Environmental heterogeneity can tip the population genetics of range expansions
Figures
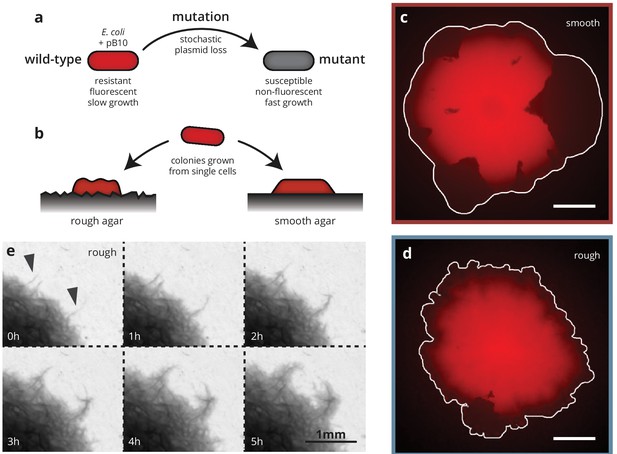
Substrate roughness impacts bacterial colony morphology.
(a and b) Colonies of E. coli were grown from single cells harboring a plasmid containing a fluorescence gene and a resistance cassette. Loss of the plasmid leads to non-fluorescent ('mutant’) sectors in the population that expand at the expense of the fluorescent ('wild-type’) population. Colony morphology depends on whether the colony is grown on smooth (c) or rough (d) agar surfaces (Materials and methods). White scale bars are 2 mm. (e) On rough surfaces, troughs in the surface direct growth along them, leading to locally accelerated regions (arrows) that widen slowly and are eventually integrated with the bulk of the population.
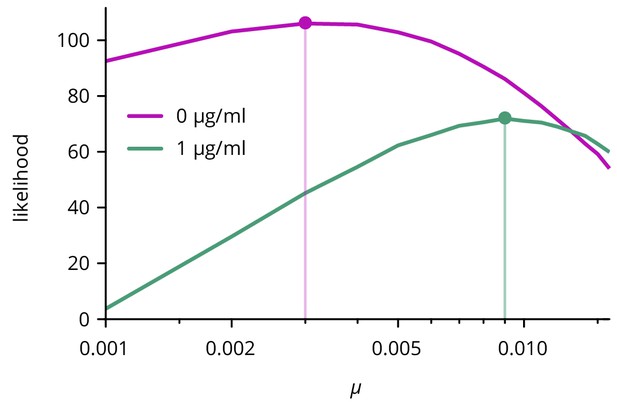
Likelihood of mutation rate for two different concentrations of doxycycline.
Only the curves with the maximum likelihood value for are shown.
-
Figure 1—figure supplement 1—source data 1
Source data for Figure 1—figure supplement 1 (Likelihood of mutation rates).
- https://doi.org/10.7554/eLife.44359.005
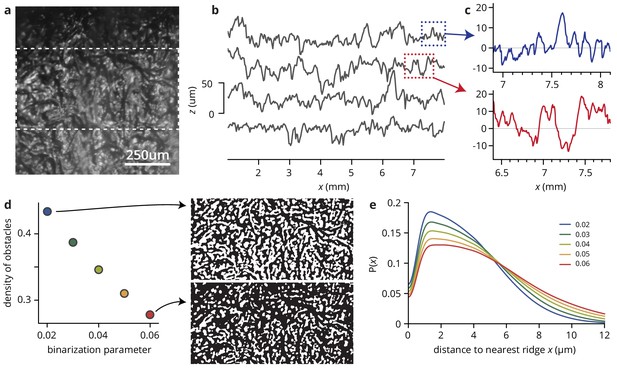
Analysis of substrate roughness.
(a) Microscopic image of an agar surface patterned with filter paper as described in Materials and methods. The image was created by stitching together six images taken on a Dektak 150 profilometer under oblique illumation. Light areas correspond to elevations, dark areas to troughs. (b) Traces along the vertical direction in panel (a), shifted for visibility (see Materials and methods). The mean roughness is 10 ± 1 μm, with a range of elevations to troughs of 50 μm. (c) Close-up of longer runs in (b). (d) Estimating the density of 'obstacles’, that is parts of the patterns that are significantly elevated compared to their surroundings. The images are binarized version of the area between the dashed lines in (a), using the LocalAdaptativeBinarize function in Mathematica with at 15 pixel radius; pixels are scored as one if they are brighter than , where and are the local mean and standard deviation in intensity, and is an intensity shift. The dependence of the proportion of pixels scored as one on is shown in the plot in (d). (e) For different values of , histograms of the distances of dark pixels from the nearest white pixel, showing that the width of 'valleys’, i.e., the distance between adjacent ridges, is limited to ≤25 μm (twice the range of ).
-
Figure 1—figure supplement 2—source data 1
Source data for Figure 1—figure supplement 2 (Five stylus runs to measure substrate roughness).
- https://doi.org/10.7554/eLife.44359.007
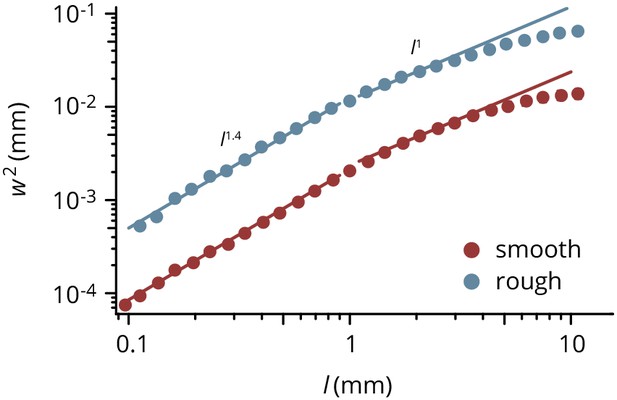
Roughness of E.coli colonies on smooth and rough substrates, defined as the mean square deviation from the best-fit circle.
Colony boundaries were extracted and the variance of the radius fluctuations measured over different window lengths . For interfaces in the KPZ universality class, . Points are averages over 118 (smooth) and 193 (rough) colonies, error bars are smaller than the symbols.
-
Figure 1—figure supplement 3—source data 1
Source data for Figure 1—figure supplement 3 (Roughness of smooth and rough colonies).
- https://doi.org/10.7554/eLife.44359.009
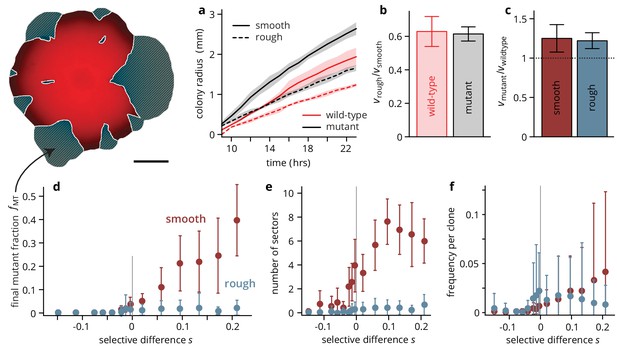
Substrate roughness limits the efficacy of selection in bacterial colonies.
(a–c) The roughness of the growth substrate reduces the radial expansion rate but has no impact on the relative growth rates between mutants and wild type (averages over four colonies per condition, shaded areas in panel (a) are ± 1 standard deviation). (d) The final mutant frequency (measured as the area fraction of non-fluorescent clones in the colonies, shown in white outline in sketch above) after 3 days of growth for mutants of a given fitness (dis)advantage differed strongly depending on the roughness of the surface they were grown on, despite relative growth rate differences being comparable (c) (throughout, points are averages over colonies, see Table 1, error bars are ± 1 standard deviation). The number of establishing sectors (e) was much smaller in rough colonies, with typically no more than two sectors per colony even for large . The sizes of individual clones are broadly distributed on both substrates (see also Figure 3), but the mean clone size (f) did not vary as strongly with in rough colonies as it did in smooth colonies.
-
Figure 2—source data 1
Source data for Figure 2.
colony radii.csv Source data for panel b (colony radii over time). fMT data.csv Source data for panel d (mutant frequency vs selective difference for rough and smooth substrates). sectors data.csv Source data for panel e (number sectors vs selective difference for rough and smooth substrates). fClone data.csv Source data for panel f (area fraction of individual clones vs selective difference for rough and smooth substrates).
- https://doi.org/10.7554/eLife.44359.020
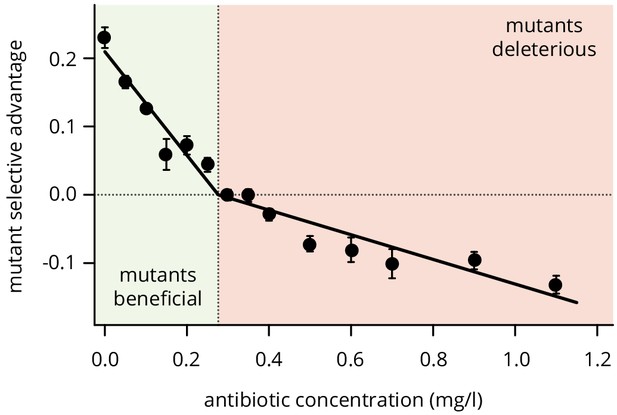
Selective difference between mutants and wild type at different concentrations of doxycycline.
For low antibiotic concentrations, plasmid loss is beneficial such that mutants have an advantage, while the mutants become first neutral and then deleterious for higher concentrations. Points are averages over 12 colony collisions, error bars are standard error of the mean.
-
Figure 2—figure supplement 1—source data 1
Source data for Figure 2—figure supplement 1 (Fitness measurements at various concentrations of doxycycline).
- https://doi.org/10.7554/eLife.44359.012
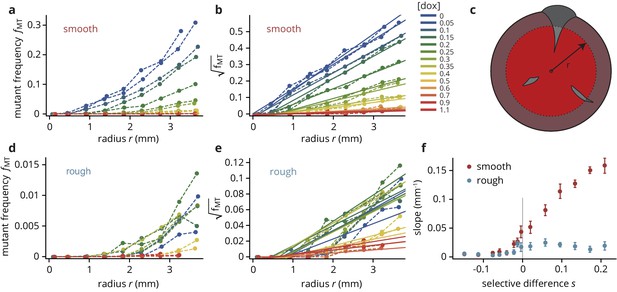
Mutant frequency as a function of radius (see panel (c) for schematic), for smooth (a–b) and rough (d–e) colonies.
The traces are approximately quadratic in , such that we can fit straight lines to the square-root transformed traces whose slopes provide a useful summary observable of the traces, shown in panel (f).
-
Figure 2—figure supplement 2—source data 1
Source data for Figure 2—figure supplement 2 (Mutant frequency as a function of radius for smooth and rough colonies).
- https://doi.org/10.7554/eLife.44359.014
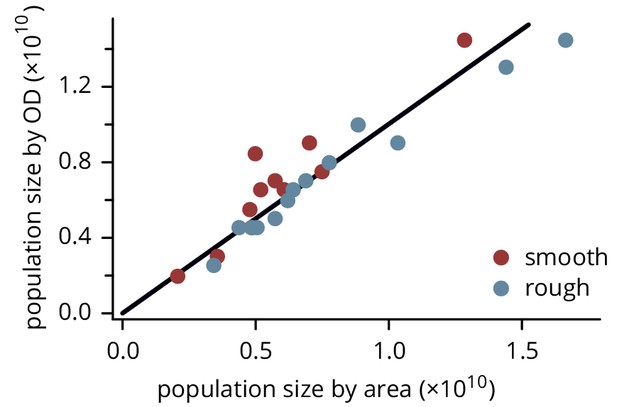
Population size of colonies estimated by measuring colony height and area, and by resuspending and measuring the resulting optical density.
The black line has slope 1. Note that the colonies were chosen to span a wide range of sizes; their sizes are not representative of the overall distribution of colony sizes.
-
Figure 2—figure supplement 3—source data 1
Source data for Figure 2—figure supplement 3 (Colony sizes on rough and smooth substrates).
- https://doi.org/10.7554/eLife.44359.016
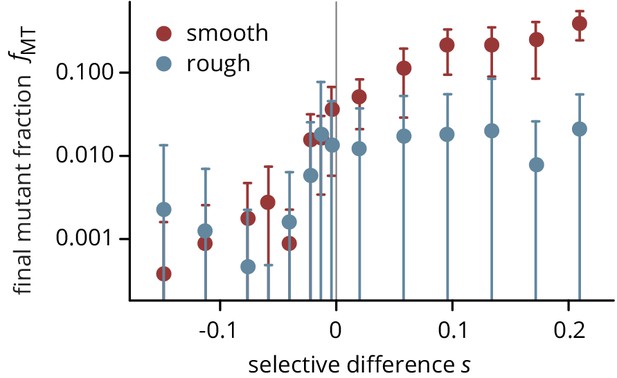
Final frequency of mutants after 3 days of growth, on smooth (red) and rough (blue) plates.
Same data as Figure 2a, plotted on a semi-logarithmic scale.
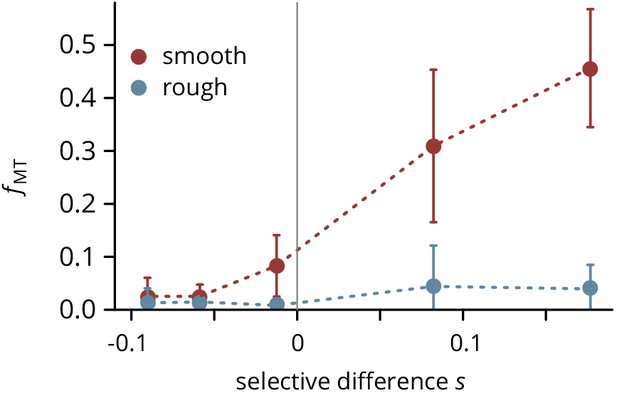
Mutant frequency measured in colonies grown on smooth and rough substrates at five doxycycline concentrations (converted to fitness differences as described in the main text) in a biological replicate experiment.
Error bars are ± 1 standard deviation.
-
Figure 2—figure supplement 5—source data 1
Source data for Figure 2—figure supplement 5 (Mutant frequency vs selective difference in replicate experiment).
- https://doi.org/10.7554/eLife.44359.019
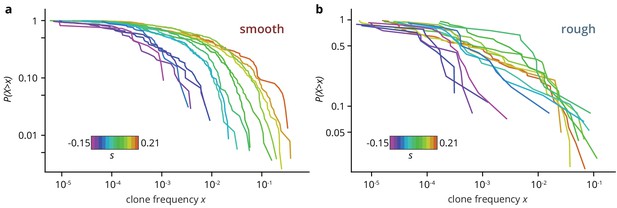
Clone size distribution for colonies grown on smooth (a) and rough (b) substrates.
Deleterious mutants are shown in magenta tones; their clones are typically small. Neutral clones are shown in green; their size distribution had a broad tail. Advantageous mutations in smooth colonies were even more broadly distributed, as large sectors establish more often. By contrast, beneficial clones in rough colonies had size distributions that were indistinguishable from the distribution for neutral mutations.
-
Figure 3—source data 1
Source data for Figure 3.
sfs_smooth.csv Source data for panel a (clone size distribution for smooth colonies). sfs rough.csv Source data for panel b (clone size distribution for rough colonies).
- https://doi.org/10.7554/eLife.44359.022
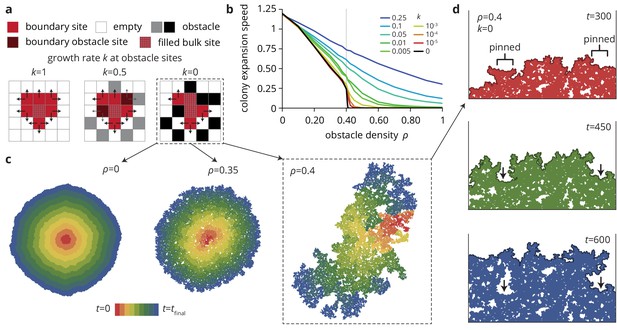
A minimal model captures the change in colony morphology in heterogeneous environments.
(a) The simulation proceeds by allowing cells with empty neighbors ('boundary sites’) to divide into empty space. Disorder sites (gray/black, density ) are randomly distributed over the lattice. (b) As the obstacle transparency, that is the growth rate at disorder sites, is reduced, the colony expansion speed decreases as a function of the obstacle density. For impassable disorder sites ('obstacles’, ), the expansion speed vanishes above a critical obstacle density , beyond which the population was trapped and could not grow to full size. (c) As approached colony morphology became increasing fractal. Colors from red to blue show the colony shape at different times. (d) Near the critical obstacle density , only few individual sites near the front have empty neighbors, leading to local pinning of the front (arrows), where the front can only progress by lateral growth into the pinned region.
-
Figure 4—source data 1
Source data for Figure 4 (Colony expansion rate in heterogeneous environments).
- https://doi.org/10.7554/eLife.44359.027
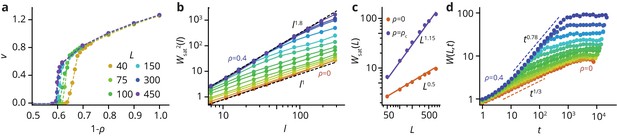
Characterization of Eden clusters with obstacles ().
(a) Taking the front speed as the order parameter, there is a phase transition at a critical obstacle density depending on system size . For an infinite system, . For , we have . (b) Roughness of fully developed interfaces at for varying obstacle densities, as a function of the window length , such that . At , we find , consistent with the KPZ universality class. At , we find , consistent with the QEW universality class (see Theory in the Materials and methods section), which is known to be characterized by two roughness exponents, a local exponent (, panel b), and a global exponent when the roughness is measured over the whole system size (see panel c) (Amaral et al., 1995). Importantly for , there is a crossover length scale below which the system displays QEW scaling, whereas it returns to the KPZ scaling on longer length scales. (d) The time evolution of the interface also follows dynamics consistent with KPZ () and QEW () in the limiting cases. For intermediate , there is a crossover from qEW at short times to KPZ dynamics at longer times, before the roughness saturates at a -dependent value. For easier analysis, all simulations were performed in a box-like geometry.
-
Figure 4—figure supplement 1—source data 1
Source data for Figure 4—figure supplement 1 (Analysis of colony expansion rate (v(rho).xlsx), interface width as a function of window length (w(l).xslx) and time (w(t).xslx), and saturation width (Wsat.xlsx)).
- https://doi.org/10.7554/eLife.44359.026
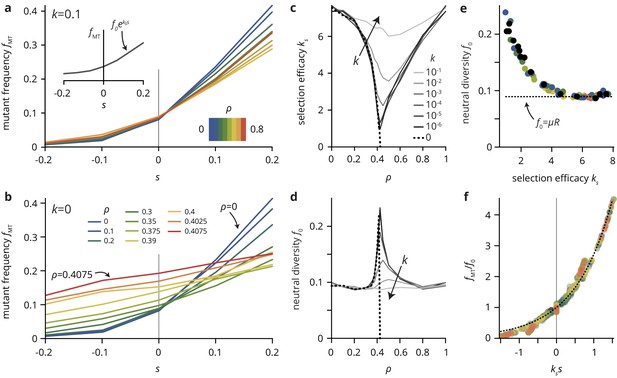
A simple lattice model reproduces strong impact of environmental heterogeneity on evolutionary dynamics.
(a,b) The mutant frequency as a function of the selective advantage of the mutants always increases with to a degree that depends on the density and the transparency of the disorder sites. (c,d) Parametrizing the mutant frequency for various values of and heuristically with (panel a, inset), we fit the neutral diversity (shown in panel d) and the selection efficacy (panel c). The selection efficacy serves as an inverse selection scale, describing the dependence of on . (e) A scatter plot of the fit parameters and for all values of and reveals that they are effectively not independent parameters, but that entirely determines and vice-versa (for a given population size and mutation rate μ; here, and ). The dashed line represents the expect neutral diversity for a circular colony, where , with the colony radius (Fusco et al., 2016). (f) Rescaling all curves by fitted values of and , all points fall close to a master curve given by (dashed line), motivating the parametrization introduced in (a).
-
Figure 5—source data 1
Source data for Figure 5.
ks_values.csv. Source data for panel c (fit parameter for various parameter values and ). f0 values.csv Source data for panel d (fit parameter for various parameter values and ). rescaled fMT data.xlsx Source data for panel f (rescaled for various parameter values and ).
- https://doi.org/10.7554/eLife.44359.031
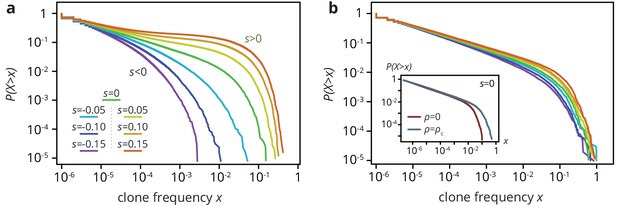
Clone size distribution for colonies grown on smooth (a) and rough (b) substrates in simulations.
Deleterious mutants are shown in magenta tones; their clones are typically small. Neutral clones are shown green; their size distribution is broad. Advantageous mutations (red tones) are even more broadly distributed, as large sectors establish more often. In the absence of environmental heterogeneity, the clone size distribution was very broad, with a low-frequency power-law regime corresponding to mutant bubbles and a steeper power-law at high frequencies characterizing sector sizes (Fusco et al., 2016). A deleterious fitness effect of the mutations created an effective cut-off because sectors no longer formed, whereas positive increased the likelihood of high-frequency clones, because sectors establish more often and grow to larger frequencies when they do. At the critical obstacle density, we found that a neutral clone size distribution that was remarkably similar to what we found without environmental heterogeneity (b, inset). By contrast, selective differences between mutants and wild type had a much less pronounced effect on the clone size distribution as the density of obstacles increased. The clone size distribution for both beneficial and deleterious mutations thus resembled the distribution for neutral mutations. In simulations at the critical obstacle density , became roughly independent of , such that fitness effects associated with the mutations were effectively inconsequential at the level of individual clones.
-
Figure 5—figure supplement 1—source data 1
Source data for Figure 5—figure supplement 1 (Clone size distribution for various for and ).
- https://doi.org/10.7554/eLife.44359.030
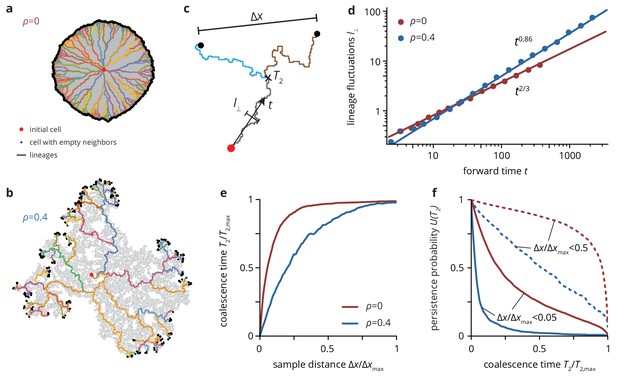
Neutral lineage dynamics change with environmental heterogeneity.
Lineage trees extracted from simulated colonies without (), (a) and with critical (), (b) environmental heterogeneity. (c) The lineage structure is characterized by the fluctuations of the lineages in forward time , and the pair coalescence time (backwards in time) of two samples that are a distance apart. (d) The lineage fluctuations depend on the environmental heterogeneity: in the absence of environmental disorder, lineage fluctuations scale as , whereas the lineages are rougher in strong disorder, scaling as . Strong lineage fluctuations in heterogeneous environments also impact the mean pair coalescence time of two samples a distance apart by allowing lineages from distant regions of the colony to coalesce earlier than without environmental heterogeneity (panel e). As a consequence, the persistence probability , that is the probability that two sampled lineages have a pair coalescence time greater than is typically greater in homogeneous than in heterogeneous environments, even when conditioning on small distance between samples (solid lines, ; dashed lines, ).
-
Figure 6—source data 1
Source data for Figure 6.
lineage_fluctuations.csv Source data for panel d (lineage fluctuations vs forward time ). T2vsDeltaX data.csv Source data for panel e (mean pair coalescence time vs pair distance ). P(T2) data.csv Source data for panel f (persistence probability vs pair coalescence time ).
- https://doi.org/10.7554/eLife.44359.035
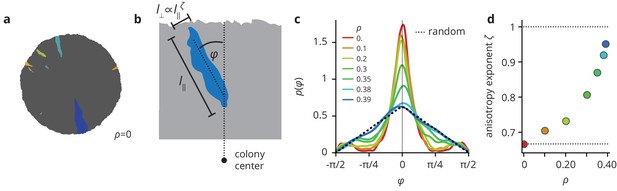
Environmental heterogeneity alters the shapes of mutant clones.
(a) Mutant clones (colored by the time of mutation) in a simulated colony grown in a homogeneous environment. (c) Environmental heterogeneity impacts the directionality of clones (measured by the angle that a clone’s semi-major axis makes with the radial direction, see panel (b)), which are mostly aligned with the radial direction for smooth colonies, but point in essentially random directions in heterogeneous environments. (d) Clones become more isotropic as the pinning transition is approached, as measured by the anisotropy exponent which quantifies how the typical width of a clone scales with its length .
-
Figure 6—figure supplement 1—source data 1
Source data for Figure 6—figure supplement 1 (Clone angles and anisotropy exponents for neutral mutations for various ).
- https://doi.org/10.7554/eLife.44359.034
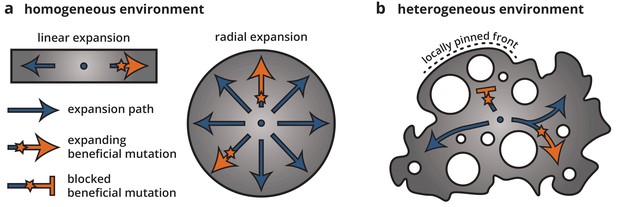
Cartoon of how environmental heterogeneity shapes evolutionary dynamics during range expansions.
In homogeneous environments (a), a locally established beneficial mutation (orange star), having survived genetic drift, can expand freely (arrows). By contrast, in heterogeneous environments (b), a beneficial mutation can become trapped in pinned stretches of the population, whereas expansion along open paths resembles a one-dimensional expansion.
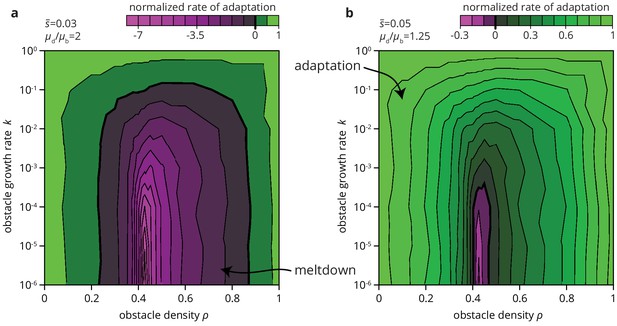
Environmental heterogeneity can alter evolutionary outcomes.
Rate of adaptation, defined as , computed for an exponential distribution of fitness effects at various values of the environmental parameters and (see Materials and methods). The distribution of fitness effects is characterized by two parameters: the ratio of deleterious to beneficial mutation rate ( in panel a, 1.25 in b), and , the average fitness effect of a mutation ( in panel a, 0.05 in b). The rate of adaptation is normalized to its maximal value at . Green tones correspond to adaptation (accumulation of beneficial mutations), magenta tones to accumulation of deleterious mutations.
Tables
Number of colonies assayed for each condition.
Pilot experiment one is reported in Figure 2—figure supplement 5, experiment two is reported in the main text.
Experiment 1 (Figure 2—figure supplement 5) | ||||||||||||||
---|---|---|---|---|---|---|---|---|---|---|---|---|---|---|
dox (μg/ml) | 0 | 0.2 | 0.4 | 0.6 | 0.8 | |||||||||
n (smooth) | 13 | 16 | 16 | 20 | 21 | |||||||||
n (rough) | 11 | 20 | 16 | 19 | 14 | |||||||||
Experiment 2 (main text) | ||||||||||||||
dox (μg/ml) | 0 | 0.05 | 0.1 | 0.15 | 0.2 | 0.25 | 0.3 | 0.35 | 0.4 | 0.5 | 0.6 | 0.7 | 0.9 | 1.1 |
n (smooth) | 8 | 30 | 37 | 26 | 31 | 27 | 32 | 41 | 30 | 36 | 30 | 24 | 31 | 28 |
n (rough) | 20 | 24 | 32 | 20 | 32 | 25 | 19 | 22 | 31 | 28 | 18 | 19 | 27 | 29 |
Characteristic exponents for the KPZ and QEW universality classes and measured in our simulations, which are in good agreement with the literature values in Amaral et al. (1995).
https://doi.org/10.7554/eLife.44359.038Exponent | KPZ | This study () | qEW | This study () |
---|---|---|---|---|
1/2 | 0.5 ± 0.05 | 0.92 ± 0.04 | 0.9 ± 0.05 | |
1/2 | 0.5 ± 0.05 | 1.23 ± 0.04 | 1.15 ± 0.05 | |
1/3 | 0.3 ± 0.03 | 0.86 ± 0.03 | 0.78 ± 0.05 | |
N/A | N/A | 0.24 ± 0.03 | 0.21 ± 0.03 | |
2/3 | 0.66 ± 0.02 | - | 0.86 ± 0.04 |
Additional files
-
Transparent reporting form
- https://doi.org/10.7554/eLife.44359.039