Offline replay supports planning in human reinforcement learning
Figures
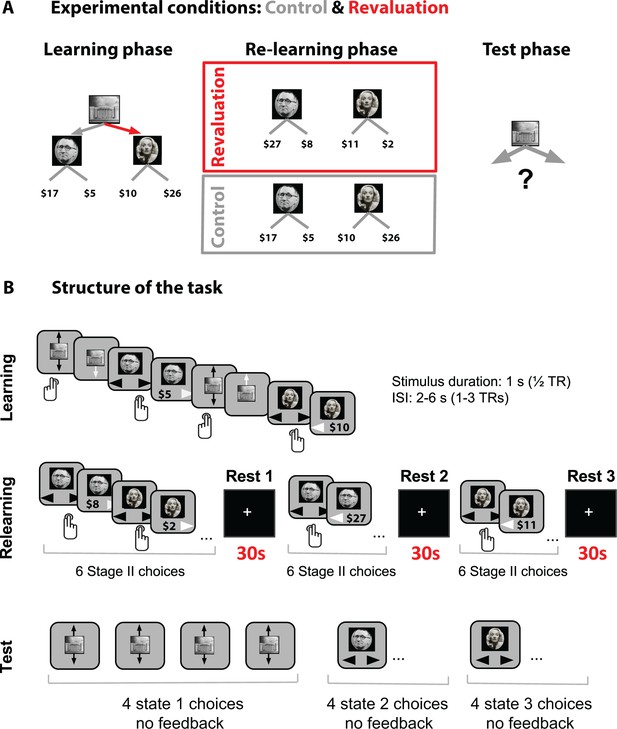
(A) Task design Each block had three phases.
During the Learning phase, participants explored a 2-stage environment via button presses to learn optimal reward policy. During the Relearning phase, they only explored the Stage II states: on revaluation trials the optimally rewarding Stage II state changed during the Relearning phase; on control blocks the mean rewards did not change. The final phase was the Test phase: participants were asked to choose an action from the starting (Stage I) state that would lead to maximal reward. The red arrow denotes the action that provides access to the highest reward in the learning phase. The optimal policy during the Learning phase remains optimal in the control condition but is the suboptimal choice in the revaluation condition. (B) The time course of an example block. Stimuli images were downloaded from the public domain via Google images.
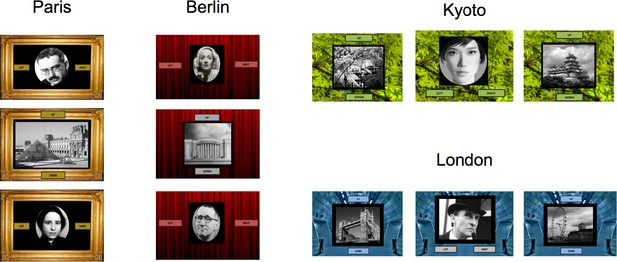
The four different blocks.
The cover story described each block, with its own Markov decision process (MDP), in terms of stealing money in a new building in a new city. For each participant, half of the cities were used in the revaluation condition and half in the control condition. In each of these conditions, one block had noiseless rewards (participants experienced the same reward each time they visited a state) and one block had noisy rewards (participants experienced the reward of a given state with variance around the fixed mean reward). Stimuli images were downloaded from the public domain via Google images.
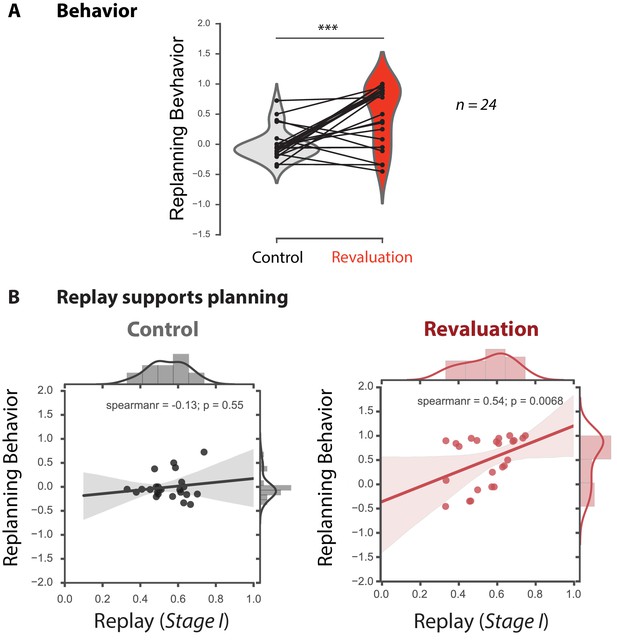
Offline replay of distal past states supports planning.
(A) Behavioral results (n = 24). Participants significantly changed their choice from the Learning phase to the Test phase in the revaluation condition, but not the control condition. (B) Replay supports planning. Correlation between Stage I replay during off-task rest periods and replanning magnitude during the subsequent Test phase for Control (left) and Revaluation (right) blocks. Stage I replay was operationalized as MVPA evidence for the Stage I stimulus category, in category-selective regions of interest, during all rest periods of control and revaluation conditions (n = 24). The correlation was significant in the Revaluation blocks (Spearman rho = 0.54, p = 0.0068) but not Control blocks (Spearman rho = −0.13, p = 0.55), and it was significantly larger in Revaluation than Control blocks (p = 0.0230, computed using a bootstrap, computing Spearman rho 1000 times with replacement). Regression lines are provided for visualization purposes, but statistics were done on Spearman rho values. The bottom figures display both fitted lines as well as Spearman’s rho values for clarity.
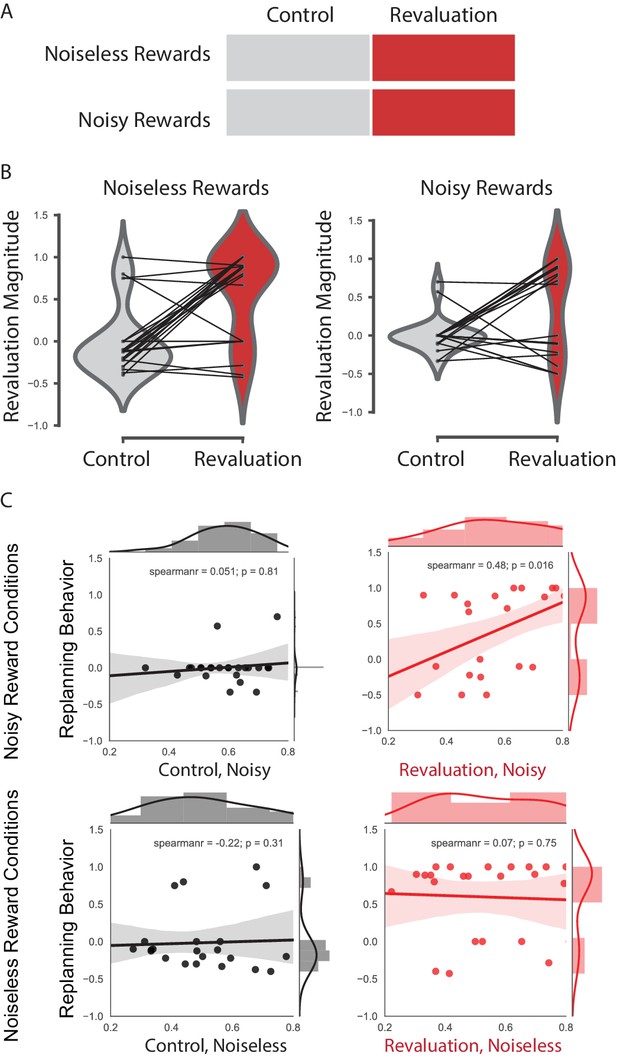
(A) Illustration of experimental conditions obtained by crossing revaluation and noise.
(B) Behavioral revaluation scores in the control and revaluation conditions, assessed separately in the noisy rewards vs. noiseless rewards conditions. Half of the trials in the control and revaluation conditions had fixed rewards (noiseless rewards condition) and half had noisy rewards (noisy rewards condition). We used an ANOVA to compare replanning behavior, evidenced by the revaluation magnitude, in the conditions with no variance in the rewards (noiseless condition) vs. conditions with noisy reward (noisy condition). Analysis of variance revealed a significant effect of the revaluation condition on behavior (F(1, 23) = 29.57, p < 0.0001) but no significant effect of the noise condition (F(1, 23) = 0.91, p = 0.34), and no significant interactions (F(1, 23) = 1.35, p = 0.25. Within each of the noise conditions (noiseless, noisy), participants significantly changed their choice from the Learning phase to the Test phase in the revaluation condition, but not the control condition: noiseless rewards condition: t(23) = 4.6, p = 0.00003; noisy rewards condition: t(23) = 3.06, p = 0.003. (C) Offline replay of distant past states predicts replanning. Breakdown of correlation between MVPA evidence for replay and subsequent replanning behavior, computed separately for the noisy and noiseless rewards conditions. All correlations were conducted using the last 10 TRs (out of 15 TRs) of each rest period, excluding the first 5 TRs to reduce residual effects of Stage II stimulus-presentation prior to rest. This breakdown revealed that MVPA evidence for Stage I replay was significantly correlated with subsequent replanning behavior in revaluation blocks in the noisy rewards condition (Spearman’s rho = 0.48, p = 0.016), but not the noiseless rewards condition (Spearman’s rho = 0.07, p = 0.75). We then ran bootstrap analyses to assess the differences in correlations between conditions. The difference in correlations between revaluation and control was trending but not significant in the noisy rewards condition (p = 0.066) and it was not significant in the noiseless condition (p = 0.16). There was no overall interaction between revaluation/control and noisy/noiseless; i.e., the revaluation vs. control difference was not significantly larger in the noisy condition than the noiseless condition (p = 0.34).
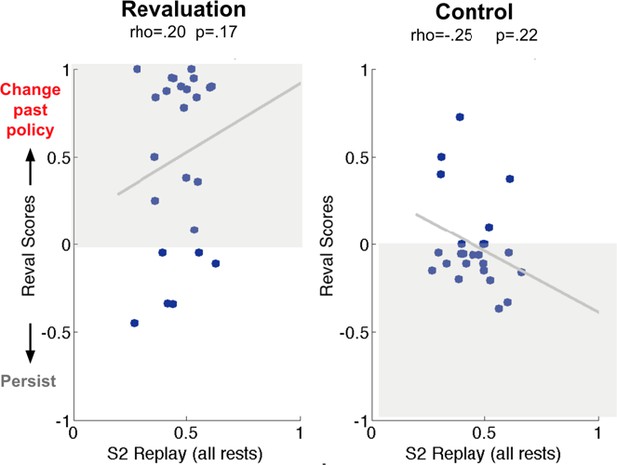
We tested whether Stage two replay was also correlated with subsequent planning (revaluation) behavior.
We found no significant correlation. The Figure shows the correlation between replay of Stage two category during rest and subsequent revaluation scores, which is non-significant for both conditions (revaluation: rho = 0.2, p = 0.17; control: rho = -0.25, p = 0.22). Multiple regression did not reveal any significant effect of Stage two replay (ß = 0.84, p = 0.44) but only a significant effect of the Stage one replay on the revaluation scores (ß = 1.89, p = 0.04).
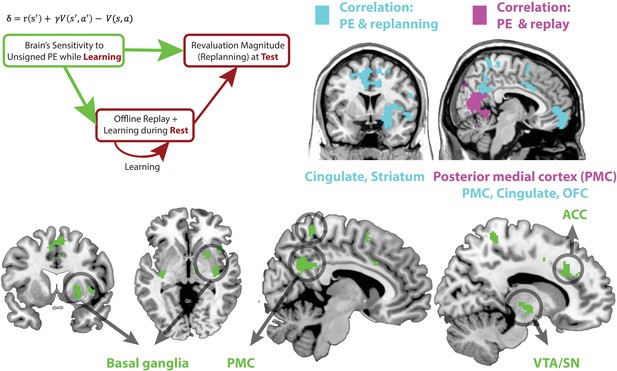
(Top left) Schematic of a theoretical account of revaluation.
We propose that neural sensitivity to reward prediction errors during learning ‘tags’ or ‘prioritizes’ memories for replay during later rest periods. Replay during rest, in turn, allows the comparison of past policy with new simulated policy and updating of the past policy when needed. (Top right) Regions where sensitivity to unsigned PE in Revaluation blocks correlates with subsequent replay during rest (extent threshold p < 0.005, cluster family-wise error [FWE] corrected, p < 0.05, shown in purple) and revaluation behavior (extent threshold p < 0.005, cluster FWE corrected, p < 0.05, shown in blue). (Bottom) Green reveals the conjunction of regions where sensitivity to unsigned PE in Revaluation blocks correlates with subsequent replay during rest (extent threshold p < 0.005, cluster FWE corrected, p < 0.05) and revaluation behavior (threshold p < 0.005, cluster FWE corrected, p < 0.05) in those blocks; the conjunction is shown at a p < 0.05 threshold. We found that the sensitivity of broad regions in the basal ganglia, the cingulate cortex (including the ACC), and the posterior medial cortex (precuneus) to unsigned prediction errors (signaling increase in uncertainty) correlated with both future replay during rest as well as subsequent revaluation behavior. See Tables 1 and 2 for coordinates.
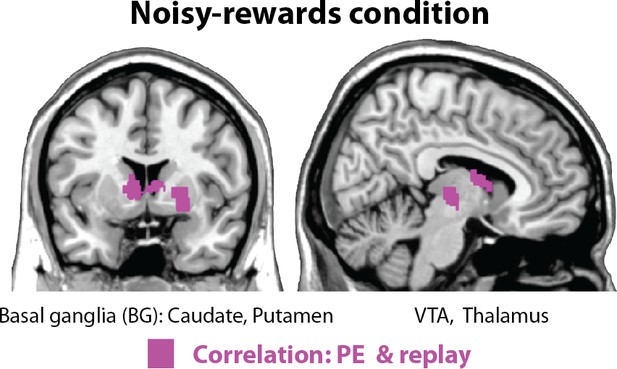
Correlation between the brain’s response to unsigned prediction errors and subsequent replay (purple), replanning behavior (blue), and their conjunction (green) in revaluation blocks, run separately for the noisy and noiseless conditions.
Results are shown based on extent threshold p < 0.005 and cluster (family wise error) correction at p < 0.05. The only one of these analyses to yield any significant clusters was the correlation between PE and replay in the noisy condition (shown below), in which the magnitude of sensitivity to unsigned PE in the ventral tegmental area (VTA), the thalamus, and the basal ganglia (including the caudate and putamen) predicted subsequent replay during rest periods.
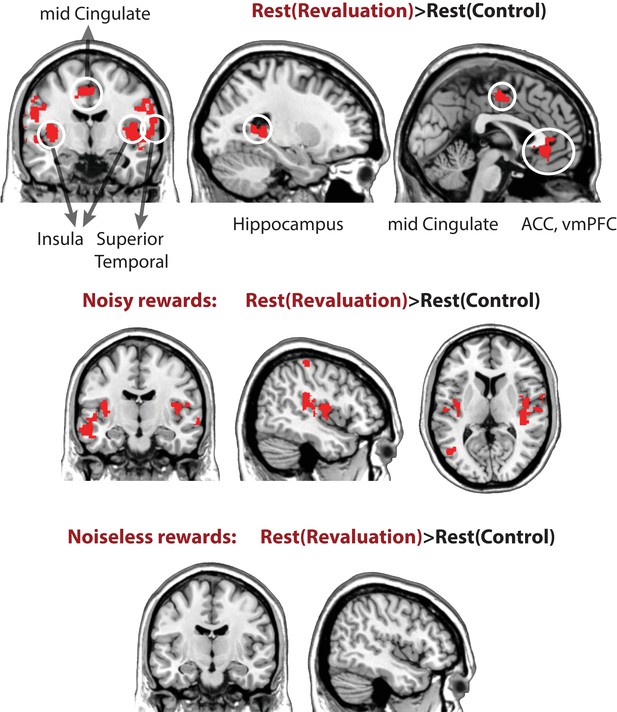
Univariate general linear model contrasts comparing activity rest periods of revaluation vs. control blocks, Restrevaluation> Restcontrol.
The contrast reveals higher activity in the hippocampus, the anterior cingulate cortex, mid cingulate (shown above), as well as bilateral insula and superior temporal cortices (extent threshold p < 0.005, cluster level family-wise error corrected at p < 0.05). See Table 3 for coordinates, cluster size, and p values.
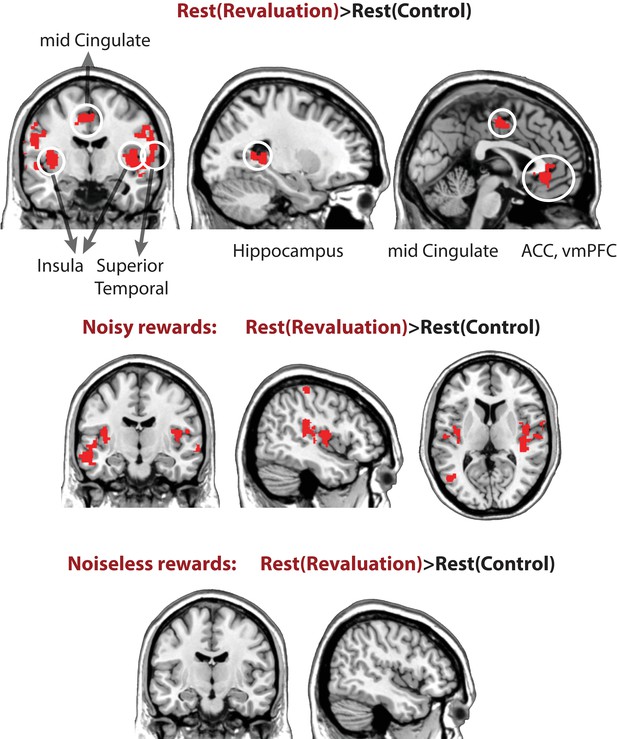
Differences in off-task univariate activation in revaluation vs. control.
We compared the difference between univariate activation during the rest periods of control vs. revaluation blocks in all runs (top), in the noisy-rewards condition (middle), and the noiseless-rewards condition (bottom). The Rest(revaluation) > Rest(control) contrast in the noisy rewards conditions reveals lateral temporal cortices and the insula, whereas the noiseless condition did not reveal any significant regions (extent threshold p < 0.005, cluster FWE corrected, p < 0.05).
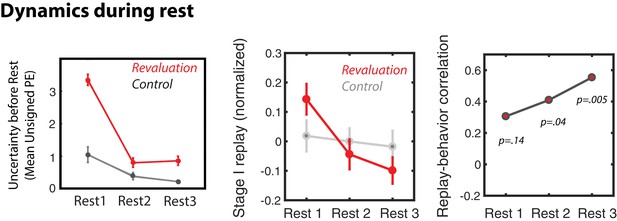
Dynamics of replay and activity during rest periods.
Dynamics of prediction errors prior to each rest period (left), MVPA evidence for Stage I replay during each rest period (middle), and replay-behavior correlation across the three rest periods of revaluation runs (right).
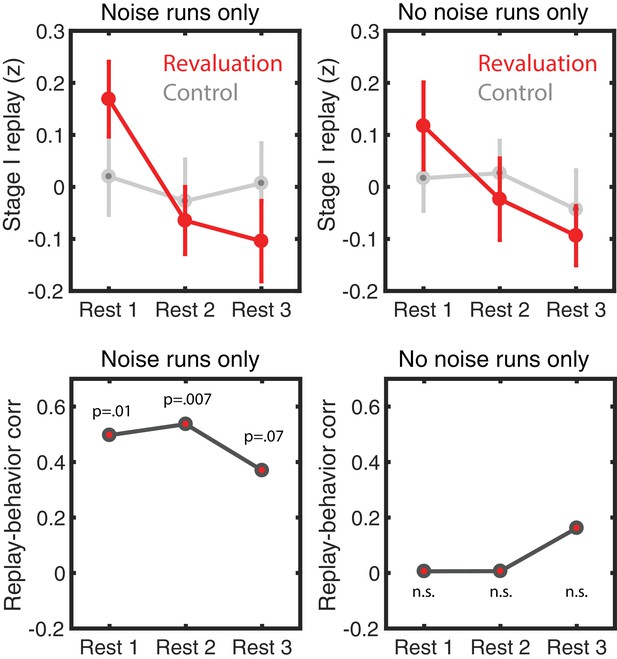
Breakdown of replay during the three rest periods in the revaluation vs. control conditions during noiseless rewards and noisy rewards conditions (top) and the correlation of these replay magnitudes with revaluation magnitude (bottom) in the noiseless vs. noisy rewards conditions.
https://doi.org/10.7554/eLife.32548.015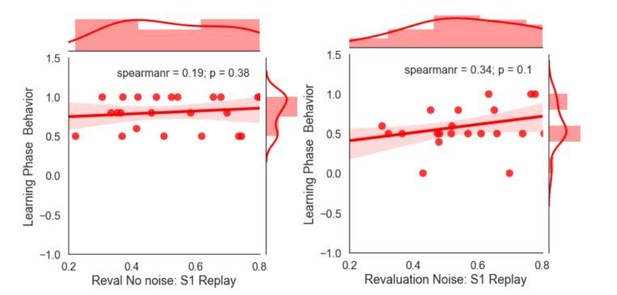
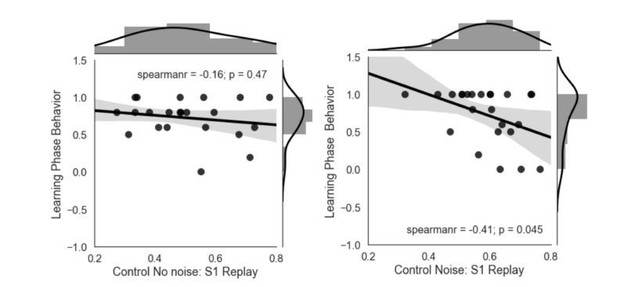
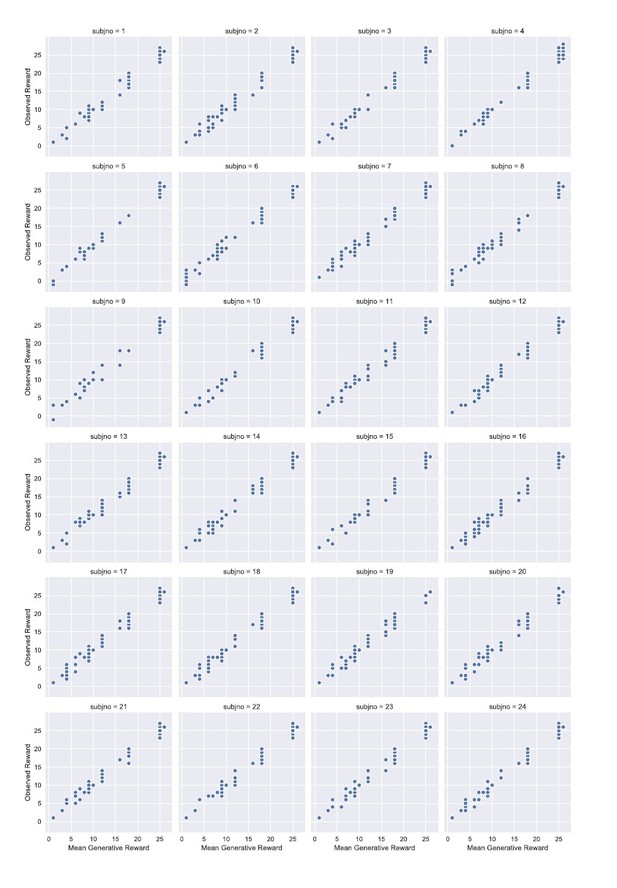
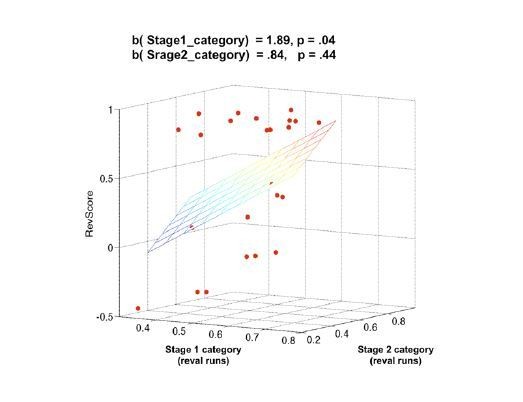
Tables
Coordinates of voxels where parametric modulation with unsigned PEs during learning predicted future replay of Stage I during rest periods, extent threshold p < 0.005, corrected at cluster level family-wise error p < 0.05 (these correspond to purple regions in Figure 3).
https://doi.org/10.7554/eLife.32548.009Region | Z score | X | Y | Z | K voxels | P |
---|---|---|---|---|---|---|
Right Cuneus Left Cuneus Right Lingual Left Lingual Calcarine | 3.31 3.29 3.21 3.15 3.14 | -2 4 −14 16 6 | −68 −66 −68 −46 −70 | 4 22 22 -8 4 | 1898 | .000488 |
Coordinates of voxels where parametric modulation with unsigned PEs during learning predicted future replanning behavior (revaluation magnitude), extent threshold p < 0.005, corrected at cluster level family-wise error p < 0.05 (these correspond to blue regions in Figure 3).
https://doi.org/10.7554/eLife.32548.010Region | Z score | X | Y | Z | K voxels | P |
---|---|---|---|---|---|---|
Right precentral Orbitofrontal cortex (OFC) Supplementary motor area Right superior frontal cortex ACC Right OFC | 3.67 3.62 3.59 3.49 3.49 3.42 | 26 0 -8 18 −14 6 | −20 58 4 32 50 58 | 68 −12 48 38 0 −18 | 5240 | 1.36e-08 |
Right superior temporal Right supra-marginal Inferior parietal Right putamen Right superior temporal pole | 3.67 3.6 3.58 3.39 3.35 | 56 34 36 26 46 | −30 −36 −42 8 18 | 14 44 44 2 −16 | 5165 | 1.67e-08 |
Left superior temporal pole Left putamen | 3.4 3.35 | −46 −20 | −10 18 | 0 8 | 2161 | .00021 |
.Coordinates of peak voxels of regions with higher off-task activity during rest periods of revaluation > control condition.
The clusters were selected with threshold p < 0.005, corrected at cluster level family-wise error p < 0.05 (these correspond to red regions in Figure 4).
Region (cluster) | Z score | X | Y | Z | K voxels | P |
---|---|---|---|---|---|---|
Left hippocampus | 4.74 | −20 | −38 | 10 | 297 | .014 |
Left insula Left superior temporal | 4.57 4.57 | −36 −42 | -8 −24 | 6 6 | 1959 | .000 |
Right superior temporal | 4.11 | 44 | −12 | 2 | 2249 | .000 |
Right anterior cingulate, ventromedial PFC | 4.65 | 2 | 38 | 6 | 238 | .048 |
Left mid cingulate Left Supplementary motor area | 3.31 3.33 | −10 -6 | −10 −12 | 46 62 | 258 | .030 |
Additional files
-
Transparent reporting form
- https://doi.org/10.7554/eLife.32548.016