Removing physiological motion from intravital and clinical functional imaging data
Figures
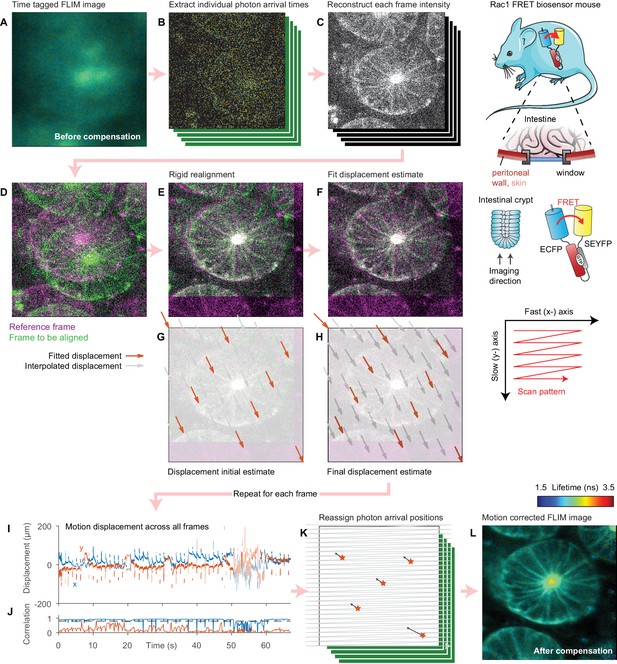
Illustration of motion correction procedure.
(A) Intensity merged FLIM image of intestinal crypt acquired in vivo using a titanium optical window from a Rac1 FRET biosensor mouse, composed of 443 separate frames. (B) As the data are acquired in a time-tagged mode, the arrival time and position of each photon in the dataset can be extracted. (C) Using these data, the intensity of each frame can be reconstructed. (D) One frame is selected as the reference (magenta). To realign subsequent frames (example shown in green), (E) a rigid realignment step is first performed, estimating the coarse offset between the images. (F) To estimate the motion during the frame leading the residual difference between the two images, we select a number of points equally spaced in time during the frame scan and estimate the displacement of the sample at each point (red arrows). (G) We use the rigid realignment as the initial estimate for the displacement. (H) As the fit progresses, the displacement estimates reduce the difference between the two images. The displacement of pixels between each point are computed using linear interpolation (grey arrows). The (I) displacement during each frame and (J) correlation between each frame and the reference is computed by repeating this process for each frame. (K) The FLIM image is reprocessed and each photon arrival is reassigned to its estimated origin point on the sample using the interpolated displacements. (L) The final intensity merged FLIM image can then be analyzed using conventional approaches. Mouse and intestine illustrations were adapted from Servier Medial Art, licensed under the Creative Commons Attribution 3.0 Unported license.
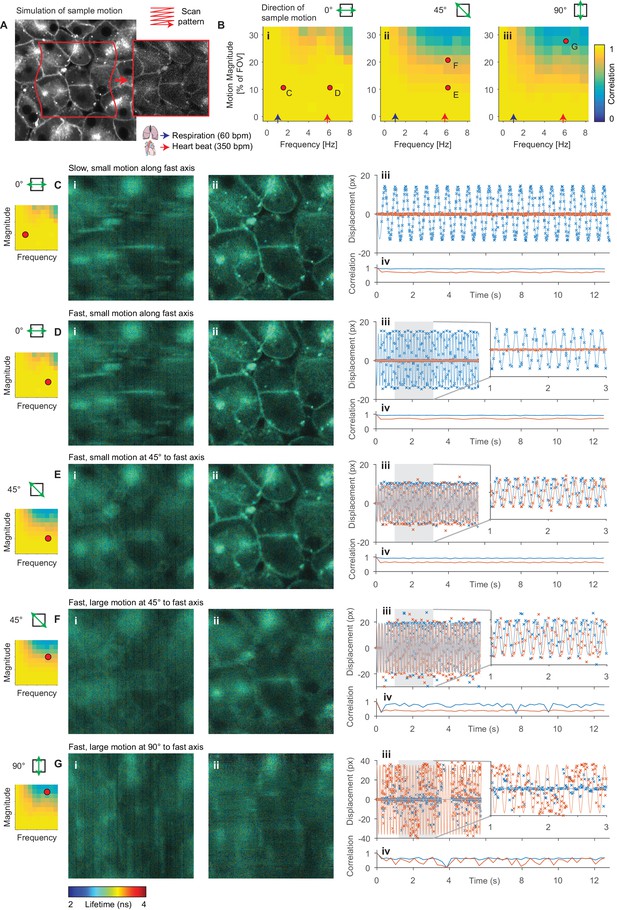
Evaluation of motion correction performance with simulated data.
(A) Illustration of generation of a frame in a simulated TTTR dataset. Main image shows high SNR intensity image used as the reference sample intensity. Red lines indicate the sample motion during the illustrated frame. Inset image shows the resultant simulated frame. (B) Average correlation coefficient over a range of magnitudes and frequencies of sinusoidal sample motion along an axis (i) 0°, (ii) 45° and (iii) 90°, respectively, from the fast axis. For comparison, indicative average values for the heart rate (red arrow, 350 bpm, 5.8 Hz) and respiration rate (blue arrow, 60 bpm, 1 Hz) of an adult mouse anaesthetized under ~1% isoflurane are shown. Black dots indicate results illustrated in the following panels. (C–G) Exemplar simulation results showing intensity merged lifetime images (i) without and (ii) with motion compensation. (iii) Estimated displacement traces in (blue) x and (red) y directions over time. In D-Giii, inset panels show expanded views of displacements between 1 and 3 s. (iv) Correlation between reference frame and (red) uncorrected and (blue) corrected images over time. Examples shown in C–E were corrected successfully, while the motion in examples F and G was too large to effectively compensate. (C) Motion parallel to fast axis with frequency 1.5 Hz and magnitude 10% of FOV. (D) Motion parallel to fast axis with frequency 6 Hz and magnitude 10% of FOV. (E) Motion at 45° to fast axis with frequency 6 Hz and magnitude 10% of FOV. (F) Motion at 45° to fast axis with frequency 6 Hz and magnitude 20% of FOV. (G) Motion at 90° to fast axis with frequency 6 Hz and magnitude 28% of FOV.
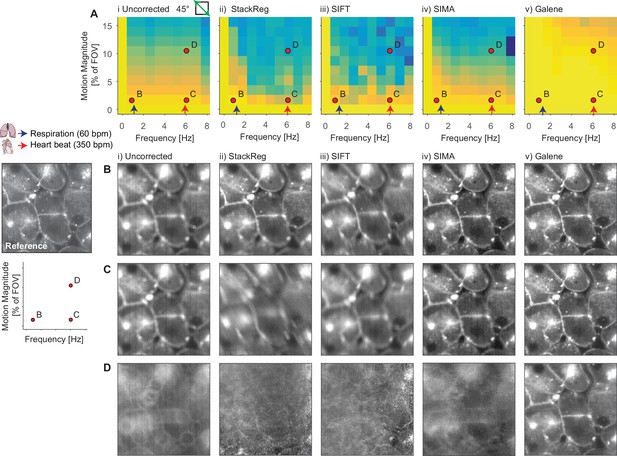
Benchmarking of intensity-only motion correction performance with simulated data.
Intensity time-series data were generated by temporal averaging of the simulated data with motion at 45° to the fast-axis used in Figure 2. The data were motion corrected using a panel of open source packages. (A) Average correlation coefficient over a range of magnitudes and frequencies of sinusoidal sample using (i) no correction, (ii) ImageJ plugin StackReg, (iii) ImageJ plugin ‘Linear Stack Alignment with SIFT,’ (iv) python package SIMA and (v) Galene. For comparison, indicative average values for the heart rate (red arrow, 350 bpm, 5.8 Hz) and respiration rate (blue arrow, 60 bpm, 1 Hz) of an adult mouse anaesthetized under ~1% isoflurane are shown. Red dots indicate results illustrated in the following panels (B-D) Exemplar simulation results showing frame-averaged motion correction results for a number of simulation conditions (marked by red dots in (A)). The examples represents approximately (B) a small motion due to respiration, (C) a small motion due to the heart beat and (D) a larger motion relative to the FOV due to the heartbeat (e.g. zoomed in on a small region or experiencing a large displacement).
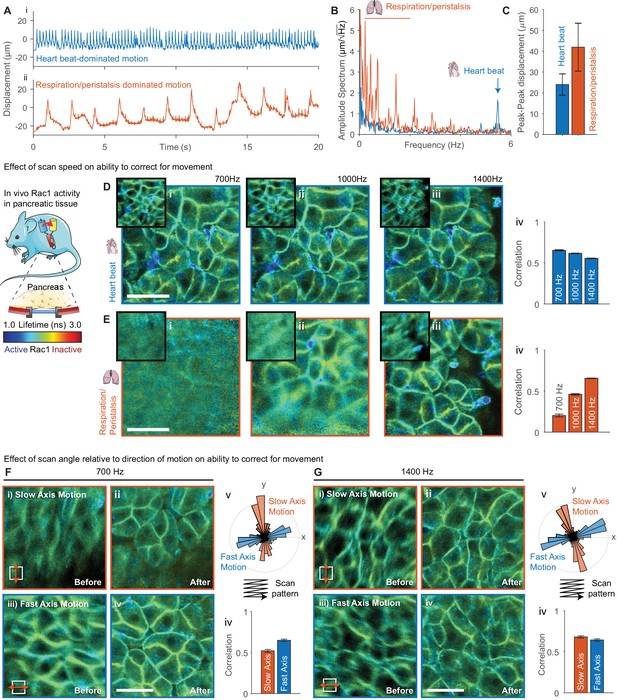
Evaluation of effect of scan speed and angle on motion correction performance.
(A) Imaging of pancreatic tissue in vivo in a Rac1 FRET biosensor mouse using an abdominal titanium imaging window. (A) Estimated displacement for first 20 s of motion (i) dominated by heartbeat and (ii) dominated by respiration and peristalsis recorded at 1400 Hz. (B) Amplitude spectrum of displacements for two data series showing dominant contributions from the heartbeat (~5.6 Hz) and respiration and peristalsis (0.5–2.5 Hz). (C) Peak-to-peak displacements for the data series. Error bars show standard deviation over series. (D,E) Motion corrected FLIM images acquired in (D) heartbeat dominated regime and (E) respiration dominated regime at (i) 700 Hz, (ii) 1000 Hz and (iii) 1400 Hz, (iv) average correlation of corrected frames over series. (F,G) FLIM data were acquired with predominant motion occurring along the slow scan axis (red, top-bottom) and fast scan axis (blue, left-right) at (F) 700 Hz and (G) 1400 Hz. FLIM images are shown (i) before and (ii) after motion correction for motion along the slow axis and (iii) before and (iv) after motion correction for motion along the fast axis. (v) Angular histogram of displacements for data acquired along the slow and fast axis. (iv) Average correlation of data acquired with motion aligned along the slow and fast axis. White scale bars 100 μm. Mouse and pancreas illustrations were adapted from Servier Medial Art, licensed under the Creative Commons Attribution 3.0 Unported license.
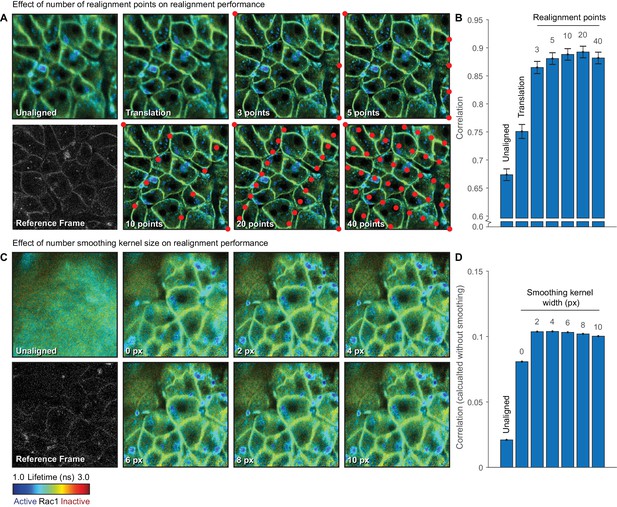
Evaluating the effect of realignment parameters on motion correction performance.
FLIM images were acquired of the pancreas in a Rac1-FRET biosensor mouse through an optical window. (A) FLIM images without realignment, realignment using only translation information from phase-correlation and realignment with 3, 5, 10, 20 and 40 realignment points are shown alongside the reference frame. Red dots indicate the position of the realignment points within the frame. (B) Quantification of average correlation between each corrected frame and the reference frame for each realignment condition. (C) FLIM images without realignment and realignment with a smoothing kernel of width 0, 2, 4, 6, 8 and 10 pixels in the x-axis are shown alongside the reference frame. (D) Quantification of average correlation between each corrected frame and the reference frame for each realignment condition, computed without smoothing. Results show mean ± SEM, calculated per time point.
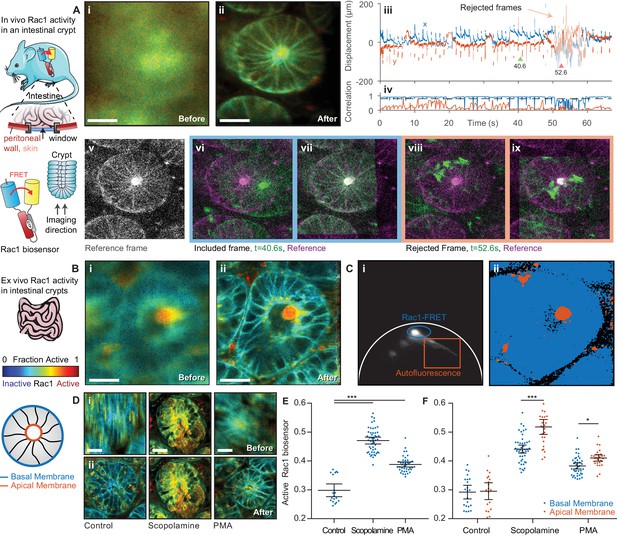
FLIM of intestinal crypts from the Rac1-FRET biosensor mouse in vivo and ex vivo using motion compensation.
(A) Imaging of intestinal crypts in vivo using an abdominal titanium imaging window (i–ii) Example FRET biosensor activity maps (i) before and (ii) after motion compensation showing fraction of active FRET biosensor determined by fitting to a FRET model accounting for the complex exponential decay of ECFP. White scale bars, 50 μm. (iii) Estimated displacement traces in (blue) x and (red) y directions over time. Pastel shaded regions indicate frames that could not be successfully corrected with correlation coefficients < 0.8. (iv) Correlation between reference frame and (red) uncorrected and (blue) corrected images over time. Black dashed line denotes threshold (0.8) used to reject frames which could not be corrected. (v) Selected reference frame (vi,vii) Example of a successfully corrected frame (vi) before and (vii) after correction. (viii,ix) Example of a frame that could not be corrected. (B) Imaging of intestinal crypts ex vivo. (i–iv) as (A), no correlation threshold applied. (C) (i) Phasor plot of image shown in (B) to separate biosensor fluorescence (blue) from autofluorescence (red) and i) back projection of selected gates. (D) Intensity merged lifetime images of crypts (i) before and (ii) after motion compensation treated with (left-right) no drug, 200 nM PMA or 1 μM scopolamine. White scale bars 50 μm. (E) Quantification of fraction of active biosensor in crypts after drug treatment. (F) Subcellular analysis of fraction of active biosensor in basal (blue) and apical (red) membranes after drug treatment, shown per cell. Error bars show means ± SEM. **p<0.01; ***p<0.001 using one-way ANOVA. Mouse and intestine illustrations were adapted from Servier Medial Art, licensed under the Creative Commons Attribution 3.0 Unported license.
-
Figure 4—source data 1
Source data for graphs show in Figure 4E and F.
(Sheet 1) Fraction of active Rac1-FRET biosensor in each cell. (Sheet 2) Fraction of active Rac1-FRET biosensor in the basal and apical membrane respectively per cell.
- https://doi.org/10.7554/eLife.35800.012
-
Figure 4—source data 2
Source data for graph show in Figure 4—figure supplement 1C, showing (Sheet 1) average optical densities for active-Rac1 IHC staining per mouse and (Sheet 2) individual optical densities for active Rac1 IHC staining per cell for each mouse.
- https://doi.org/10.7554/eLife.35800.013
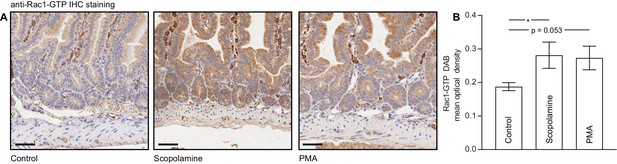
IHC for Rac1-GTP in intestinal crypts.
Freshly collected intestinal crypts were treated with a vehicle, 1 μM Scopolamine for 30 min or 200 nM PMA for 15 min and stained for Rac1-GTP. (A) Example IHC images, black scale bar, 50 μm. (B) Quantification of staining optical density in intestinal crypt cells, averaged over n > 1,500 cells per condition. Results show mean ± SEM (shaded) over n = 3 mice. p values were determined per-mouse using non-parametric one way ANOVA; *p<0.05.
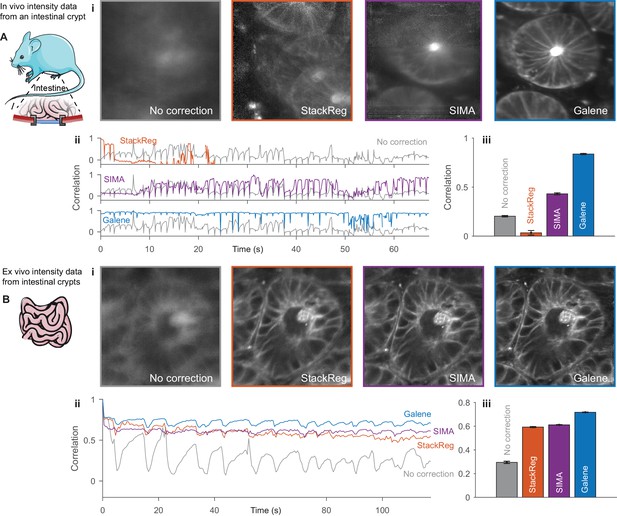
Benchmarking of intensity-only motion correction performance with frames from intestinal crypts.
Intensity frames were extracted from the FLIM images of intestinal crypts shown in Figure 4 imaged (A) in vivo through an optical window and (B) ex vivo for benchmarking against alternative motion correction packages. (i) Frame averaged motion correction results with (gray) no correction, (red) ImageJ plugin StackReg, (purple) python package SIMA and (blue) Galene. No frames were excluded from the averaging. (ii) Correlation between each frame in the sequence and the reference frame for each package. (iii) Average correlation for each correction package. Results show mean ± SEM. Mouse and organ illustrations were adapted from Servier Medial Art, licensed under the Creative Commons Attribution 3.0 Unported license.
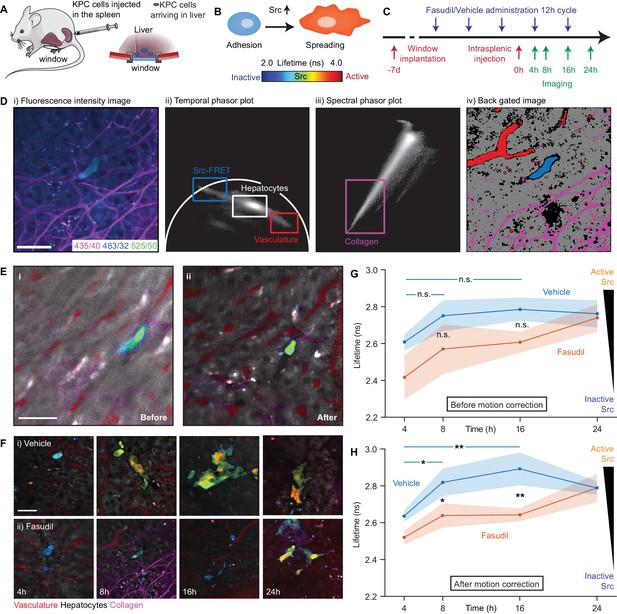
Longitudinal imaging of Src activity in cancer cells colonising the liver in an intrasplenic model of pancreatic cancer metastasis with micro-environmental context in response to priming with Fasudil.
(A) Cartoon of intrasplenic model of pancreatic cancer using KPC cancer cells expressing a Src-FRET biosensor. (B) Cartoon of morphology of KPC cells during early attachment events. (C) Experimental timeline showing timings of window implantation, intrasplenic injection, Fasudil (or vehicle) administration and imaging. (D) Illustration of identification of fluorescence components; (i) merged spectral intensity image, (ii) temporal phasor plot of 525/50 nm channel with gates used to select fluorescence components, (iii) spectral phasor plot, (iv) back projection image showing gates highlighted in (ii) and (iii). (E) Merged Src-FRET biosensor lifetime and hyperspectral unmixing image i before and (ii) after motion correction acquired 8 hr after intrasplenic injection. Cancer cells expressing the Src biosensor are colour-coded using the rainbow lifetime scale. Autofluorescence contributions obtained using hyperspectral unmixing shown in (red) vasculature, (grey) hepatocytes and (magenta) collagen. (iii) Estimated displacement traces in (blue) x and (red) y directions over time. (iv) Correlation between reference frame and (red) uncorrected and (blue) corrected images over time. (F) (i,ii) Example images showing Src activity and micro-environmental context in mice treated with (i) vehicle and (ii) 100 mg/kg Fasudil according to the timeline show in (C) at 4, 8, 16 and 24 hr after intrasplenic injection respectively. (G,H) Average Src biosensor lifetime in cancer cells colonising the liver in response to Fasudil treatment, using images (G) before and (H) after motion correction. n = 3 mice per condition, 20–45 cells per time point. Results show means ± SEM (shaded). p values were determined per-mouse by unpaired t-test, *p<0.05; **p<0.01. Mouse and liver illustrations were adapted from Servier Medial Art, licensed under the Creative Commons Attribution 3.0 Unported license.
-
Figure 5—source data 1
Source data for graphs show in Figure 5H and G, showing average Src-FRET biosensor lifetimes at 4, 8, 16 and 24 hr after intrasplenic injection per mouse, (Sheet 1) before motion correction and (Sheet 2) after motion correction.
Values are given in picoseconds.
- https://doi.org/10.7554/eLife.35800.017
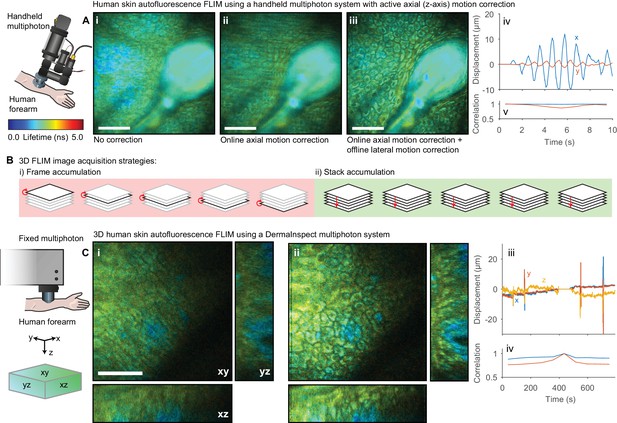
Motion correction of autofluorescence imaging of human skin in vivo.
(A) Example intensity merged FLIM image of human skin around a hair follicle on a handheld multiphoton microscope with an active axial motion correction system, (i) without motion compensation, (ii) with axial motion correction and (iii) with axial and lateral motion compensation. White scale bars 50 μm. (iv) Estimated displacement traces in (blue) x and (red) y directions over time. Correlation between reference frame and (red) uncorrected and (blue) corrected images over time. (B) Cartoon illustration of (i) conventional frame-accumulation 3D stack acquisition strategy where frames from each slice in the volume are accumulated in turn and (ii) stack based accumulation where multiple acquisition of the entire stack recorded in a single pass are acquired, enabling motion correction in three dimensions. (C) Example intensity merged orthogonal projection of a 3D volume of human skin acquired on a commercial DermaInspect multiphoton microscope (i) before and (ii) after motion correction showing xy, yz, and xz sections through the volume. White scale bars 100 μm. (iv) Estimated displacement traces in (blue) x and (red) y directions over time. (v) Correlation between reference frame and (red) uncorrected and (blue) corrected images over time. Data shown in (A) were acquired at Imperial College, London (Sherlock et al., 2018) and reanalysed with kind permission under the Creative Commons Attribution 4.0 International licence. Mouse and liver illustrations were adapted from Servier Medial Art, licensed under the Creative Commons Attribution 3.0 Unported license.
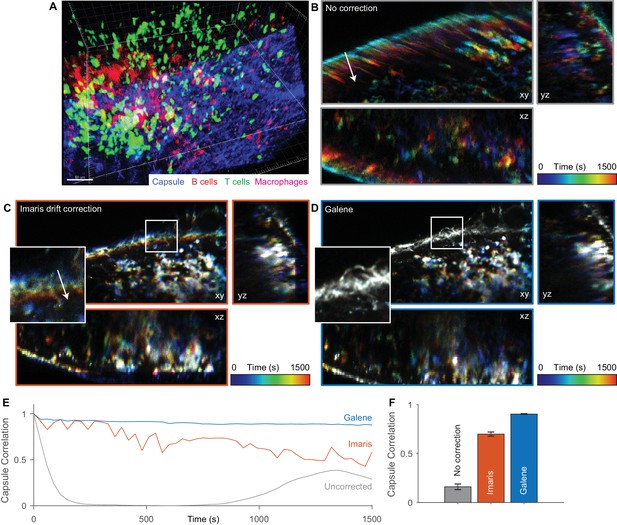
Motion correction of multispectral 3D imaging of labelled immune cells in a murine lymph node.
tdTomato labelled B cells (red), Kaede labelled OT2 T cells (green) and subcapsular sinus macrophages labelled with Alexa 680 (magenta) imaged in a 150 μm z-stack through the inguinal lymph node (SHG signal from fibrillar capsule, blue) over 30 min. (A) One time point rendered volumetrically. (B–D) Temporally colour-coded (blue, early time points; red, late time points) orthogonal projections of time series (with spectral channels merged) with (B) no correction, (C) Imaris drift correction and (D) motion correction with Galene. Inset, expanded view of region highlighted in white. (E) Correlation between the stationary collagen and macrophage signal for each volume in the sequence and the reference volume for each package. (F) Average correlation for each correction package. Results show mean ± SEM, calculated per time point.
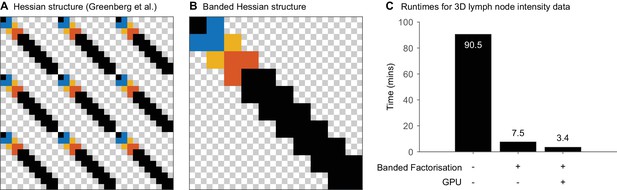
Algorithmic optimisation for realignment of three dimensional data.
(A,B) Structure of Hessian matrix used during optimisaton in the Lucas–Kanade framework in the parameter formulation used by (A) Greenberg and Kerr (2009) and (B) in this work for the 3D (x, y, z) case with 10 realignment points in total. Grey and white checkerbox indicate elements of the Hessian Matrix. Blue squares indicate matrix elements associated with the second realignment position, red squares indicate matrix elements associated with the third realignment position and yellow squares indicate matrix elements associated with both the second and third realignment position. (C) Time required for realignment of the 512 × 512 × 51 3D intensity data shown in Figure 7 using 15 realignment points per z position (2295 parameters in total) and 51 time points using (i) conventional factorisation, (iii) banded factorisation and (iii) banded factorisation with GPU computation of the error function and Jacobian.
Videos
Video abstract.
Overview of motion correction using Galene.
Motion correction of Rac1 biosensor FRET of an intestinal crypt imaged in vivo through an optical window.
Associated with Figure 3.
Motion correction of Rac1 biosensor FRET in an intestinal crypt stimulated with PMA imaged ex vivo.
Associated with Figure 3.
Motion correction of KPC Src-FRET biosensor cells and liver autofluorescence imaged in an intrasplenic experiment through optical window.
Associated with Figure 5D. (top left) Each intensity frame from the FLIM acquisition (green) superimposed on the reference frame (magenta). (top right) The motion compensated frames (green) superimposed on the reference frame (magenta); frames which have been excluded due to a poor correlation are marked as such. (bottom left) Cumulative intensity merged FLIM lifetime image without motion correction, estimated using the first moment of the decay. (bottom right) Cumulative intensity merged FLIM lifetime image with motion correction, estimated using the first moment of the decay.
Motion correction of multispectral imaging of labelled immune cells in a murine lymph node.
Associated with Figure 7. (left) Uncorrected time series. (middle) Time series corrected using Imaris drift correction (right) Time series corrected with Galene.
Tutorial screencast.
Screencast documenting motion correction of FLIM data using Galene.
Tables
Reagent type (species) or resource | Designation | Source or reference | Identifiers | Additional information |
---|---|---|---|---|
Strain, strain background (Rac1-FRET, C57BL/6 (mixed)) | Rac1-FRET biosensor mouse | Johnsson et al. (2014) | Rac1-FRET biosensor mouse | |
Strain, strain background (BALB/c-Fox1nuAusb, Mus) | BALB/c-Fox1nuAusb | Australian BioResources | BALB/c-Fox1nuAusb | |
Cell line (Mus) | KPC | Morton et al. (2010a) | KPC primary cancer cells | |
Antibody | active Rac1-GTP | NewEast Biosciences | RRID:AB_1961793 | 1:400 concentration |
Recombinant DNA reagent | Rac1-FRET biosensor | Itoh et al. (2002) | Raichu-1011X ECFP- SEYFP | |
Recombinant DNA reagent | Src-FRET biosensor | Wang et al. (2005), Vennin et al. (2017) | Src-FRET biosensor | |
Chemical compound, drug | (-)-Scopolamine-N- butylbromide | Sigma-Aldrich | PubChem:CID_6852391; Sigma:S7882 | |
Chemical compound, drug | Phorbol myristate acetate | Sigma-Aldrich | PubChem:CID_27924; Sigma:P8139 | |
Chemical compound, drug | Fasudil | Jomar Life Research | PubChem:CID_163751; Jomar:HA-1077 | |
Software, algorithm | Matlab R2018a | Mathworks | RRID:SCR_001622 | |
Software, algorithm | FLIMfit | Warren et al. (2013) | RRID:SCR_016298 |
Additional files
-
Supplementary file 1
Acquisition and realignment parameters used through the study.
- https://doi.org/10.7554/eLife.35800.024
-
Transparent reporting form
- https://doi.org/10.7554/eLife.35800.025