Computational design of thermostabilizing point mutations for G protein-coupled receptors
Figures
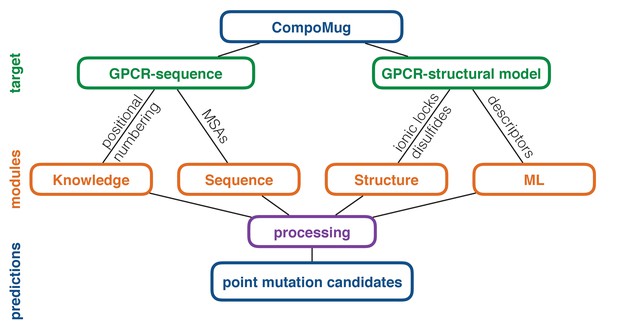
CompoMug architecture.
The method comprises four modules to predict stabilizing point mutations. The knowledge-based and sequence-based modules operate with only the sequence information about the target receptor, while the structure-based and machine-learning-based modules operate with the structural information. ML – machine learning; MSAs – multiple sequence alignments.
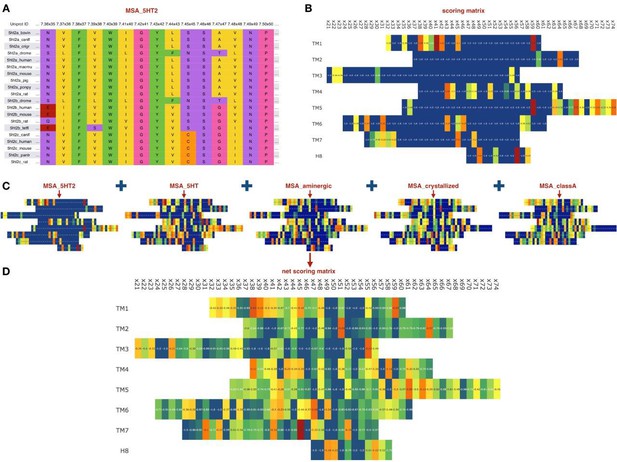
Sequence-based module.
(A) Example of an MSA for orthologs of 5HT2 receptors, residues colored according to their chemical properties. (B) Computed scoring matrix from the MSA_5HT2 in the sequence-based module (C) Example of the scoring matrices for five different MSAs. (D) Combined net scoring matrix. Each position is colored according to the score, from blue (minimal score) to red (maximal score).
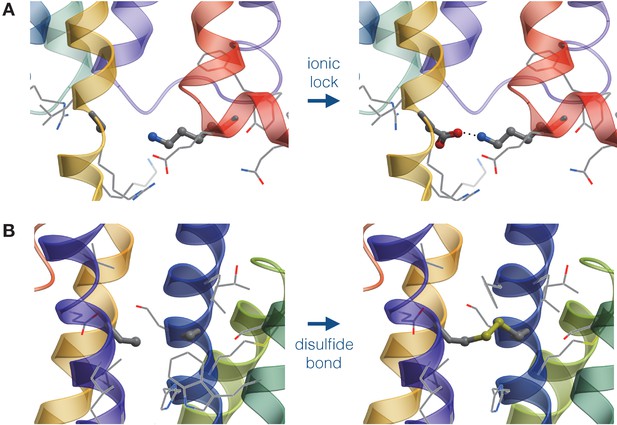
Schematic representation of mutations generated by the structure-based module.
(A) Design of an Asp-Lys ionic lock by the point mutation of an Ala residue. (B) Design of a disulfide bridge by the double mutation of Ala residues..
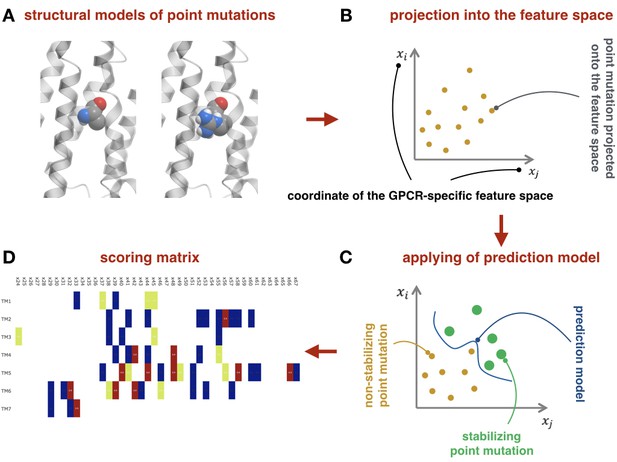
Machine-learning-based module.
(A) Example of structural models for the wild-type and mutant-type receptor. (B) Schematic representation of the point mutations mapped into the feature space. (C) Schematic representation of the prediction model as the separation curve in the feature space. (D) The net scoring matrix calculated with respect to the weights of the prediction models (blue and red colors correspond to the lowest and highest scores, respectively).
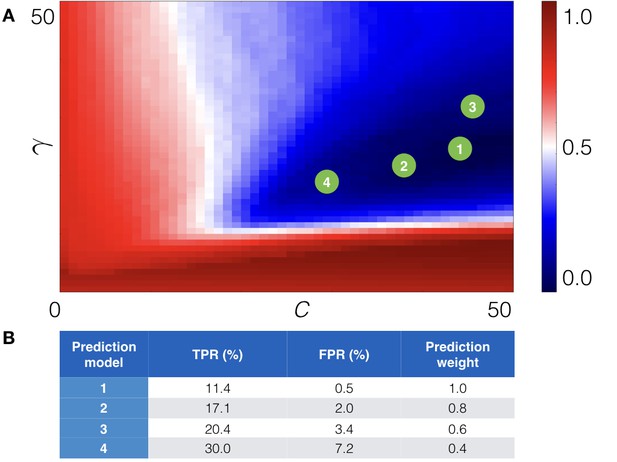
Cross-validation of the machine learning module.
(A) The cross-validation grid for the parameters C and . Pairs of C and of the top 4 prediction models are depicted with green numbered circles. (B) Estimated true (TPR) and false (FPR) positive rates for the derived prediction models along with prediction weight, which is added to score of a point mutation.
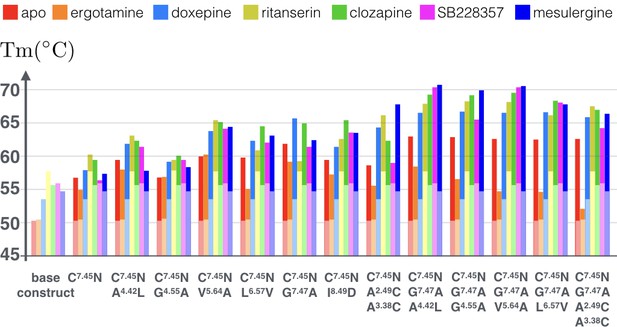
Apparent thermostability of 5-HT2C constructs with combined CompoMug mutations.
In apo form or in complex with an agonist (ergotamine) or antagonists (doxepin, ritanserin, clozapine, mesulergine, and SB228357). Light colored bars highlight the reference temperatures for the base construct, the full color bars show the additional effect of mutations on these complexes. The expected error for each measurement does not exceed 1.2°C.
-
Figure 6—source data 1
Data for apparent thermostability of 5-HT2C constructs with combined CompoMug mutations and in complexes with ligands, as shown in Figure 6 (estimated error <1.2°C).
- https://doi.org/10.7554/eLife.34729.011
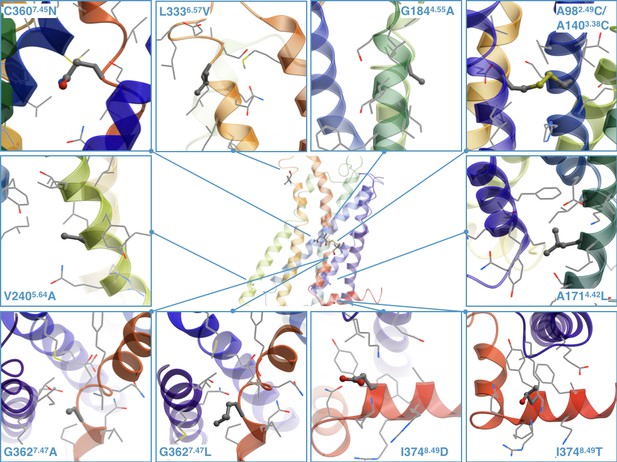
The stabilizing point mutations modeled in the structure of the ritanserin-bound 5-HT2C receptor (the ligand is not shown).
Each mutated residue and its neighboring residues are represented as sticks and wires, respectively.
Tables
Knowledge-based transferable point mutations in Class A.
https://doi.org/10.7554/eLife.34729.004Position | Mutation | Role | Receptor (PDB ID) |
---|---|---|---|
2.50 | D->N | Sodium pocket | AA2AR (5WF5) |
3.39 | S->A | Sodium pocket | AA2AR (5WF6) |
3.40 | I->V, A | P-I-F microswitch motif | ADRB1 (4BVN), APJ (5VBL) |
3.41 | X->W | stabilization of TM3, TM4, TM5 interface | 5HT2B (4IB4), 5HT1B (4IAR), ADRB1 (5A8E), ADRB2 (3NY8), CXCR4 (3ODU), DRD3 (3PBL) |
3.49 | D,G->A | DRY motif | FFAR1 (5TZR), NTR1 (4XES) |
5.58 | Y->A | Conserved activation microswitch | FFAR1 (5TZR), ADRB1 (4BVN) |
6.37 | L->A | Interferes with DRY motif function | AA2AR (5IU4), NTR1 (4GRV) |
7.49 | D->N | Sodium pocket | P2RY1 (4XNV), P2Y12 (4PXZ) |
-
X = any residue
Predicted CompoMug point mutations for 5-HT2C and results of experimental testing.
Mutations shown as bold improved aSEC and/or thermostability by more than 1.5 oC ; shown as italic had low protein yield or strong aggregation, Tm not measured.
Mutation | CompoMug module | aSEC* quality | Tm (oC) ± SEM | ΔTm (C) |
---|---|---|---|---|
WT | 50.4 ± 0.8 | 0.0 | ||
I621.41V | Sequence-based | ~ | −0.7 | |
G691.48A | Sequence-based | - | −1.4 | |
D992.50N | Knowledge-based | - | - | |
H8512.51E | Structure-based | N/A | - | |
G1032.54A | Sequence-based | - | −4.4 | |
Y1253.23K | Sequence-based | - | −2.0 | |
Y1253.23V | Sequence-based | ~ | −0.7 | |
M1433.41W | Knowledge-based | - | 0.6 | |
R1573.55T | Machine-learning and Sequence-based | - | −1.8 | |
R1573.55Q | Sequence-based | - | −2.0 | |
T1694.40K | Sequence-based | + | 0.2 | |
A1714.42L | Machine-learning | ~ | 52.3 ± 1.2 | 1.9 |
I1724.43A | Sequence-based | - | 1.1 | |
I1724.43F | Sequence-based | ~ | 0.6 | |
G1844.55A | Machine-learning | + | 51.9 ± 0.1 | 1.5 |
N203ECL2D | Structure-based | - | −2.6 | |
F2205.45I | Machine-learning | ~ | 0.0 | |
F2245.48Y | Machine-learning and Sequence-based | - | −3.3 | |
C2355.59F | Sequence-based | ~ | 0.1 | |
L2365.60R | Machine-learning and Sequence-based | N/A | - | |
V2405.64A | Sequence-based | + | 52.4 ± 0.5 | 2.0 |
V2405.64S | Sequence-based | + | 0.3 | |
G3146.38A | Machine-learning-based | - | −4.0 | |
L3336.57V | Machine-learning and Sequence-based | + | 53.7 ± 0.6 | 3.3 |
K3487.32A | Sequence-based | - | −4.4 | |
C3607.45N | Sequence-based | + | 59.2 ± 0.5 | 8.8 |
G3627.47L | Sequence-based | + | 52.3 ± 0.7 | 1.9 |
G3627.47A | Sequence-based | + | 54.3 ± 0.7 | 3.9 |
L3707.55D | Structure-based | - | −2.3 | |
K3738.48E | Structure-based | - | −0.4 | |
I3748.49D | Structure-based | + | 53.9 ± 0.8 | 3.5 |
I3748.49T | Sequence-based | + | 54.1 ± 0.9 | 3.7 |
Y3758.50F | Sequence-based | - | −2.4 | |
N3818.56R | Sequence-based | ~ | 0.6 | |
T671.46C/G1032.54C | Structure-based | - | - | |
V741.53C/A962.47C | Structure-based | - | - | |
A872.38C/A1714.42C | Structure-based | ~ | - | |
A982.49C/A1403.38C | Structure-based | ~ | 52.8 ± 1.0 | 2.4 |
T3697.54C/Y3758.50C | Structure-based | N/A | - |
-
*aSEC quality is denoted as improved (+), unchanged (~), and degraded (-) as compared to the base construct apo receptor.
Additional files
-
Supplementary file 1
Top 40 CompoMug predictions for the three benchmark receptors.
The true hits from the corresponding studies are highlighted green
- https://doi.org/10.7554/eLife.34729.013
-
Supplementary file 2
List of sequences used to construct five MSAs for 5HT2C in the Sequence-based module.
- https://doi.org/10.7554/eLife.34729.014
-
Supplementary file 3
Key resources table.
- https://doi.org/10.7554/eLife.34729.015
-
Transparent reporting form
- https://doi.org/10.7554/eLife.34729.016