Cerebellar learning using perturbations
Figures
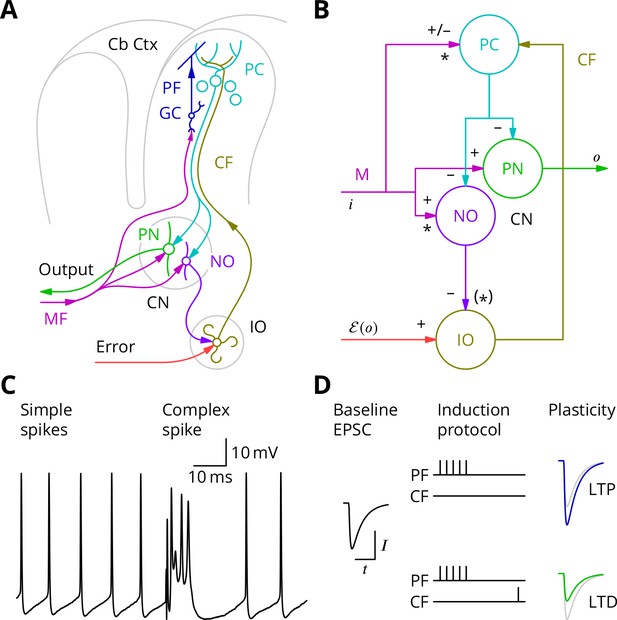
The cerebellar circuitry and properties of Purkinje cells.
(A) Simplified circuit diagram. MF, mossy fibres; CN, (deep) cerebellar nuclei; GC, granule cells; Cb Ctx, cerebellar cortex; PF, parallel fibres; PC, Purkinje cells; PN, projection neurones; NO, nucleo-olivary neurones; IO, inferior olive; CF, Climbing fibres. (B) Modular diagram. The signs next to the synapses indicate whether they are excitatory or inhibitory. The granule cell and indirect inhibitory inputs they recruit have been subsumed into a bidirectional mossy fibre–Purkinje cell input, M. Potentially plastic inputs of interest here are denoted with an asterisk. , input; , output; , error (which is a function of the output). (C) Typical Purkinje cell electrical activity from an intracellular patch-clamp recording. Purkinje cells fire two types of action potential: simple spikes and, in response to climbing fibre input, complex spikes. (D) According to the consensus plasticity rule, a complex spike will depress parallel fibre synapses active about 100 ms earlier. The diagram depicts idealised excitatory postsynaptic currents (EPSCs) before and after typical induction protocols inducing long-term potentiation (LTP) or depression (LTD). Grey, control EPSC; blue, green, post-induction EPSCs.
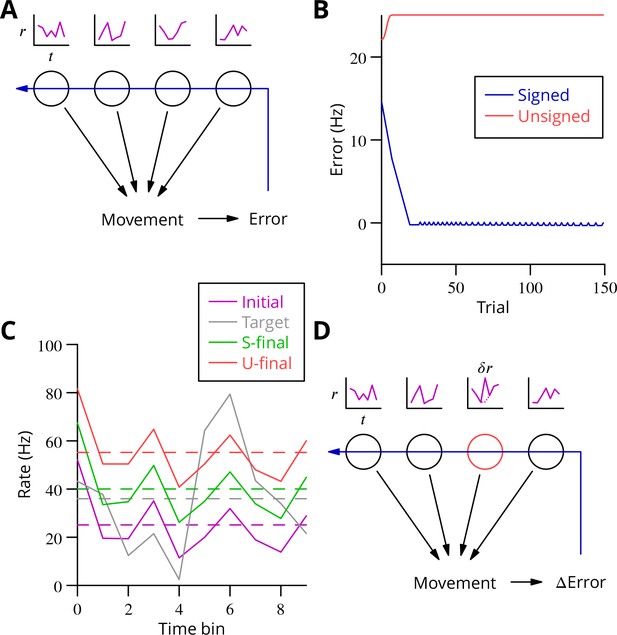
Analysis of cerebellar learning.
(A) The model of cerebellar learning we address is to adjust the temporal firing profiles (miniature graphs of rate as a function of time, , magenta) of multiple cerebellar output neurones (nuclear projection neurones) (black circles) to optimise a movement, exploiting the evaluated movement error, which is fed back to the cerebellar circuit (Purkinje cells) (blue arrow). (B) The Marr-Albus-Ito theory was simulated in a network simulation embodying different error definitions (Results, Materials and methods). The Marr-Albus-Ito algorithm is able to minimise the average signed error (blue, Signed) of a group of projection neurones, but not the unsigned error (red, Unsigned). (C) However, as expected, optimising the signed error does not optimise individual cell firing profiles: comparison of the underlying firing profiles (initial, magenta; final, green) with their target (grey) for a specimen projection neurone illustrates that neither the temporal profile nor even the mean single-cell firing rate is optimised. If the unsigned error is used, there is no convergence and the firing rates simply saturate (red) and the error increases. (D) A different learning algorithm will be explored below: stochastic gradient descent, according to which temporally and spatially localised firing perturbations (, red, of a Purkinje cell) are consolidated if the movement improves; this requires extraction of the change of error ( Error).
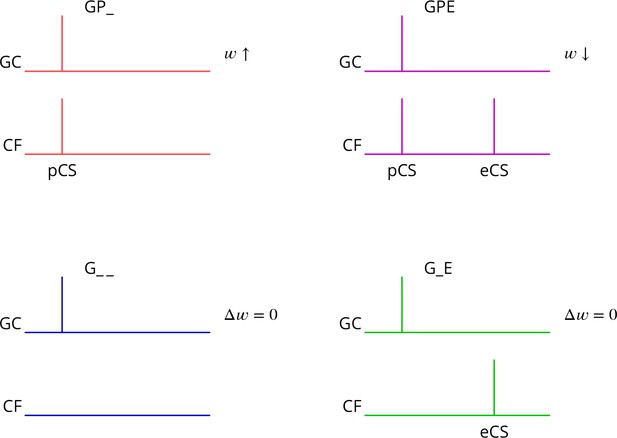
Predicted plasticity rules.
Synchronous activation of granule cell synapses and a perturbation complex spike (pCS) leads to LTP (GP_, increased synaptic weight ; top left, red), while the addition of a succeeding error complex spike (eCS) leads to LTD (GPE, top right, magenta). The bottom row illustrates the corresponding ‘control’ cases from which the perturbation complex spike is absent; no plasticity should result (G_ _ blue and G_E green).
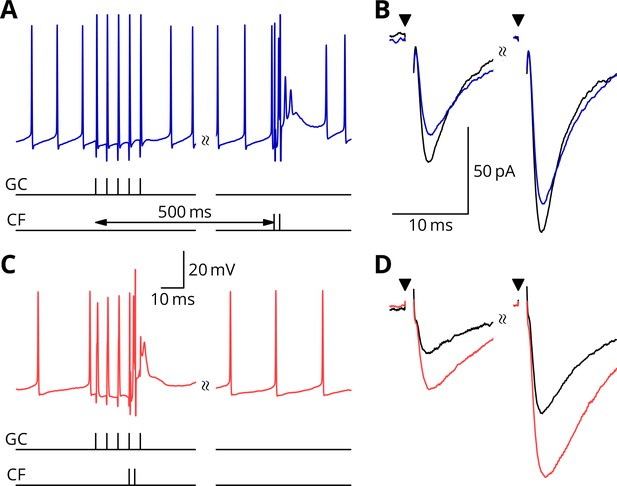
Simultaneous granule cell and climbing fibre activity induces LTP.
(A) Membrane potential (blue) of a Purkinje cell during an induction protocol (G_ _) where a burst of 5 granule cell stimuli at 200 Hz was followed after 0.5 s by a pair of climbing fibre stimuli at 400 Hz. (B) Average EPSCs recorded up to 10 min before (black) and 20–30 min after the end of the protocol of A (blue). Paired test stimuli (triangles) were separated by 50 ms and revealed the facilitation typical of the granule cell input to Purkinje cells. In this case, the induction protocol resulted in a small reduction (blue vs. black) of the amplitude of responses to both pulses. (C) Purkinje cell membrane potential (red) during a protocol (GP_) where the granule cells and climbing fibres were activated simultaneously, with timing otherwise identical to A. (D) EPSCs recorded before (black) and after (red) the protocol in C. A clear potentiation was observed in both of the paired-pulse responses.
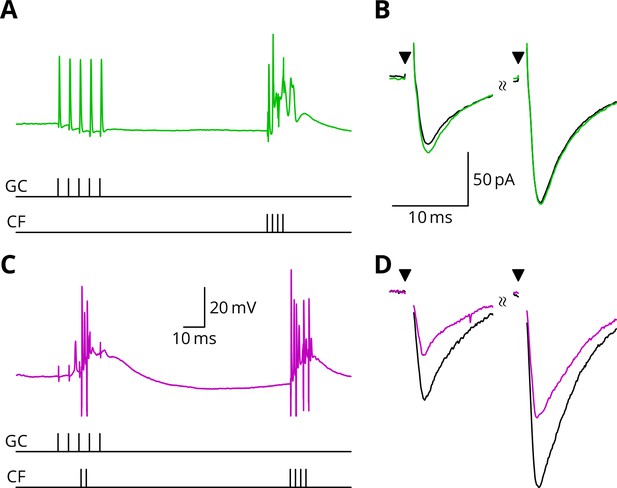
LTD requires simultaneous granule cell and climbing fibre activity closely followed by an additional complex spike.
(A) Membrane potential of a Purkinje cell (green) during a protocol where a burst of five granule cell stimuli at 200 Hz was followed after 100 ms by four climbing fibre stimuli at 400 Hz (G_E). (B) Average EPSCs recorded up to 10 min before (black) and 20–30 min after the end of the protocol of A (green). The interval between the paired test stimuli (triangles) was 50 ms. The induction protocol resulted in little change (green vs. black) of the amplitude of either pulse. (C) Purkinje cell membrane potential (magenta) during the same protocol as in A with the addition of a pair of climbing fibre stimuli simultaneous with the granule cell stimuli (GPE). (D) EPSCs recorded before (black) and after (magenta) the protocol in C. A clear depression was observed.
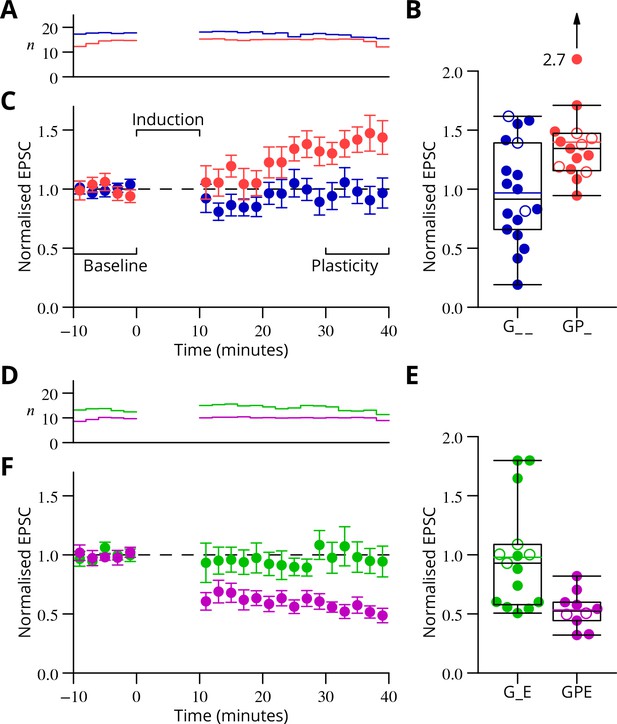
Time course and amplitude of plasticity.
(A) number, (B) box-and-whisker plots of individual plasticity ratios (coloured lines represent the means, open symbols represent cells with failures of climbing fibre stimulation; see Materials and methods) and (C) time course of the mean EPSC amplitude for GP_ (red) and G_ _ (blue) protocols of Figure 4, normalised to the pre-induction amplitude. Averages every 2 min, mean sem. Non-integer arise because the numbers of responses averaged were normalised by those expected in two minutes, but some responses were excluded (see Materials and methods) and some recordings did not extend to the extremities of the bins. Induction lasted for 10 min starting at time 0. (D, E) and (F) similar plots for the GPE (magenta) and G_E (green) protocols of Figure 5.
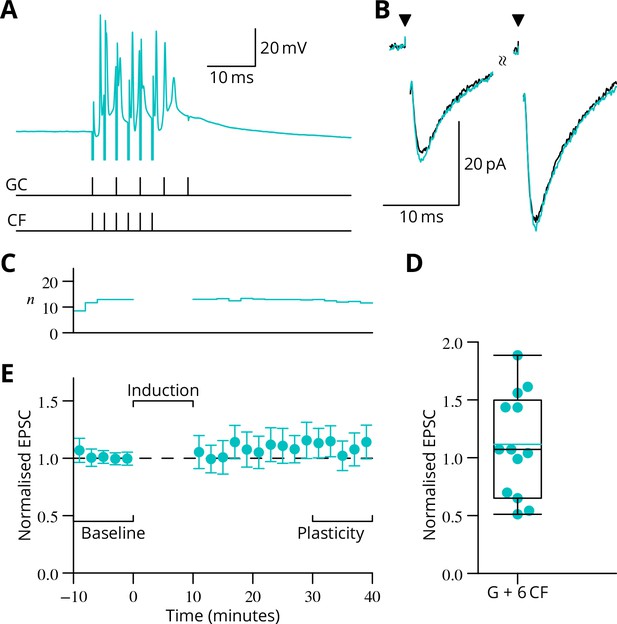
In complementary experiments, we examined the outcome of applying a burst of six climbing fibre stimuli simultaneously with the granule cell burst.
(A) Illustration of the induction protocol. (B) Specimen EPSCs before (black) and after (cyan) induction. (C) Time course of mean normalised EPSC amplitude ( SEM). (D) Scatter plot of plasticity ratios.
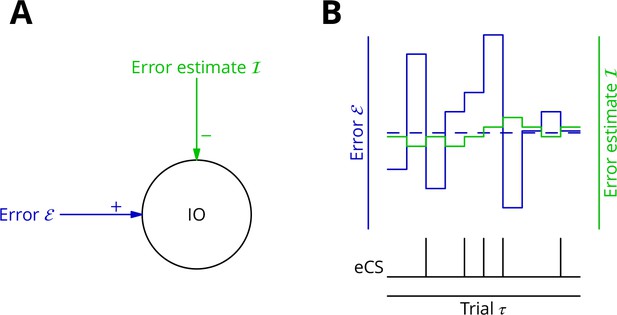
Adaptive tracking to cancel the mean error input to the inferior olive.
(A) The olive is assumed to receive an excitatory signal representing movement error and an inhibitory input from the nucleo-olivary neurones of the cerebellar nuclei. (B) The inputs to the inferior olive are represented in discrete time ()—each bar can be taken to represent a discrete movement realisation. The error (blue) varies about its average (dashed blue line) because perturbation complex spikes influence the movement and associated error randomly. The strength of the inhibition is shown by the green trace. When the excitatory error input exceeds the inhibition, an error complex spike is emitted (bottom black trace) and the inhibition is strengthened by plasticity, either directly or indirectly. In the converse situation and in the consequent absence of an error complex spike, the inhibition is weakened. In this way, the inhibition tracks the average error and the emission of an error complex spike signals an error exceeding the estimated average. Note that spontaneous perturbation complex spikes are omitted from this diagram.
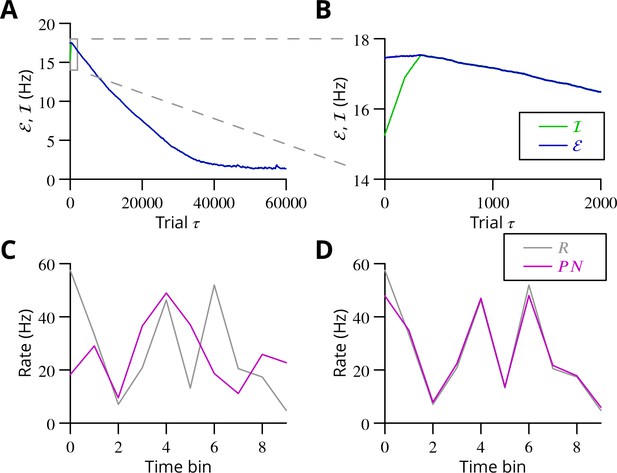
Simulated cerebellar learning by stochastic gradient descent with estimated global errors.
The total error (, blue) at the cerebellar nuclear output and the cancelling inhibition (, green) reaching the inferior olive are plotted as a function of trial number () in (A and B) for one of two interleaved patterns learnt in parallel. An approximately 10-fold reduction of error was obtained. It can be seen in A that the cancelling inhibition follows the error very closely over most of the learning time course. However, the zoom in B shows that there is no systematic reduction in error until the inhibition accurately cancels the mean error. (C) Initial firing profile of a typical cerebellar nuclear projection neurone (, magenta); the simulation represented 10 time bins with initially random frequency values per neurone, with a mean of 30 Hz. The target firing profile for the same neurone (, grey) is also plotted. (D) At the end of the simulation, the firing profile closely matched the target.
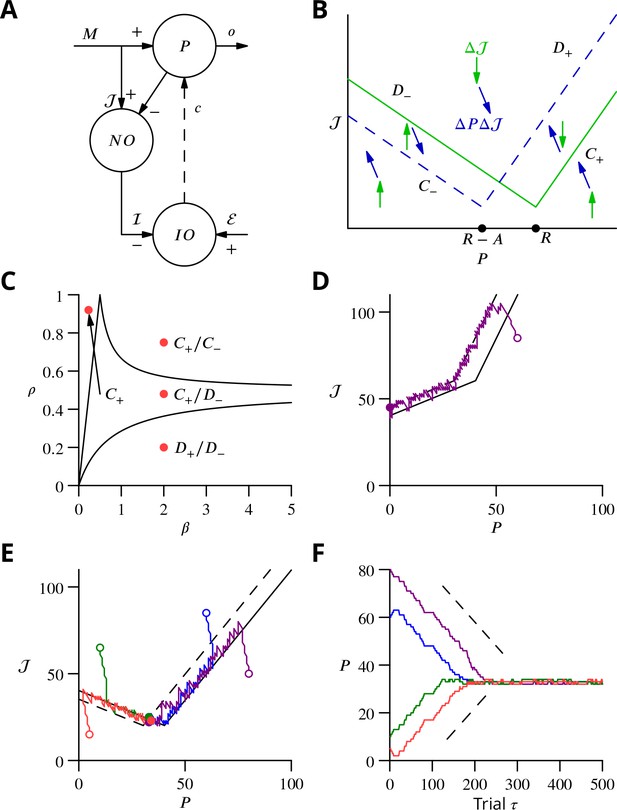
Single-cell convergence dynamics in a reduced version of the algorithm.
(A) Simplified circuitry implementing stochastic gradient descent with estimated global errors. Combined Purkinje cells/projection neurones (P) provide the output . These cells receive an excitatory plastic input from mossy fibres (). Mossy fibres also convey a plastic excitatory input to the nucleo-olivary neurones (NO), which receive inhibitory inputs from the Purkinje cells and supply the inhibitory input to the inferior olive (IO). The inferior olive receives an excitatory error input . The olivary neurones emit spikes that are transmitted to the P-cells via the climbing fibre . (B) Effects of plasticity on the simplified system in the plane defined by the P-cell rate (with optimum , perturbation ) and excitatory drive to the nucleo-olivary neurones . Plastic updates of type (blue arrows) and (green arrows) are shown. The updates change sign on the line C (dashed blue) and D (solid green), respectively. Lines and delimit the ‘convergence corridors’. The diagram is drawn for the case , in which , the perturbation of is larger than the perturbation of . (C) Parameters and determine along which lines the system converges to . The red dot in the region shows the parameter values used in panels E and F and also corresponds to the effective parameters of the perceptron simulation of Figure 10. The other red dots show the parameters used in the learning examples displayed in Appendix 1—figure 1. (D) When , and do not cross and learning does not converge to the desired rate. After going down the corridor, the point continues along the corridor without stopping close to the desired target rate . Open circle, start point; filled circle, end point. (E) Dynamics in the plane for and (). Trajectories (coloured lines) start from different initial conditions (open circles). Open circles, start points; filled circles, end points. (F) Time courses of as a function of trial for the trajectories in C (same colours). Dashed lines: predicted rate of convergence (Appendix 1). All trajectories end up fluctuating around , which is with the chosen parameters. Open circles, start points; filled circles, end points.
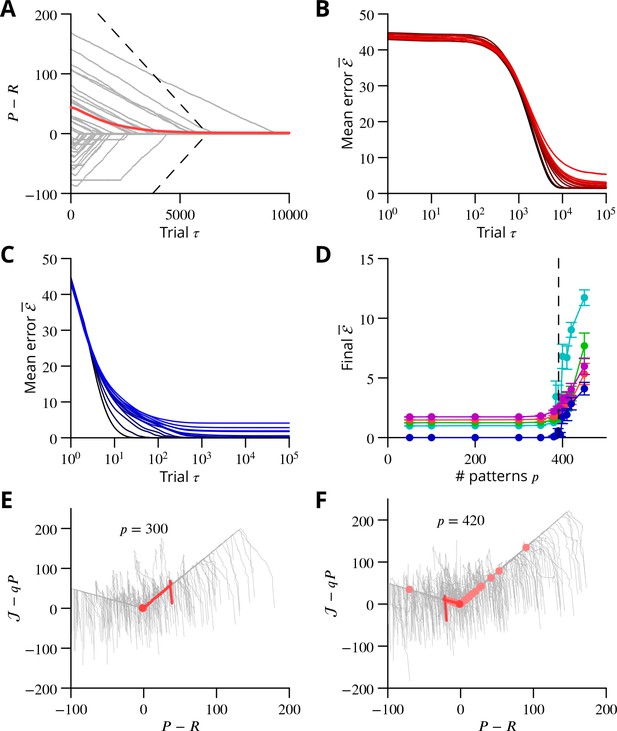
Convergence and capacity for an analog perceptron.
(A) Convergence for a single learned pattern for stochastic gradient descent with estimated global errors (SGDEGE). Different lines (thin grey lines) correspond to distinct simulations. The linear decrease of the error () predicted from the simplified model without zero-weight synapses is also shown (dashed lines). When learning increases the rate towards its target, the predicted convergence agrees well with the simulation. When learning decreases the rate towards its target, the predicted convergence is larger than observed because a fraction of synapses have zero weight. (B) Mean error vs. number of trials (always per pattern) for different numbers of patterns for SGDEGE with . Colours from black to red: 50, 100, 200, 300, 350, 380, 385, 390, 395, 400, 410, 420, 450. (C) Mean error vs. number of trials when learning using the delta rule. The final error is zero when the number of patterns is below capacity (predicted to be 391 for these parameters), up to finite size effects. Colours from black to blue: same numbers of patterns as listed for B. (D) Mean error after trials for the delta rule (blue) and for the SGDEGE with (cyan), 0.25 (green), 0.5 (red), 0.75 (magenta), as a function of the number of patterns . The mean error diverges from its minimal value close to the theoretical capacity (dashed line) for both learning algorithms. (E) Dynamics of pattern learning in the or more precisely plane below maximal capacity (). The error () corresponding to each pattern (grey) is reduced as it moves down its convergence corridor. The trajectory for a single specimen pattern is highlighted in red, while the endpoints of all trajectories are indicated by salmon filled circles but are all obscured at (0,0) by the red filled circle of the specimen trajectory. (F) Same as in E for a number of patterns above maximal capacity (). After learning several patterns, rates remain far from their targets (salmon filled circles). The SGDEGE algorithm parameters used to generate this figure are . The parameter except in panel D where it is explicitly varied. The analog perceptron parameters are and the threshold with ).
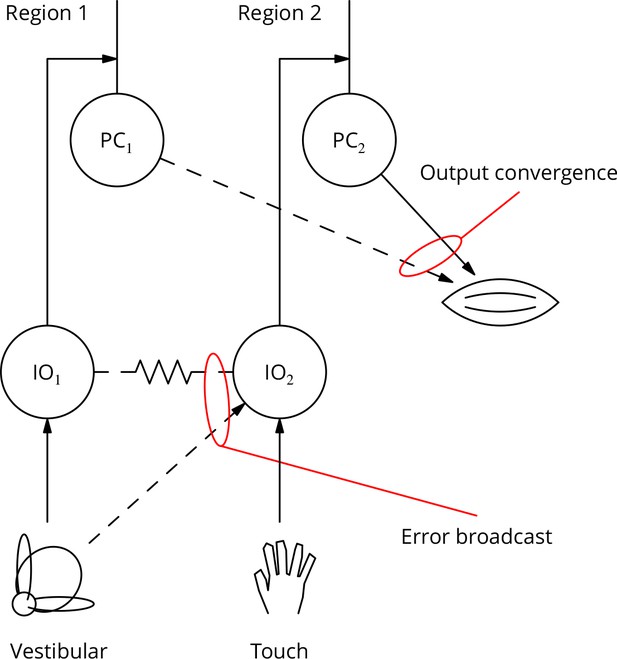
Diagram illustrating two possible solutions to the ‘bicycle problem’: how to use vestibular error information to guide learning of arm movements to ride a bicycle.
In the ‘output convergence’ solution, the outputs from cerebellar regions receiving different climbing fibre modalities converge onto a motor unit (represented by a muscle in the diagram). In the ‘error broadcast’ solution, error complex spikes are transmitted beyond their traditional receptive fields, either by divergent synaptic inputs and/or via the strong electrical coupling between inferior olivary neurones.
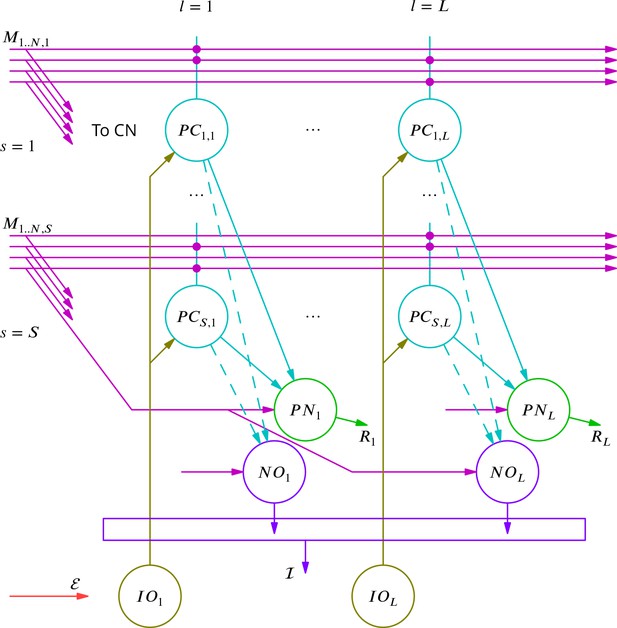
Diagram of the simulated network model.
The details are explained in the text.
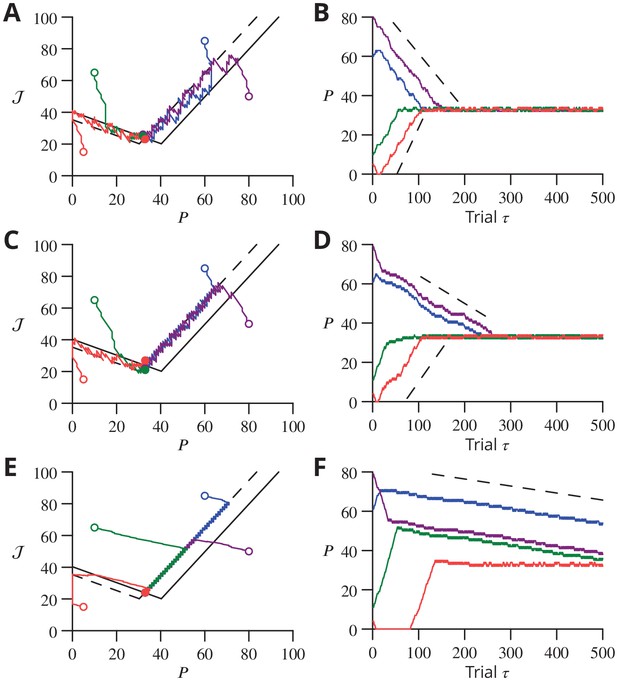
Reduced model: different cases of stochastic gradient learning are illustrated, for the parameters marked by solid red circles in Figure 9C, except for the case already shown in Figure 9E and F.
(A) Dynamics in the plane for and (). Trajectories (solid lines) from different initial conditions (open circles) are represented in the plane. The trajectories converge by oscillating around in the ‘corridor’ and around in the ‘corridor’. Trajectory endings are marked by filled circles. (B) Time courses of with corresponding colours. The slope of convergence (dotted lines) predicted by Equation 32 and 33 agrees well with the observed convergence rate for while it is less accurate for when the assumption that the ‘corridor’ width is much greater than and does not hold. (C, D) Same graphs for . The trajectories converge by oscillating around in the and around in the ‘corridor’. (E, F) Same graphs for and (i.e. ). The trajectories converge by oscillating around , which is the only attractive line.
Tables
Group data and statistical tests for plasticity outcomes.
In the upper half of the table, the ratios of EPSC amplitudes after/before induction are described and compared with a null hypothesis of no change (ratio = 1). The GP_ and GPE protocols both induced changes, while the control protocols (G_ _, G_E) did not. The bottom half of the table analyses differences of those ratios between protocols. The 95% confidence intervals (c.i.) were calculated using bootstrap methods, while the -values were calculated using a two-tailed Wilcoxon rank sum test. The p-values marked with an asterisk have been corrected for a two-stage analysis by a factor of 2 (Materials and methods).
Comparison | Mean | 95 % c.i. | |||
---|---|---|---|---|---|
GP_ | 1.40 | 1.26, | 1.72 | 0.0001 | 15 |
GPE | 0.53 | 0.45, | 0.63 | 0.002 | 10 |
G_ _ | 0.97 | 0.78, | 1.16 | 0.77 | 18 |
G_E | 0.98 | 0.79, | 1.24 | 0.60 | 15 |
GP_ vs G_ _ | 0.43 | 0.19, | 0.74 | 0.021* | |
GPE vs G_E | −0.44 | −0.72, | −0.24 | 0.0018* | |
GP_ vs G_E | 0.42 | 0.14, | 0.73 | 0.01* | |
GPE vs G_ _ | −0.43 | −0.65, | −0.22 | 0.008* | |
G_ _ vs G_E | 0.01 | −0.26, | 0.32 | 0.93 |
Parameters used in the simulation shown in Figure 12.
https://doi.org/10.7554/eLife.31599.016Parameter | Symbol | Value |
---|---|---|
Sagittal extent | 10 | |
Lateral extent | 40 | |
Time bins per movement | 10 | |
Mossy fibres per sagittal position | 2000 | |
Maximum firing rate (all neurones) | 300 Hz | |
pCS probability per cell per movement | 0.03 | |
Amplitude of Purkinje cell firing perturbation | 2 Hz | |
Learning rate of synapses | 0.02 | |
Learning rate of synapses | 0.0002 | |
Mean Purkinje cell firing rate | 50 Hz | |
Mean firing rate for nuclear neurones | 30 Hz | |
synaptic weight | 2.4 | |
synaptic weight | 0.06 | |
relative synaptic weight | 0.5 | |
Weight increment of drift (simple model) | 0.002 |
Additional files
-
Transparent reporting form
- https://doi.org/10.7554/eLife.31599.017