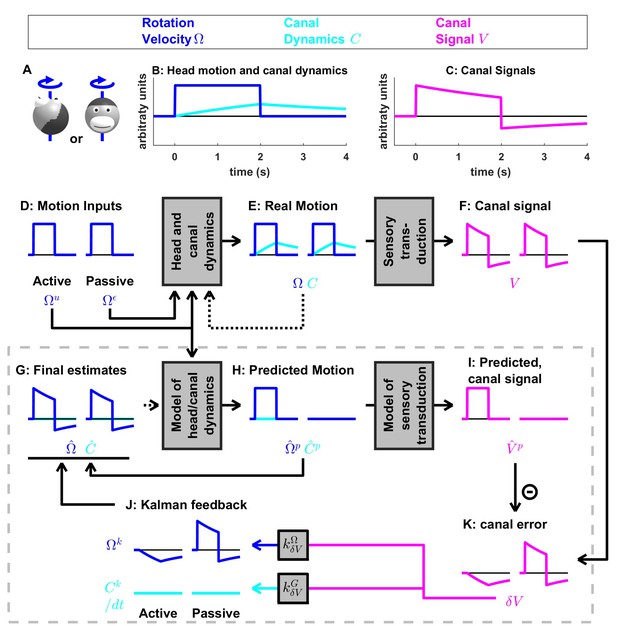
Same simulation as in Figure 4, where the internal model of canals dynamic is not used.
Note error in the final estimate of rotation (G) during both active and passive movements.
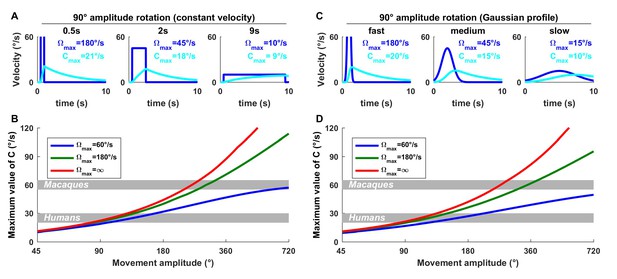
Quantitative analysis of the importance of an internal model of the canals for ecological movements.
The internal variable , which represents the difference between head angular velocity and the canal signal , and needs to be estimated by the internal model of the canals for accurate velocity perception, has low-pass dynamics. Nevertheless, its importance is highest for high velocities. To illustrate this, here we simulate during either (A,B) constant velocity or (C,D) Gaussian-velocity rotations of various amplitudes and durations. (A,C) Rotations with an amplitude of 90° and duration of 0.5, 2 and 9 s (i.e. rotations at 180, 45 and 10°/s, respectively). We find that the peak value of is, 21°/s during a fast movement (0.5 s), whereas it is only 9°/s during a slow movement (9 s). Therefore, for a given movement amplitude, the internal model contribution is larger at larger velocities. (B,D) Maximum value of as a function of movement amplitude for slow (60°/s) and rapid (180°/s, and infinite velocity) movements. During fast movements, may exceed 20°/s during 90° rotation and 40°/s during 180° rotation. We note that the velocity storage likely saturates at ~20–30°/s in humans (Laurens et al., 2011) and ~60°/s in macaques (Waespe et al., 1983) (shown in B and D as gray bands; this saturation is not included in the Kalman filter). For fast rotations (180°/s or more), the saturation would be reached for rotations of 130° in humans (assuming a saturation velocity of 30°/s) and 290° in macaques. These simulations demonstrate that the role of the velocity storage in compensating for canal dynamics is not restricted to long-duration rotations but encompasses natural movements.
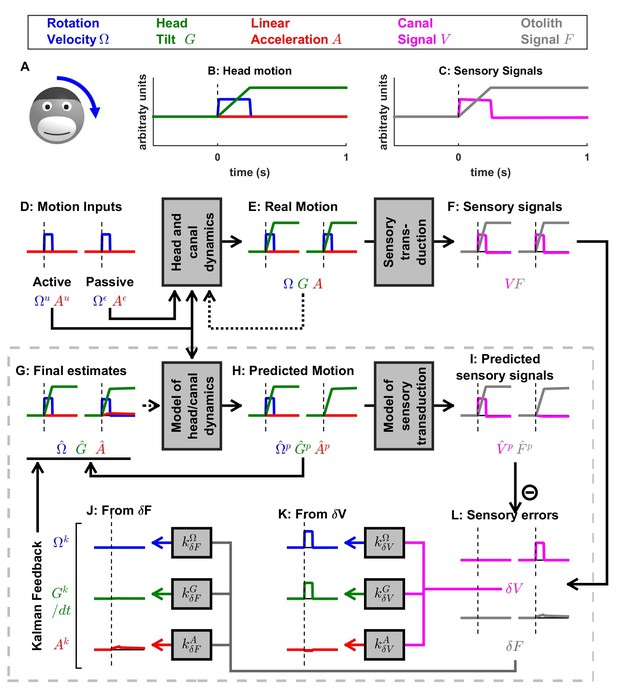
Simulation of short duration head tilt.
(A) Illustration of the stimulus lasting 0.2 s. (B,C) Time course of motion variables and sensory (canal and otolith) signals. (D–L) Simulated variables during active (left panels) and passive motions (right panels). Three state variables are shown: the angular velocity (blue), tilt position and linear acceleration . Continuous arrows represent the flow of information during one time step, and broken arrows represent the transfer of information from one time step to the next. (J, K) Kalman feedback (shown during passive motion only). Two error signals (: canal error; : otolith error) are transformed into feedback to state variables : blue, : green, : red (variable is not shown, but see Figure 5—figure supplement 1 for simulations of a 2-s tilt). Feedback originating from is shown in (J) and from in (K). The feedback to is scaled by a factor (see Supplementary methods, ‘Kalman feedback gains’). Note that in this simulation we consider an active () or passive () rotation velocity as input. The tilt itself is a consequence of the rotation, and not an independent input. The box defined by dashed gray lines illustrates the Kalman filter computations. For the rest of mathematical notations, see Table 1.
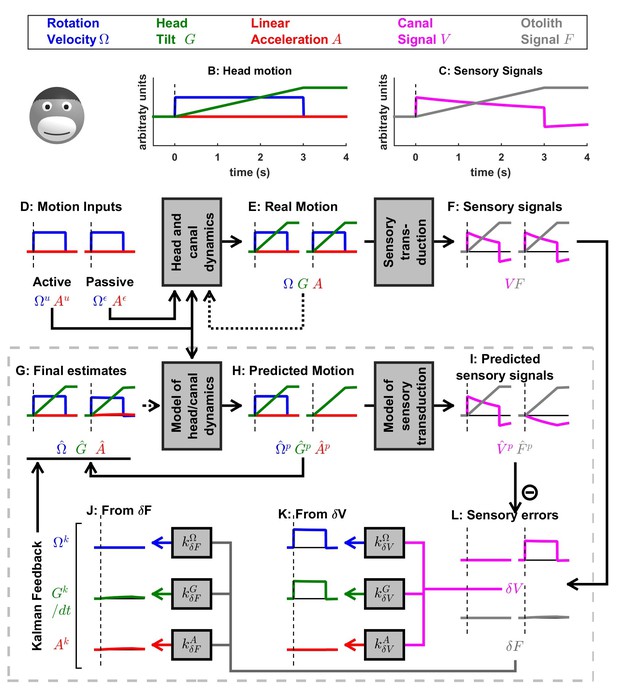
Simulation of medium duration (2 s) head tilt movement.
Panels as in Figure 5; the canal dynamics state variable, , is shown in cyan. Although canal responses exhibit significant dynamics (magenta in panel C), rotation perception remains accurate (panel G, blue traces) during both active and passive tilts (as in Figure 4). The final estimate of tilt (panel G, green traces) is also accurate during active and passive tilts. As a consequence, the otolith error (panel L, gray traces) and the corresponding Kalman feedback (J) are negligible. Note that the feedback to and is scaled by a factor (because of the integration; see Supplementary methods, ‘Kalman feedback gains’).
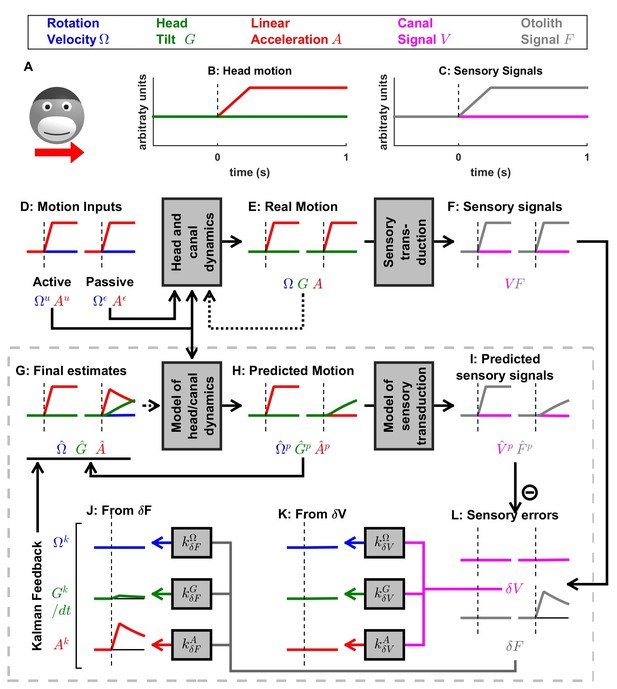
Simulation of short duration translation.
Same legend as Figure 5. Note that is identical in Figures 5 and 6: in terms of sensory inputs, these simulation differ only in the canal signal.
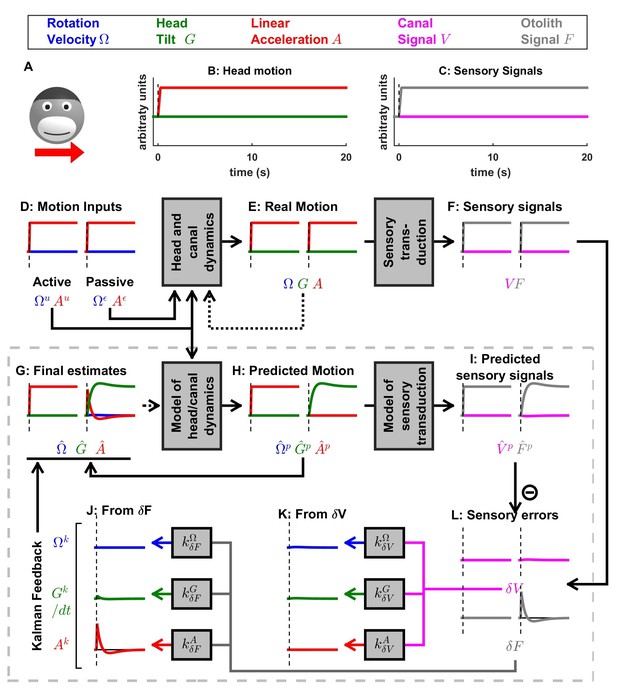
Long duration translation, demonstrating the time course of the somatogravic effect.
Note that slightly overshoots transiently: this is the indirect consequence of an additional feedback from to (not shown here for simplicity; but detailed in Laurens and Angelaki, 2011). In this simulation, we observe that the tilt estimate develops until its magnitude matches the otolith signal . At this point, the corresponding predicted otolith signal matches the real signal . Therefore, the otolith error, , as well as the net acceleration estimate, , vanish. This feedback, which allows the otolith organs to create a rotation signal, has only a low magnitude and a slight effect on the dynamics of in this simulation. In general, however, it allows the otolith system to detect low-frequency head rotation velocity relative to gravity (Figure 6—figure supplement 3; see also Angelaki and Hess (1995a),b; Laurens et al. (2010); Laurens and Angelaki, 2011).
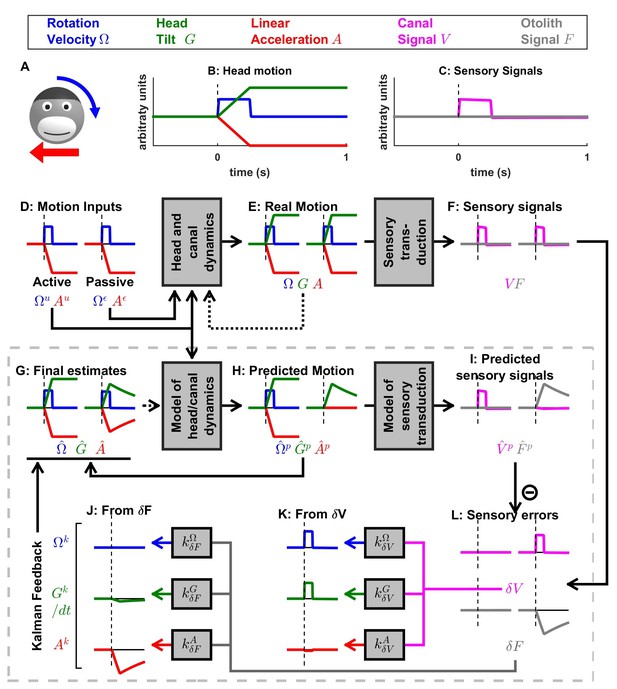
Simulation of simultaneous tilt and translation.
Head tilt (as in Figure 5) and translation (as in Figure 6 but in the opposite direction) are performed simultaneously. As a consequence, the otolith signals (that are identical in Figure 5C and Figure 6C) now cancel each other (panel C, gray trace). This protocol can be seen as an activation of the semicircular canals that indicates a tilt movement, but in the absence of the corresponding tilt-induced activation of the otolith organs. As expected, and in agreement with behavioral (Angelaki et al., 1999; Merfeld et al., 1999) and neuronal findings (Angelaki et al., 2004; Shaikh et al., 2005; Yakusheva et al., 2007; 2008; 2013; Laurens et al., 2013a; Laurens et al., 2013b), the results of a tilt-translation simulation are the sum of a tilt simulation and a translation simulation (the latter being reversed). Interestingly, this simulation illustrates how activation of the semicircular canals without a corresponding activation of the otoliths leads to an otolith error (panel L, gray trace signaling ). This simulates how translation-selective neurons in the vestibular nuclei and cerebellum modulate during tilt-translation in experimental studies using this stimulus (Angelaki et al., 2004; Shaikh et al., 2005; Yakusheva et al., 2007; 2008; 2013; Laurens et al., 2013a; Laurens et al., 2013b).
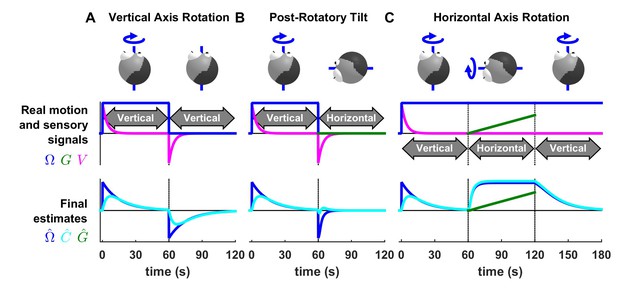
Otolith influence on rotation estimate during passive rotations.
These simulations show that signals from the otoliths (that sense indirectly whether or not the head rotates relative to gravity) can also influence the rotation estimate at low frequencies (this property has been extensively considered by Laurens et al., 2010; Laurens and Angelaki, 2011). (A) Long-duration EVAR (‘Vertical’ refers to the orientation of the rotation axis), similar to Figure 4—figure supplement 2B. The post-rotatory response (from t = 60 s to t = 120 s) is identical (with a reversed sign) to the initial rotatory response (from t = 0 to t = 60 s). (B) Post-rotatory tilt (Angelaki and Hess, 1995a; Laurens et al., 2010), where the head is rapidly reoriented at t = 60 s such that the rotation axis (i.e. around which the head rotated from t = 0 to t = 60 s) becomes horizontal. The post-rotatory response is largely suppressed (time constant = 2.7 s versus 16 s in A). (C) Earth-horizontal Axis Rotation (Angelaki and Hess, 1995b; Laurens et al., 2010), where the head is first rotated around a vertical axis until the canal signal (and rotation estimates and ) subside. Next (t = 60 s) the rotation axis is reoriented. The reorientation does not activate the canals (magenta). Yet, a rotation estimate develops, rising exponentially with a short time constant (3.1 s) close to the time constant of the post-rotatory response (as in Laurens et al., 2010). The rotation estimates and decrease exponentially when the rotation axis is reoriented back to vertical at t = 120 s. These simulations illustrate the influence of the velocity feedback from the otoliths to (detailed can be found in Laurens and Angelaki, 2011). As in Figure 4—figure supplement 1B,C, the rotation estimate is equal to the Kalman feedback signal in (B) and (C). As expected, VN neurons responses follow the same dynamics than in these conditions (Reisine and Raphan, 1992; Yakushin et al., 2017).
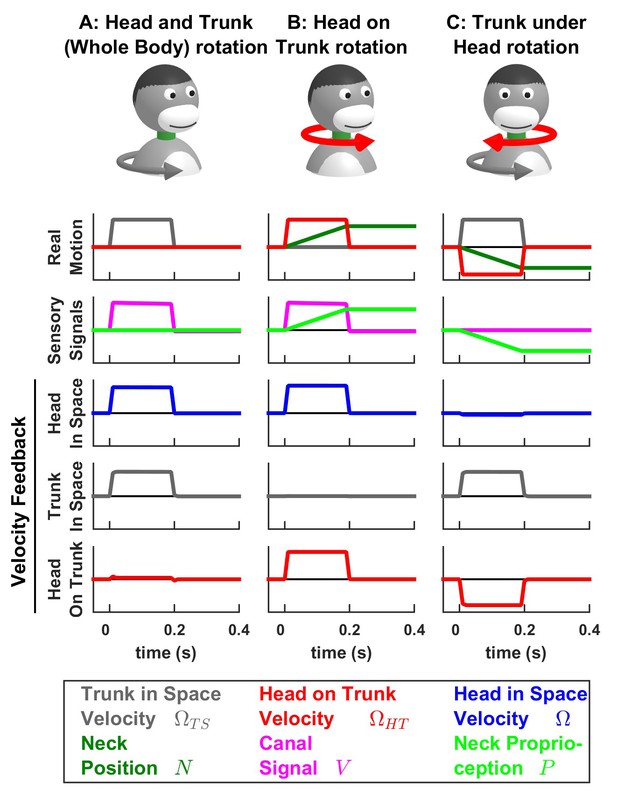
Simulations of passive trunk and head movements.
We use a variant of the Kalman filter model (see Supplementary methods) that tracks the velocity of both head and trunk (trunk in space: gray; head in space: blue; head on trunk: red) based on semicircular canal and neck proprioception signals. The real motion (first line), sensory signals (second line) and velocity feedback signals (third to fifth lines) are shown during (A) passive whole head and trunk rotation, (B) passive head on trunk rotation, and (C) passive trunk under head rotation. See Figure 7—figure supplement 1–3 for other variables and simulations of active motion.
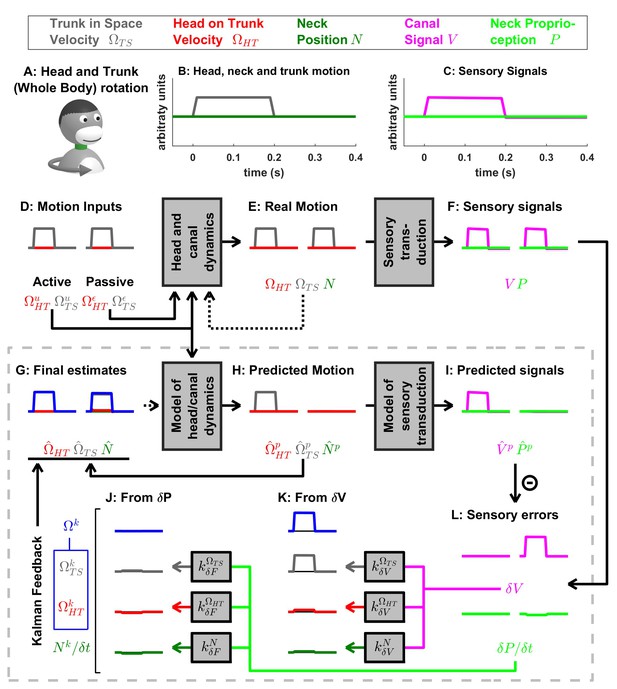
Active and passive head and trunk rotation.
(A) Illustration of the stimulus. (B) Motion variables. (C) Sensory signals. (D–J) Simulated variables during active (left panels) and passive motions (right panels). Continuous arrows represent the flow of information during one time step, and broken arrows the transfer of information from one time step to the next. (K,J) Kalman feedback, shown during passive motion only (it is always zero during active movements in the absence of any perturbation and noise). For the rest of mathematical notations, see Table 1. In this simulation, the head/trunk rotates for 0.2 s (thus, canal dynamics are excluded for simplicity). During active rotation, the predicted motion and sensory signals match the real motion and sensory signals, and sensory errors are null. During passive rotation, sensory errors are transformed into feedback signals (J,K) to (gray), (red) and (green; note that the feedback is scaled by , see Supplementary methods, ‘Feedback signals during neck movement’). The feedback about head velocity in space (blue) is not directly computed by the model, but defined as the sum of and . The canal error (panel L, magenta), which is similar to Figures 3 and 4, is converted into a feedback about trunk in space velocity (, panel K, gray) and a corresponding feedback about head in space velocity , whereas feedback originating from has a limited magnitude (see Table 3). The proprioceptive error (panel L, green; note that is scaled by , see Supplementary methods, ‘Feedback signals during neck movement’) is close to zero. In conclusion, as expected, an activation of the semicircular canals (when proprioceptive information does not indicate a movement of the neck) induces a perception of whole head and trunk rotation, where the head and the trunk move together.
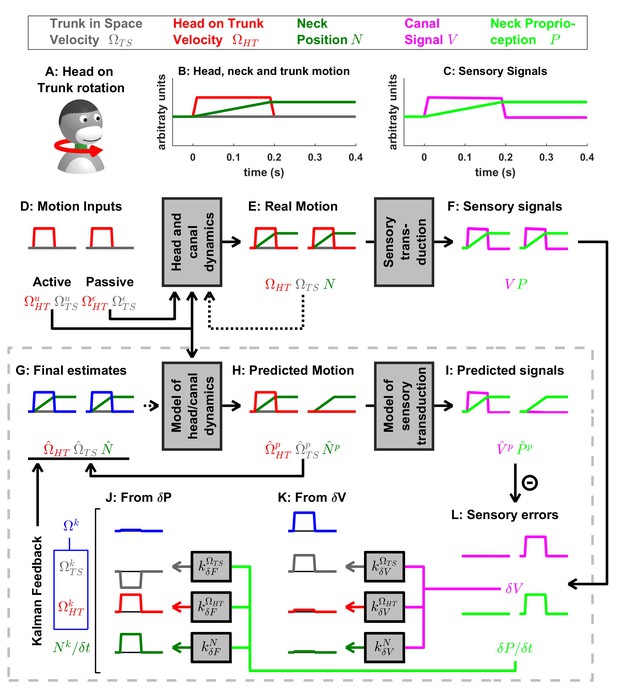
Active and passive head on trunk rotation.
(A) Illustration of the stimulus. (B) Motion variables: the head rotates on the trunk (, red) for 0.2 s, and the neck position increases during this period. (C) The sensory signals encode head velocity (canal signal, , magenta) and neck position (proprioceptive signal, , green). (D–J) Simulated variables during active (left panels) and passive motion (right panels). Continuous arrows represent the flow of information during one time step, and broken arrows the transfer of information from one time step to the next. (K,J) Kalman feedback, shown during passive motion only (it is always zero during active movements in the absence of any perturbation and noise). Passive motion induces the same canal error as in Figure 7—figure supplement 1. Furthermore, during passive motion, there is a small but significant mismatch between the predicted and actual proprioceptive signals (see Supplementary methods, ‘Feedback signals during neck movements’) resulting in a proprioceptive error . Note that, although proprioceptive signals encode neck position, encodes the velocity of the movement (panel L; green). As in Figure 7—figure supplement 1, the error is converted into a feedback that encodes rotation of the trunk in space (panel K, , gray). However, the error is converted into an opposite feedback (panel J, , gray). Therefore, the total trunk rotation feedback and the final estimate of trunk velocity (panel G, gray) are zero. Furthermore, is converted into feedback that encodes the velocity of the head relative to the trunk (panel J, red).
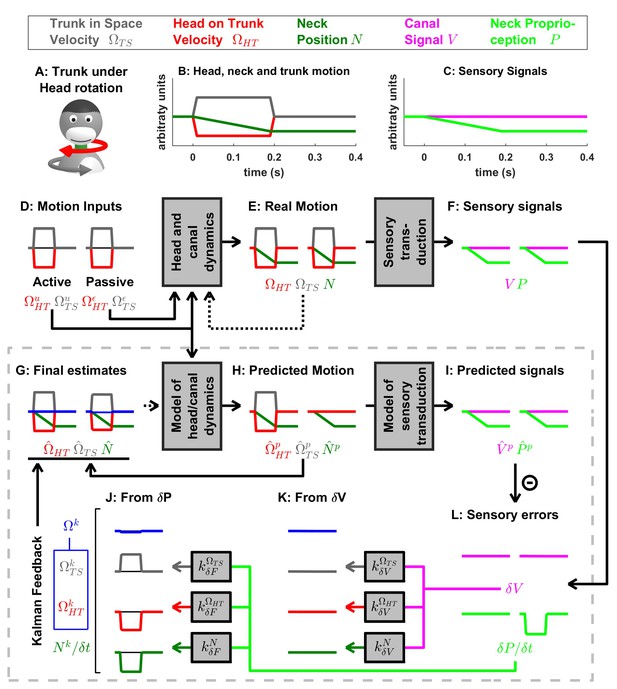
Active and passive rotation of the trunk while the head is stationary.
This paradigm illustrates information processing in response to neck rotation in the absence of semicircular canal stimulation. Signals and parameters as in Figure 7—figure supplements 1 and 2.
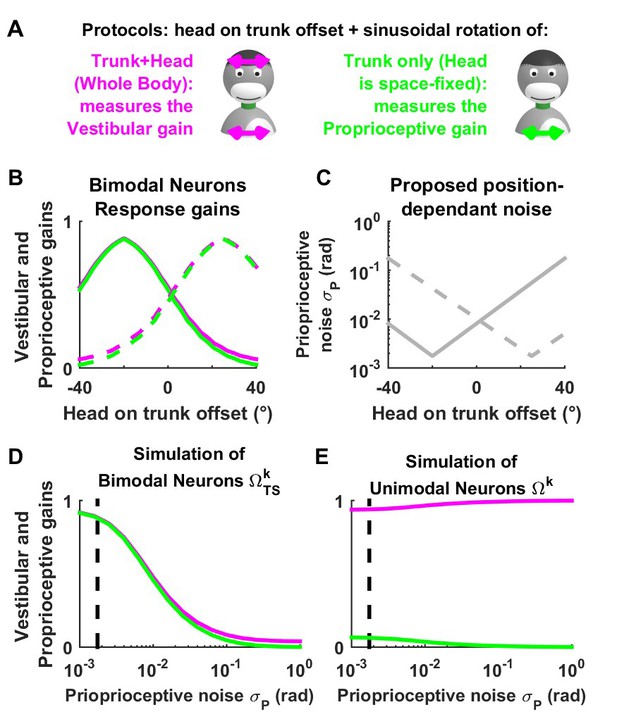
Simulated tuning of unimodal and bimodal neuron as a function of neck position offset.
(A) Experimental manipulation used by (Brooks and Cullen, 2009) to demonstrate that the response of bimodal neurons to sinusoidal motion varies if a head rotation offset is superimposed to the sinusoidal stimulus. (B) Schematic responses of two bimodal neurons (one in solid lines and the other in broken lines). Individual neurons exhibit hill-shaped tuning curves as a function of head rotation offset, and the location of the peak varies from neuron to neuron. Remarkably, neurons exhibit similar tuning curves in response to canal stimulation (rotation of the head and trunk; magenta curves), and to neck proprioceptor stimulation (rotation of the trunk only, green). The fact that these tuning curves are matched allows bimodal neurons to encode trunk velocity during combined trunk and head motion, regardless of head position offset (Brooks and Cullen, 2009). However, the reason why bimodal neuron responses are modulated as a function of head offset is unknown. We propose that head rotation offset extends or contracts individual neck muscles and affects the signal-to-noise ratio of their afferent proprioceptive signals. Assuming that individual neurons receive proprioceptive afferents from distinct pools of muscles, the signal-to-noise ratio of proprioceptive signals would form a distinct curve in each neuron (C: the solid and broken curves correspond to the solid and broken curves in B). (D) Simulations of bimodal neurons (i.e. the trunk velocity feedback ) during passive trunk and head rotation (magenta) and trunk only rotation (green), with increasing amount of proprioceptive noise (the vertical broken lines in (A,B) indicate the value used in other figures). We find that increasing leads to a concomitant decrease of the response gains in both conditions. The responses curves in (B) can be obtained by combining panels (C) and (D). (E) Furthermore, we find that the simulated response of unimodal neurons (assumed to encode the trunk velocity feedback ) is independent of proprioceptive noise, in agreement with experimental results (Brooks and Cullen, 2009) that demonstrate that that gain of unimodal neurons is not sensitive to static neck position.
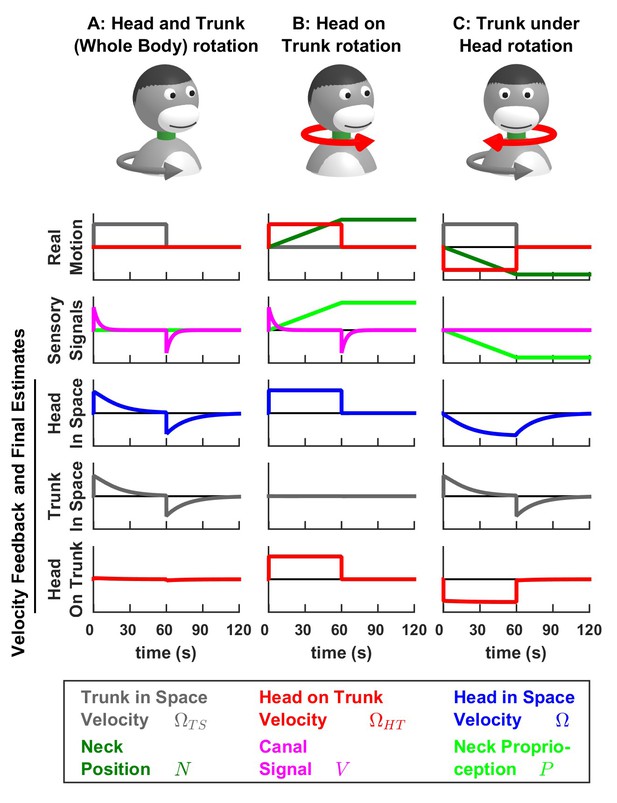
Long-duration passive head and trunk movements.
The dynamics of head and trunk rotation perception during combined passive head and trunk movements were studied by (Mergner et al., 1991). Here, we reproduce the results of this study by simulating the same movements as in Figure 7 with a longer duration (60 s). The feedback signals about head in space velocity (third row, blue), trunk in space velocity (fourth row, gray) and head on trunk velocity (fifth row, red) are shown. During passive motion, this feedback is identical to the final motion estimate, and therefore, the curves in rows 3 to 5 also represent the perception of motion during these stimuli. A combined rotation of the head and trunk (A) activates the canals (magenta curve). Following the initial acceleration at t = 0, canal signals fade away with a time constant of 4 s. A canal aftereffect occurs following the deceleration at t = 60 s. The estimates of head in space and trunk in space velocity are identical to each other, and exhibit similar dynamics as the canal signal, although with a longer time constant of 16.5 s (for the same reasons as those described in Figure 4—figure supplement 1B). When rotating the head while the trunk is stationary (B), the simulated estimates of head in space (third row, blue) and head on trunk (fifth row, red) velocity persist indefinitely, as illustrated experimentally by Mergner et al. (1991). The difference in the time course of the signals in panels A and B is due to the additional presence of the neck proprioceptive signal. Strinkingly, during long duration rotation (C), the estimate of trunk in space velocity (fourth row, gray) decreases with an identical time constant as in (A), although the underlying sensory signals, that originate from the canals in A and from neck proprioception in C, have fundamentally different dynamics. Futher, the perception of trunk rotation is replaced by an illusion of head rotation (third row, blue) in an opposite direction. These model predictions exactly match experimental findings by Mergner et al. (1991).
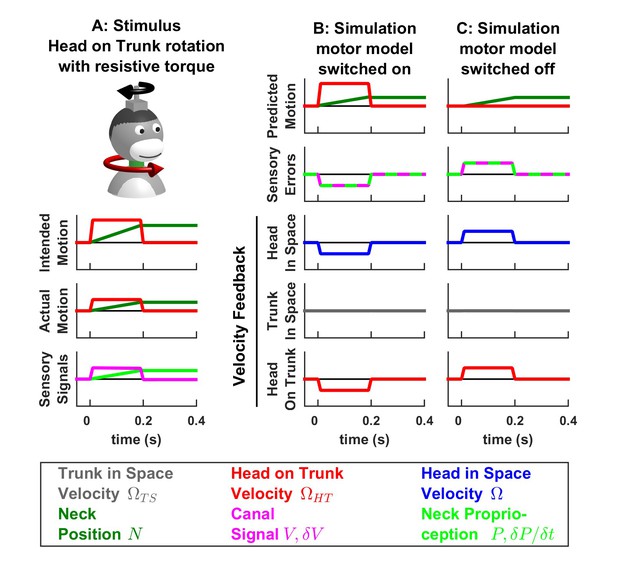
Modeling the impact of perturbing motor activity during active movement.
Experimental studies (Roy and Cullen, 2004; Carriot et al., 2013; Brooks et al., 2015) have shown that VN neurons encode active and passive motion (i.e. head velocity or linear acceleration) indiscriminately when active movements are blocked or perturbed by unexpected torques, as simulated here. (A) Simulated experimental condition, where the animal attempts to perform a head movement (while the trunk remains immobile). Due to an unexpected resistive torque, the actual movement amplitude and velocity are only half of the intended motion, as indicated by neck position (green) and head velocity (red) traces (compare ‘Intended Motion’ and ‘Actual Motion’ panels). The resulting sensory signals (canals, magenta, and neck proprioceptors, green) encode the actual (reduced) velocity and amplitude of the movement. (B) Kalman filter simulation using the same model as in Figure 7. Because the actual head velocity (A, ‘Actual Motion’) is slower than the predicted velocity (B, ‘Predicted Motion’), a negative canal error occurs. As a result, the velocity feedback about head in space (blue) is negative. (C) Alternative simulation, where the motor input to the internal model () is set to zero. In this situation, the motion is perceived as if it was entirely passive (exactly as in Figure 7—figure supplement 2, with only half the amplitude) and therefore the feedback pathways encode a positive head in space velocity signal. Thus, the simulation in B predicts that central neurons that encode feedback signals about head velocity in space, are inhibited in this experimental condition. In contrast, the simulation in C predicts that these neurons are activated (and encode net head velocity with the same gain as in Figure 7—figure supplement 2). Experiments (Brooks et al., 2015) yield results consistent with (C) but contrary to (B). Note that, in (B) and (C), the predicted neck position is close to the actual position and the proprioceptive error encodes a velocity error (close to ). This occurs for the same reason as during passive neck movements, as described in Supplementary methods, ‘Feedback signals during neck movement’.
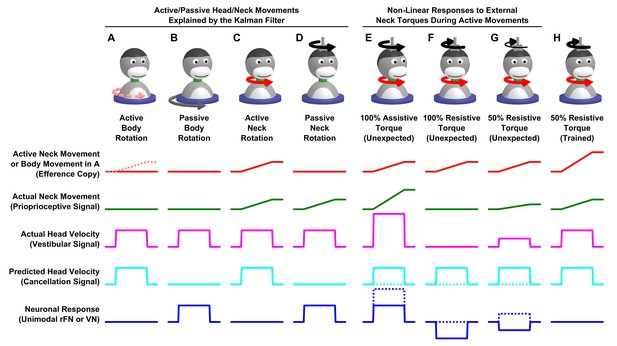
Neuronal responses when perturbing motor activity during active movement.
This figure recapitulates neuronal responses (VN and unimodal rFN neurons encoding passive head rotations) during various combinations of passive and active whole body or neck rotation, and corresponding sensory and motor variables. (A-D) Movements modeled in Figure 7 and Figure 7—figure supplement 1–5, where neurons encode the difference between the vestibular rotation signal (magenta) and a cancellation signal (cyan), which in the Kalman filter model is interpreted as a predicted vestibular signal based on efference copies of neck (red lines and arrows) or body (in A; pink broken line and arrow) motor commands. (E-H) Here, assistive or resistive torques are applied during an active neck movement, increasing or preventing the actual neck rotation (magenta). If responses were additive (solid lines), protocols E and F would be equivalent to (C + D) and (C-D), respectively, and neuronal firing rate would encode the head rotation component generated by the passive torque. In contrast, Roy and Cullen (2004) and Brooks and Cullen (2014) showed that VN neurons encode net head velocity (broken line), indicating that the process of transforming motor commands into a cancellation signal stops. Similarly, Brooks et al. (2015) applied a resistive torque during active head movements and found that, early in the experiments, rFN encoded net head velocity (G, broken line). They also found that the animals learned to compensate for the torque, at which point rFN neurons responses were again suppressed (H). Together, these results indicate that a mismatch between expected and actual head proprioception occurring during active neck movements results in a non-additive response that has been modeled by a non-linear ‘switching’ mechanism (Roy and Cullen, 2004). In contrast, vestibular mismatches do not induce any non-linear interactions, at least in the ensemble of stimuli considered here.
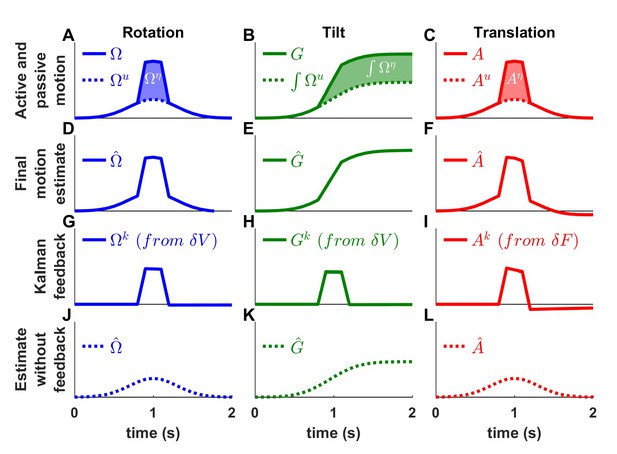
Interaction of active and passive motion.
Active movements (Gaussian profiles) and passive movements (short trapezoidal profiles) are superimposed. (A) Active () and passive () rotations. (B) Head tilt resulting from active and passive rotations (the corresponding tilt components are and ). (C) Active () and passive () translations. (D-F) Final motion estimates (equal to the total motion). (G-I) The Kalman feedback corresponds to the passive motion component. (J-K) Final estimates computed by inactivating all Kalman feedback pathways. These simulations represent the motion estimates that would be produced if the brain suppressed sensory inflow during active motion. The simulations contradict the alternative scheme of Figure 1—figure supplement 1A.
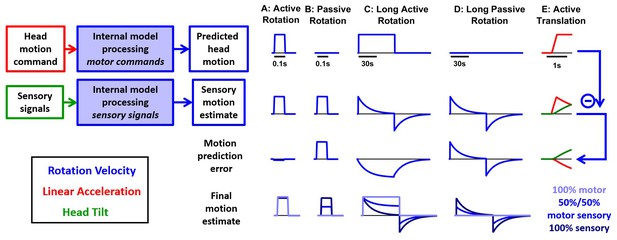
Simulations of the alternative scheme where motor commands cancel the output of a sensory internal model.
In this figure, we consider an alternative scheme (Figure 1—figure supplement 1B), where the motor commands (first row), which are assumed to encode head angular velocity and linear acceleration (as in the Kalman filer model), are used to cancel the output of a ‘sensory only’ internal model (second row, also identical to the Kalman filter model) to compute motion prediction errors (third row), instead of sensory prediction error, as in the Kalman filter model. (A) During a short active rotation (same as in Figure 3), both the motor prediction and the sensory self-motion estimate are close to the real motion and therefore the motor prediction cancels the sensory estimate accurately. (B) Similarly to Figure 3, the motor prediction is null and the sensory estimate is not cancelled during passive rotation. (C) During long-duration active rotation (same motion as in Figure 4—figure supplement 1A,B), the motor prediction (top row) does not match the sensory signal (second row), resulting in a substantial prediction error (third row). This contrasts with Kalman filter simulations, where no sensory prediction errors occur during active motion. (D) During long-duration passive motion, results agree with Kalman filter predictions. (E) During active translation (same motion as in Figure 6), the somatogravic effect would induce a tilt illusion (green) and an underestimation of linear acceleration (red), again leading to motion prediction errors. Thus, although the predictions of this alternative model resemble those of the Kalman filter during short active rotations, they differ during long rotations or active translations and are contradicted by experimental observations. The last row shows the final self-motion estimate obtained by computing a weighted average of the predicted head motion and sensory motion estimates. Three different weights are considered: 100% motor signals (dark blue), 100% sensory estimates (dark blue) or 50%/50% (blue).
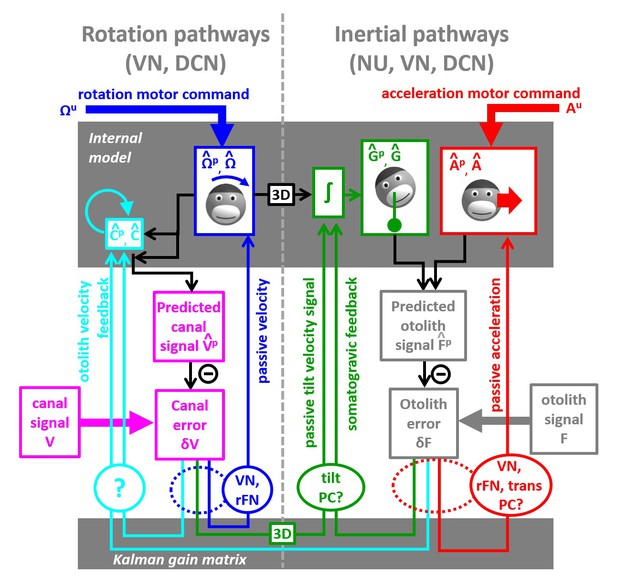
Schematic diagram of central vestibular computations.
This diagram is organized to offer a synthetic view of the processing elements, as well as their putative neural correlates. An internal model (top gray box) predicts head motion based on motor commands and receives feedback signals. The internal model computes predicted canal and otolith signals that are compared to actual canal and otolith inputs. The resulting sensory errors are transformed by the Kalman gain matrix into a series of feedback ‘error’ signals. Left: canal error feedback signals; Right: otolith error feedback signals. Rotation signals are spatially transformed (‘3D’ boxes) into tilt velocity signals. Ovals indicate putative neuronal correlates of the feedback signals (VN: vestibular only vestibular nuclei neurons; rFN: rostral fastigial nuclei neurons, PC: Purkinje cells in the caudal vermis, DCN: deep cerebellar nuclei).
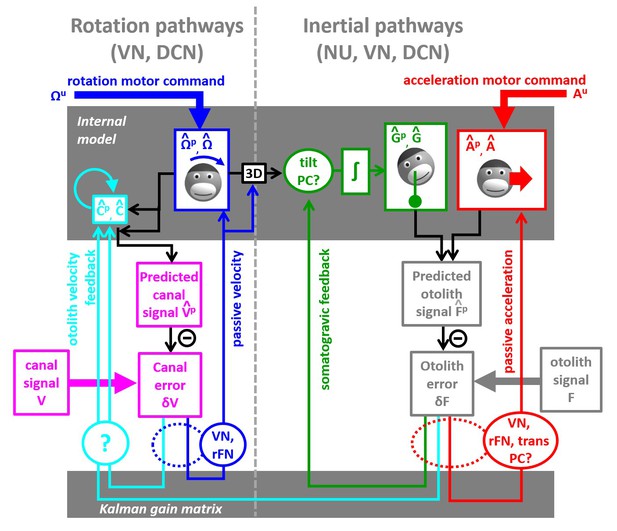
Alternative diagram.
Where the Kalman feedback encoding passive tilt velocity is removed. Instead, a final rotation estimate is computed and transformed into a tilt velocity signal.
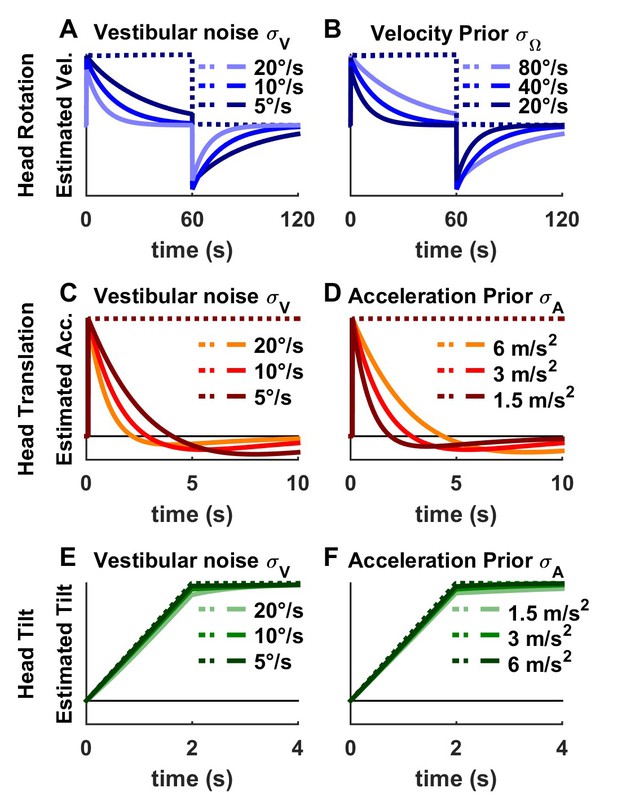
Influence of model parameters.
This figure recapitulates the consequences of increasing or decreasing the Kalman filter’s parameters (: vestibular noise; : standard deviation of prior distribution of passive rotation and rotational motor error; : standard deviation of passive linear acceleration and translational motor error) by a factor of 2. (A,B) Influence during rotation (as in Figure 4—figure supplement 1A,B). During passive rotation (solid lines), the rotation estimate decreases over time because (1) noise on the vestibular signal causes the rotation estimate of be unreliable and (2) the prior on rotation velocity drives the unreliable estimate towards zero. Accordingly, the time constant of the estimated rotation velocity decreases when vestibular noise increases or when the velocity prior is stronger (i.e. is low). There is also a slight decrease of the rotation estimate at t = 0 (that has a gain of 0.8, 0.94 and 0.98 when varies from, 20°/s to 5°/s). During active rotation (broken line), the rotation estimate is driven by motor commands and independent of the model’s parameters (all lines are superimposed). (C,D) Influence during translation (as in Figure 6—figure supplement 1). During passive translation (solid lines), the translation estimate decreases over time because (1) noise on the vestibular signal causes the estimate of tilt to be unreliable and (2) the prior on linear acceleration generates a tilt illusion that minimizes the acceleration estimate (the tilt estimate, not shown here, mirrors the translation estimate as in Figure 6). Accordingly, the time constant of the estimated translation decreases when vestibular noise increases or when the acceleration prior is stronger (i.e. is low). During active translation (broken line), the rotation estimate is driven by motor commands and thus is independent of the model’s parameters (all lines are superimposed). (E,F) Influence during tilt (as in Figure 5). During passive tilt, the estimated tilt is practically veridical, irrespective of model parameters, except when the vestibular noise is large (E) in which case the estimate undershoots head tilt transiently (light green line at t = 2 s) because the gain of the rotation estimate is lower.
Tables
List of motion variables and mathematical notations.
https://doi.org/10.7554/eLife.28074.026Motion variables | |
Head rotation velocity (in space) | |
G | Head Tilt |
Linear Acceleration | |
Canals dynamics | |
Trunk in space rotation velocity (variant of the model) | |
Head on trunk rotation velocity (variant of the model) | |
Neck position (variant of the model) | |
Matrix containing all motion variables in a model | |
Sensory variables | |
Semicircular canal signal | |
Otolith signal | |
Neck proprioceptive signal | |
Visual rotation signal | |
Matrix containing all sensory variables in a model | |
Accent and superscripts (motion variables) | |
Real value of a variable | |
Final estimate | |
Predicted (or preliminary) estimate | |
Motor command affecting the variable | |
Perturbation or motor error affecting the variable (standard deviation ) | |
Standard deviation of | |
Kalman feedback on the variable | |
Accent and superscripts (sensory variables) | |
Real value of a variable | |
Predicted value | |
Sensory noise | |
Standard deviation of | |
Sensory error | |
Kalman gain of the feedback from S to a motion variable X | |
Other | |
Time step used in the simulations | |
Transposed of a matrix M | |
Time constant of the semicircular canals |
Kalman feedback gains during EVAR and tilt/translation.
Some feedback gains are constant independently of while some other scale with (see Supplementary methods, ‘Feedback gains’ for explanations). Gains that have negligible impact on the motion estimates are indicated in normal fonts, others with profound influence are indicated in bold. The feedback gains transform error signals into feedback signals.
Gains during EVAR | Gains during tilt | Notes | ||
---|---|---|---|---|
Canal feedbacks | 0.94 | 0.94 | ||
0.19 t | 0.23 t | Integrated over time | ||
0.00 | 0.90 t | |||
0.00 | -0.90 t | Negligible | ||
Otolith feedbacks | - | 0.00 | Negligible | |
- | 0.14 t | Integrated over time | ||
- | 0.76 t | |||
- | 0.99 |
Kalman feedback gains during head and neck rotation.
As in Table 2, some feedback gains are constant and independently of , while some others scale with or inversely to (see Supplementary methods, ‘Feedback gains of the model of head and neck motion’ for explanations). Gains that have negligible impact on the motion estimates are indicated in normal fonts, others with profound influence are indicated in bold. The feedback gains and are computed as and .
Gains | Notes | ||
---|---|---|---|
Canal feedbacks | 0.85 | ||
0.10 | |||
0.05 t | Negligible | ||
0.22 t | Integrated over time | ||
0.95 | |||
Proprioceptive feedbacks | -0.84/t | ||
0.89/t | |||
0.94 | |||
0.03 | Negligible | ||
0.05/t | Negligible |
Additional files
-
Transparent reporting form
- https://doi.org/10.7554/eLife.28074.034