The influence of task outcome on implicit motor learning
Figures
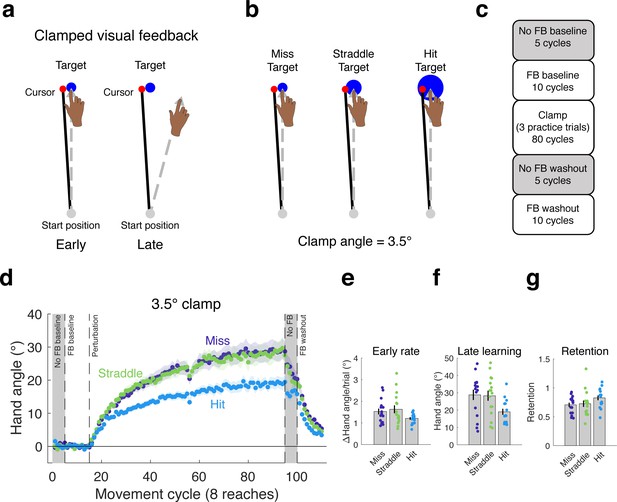
Hitting the target attenuates the behavioral change from clamped feedback.
(a) During clamped visual feedback, the angular deviation of the cursor feedback is held constant throughout the perturbation block, and participants are fully informed of the manipulation. (b) The clamp angle was equal across all three conditions tested in Experiment 1, with only the target size varying between conditions. (c) Block design for experiment. (d) As in previous studies with clamped feedback, the manipulation elicits robust changes in hand angle. However, the effect was attenuated in the Hit Target condition, observed in the (e) rate of early adaptation, and, more dramatically, in (f) late learning. (g) The proportion of learning retained over the no feedback block following the clamp did not differ between groups. Dots represent individuals; shading and error bars denote SEM.
-
Figure 1—source data 1
This file contains hand angle data for each trial and participant in Experiment 1, and was used to generate Figure 1d–g.
Reaction times (RTs) and movement times (MTs) are also included. Note that hand angles were flipped for participants who experienced a counter-clockwise clamp.
- https://doi.org/10.7554/eLife.39882.003
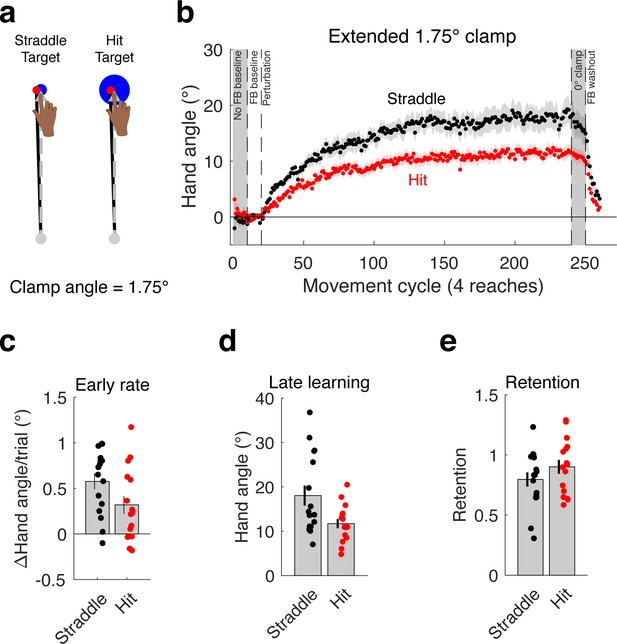
The effects of hitting a target generalize to a different context and remain consistent at asymptote.
The attenuation of adaptation caused by hitting the target (a) generalizes to a different clamp angle and is stable over an extended clamp block (b). As in Experiment 1, there was (c) a marginal difference in early adaptation rate that became (d) a more dramatic difference in late learning. (e) Again, there was no difference in the proportion of retention, this time during a 0° clamp block. Dots represent individuals; shading and error bars denote SEM.
-
Figure 2—source data 1
This file contains hand angle data for each trial and participant in Experiment 2, and was used to generate Figure 2b–e.
Reaction times (RTs) and movement times (MTs) are also included. Note that hand angles were flipped for participants who experienced a counter-clockwise clamp.
- https://doi.org/10.7554/eLife.39882.005
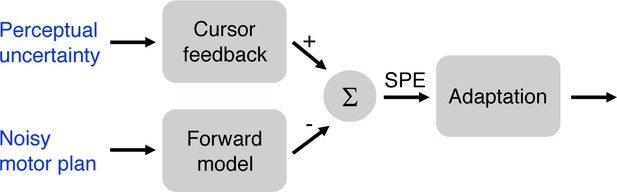
Target size could affect adaptation due to increased perceptual uncertainty or greater variability in motor planning.
In the case of perceptual uncertainty, the feedback signal is weakened, thus leading to a weaker SPE signal. In the case of noisy motor planning, the forward model prediction would also be more variable and effectively weaken the SPE.
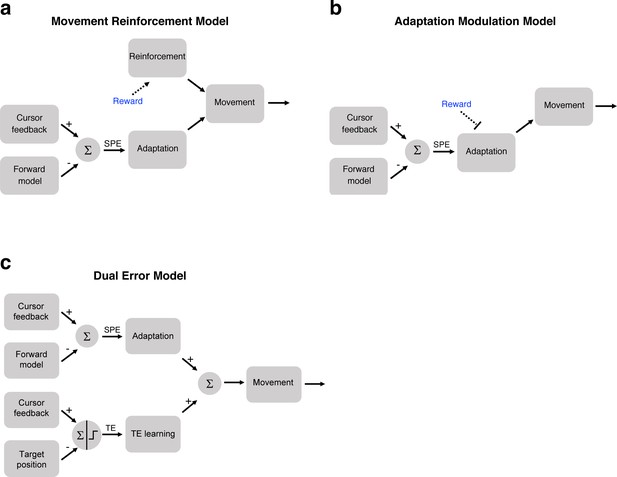
Three models of how intrinsic reward or target error could affect learning.
(a) In the Movement Reinforcement model, reward signals cause reinforcement learning processes to bias future movements toward previously rewarded movements. The adaptation process is sensitive only to SPE and not reward. The overall movement reflects a composite of the two processes. (b) In the Adaptation Modulation model, reward directly attenuates adaptation to SPE. (c) In the Dual Error model, a second, independent implicit learning process, one driven by TE, combines with SPE-based adaptation to modify performance.
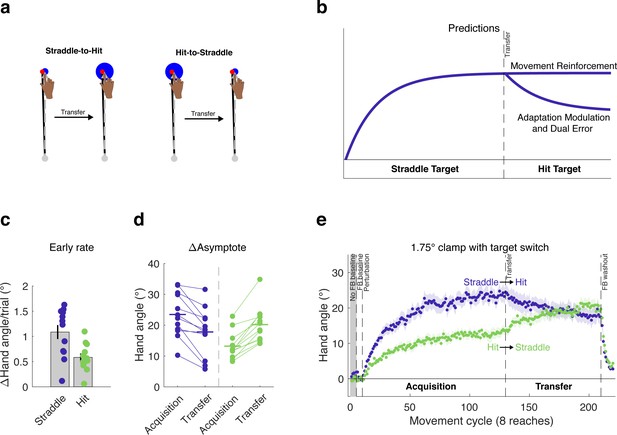
Within-subject transfer design to evaluate models of the impact of task outcome on implicit motor learning.
(a) Using a transfer design, (b) the models diverge in their behavioral predictions for the Straddle-to-Hit group following transfer. The Movement Reinforcement model predicts a persistent asymptote following transfer, whereas the Adaptation Modulation and Dual Error models predict a decay in hand angle. During the acquisition phase, we again observed differences between the Hit and Straddle groups in the (c) early adaptation rate as well as (d) late learning. All participants in both groups demonstrated changes in reach angle consistent with the Adaptation Modulation and Dual Error models. (e) The learning functions were inconsistent with the Movement Reinforcement model. Note that the rise in hand angle for the Hit-to-Straddle group is consistent with all three models. Dots represent individuals; shading and error bars denote SEM.
-
Figure 5—source data 1
This file contains hand angle data for each trial and participant in Experiment 3, and was used to generate Figure 5c–e and Figure 7.
Reaction times (RTs) and movement times (MTs) are also included. Note that hand angles were flipped for participants who experienced a counter-clockwise clamp.
- https://doi.org/10.7554/eLife.39882.009
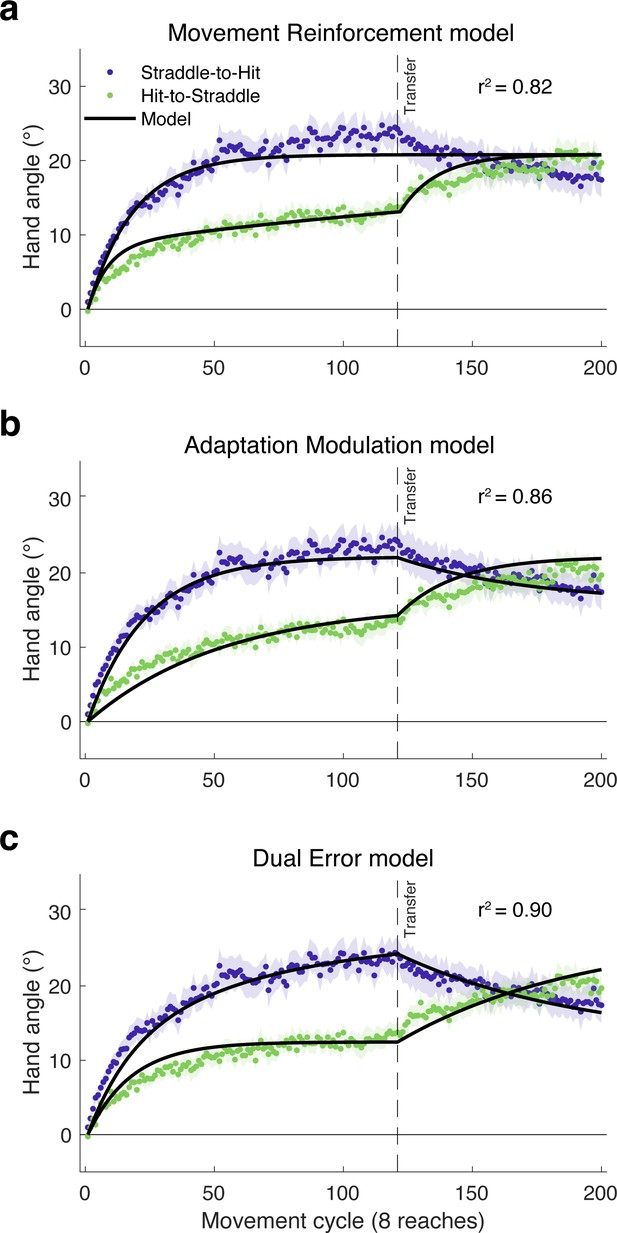
Model fits of the learning functions from Experiment 3.
The failure of the (a) Movement Reinforcement model to qualitatively capture the decay in hand angle following transfer in the Straddle-to-Hit condition argues against the idea that the effect of task outcome arises solely from a model-free learning process that operates independent of model-based adaptation. In contrast, both the (b) Adaptation Modulation and (c) Dual Error models accurately predict the changes in hand angle following transfer in both the Hit-to-Straddle and Straddle-to-Hit conditions.
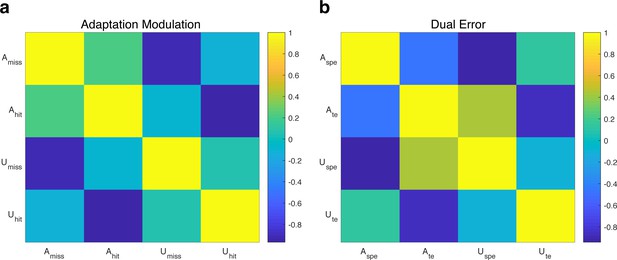
Correlations between bootstrapped parameter estimates.
Heat map of Pearson correlation coefficients between different parameters of the (a) Adaptation Modulation model and (b) Dual Error model. The strong negative correlations between retention and learning rate parameters (e.g. Amiss and Umiss) indicate a trade-off between these parameters. As such, caution is required concerning inferences about the impact of task outcome on the mechanisms of learning. Note that Amiss and Ahit refer to γA*A during miss or hit trials, respectively. Similarly, Umiss and Uhit refer to γu*U(e) during miss or hit trials.
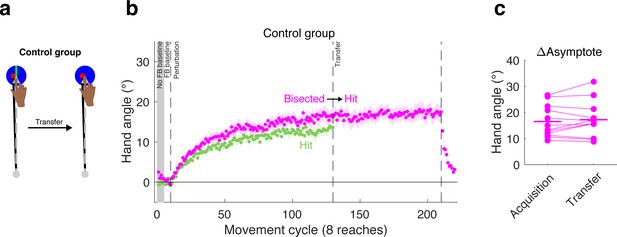
Effect of large target is not due to perceptual uncertainty.
(a) A control group was tested in a transfer design in which the large target was used in both phases, but a bisection line was present only during the acquisition phase. (b) The behavior of the control group (magenta) during the acquisition phase was not significantly different than that observed for the group that was tested with the (non-bisected) large target in the acquisition phase of Experiment 3 (re-plotted here in green), suggesting that perceptual uncertainty did not make a substantive contribution to the effects of hitting the target. Note that we do not display transfer data for the large target group since the target size changed for this group. (c) No change in asymptote was observed when going from the bisected target to the standard large target.
Tables
Model evaluations.
https://doi.org/10.7554/eLife.39882.012Basic models | # of free parameters | R-squared | AIC |
---|---|---|---|
Movement Reinforcement | 4 | 0.824 | 363 |
Adaptation Modulation | 4 | 0.861 | 269 |
Dual Error | 4 | 0.895 | 156 |
Hybrid Models | |||
Movement Reinforcement + Adaptation Modulation | 6 | 0.945 | −100 |
Movement Reinforcement + Dual Error | 6 | 0.945 | −97 |
Average Reaction Times (RTs) in ms.
Values represent mean ± SEM.
Experiment 1 | Baseline | Early clamp | Late clamp | No feedback |
---|---|---|---|---|
Hit | 325 ± 7 | 327 ± 7 | 347 ± 11 | 344 ± 12 |
Straddle | 362 ± 12 | 359 ± 14 | 397 ± 32 | 407 ± 33 |
Miss | 386 ± 22 | 383 ± 19 | 378 ± 15 | 385 ± 15 |
Experiment 2 | 0° clamp | |||
Hit | 378 ± 22 | 376 ± 27 | 354 ± 9 | 351 ± 9 |
Straddle | 373 ± 12 | 366 ± 13 | 368 ± 15 | 373 ± 16 |
Experiment 3 | ||||
Hit-to-Straddle | 356 ± 19 | 350 ± 15 | 326 ± 9 | N/A |
Straddle-to-Hit | 360 ± 8 | 360 ± 7 | 355 ± 7 | N/A |
Bisected-to-Normal | 400 ± 28 | 395 ± 27 | 400 ± 25 | N/A |
Average Movement Times (MTs) in ms.
Values represent mean ± SEM.
Experiment 1 | Baseline | Early clamp | Late clamp | No feedback |
---|---|---|---|---|
Hit | 153 ± 11 | 150 ± 10 | 137 ± 8 | 133 ± 9 |
Straddle | 162 ± 8 | 149 ± 8 | 139 ± 7 | 131 ± 7 |
Miss | 137 ± 7 | 134 ± 7 | 124 ± 6 | 118 ± 6 |
Experiment 2 | 0° clamp | |||
Hit | 149 ± 8 | 159 ± 20 | 155 ± 11 | 127 ± 7 |
Straddle | 157 ± 8 | 161 ± 15 | 170 ± 18 | 130 ± 8 |
Experiment3 | ||||
Hit-to-Straddle | 158 ± 7 | 189 ± 12 | 168 ± 12 | N/A |
Straddle-to-Hit | 164 ± 11 | 207 ± 28 | 169 ± 13 | N/A |
Bisected-to-Normal | 151 ± 11 | 165 ± 14 | 166 ± 15 | N/A |
Movement variability during baseline block.
Values represent mean ± SEM.
Experiment 1 | Baseline SD |
---|---|
Hit | 4.19 ±. 26° |
Straddle | 3.61 ±. 16° |
Miss | 3.80 ±. 15° |
Experiment 2 | |
Hit | 3.09 ±. 18° |
Straddle | 3.57 ±. 16° |
Experiment 3 | |
Hit-to-Straddle | 3.30 ±. 22° |
Straddle-to-Hit | 3.85 ±. 37° |
Bisected-to-Normal | 3.97 ±. 31° |
Additional files
-
Supplementary file 1
Target size experiment instructions.
- https://doi.org/10.7554/eLife.39882.014
-
Transparent reporting form
- https://doi.org/10.7554/eLife.39882.015