Linking glycemic dysregulation in diabetes to symptoms, comorbidities, and genetics through EHR data mining
Figures
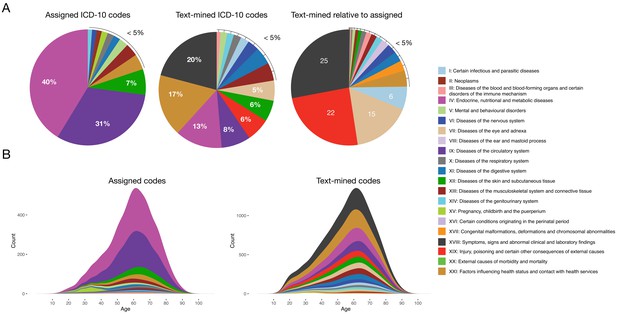
Comparison of distributions of ICD-10 diagnosis codes with and without text mining.
(A) Percentage of diagnosis codes belonging to the different ICD-10 chapters and the relative increase in diagnosis codes from the different chapters when combining the text-mined and assigned codes. (B) Age distributions of text-mined and assigned ICD-10 diagnosis codes from the SDCC corpus divided into the 21 ICD-10 chapters.
-
Figure 1—source data 1
Diagnosis code breakdown data.
- https://cdn.elifesciences.org/articles/44941/elife-44941-fig1-data1-v1.txt
-
Figure 1—source data 2
Age distribution data.
- https://cdn.elifesciences.org/articles/44941/elife-44941-fig1-data2-v1.txt
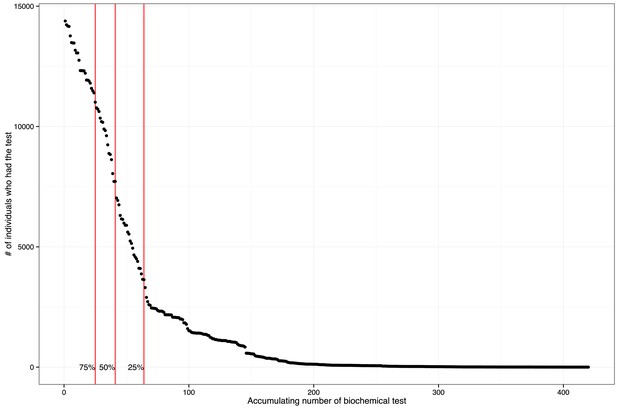
Distribution of patients per physiological and biochemical test.
Number of unique patients who have had a given biochemical test. Shows that the majority of biochemical tests were performed on only a few individuals. The red lines mark the 25, 50, and 75% of individuals in the cohort: 26, 41, 64, and 356 biochemical tests were taken in 75%, 50%, 25%, and less than 25% of the cohort, respectively.
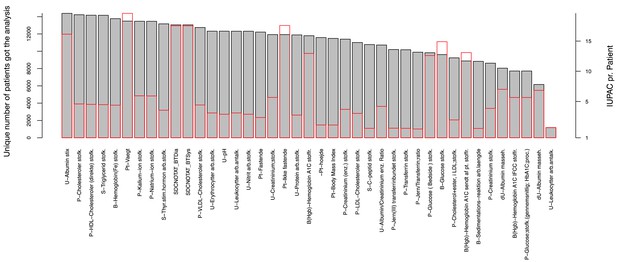
Physiological and biochemical tests in the SDCC corpus.
Bar plots of unique individuals who have had the test taken (grey bars) and the number of times each individual have had the test taken (red outline bars).
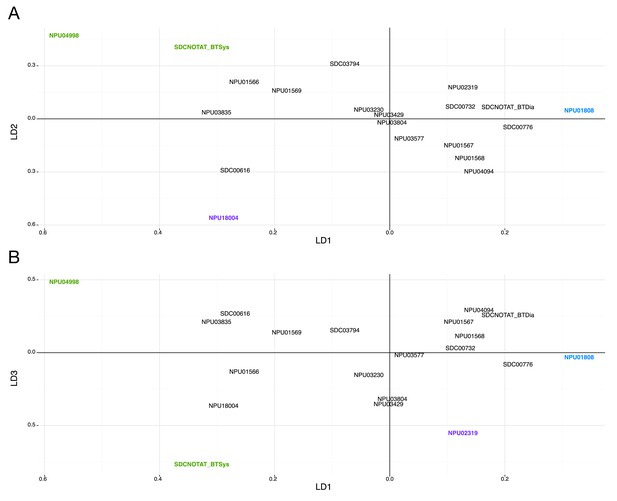
Linear Discriminant Analysis 1 (LDA).
Linear Discriminant Analysis (LDA) was performed on the biochemical tests for the 71 clusters with at least 50 individuals. The linear discriminants (LD) 1 and 2 (A) and 1 and 3 (B) for the LDA, are shown using the biochemical test identifiers. Identifiers in blue contributes most to the variance among clusters for LD1, purple identifiers contribute most to the variance from LD2 or LD3, and green colored identifiers are common across LD1 and LD2 or LD1 and LD3.
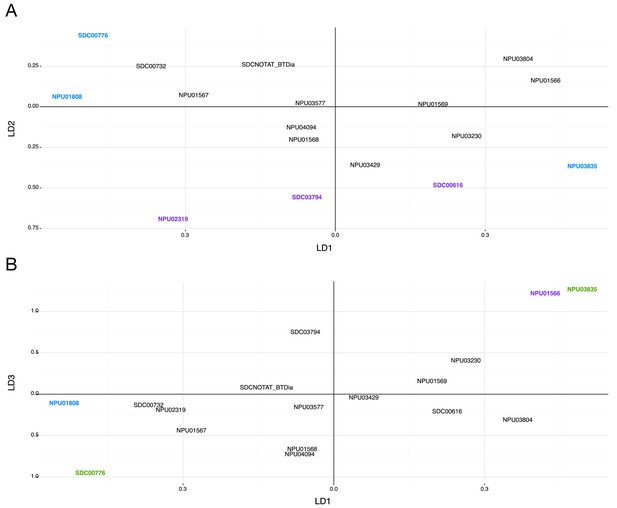
Linear Discriminant Analysis 2 (LDA).
The three identifiers contributing most to the variance in the LDA (NPU04998, NPU18004, and SDCNOTAT_BTSys) were removed, and a new LDA analysis was performed. A and B display the relationship between LD 1, 2, and 3. Blue colored identifiers contribute the most to the variance between clusters for LD1, purple colored contribute the most to the variance for LD2 and 3, and green colored identifiers are the ones common among LD1 and 2, or LD2 and LD3.
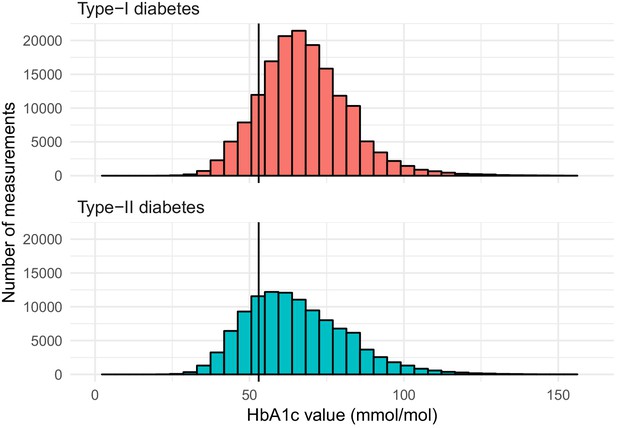
Distribution of HbA1c measurements for T1D and T2D patients.
The vertical line corresponds to the HbA1c threshold used when defining dysregulation.
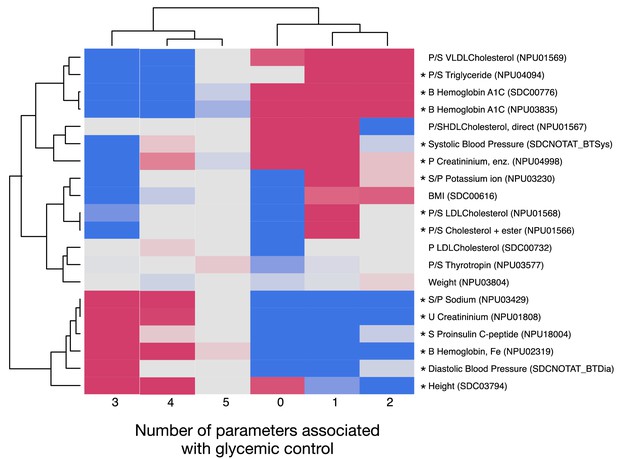
Biochemical patterns for the level of glycemic dysregulation.
The groups are based on numbers of parameters of glycemic dysregulation. A MANOVA test was performed to detect if there were any differences in means among the groups, for each biochemical test (Bonferroni adj. p-value<=0.01). These groups are marked with an asterisk (*). Subsequently a Kolmogorov-Smirnov test was applied to discover whether the distribution of mean biochemical values for each group was significantly higher or lower than the other groups (Bonferroni adj. p-value<=0.01). Blue indicates mean distributions that are significantly higher than the other groups, and red indicates significantly lower distributions. They grey and less clear color indicates that the distribution within this group was not significantly different from the other groups. B = blood, p=Plasma, S = serum, U = urine.
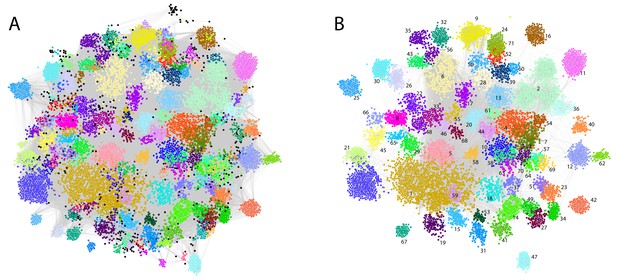
Phenotypic clusters found in the SDCC cohort.
The clustering was created with diagnosis vectors of 13,928 patients (with text in the record) comprising both text-mined and assigned ICD-10 codes. A total of 172 clusters were created, where 11,208 patients (80.47%) were captured in the clustering (clusters with five or less patients were discarded for statistical reasons). (A) Each node represents a patient within the corpus colored by the association to one of the 172 unique clusters. (B) The 71 clusters with at least 50 patients colored with the same palette as in (A).
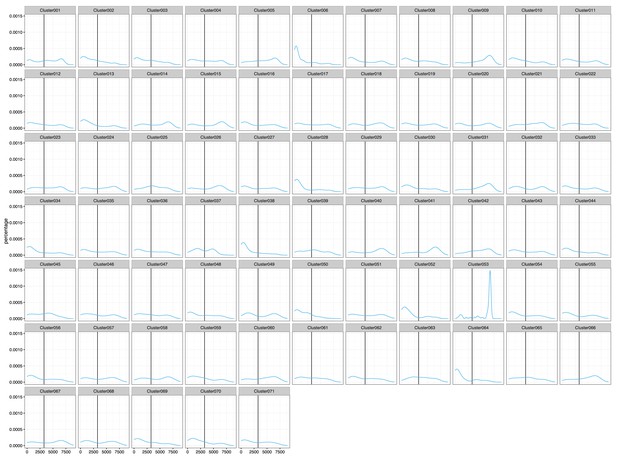
Density of days in contact with SDCC for each cluster.
Density diagram of days an individual has been connected to Steno Diabetes Center Copenhagen (SDCC) divided by each cluster. The black line indicates the mean of SDCC connection time for the entire cohort. Some clusters for example Cluster1, show two peaks indicating that there are at least two groups of individuals in a cluster; one connected to SDCC more than the average cohort, and one connected less.
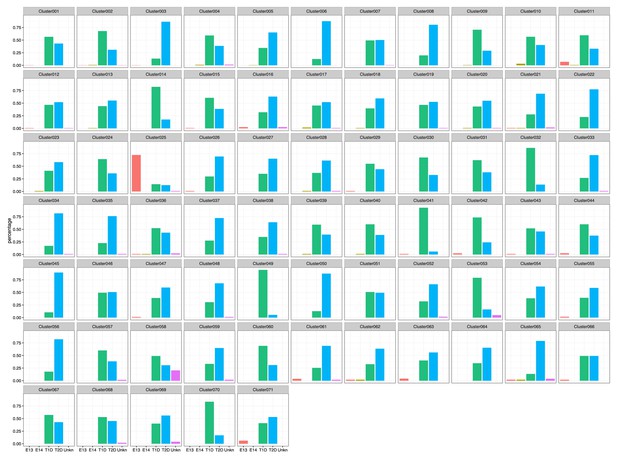
Distribution of assigned primary diabetes type for each cluster.
Density diagram of days an individual has been connected to Steno Diabetes Center Copenhagen (SDCC) divided by each cluster. The black line indicates the mean of SDCC connection time for the entire cohort. Some clusters for example Cluster1, show two peaks indicating that there are at least two groups of individuals in a cluster; one connected to SDCC more than the average cohort, and one connected less.
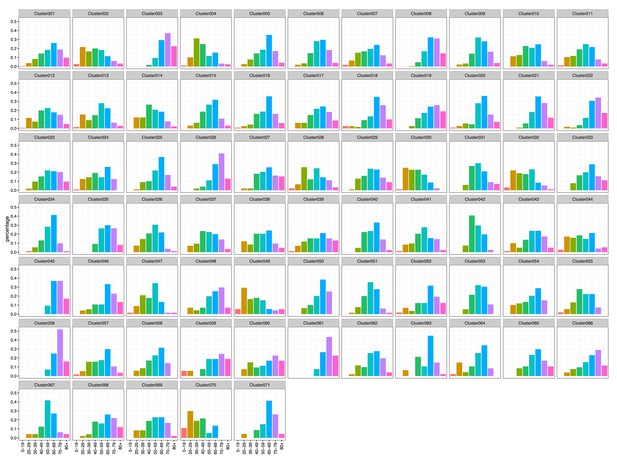
Distribution of age for each cluster.
Density diagram of days an individual has been connected to Steno Diabetes Center Copenhagen (SDCC) divided by each cluster. The black line indicates the mean of SDCC connection time for the entire cohort. Some clusters for example Cluster1, show two peaks indicating that there are at least two groups of individuals in a cluster; one connected to SDCC more than the average cohort, and one connected less.
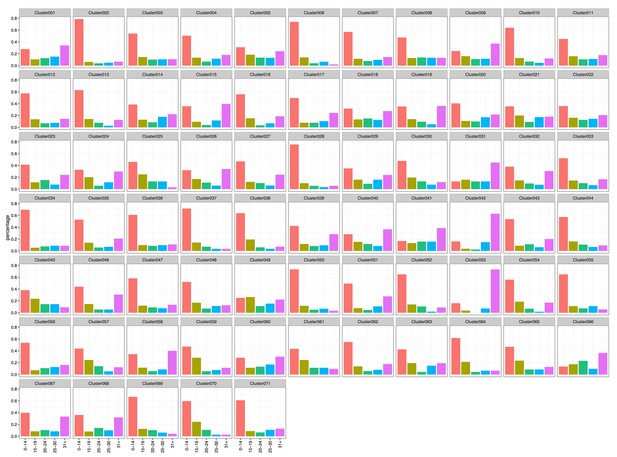
Distribution of duration of diabetes for each cluster.
The diabetes duration distribution for individuals in each cluster. Each bar corresponds to a bin for a given interval of the diabetes duration. The height of the bins is the percentage of individuals in the cluster being in that diabetes age bin. The diabetes duration is calculated as the difference in years between diabetes onset and the date for the latest SDCC data entry.
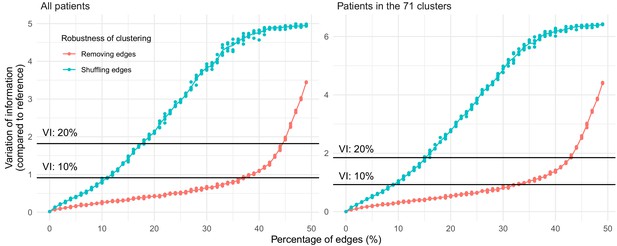
Clustering robustness analysis.
To assess the robustness of the clustering, various diluted (points in blue) and shuffled realizations (points in red) of the similarity network were used as input for the MCL algorithm, and the resulting clustering’s were compared to the reference clustering using the Variation of Information (VI) measure. The two horizontal lines show the value that the VI would take if we were to randomly assign 10% and 20% of the vertices to different random clusters, respectively.
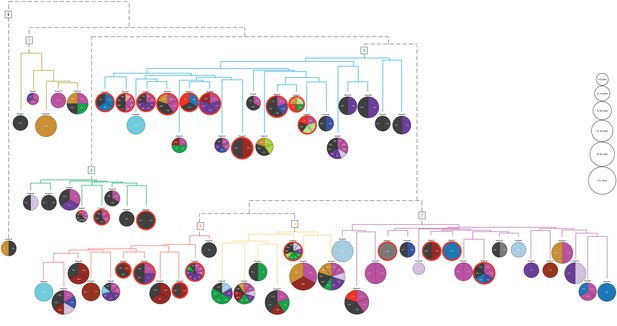
Hierarchical clustering based on enriched comorbid ICD-10 diagnoses.
The comorbidities present in a minimum of 10 patients and significantly enriched (adj. p-value<=0.05) in each cluster are shown in the pie charts. The number of significant codes ranges from 1 to 10. Each color corresponds to an ICD-10 code chapter as listed in the legend of Figure 1. Six main groups and an outlier (cluster 70) resulted, and the colors of the dendrogram branches indicate to which hierarchical groups the clusters belong. The size of the pie charts represents the average diabetes duration (years with diabetes) divided into six bins. The 21 clusters where at least 50% of the patients have three or more HbA1c severity parameters are marked with a red line surrounding the pie chart.
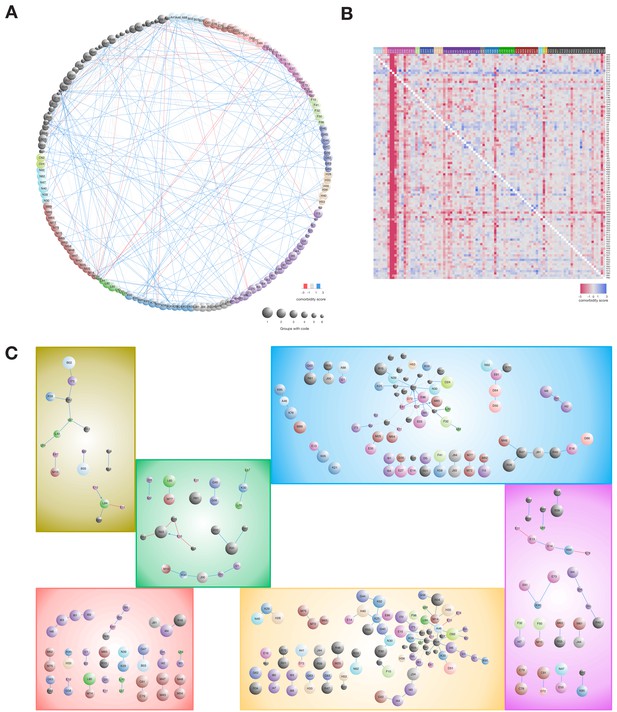
Comorbidity patterns within the six symptom groups.
(A) Comorbidity correlations between the combined symptom groups. (B) Asymmetric comorbidity matrix for observing row diagnosis codes before column diagnoses. First, we calculated Bonferroni corrected p-values for diagnosis pair directionality, second, we extracted the top 100 unique diagnosis codes pairs with lowest adjusted p-values and lastly, we calculated a comorbidity score (CS) by using the log2 of observing the pair more or less than expected. The heat-map colors reflect the CS quantification. (C) Comorbidity pairs unique for each of the symptom groups. All interactions are observed significantly more (blue) or less (red) than expected (adj. p-value<=0.01). Arrows indicate that the diagnoses are observed in the particular order (Fischer’s exact test with Bonferroni correction p-value<=0.01). Node size indicates in how many symptom groups the diagnosis code is observed in, ranging from one group (the diagnosis is unique for the group, largest nodes) to six groups (all groups have the code, smallest nodes).
-
Figure 4—source data 1
Comorbidity pattern data.
- https://cdn.elifesciences.org/articles/44941/elife-44941-fig4-data1-v1.txt
Additional files
-
Supplementary file 1
Statistics for the metadata.
- https://cdn.elifesciences.org/articles/44941/elife-44941-supp1-v1.docx
-
Supplementary file 2
Statistics for the physiological tests.
- https://cdn.elifesciences.org/articles/44941/elife-44941-supp2-v1.docx
-
Supplementary file 3
Enrichment of drug prescriptions.
- https://cdn.elifesciences.org/articles/44941/elife-44941-supp3-v1.docx
-
Supplementary file 4
Enrichment of ICD-10 and SDC-custom codes.
- https://cdn.elifesciences.org/articles/44941/elife-44941-supp4-v1.docx
-
Supplementary file 5
The five top ranked independent genetic associations for individual clusters, symptom clusters, and dysregulation.
- https://cdn.elifesciences.org/articles/44941/elife-44941-supp5-v1.docx
-
Supplementary file 6
The SDC-custom dictionary.
- https://cdn.elifesciences.org/articles/44941/elife-44941-supp6-v1.docx
-
Transparent reporting form
- https://cdn.elifesciences.org/articles/44941/elife-44941-transrepform-v1.docx