Self-organized reactivation maintains and reinforces memories despite synaptic turnover
Figures
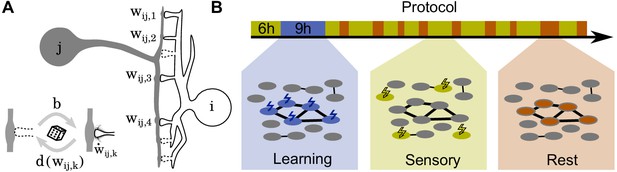
Schematics of model and stimulation paradigm.
(A) Neuron is connected to neuron at potential synaptic locations (here ). (Inset) Non-functional potential synapses (dashed) become functional synapses (solid) with a constant rate and are deleted with a weight-dependent rate . The weights of functional synapses are adapted by a Hebbian plasticity rule . (B) The simulation protocol is structured in three phases: During a learning phase (blue) groups of neurons are strongly stimulated in an alternating fashion. During subsequent sensory phases (yellow), the network is bombarded with quickly changing patterns. Finally, during rest phases (red), the network receives no stimulation but spontaneously reactivates.
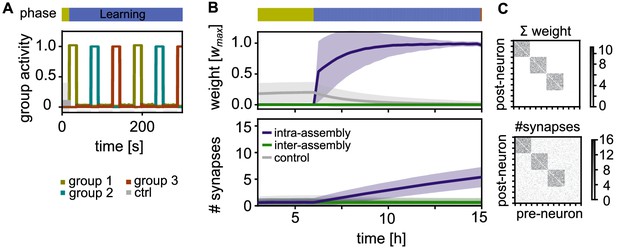
Activity and connectivity during the learning phase.
(A) Activity during the learning phase when assemblies receive strong, alternating stimulation. (B) Top: Time-course of the synaptic weights per synapse during learning. Between neurons within an assembly (purple), between control neurons (grey), and between different assemblies or assembly and control neurons (green). Curves depict the mean and shadings the standard deviation. Bottom: The slower time-course of the number of synapses per connection. (C) Top: Matrix depicting the sum of weights between 120 exemplary neurons (including all three stimulated groups) after learning at . Each point represents the synaptic weight summed over all the synaptic connections between the two neurons (x-axis pre- and y-axis-postsynaptic neuron; sorted). Bottom: Corresponding connectivity matrix of the number of synapses.
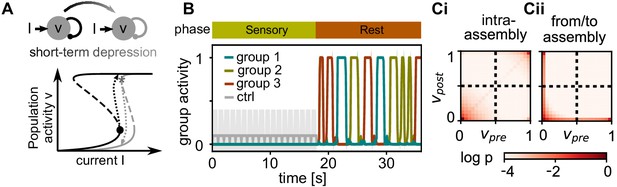
Activity after learning.
(A) Sketch of the dynamics during the rest phase. Solid lines mark the fixed points of population activity in a strongly connected assembly for relaxed weights (black) and after short-term depression (grey). When the assembly is taken beyond a upper bifurcation point (black dot), it becomes highly active (black dashed arrow). Subsequently, short-term depression decreases the recurrent connectivity and the population activity falls back to the low activity (grey dashed arrow). (B) Mean activity in stimulated groups and control neurons during sensory and rest phase. Shadings depict standard deviations. (Ci) Correlation of pre- and postsynaptic activity during the rest phase for intra-assembly connections. (Cii) Same for connections between two stimulated groups and between stimulated groups and control neurons.
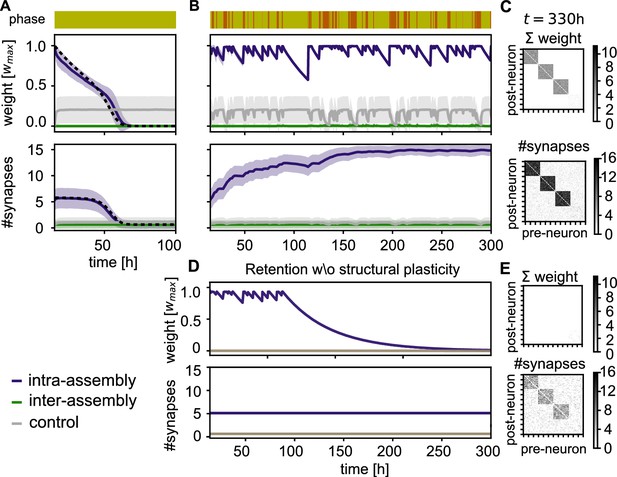
Stabilization of connectivity after learning.
(A) Without resting intervals mean intra-group weights decay (purple), followed by a decay in connectivity (bottom panel). Weights between control neurons (grey) and between different assemblies or assembly and control neurons (green) remain low. Dashed line depicts theoretical prediction; shading represents standard deviation. (B) Reactivation during rest phases rescues the assemblies. (C) Connectivity matrix depicting the sum of weights (top) and number of synapses (bottom) between 120 exemplary neurons at the end of the simulation in panel B. (D) Simulation with the same sequence of sensory and rest phases, but all structural plasticity is blocked after learning (). The memory is retained for a while, but after about 100 hr, when there is a longer period without reactivation, the assemblies decay. (E) Connectivity matrices as in panel C for the simulation in panel D. Although the number of synapses is similar, the synaptic weights have decayed.
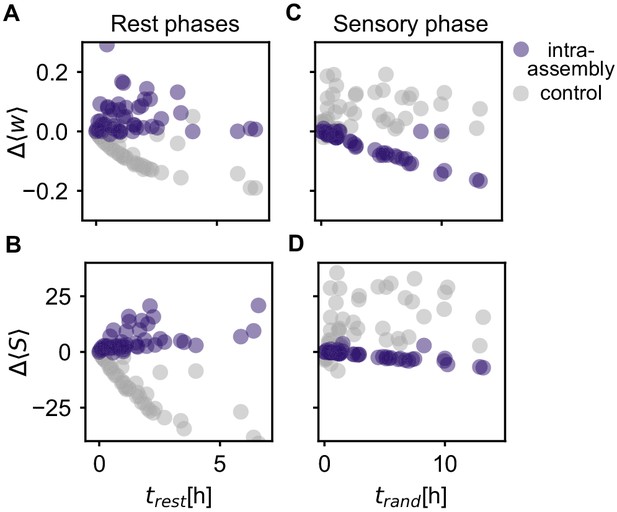
Connectivity changes in individual phases.
Rest phases strengthen intra-assembly connectivity and weaken extra-assembly connectivity. (A) Change of the average synaptic weight between neurons within the same assembly (blue) and between neurons outside the assemblies (grey) during individual rest phases depending on the length of the resting interval. (B) Change in the mean number of stable synapses (synapses above ) during individual rest phase. Both intra-assembly weights and number of connections grow, whereas connectivity outside the assemblies decays. (C) During the sensory intervals, the opposite occurs. (D) Change in the mean number of stable synapses during sensory intervals. .
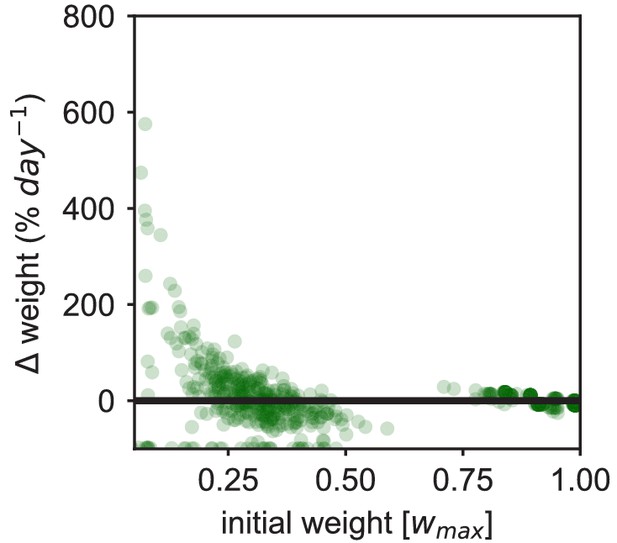
Net weight change after 24 hr of repeated sensory and rest phases.
Relative change of the synaptic weight after 24 hr of repeated sensory and rest phases. Difference between synaptic weight (of individual synapses) at each full day in the simulation (starting from ) and the same weight 24 hr later (if still existent normalized by the initial weight).
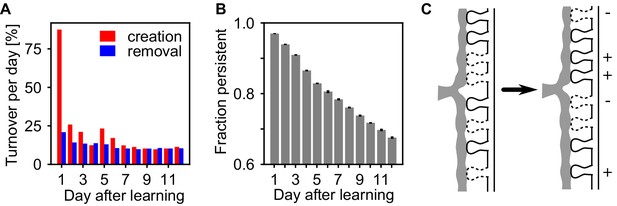
Assemblies undergo strong synapse turnover.
(A) Synapse creation (red) and removal (blue) relative to the synapses existing at the previous day. Synapse creation is strongly elevated the first day after learning. (B) The decay of persistent synapses created during learning indicate ongoing removal and replacement of synapses that originally formed the memory. (C) Sketch of the structural changes occurring in the presence of self-reactivation. Although the synapses that have been created during learning are continuously removed (-), the collective dynamics of the assembly stabilizes new synapses (+) between its neurons which counteracts synapse loss and can lead to a net strengthening of the assembly.
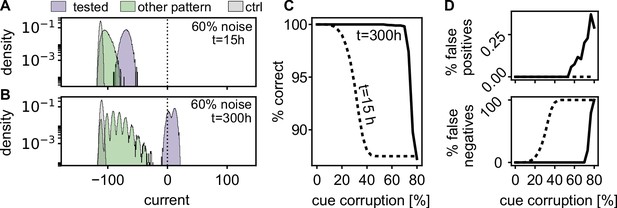
Spontaneous reactivation improves robustness of pattern completion.
(A) Histograms of the incoming currents to different classes of neurons right after learning (), when presented with corrupted memory patterns (histograms from 100 realizations). For successful pattern completion, the incoming currents of neurons in the tested stimulation pattern (blue) should be above the threshold at zero while the currents from neurons that are part of another pattern (green) and neurons that have not been stimulated during learning (grey) should be below. (B) After the reactivations during rest phases, the distributions are more separated (). (C) Fraction of correctly classified neurons (active and inactive) for different levels of cue corruption. (D) Fraction of wrongly inactive (bottom) and wrongly active neurons (top).
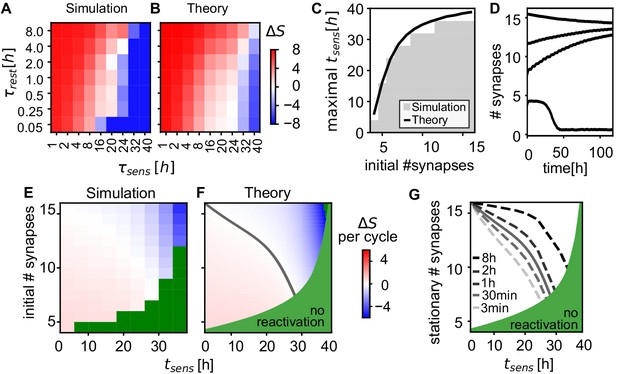
Analysis of connectivity decay and stationary weights.
(A) Change of the number of synapses between intra-assembly neurons after 5 days. The intra-assembly connectivity grows stronger for longer rest phases and shorter sensory phases. For long sensory phases connectivity decreases. (Averaged over fifteen 30-neuron assemblies initialized with eight synapses per connection.) (B) The theoretical prediction matches the simulation. (C) Maximal duration of sensory phase after which assemblies still self-reactivate increases when starting of with a larger initial numbers of synapses. The curve shows the theoretical predictions of the latest possible reactivation. (D) Time-course of the average number of synapses (network alternating between sensory phases and rest phases). Above a minimal initial number of synapses, a convergence to a stable state can be observed. (E) Change in the number of synapses after one cycle of sensory and rest phase for different initial numbers of synapses and durations of the sensory phase (). In the green region, reactivations will not occur (see panel C) and assemblies will decay (see panel D). (F) Theoretical prediction of the change per cycle (color code) matches experiment and exhibits a stable stationary value (solid grey curve). (G) Theoretically predicted stationary number of synapses for different rest phase durations.
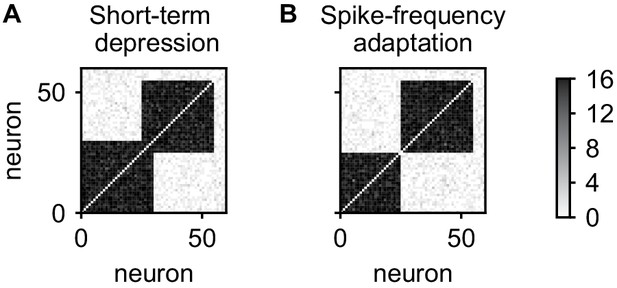
Stability of overlapping cell assemblies for alternative adaptation mechanisms.
(A) Connectivity matrix of the number of synapses after 5 days for two overlapping assemblies and the combination of plasticity processes used in this paper. (B) Same for using spike-frequency adaptation instead of short-term depression. Neurons from the overlap-region have become associated to one of the assemblies and disconnected from the other. In all cases, connections within assemblies were initialized with eight synapses.
Additional files
-
Source code 1
Simulation source code.
- https://doi.org/10.7554/eLife.43717.012
-
Transparent reporting form
- https://doi.org/10.7554/eLife.43717.013