The role of structural pleiotropy and regulatory evolution in the retention of heteromers of paralogs
Figures
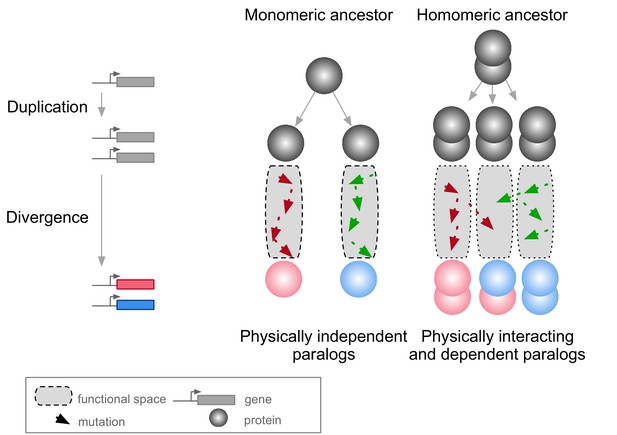
Mutations in paralogous proteins originating from an ancestral homomer are likely to have pleiotropic effects on each other’s function due to their physical association.
Gene duplication leads to physically interacting paralogs when they derive from an ancestral homomeric protein. The evolutionary fates of the physically associated paralogs tend to be interdependent because mutations in one gene can impact on the function of the other copy through heteromerization.
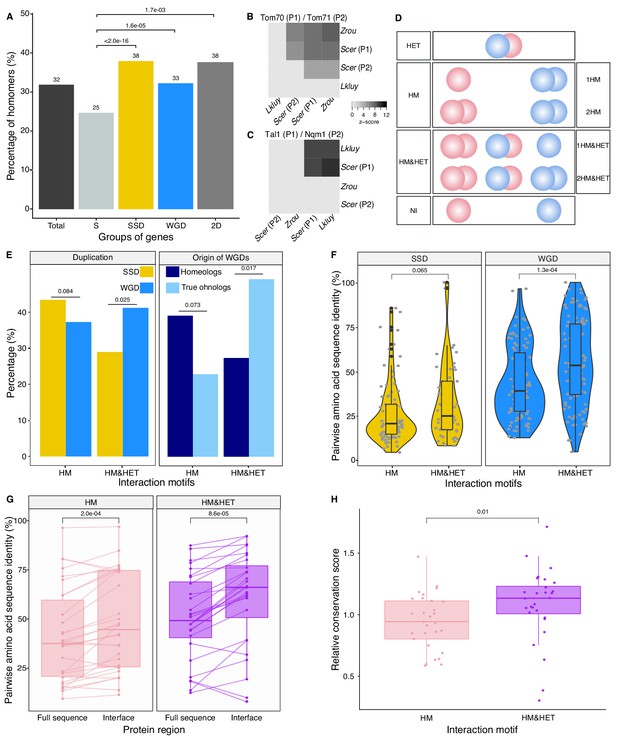
Homomers and heteromers of paralogs are frequent in the yeast protein interaction network.
(A) The percentage of homomeric proteins in S. cerevisiae varies among singletons (S, n = 2521 tested), small-scale duplicates (SSDs, n = 2547 tested), whole-genome duplicates (WGDs, n = 866 tested) and genes duplicated by the two types of duplication (2D, n = 136 tested) (global Chi-square test: p-value<2.2e-16). Each category is compared with the singletons using a Fisher’s exact test. P-values are reported on the graph. (B and C) Interactions between S. cerevisiae paralogs and pre-whole-genome duplication orthologs using DHFR PCA. The gray tone shows the PCA signal intensity converted to z-scores. Experiments were performed in S. cerevisiae. Interactions are tested among: (B) S. cerevisiae (Scer) paralogs Tom70 (P1) and Tom71 (P2) and their orthologs in Lachancea kluyveri (Lkluy, SAKL0E10956g) and in Zygosaccharomyces rouxii (Zrou, ZYRO0G06512g) and (C) S. cerevisiae paralogs Tal1 (P1) and Nqm1 (P2) and their orthologs in L. kluyveri (Lkluy, SAKL0B04642g) and in Z. rouxii (Zrou, ZYRO0A12914g). (D) Paralogs show six interaction motifs that we grouped in four categories according to their patterns. HET pairs show heteromers only. HM pairs show at least one homomer (one for 1HM or two for 2HM). HM&HET pairs show at least one homomer (one for 1HM&HET or two for 2HM&HET) and the heteromer. NI (non-interacting) pairs show no interaction. We focused our analysis on pairs derived from an ancestral HM, which we assume are pairs showing the HM and HM&HET motifs. (E) Percentage of HM and HM&HET among SSDs (202 pairs considered, yellow) and WGDs (260 pairs considered, blue) (left panel), homeologs that originated from inter-species hybridization (47 pairs annotated and considered, dark blue) (right panel) and true ohnologs from the whole-genome duplication (82 pairs annotated and considered, light blue). P-values are from Fisher’s exact tests. (F) Percentage of pairwise amino acid sequence identity between paralogs for HM and HM&HET motifs for SSDs and WGDs. P-values are from Wilcoxon tests. (G) Pairwise amino acid sequence identity for the full sequences of paralogs and their binding interfaces for the two motifs HM and HM&HET. P-values are from paired Wilcoxon tests. (H) Relative conservation scores for the two motifs of paralogs. Conservation scores are the percentage of sequence identity at the binding interface divided by the percentage of sequence identity outside the interface. Data shown include 30 interfaces for the HM group and 28 interfaces for the HM&HET group (22 homomers and 3 heterodimers of paralogs) (Supplementary file 2 Table S13). P-value is from a Wilcoxon test.
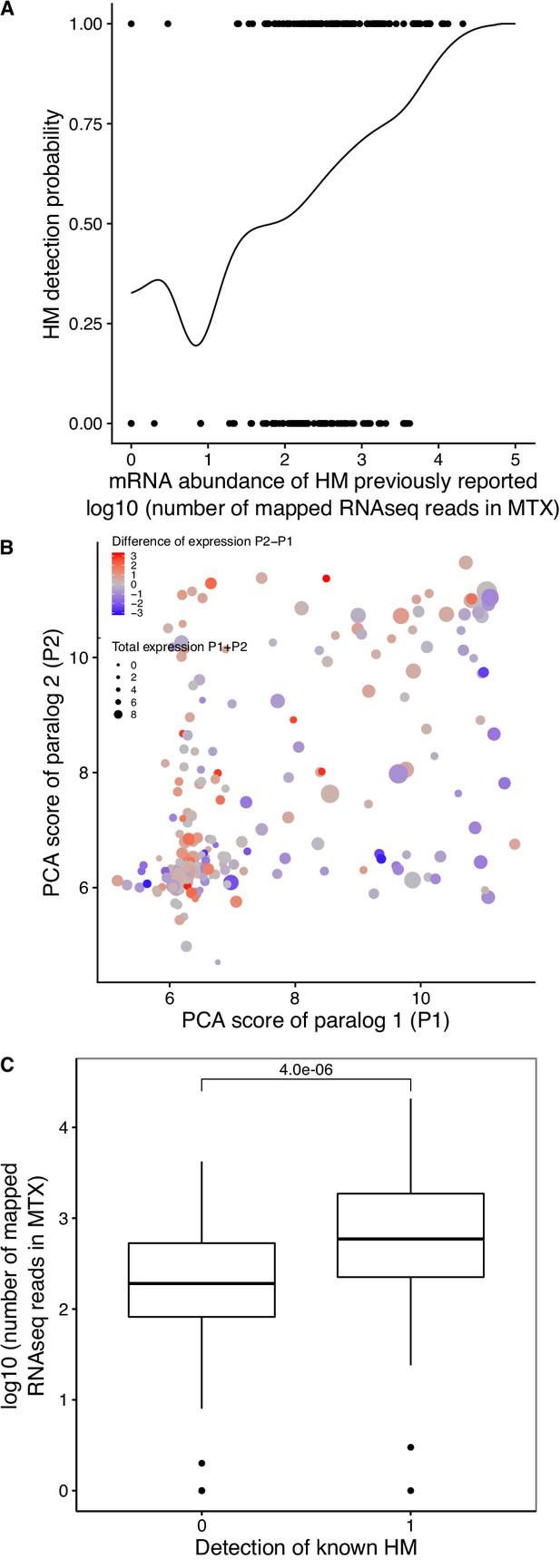
Association between mRNA abundance and the probability of HM detection by PCA in this study.
(A) The probability that PCA detects a HM is correlated with expression level, as estimated by RNAseq. The plot shows the detection probability of HMs as a function of mRNA abundance for previously reported HMs. Kernel regression of the HM detection (one for detected, 0 for not detected) on the number of mapped reads per gene (log10). (B) Difference in HM formation between paralogs results in part from their differential mRNA abundance. The PCA score of paralog 1 (P1) is compared to the PCA score of paralog 2 (P2). PCA scores are median colony sizes from the PCA experiments performed in this study. The total mRNA abundance of paralogs is shown by the size of the points and the difference of expression levels is represented by a color gradient (red for overexpression of P2 compared to P1 and blue overexpression of P1 compared to P2). Red points tend to be above the diagonal, blue points, below the diagonal. (C) Comparison of expression levels of previously reported HMs for HMs undetected and detected in the PCA experiments performed in this study. P-value from a Wilcoxon test is shown.
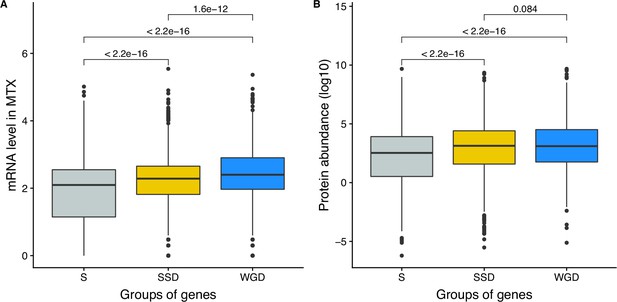
mRNA and protein abundance of singletons and duplicates.
(A) Comparison of mRNA abundance of genes as a function of whether they rare duplicated and of their type of duplication. (B) Comparison of the protein abundance as a function of whether they rare duplicated and their type of duplication. (S: singleton, SSD: Small-Scale Duplicates, WGD: Whole-Genome Duplicates). Numbers indicate p-values from Wilcoxon tests.
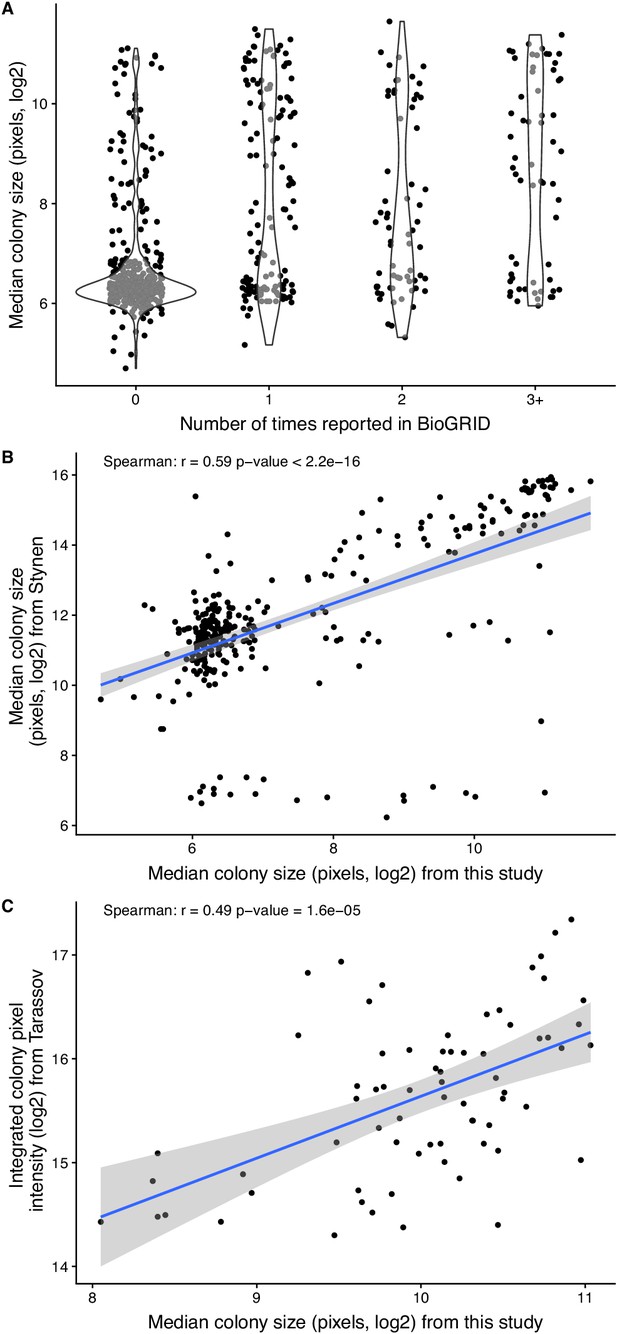
Comparison of PCA data generated in this study with published data.
(A) Colony size (estimated as the integrated pixel intensity) in the PCA experiment as a function of the number of times the corresponding interaction is reported in BioGRID version BIOGRID-3.5.166 (Chatr-Aryamontri et al., 2013; Chatr-Aryamontri et al., 2017). (B) Correlation between colony size of the study of Stynen et al. (2018) on homomers and of the PCA experiment performed in this study. (C) Correlation between colony size of Tarassov et al. (2008) and of the PCA experiment performed in this study.
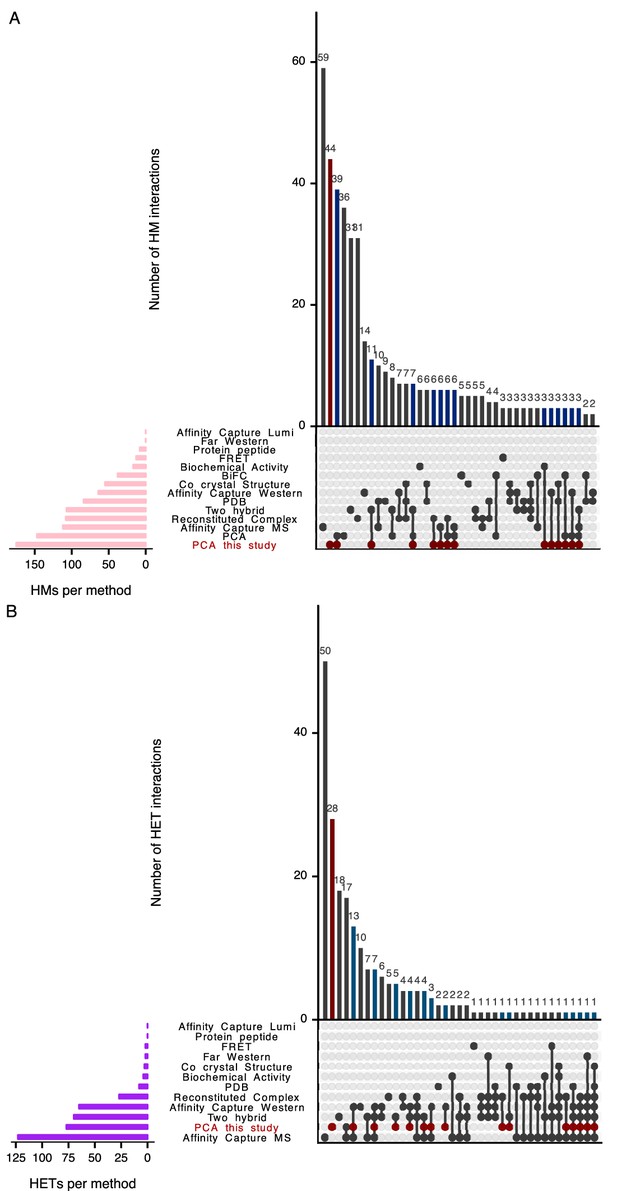
Intersections of detected HMs.
(A) and HETs (B) from this study and previously reported HMs and HETs. We considered HMs and HETs reported in crystal structures from the Protein Data Bank on September 21st, 2017 (Berman et al., 2000) and by PCA based on fluorescent proteins (BiFC) (Kim et al., 2019). We also include HMs and HETs reported in BioGRID (BIOGRID-3.5.166; Chatr-Aryamontri et al., 2013; Chatr-Aryamontri et al., 2017) with these methods: Affinity Capture-MS, Affinity Capture-Western, Reconstituted Complex, Two-hybrid, Biochemical Activity, Co-crystal Structure, Far Western, FRET, Protein-peptide, Affinity Capture-Luminescence and PCA. We added data from Stynen et al. (2018) to the BioGRID PCA data. Results of the PCA experiments from this study are highlighted in red. Turquoise-blue bars show HMs and HETs detected in this study and previously observed. The intersections were computed and plotted using the R package UpSetR (Lex et al., 2014).
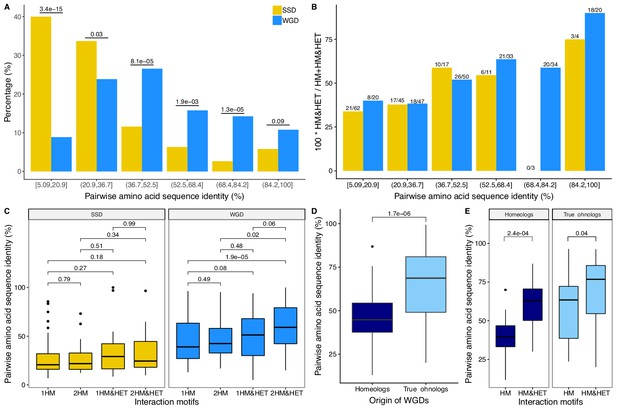
Interaction motifs and percentage of pairwise amino acid sequence identity between paralogs.
(A) Pairs of paralogs were clustered in six pairwise amino acid sequence identity groups and the distribution (in percentage) of these groups were compared between SSD and WGD. P-values are from Fisher’s exact tests. (B) The percentage of paralog pairs forming HM&HET among the total number of paralog pairs forming at least one HM (HM and HM&HET) is shown as a function of the percentage of pairwise amino acid sequence identity (SSDs in yellow and WGDs in blue). For each group, the number of HM&HET pairs and the total number are indicated above the bars. (C) Percentage of pairwise amino acid sequence identity between paralogs for each motif. 1HM: shows one homomer only, 2HM: shows both homomers, 1HM&HET: shows one homomer and the heteromer, and 2HM&HET: shows both homomers and the heteromer. P-values are from Wilcoxon tests. (D) The percentage of pairwise amino acid sequence identity among homeologs (dark blue) and true onhologs (light blue). P-value is from a Wilcoxon test. (E) Percentage of pairwise amino acid sequence identity between paralogs for HM and HM&HET motifs for homeologs and true ohnologs. P-values are from Wilcoxon tests.
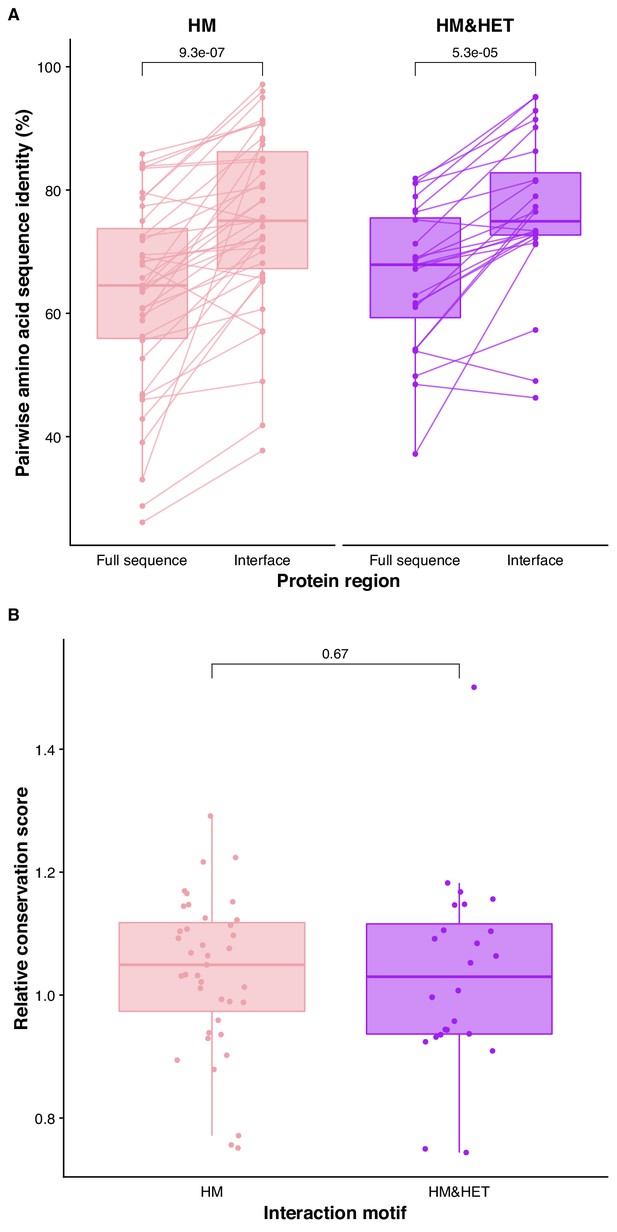
Conservation of binding interfaces of human paralogs in HM&HET complexes with solved structures.
(A) Pairwise amino acid sequence identity for the full sequences of paralogs and their interfaces are shown for the two motifs). P-values from paired Wilcoxon tests are shown. (B) Relative conservation scores are shown for the two motifs of paralogs. Relative conservation scores are calculated based on the protein regions solved by crystallography as the percentage of sequence identity at the binding interface divided by the percentage of sequence identity outside the interface. Paralog pairs were classified as HM or HM&HET according to the dataset compiled in Supplementary file 2 Table S14. Homologous interfaces were identified in alignments of the paralogous sequences. Supplementary file 2 Table S13 contains the list of PDB IDs used for these analyses, which include 40 interfaces from homomeric structures for the HM group and 25 interfaces for the HM&HET group (24 homomers and 1 heterodimer of paralogs). P-value is from a Wilcoxon test.
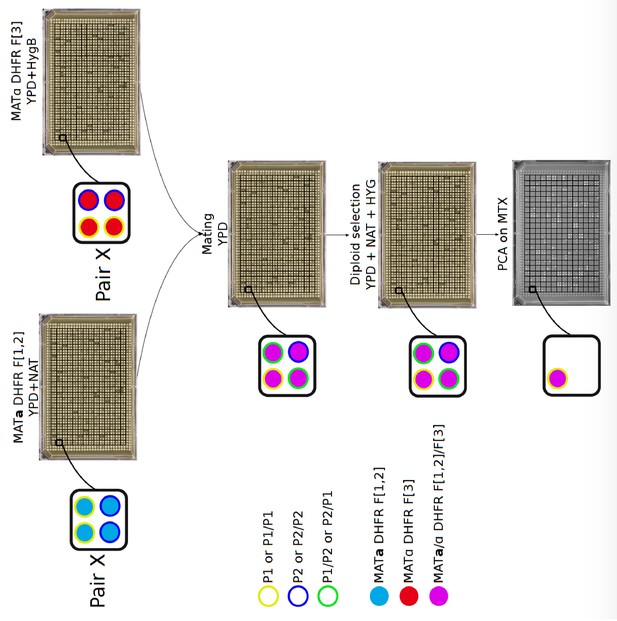
Plate organization for DHFR PCA experiments.
On the haploid arrays (MATa and MATα), each plate has two rows and two columns of control strains at the border (blue lines). Paralogs of a pair are positioned in blocks of four strains. A given pair (example here of pair X) occupies the same position in the MATa and MATα plates. Inside a square, paralogs are positioned horizontally in MATa DHFR F[1,2] plates (P1 are at the top and P2 at the bottom of the square) while they are vertically positioned in MATα DHFR F[3] plates (P1 are at the left and P2 at the right of the square). The two haploid plates were printed on top of each other on a mating plate, generating the following crosses: P1-DHFR F[1,2]/P1 DHFR F[3] at top left, P1-DHFR F[1,2]/P2 DHFR F[3] at top right, P2-DHFR F[1,2]/P1 DHFR F[3] at bottom left and P2-DHFR F[1,2]/P2 DHFR F[3] at bottom right. Two diploid selections and two replications on MTX medium were performed.
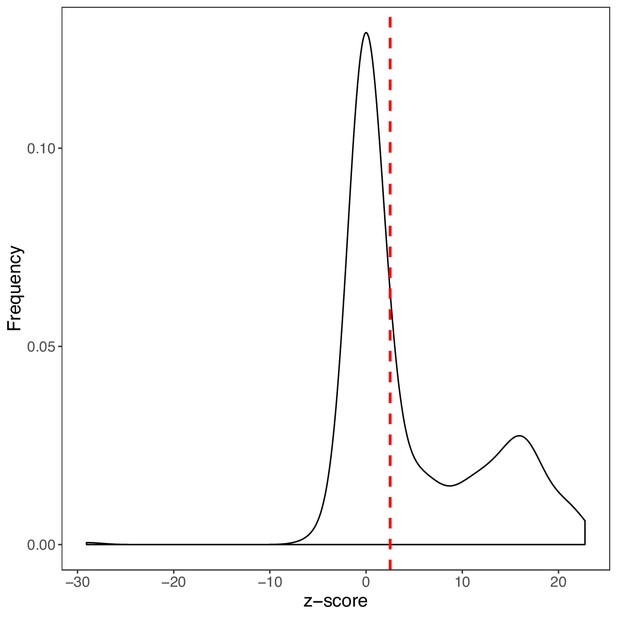
Density of colony size converted to z-score.
Colony sizes from the PCA experiment of this study were converted to z-score using the mean (μb) and standard deviation (sdb) of the background distribution (Zs = (Is - μb)/sdb)). The density of z-scores is shown in black. A protein-protein interaction was considered as detected if the corresponding z-score was larger than 2.5 (red dashed line).
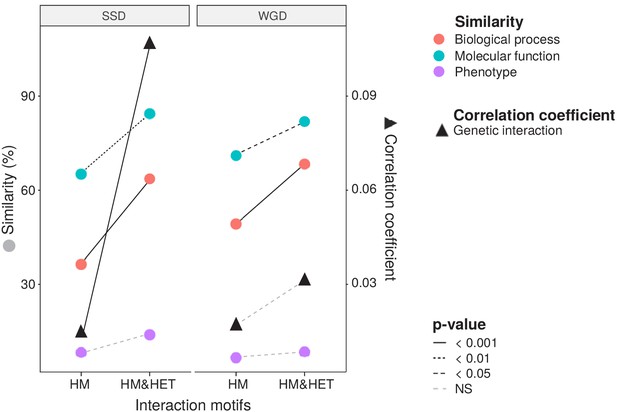
Maintenance of heteromerization between paralogs leads to greater functional similarity.
The similarity score is the average proportion of shared terms (100% * Jaccard's index) across pairs of paralogs for GO molecular functions, GO biological processes and gene deletion phenotypes. The mean values of similarity scores and of the correlation of genetic interaction profiles are compared between HM and HM&HET pairs for SSDs and WGDs. P-values are from Wilcoxon tests.
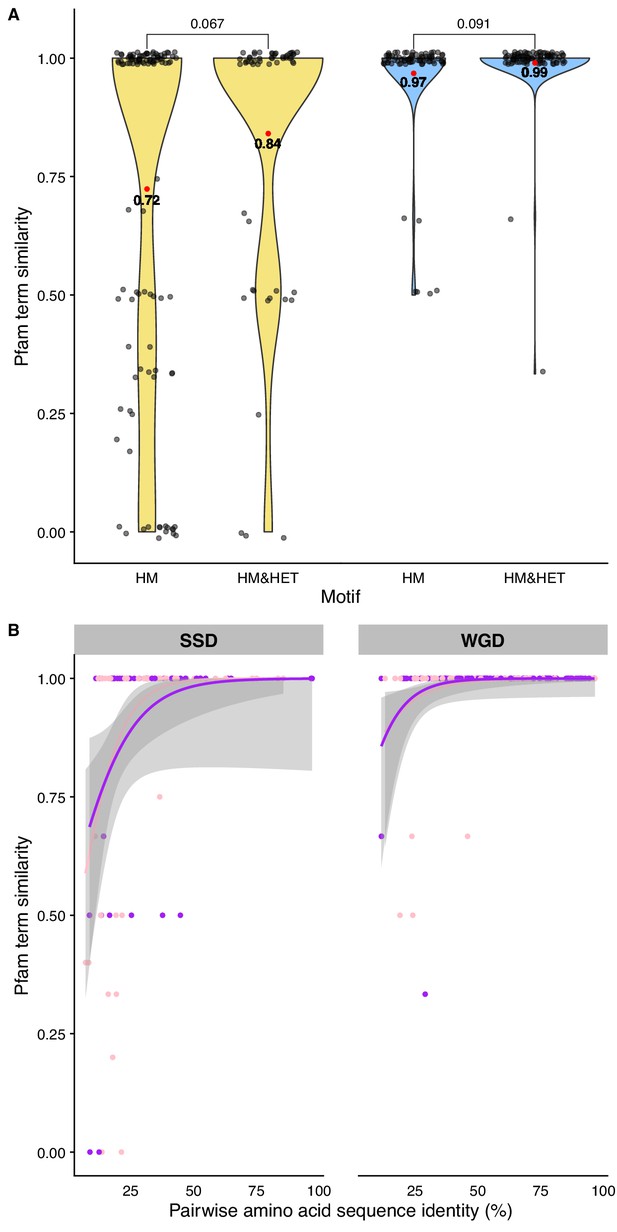
Comparison of Pfam domain composition similarity between pairs of paralogs.
(A) Pfam domain composition similarity (Jaccard’s index) between SSDs (yellow) and WGDs (blue) for each interaction motif (HM or HM&HET). (B) Pfam domain composition similarity as a function of pairwise amino acid sequence identity for HM motifs (pink) and HM&HET motifs (purple). Regression lines were smoothed using the GLM function with the quasibinomial family.
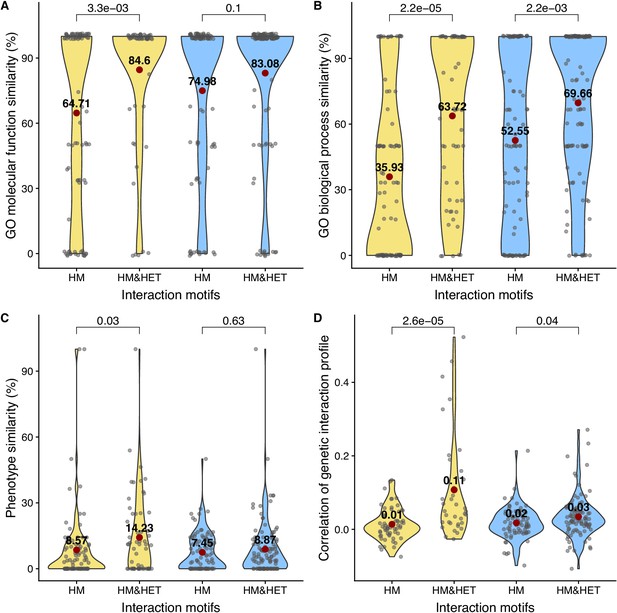
Comparison of functional similarity between HM and HM&HET pairs.
The similarity of function (100% * Jaccard’s index) between SSDs (yellow) and WGDs (blue) was estimated using GO terms for (A) molecular functions and for (B) biological processes. The similarity of function was also estimated using (C) growth phenotypes and (D) the correlation of genetic interaction profiles. P-values are from Wilcoxon tests.
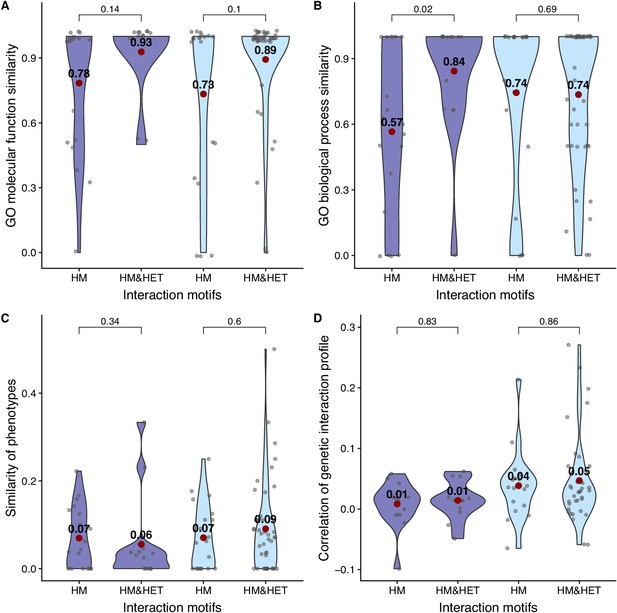
Comparison of functional similarity between WGDs, considering homeologs and true ohnologs separately.
The similarity of function (100% * Jaccard’s index) between homeologs (dark blue) and true ohnologs (light blue) was estimated using GO terms for (A) molecular functions and for (B) biological processes. The similarity of functions was also estimated using (C) growth phenotypes and (D) the correlation of genetic interaction profiles. P-values are from Wilcoxon tests.
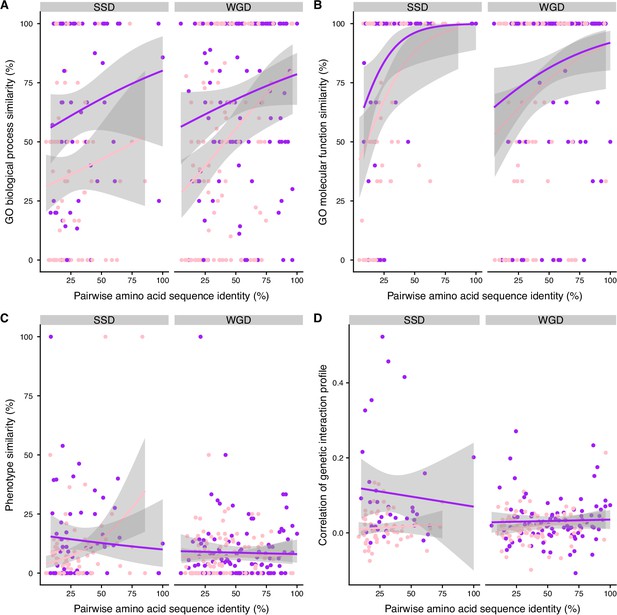
Functional similarity between paralogs as a function of their pairwise amino acid sequence identity.
The similarity of function (100% * Jaccard’s index) between paralogs for HM (pink) and HM&HET (purple) as a function of pairwise amino acid sequence identity for SSDs and WGDs. Similarity of function was estimated using (A) molecular functions and (B) biological processes GO terms, (C) growth phenotypes and (D) the correlation of genetic interaction profiles. The regression lines were smoothed using the R geom_smooth function.
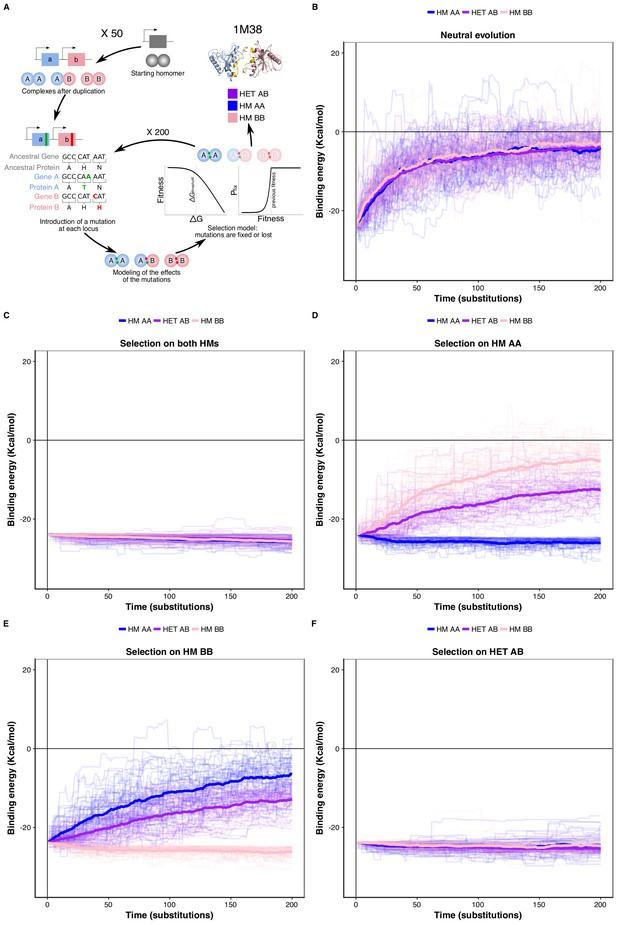
Negative selection to maintain homomers also maintains heteromers.
(A) The duplication of a gene encoding a homomeric protein and the evolution of the complexes is simulated by applying mutations to the corresponding subunits A and B. Only mutations that would require a single nucleotide change are allowed. Stop codons are disallowed. After introducing mutations, the selection model is applied to complexes and mutations are fixed or lost. (B to F) The binding energy of the HMs and the HET resulting from the duplication of a HM (PDB: 1M38) is followed through time under different selection regimes applied on protein stability and binding energy. More positive values indicate less favorable binding and more negative values indicate more favorable binding. (B) Accumulation and neutral fixation of mutations. (C) Selection on both HMs while the HET evolves neutrally. (D) Selection on HM AA or (E) HM BB: selection maintains one HM while the HET and the other HM evolve neutrally. (F) Selection on HET while the HMs evolve neutrally. (E) Selection on HM AA or (F) HM BB: selection maintains one HM while the HET and the other HM evolve neutrally. Mean binding energies among replicates are shown in thick lines and the individual replicates are shown with thin lines. Fifty replicate populations are monitored in each case and followed for 200 substitutions. PDB structure 1M38 was visualized with PyMOL (Schrödinger LLC, 2015). The number of substitutions that are fixed on average during the simulations are shown in Supplementary file 2 Table S8.
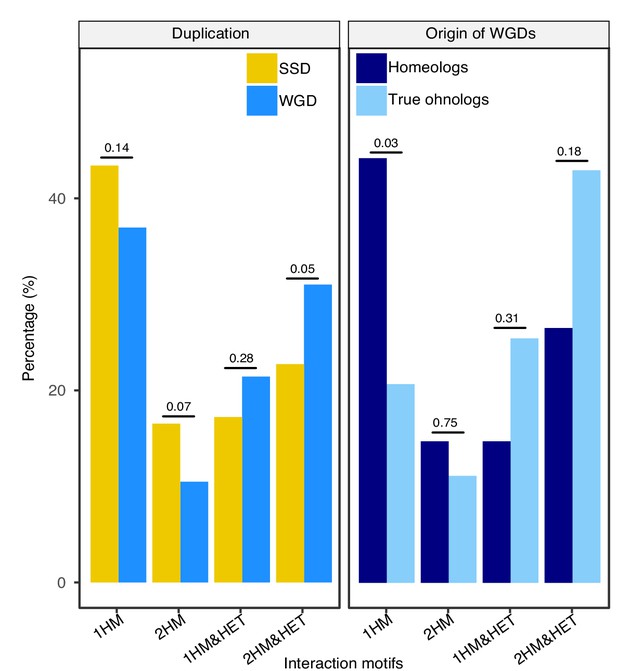
Percentage of interaction motifs for SSDs, WGDs and the two types of WGDs.
The data is the same as shown in Figure 2 but all four possible HM and HM&HET motifs are shown. 1HM: shows one homomer only, 2HM: shows both homomers, 1HM&HET: shows one homomer and the heteromer and 2HM&HET: shows both homomers and the heteromer. The percentage of motifs of interaction for SSDs (yellow) and WGDs (blue) (left panel) and for homeologs (dark blue) and true ohnologs (light blue) (right panel). P-values are from Fisher’s exact tests.
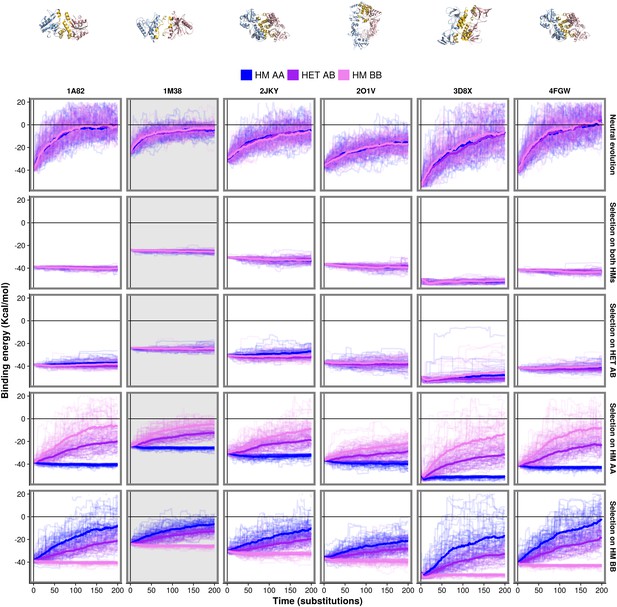
Similar evolutionary trajectories are observed for six different PDB structures.
The binding energy of six HMs and HETs is followed through time under the same scenarios as shown in Figure 4. Panels shown in Figure 4 are highlighted with a gray background here.
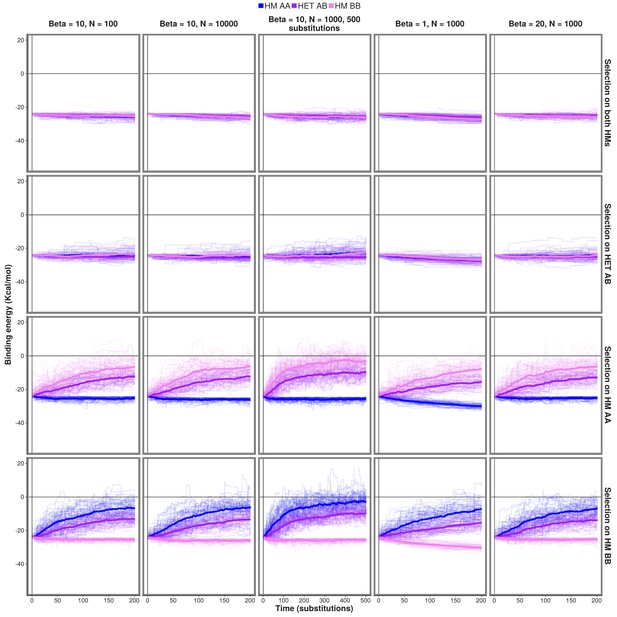
Effect of changes in parameters on the observed evolution trajectories.
Simulations were run for different combinations of parameters controlling the efficiency of selection ( and ) and the length of the simulations for PDB structure 1M38.
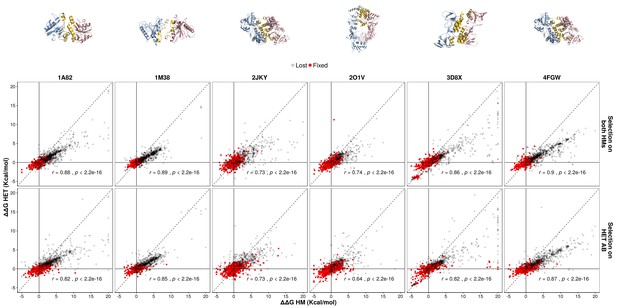
Single mutants have pleiotropic effects for HM and HET.
The observed effects of sampled single mutants on the HET are compared with their effects on HMs. Pearson's correlation coefficients are shown. Parameters used for and were 10 and 1000, respectively.
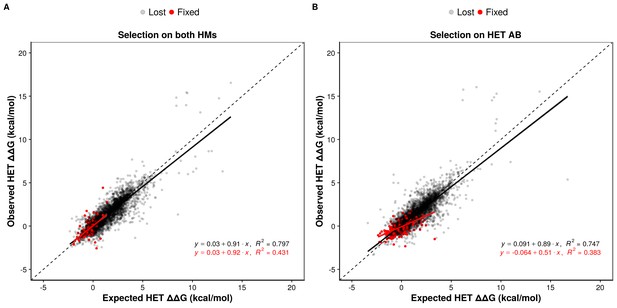
Epistasis favors the maintenance of HETs and the loss of HMs.
(A and B) Observed effects of double mutants on HET (y-axis) are compared to their expected effects (x-axis) based on the average of their effects on the HMs when selection is applied on both HMs (n = 6777 pairs of mutations) (A) or on the HET (n = 6760 pairs of mutations) (B). Dashed lines indicate the diagonal for perfect agreement between observations and expectations (no epistasis), black regression lines indicate the best fit for the lost mutants, and red regression lines indicate the best fit for the fixed mutants. Data were obtained from simulations with PDB structure 1M38. The regression coefficients, intercepts and R2 values are indicated on the figure for fixed and lost mutations. A regression coefficient lower than one means that pairs of mutations have a less destabilizing effects on the HET than expected based on their average effects on the HMs.
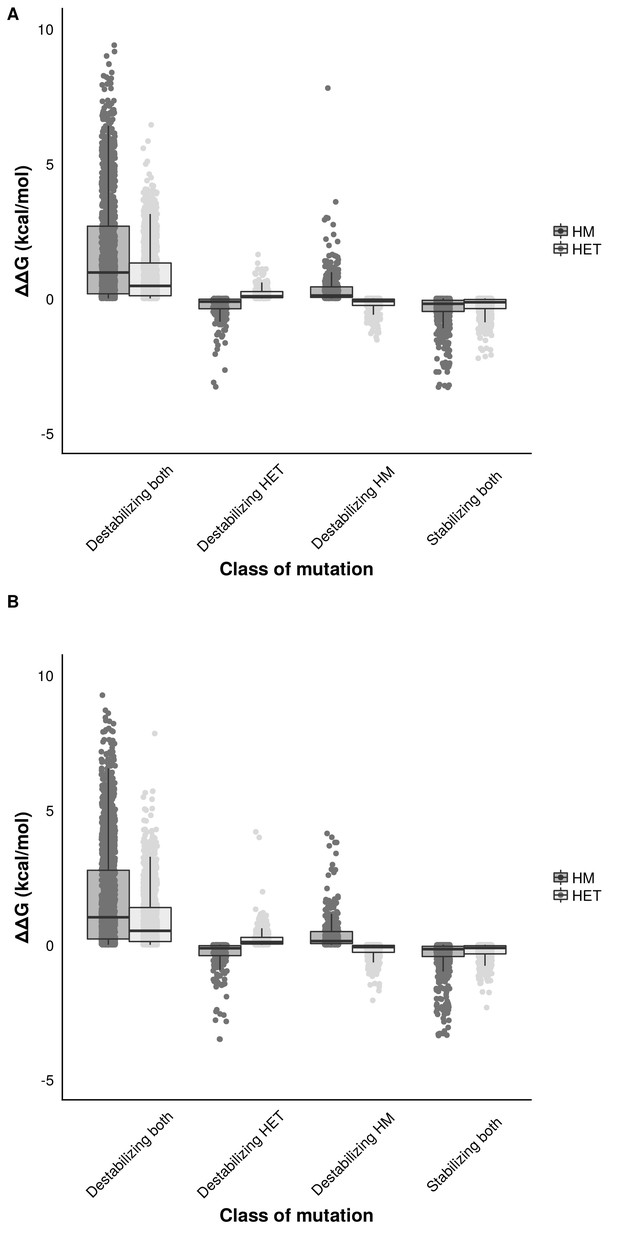
Distribution of effect sizes of mutations on the binding energy (ΔΔG) of HMs and HETs as estimated using FoldX.
Effects of single mutants on the binding energy of HMs and HETs. Mutants were classified (x-axis) according to their effects on the binding energy of HMs and HETs, depending on whether they stabilize or destabilize both the HM and the HET or they only destabilized one of them. Mutations that destabilize one of the complexes have smaller effect sizes on binding energy than mutations that destabilize or stabilize both. (A) Mutations sampled when negatively selecting for the stability of both HMs. (B) Mutations sampled when negatively selecting for the stability of the HET. Parameters used for and were 10 and 1000, respectively.
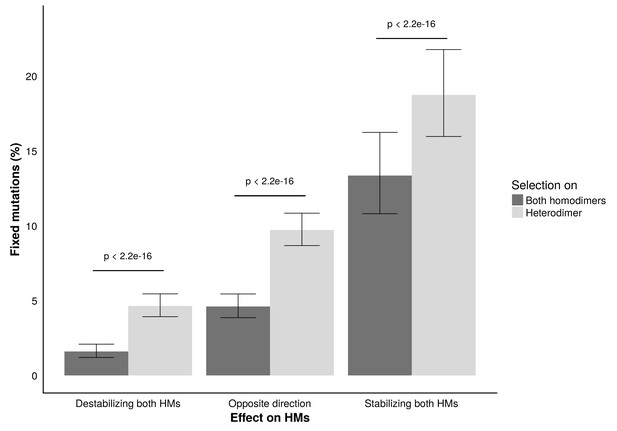
Fixation rates of double mutants during the simulations.
Fixation rates of double mutants classified based on their effect on the two HMs and the complexes (both HMs or HET) under selection. Clopper-Pearson 95% confidence intervals are shown. P-values were calculated with a two proportion z-test. Parameters used for and were 10 and 1000, respectively.
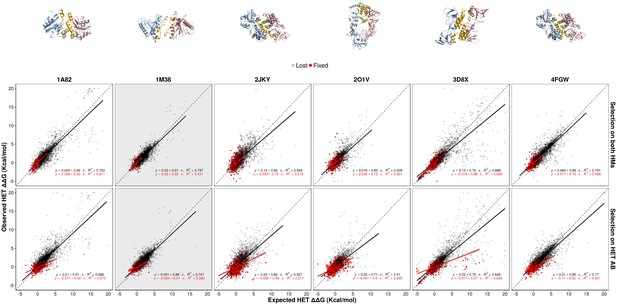
Contribution of epistasis to the evolution of HET for six different PDB structures.
The observed effects of double mutants on the HET are compared with their expected effects based on the effects on the HMs throughout the simulations. Simulations were run under the same scenarios shown in Figure 5. Panels shown in Figure 5 are highlighted with a gray background. Red points are for mutations that were fixed, gray ones those that were eliminated by selection. The regression equations are shown for fixed and lost mutations separately. Parameters used for and were 10 and 1000, respectively.
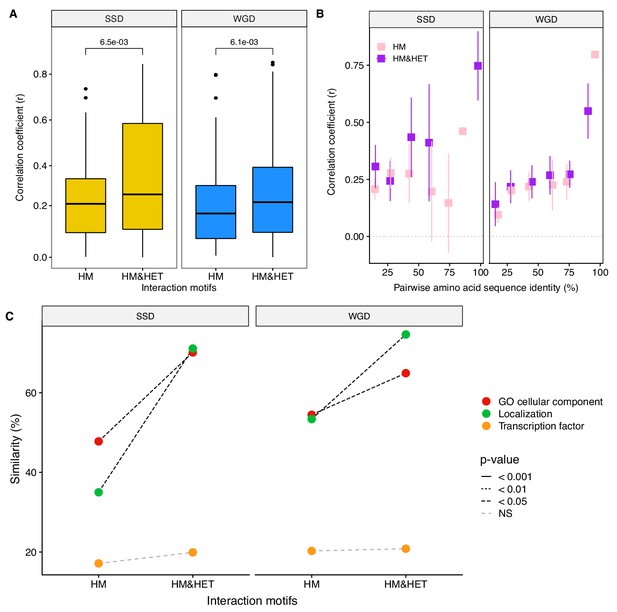
Loss of heteromerization between paralogs may result from regulatory divergence.
(A) Correlation coefficients (Spearman’s r) between the expression profiles of paralogs. The data derives from mRNA relative expression across 1000 growth conditions (Ihmels et al., 2004). HM and HM&HET are compared for SSDs (yellow) and WGDs (blue). P-values are from t-tests. (B) Correlation of expression profiles between paralogs forming only HM (pink) or HM&HET (purple) as a function of their amino acid sequence identity. The data was binned into six equal categories for representation only. (C) Similarity of GO cellular component, GFP-based localization, and transcription factor binding sites (100% * Jaccard’s index) are compared between HM and HM and HET for SSDs and WGDs. P-values are from Wilcoxon tests.
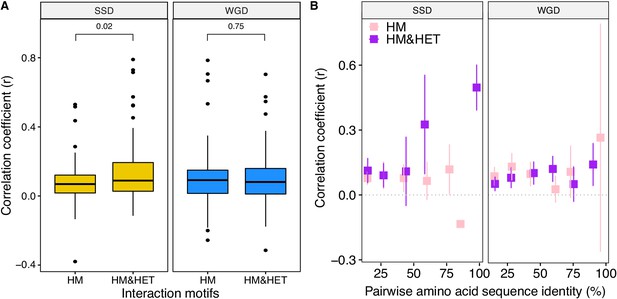
The loss of HETs may result from regulatory divergence (single cell RNAseq data; Gasch et al., 2017).
(A) Correlation (Spearman's r) between the expression profile of paralogs are compared among the different interaction motifs for SSDs (yellow) and WGDs (blue). P-values are from t-tests. (B) Correlation of expression profiles between paralogs forming only HM (pink) or HM&HET (purple) as a function of their pairwise amino acid sequence identity.
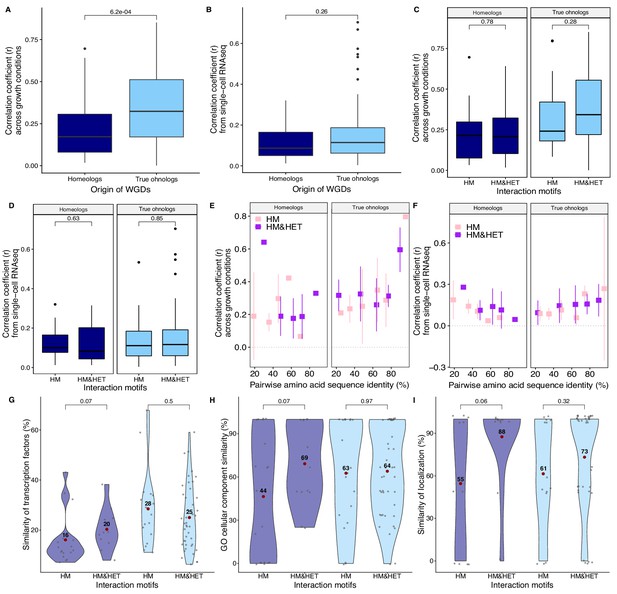
Expression of WGDs and consequences on interaction motifs.
Correlation coefficients (Spearman’s r) between the expression profiles of paralogs (A) from mRNA relative expression across 1000 growth conditions (Ihmels et al., 2004) and (B) from single-cell RNAseq (Gasch et al., 2017) are compared between homeologs and true ohnologs. Correlation coefficients (Spearman’s r) (C) across growth conditions and (D) from single-cell RNAseq data (Gasch et al., 2017) are compared among the different interaction motifs for homeologs and true ohnologs. Correlation coefficients (E) across growth conditions and (F) from single-cell RNAseq as a function of the percentage of pairwise amino acid sequence identity between paralogs forming only HM or HM&HET. (G) Similarity of transcription factor binding sites (100% * Jaccard’s index). (H) Similarity of GO cellular components. (I) Similarity of localization. P-values are from Wilcoxon tests.
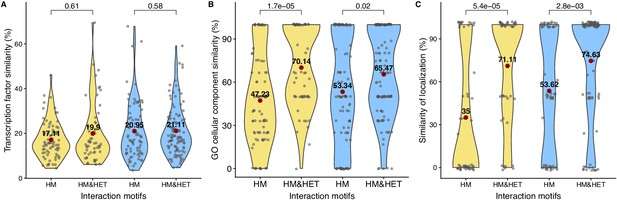
Interaction motifs and similarity of functions for SSDs and WGDs.
The similarity of regulation (100% * Jaccard’s index) for (A) transcription factor binding sites, (B) GO cellular components and (C) localization. P-values are from Wilcoxon tests.
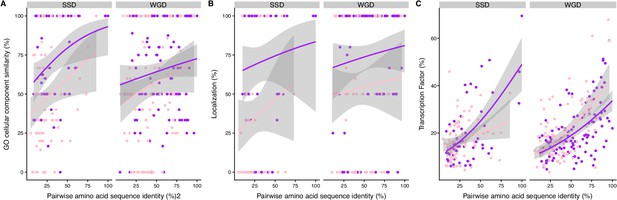
Similarity of regulation between paralogs as a function of their pairwise amino acid sequence identity.
The similarity of co-expression of HM (pink) and HM&HET (purple) pairs was compared while controlling for pairwise amino acid sequence identity for both SSD and WGD. Similarity of co-expression was estimated using (A) cellular component similarity GO term, (B) similarity of localization and (C) similarity of transcription factor binding sites. The regression lines were smoothed using glm method with quasibinomial family.
Tables
Reagent type (species) or resource | Designation | Source or reference | Identifiers | Additional information |
---|---|---|---|---|
Strain, strain background (Saccharomyces cerevisiae) | Yeast Protein Interactome Collection - DHFR F[1,2] and DHFR F[3] strains, BY4741 and BY4742 (MATa and MATα) | GE Healthcare Dharmacon Inc, Tarassov et al., 2008 | Cat. #YSC5849 | See Supplementary file 2 Tables S9 and S10 for the complete list of strains |
Strain, strain background (Saccharomyces cerevisiae) | DHFR F[1,2] strains, BY4741 (MATa) | Diss et al., 2017 and this paper | See Supplementary file 2 Tables S9 and S10 for the complete list of strains | |
Strain, strain background (Saccharomyces cerevisiae) | DHFR F[3] strains, BY4742 (MATα) | Diss et al., 2017 and this paper | See Supplementary file 2 Tables S9 and S10 for the complete list of strains | |
Strain, strain background (Saccharomyces cerevisiae) | RY1010, PJ69-4A (MATa) | Yachie et al., 2016 | ||
Strain, strain background (Saccharomyces cerevisiae) | RY1030, PJ69-4alpha (MATα) | Yachie et al., 2016 | ||
Strain, strain background (Saccharomyces cerevisiae) | YY3094, PJ69-4A (MATa) | This paper – available from Christian Landry upon request | ||
Strain, strain background (Saccharomyces cerevisiae) | YY3095, PJ69-4alpha (MATα) | This paper – available from Christian Landry upon request | ||
Strain, strain background (Lachancea kluyveri) | Lachancea kluyveri, CBS 3082 | Kurtzman, 2003 | ||
Strain, strain background (Zygosaccharomyces rouxii) | Zygosaccharomyces rouxii, CBS 732 | Pribylova et al., 2007 | ||
Strain, strain background (Escherichia coli) | MC1061 | CGSC | Cat. #6649 | |
Recombinant DNA reagent | pAG25-linker-F[1,2]-ADHterm (plasmid) | Tarassov et al., 2008 | ||
Recombinant DNA reagent | pAG32-linker-F[3]-ADHterm (plasmid) | Tarassov et al., 2008 | ||
Recombinant DNA reagent | pDEST-AD (TRP1) (plasmid) | Rual et al., 2005 | ||
Recombinant DNA reagent | pDEST-DB (LEU2) (plasmid) | Rual et al., 2005 | ||
Recombinant DNA reagent | pDN0501 (TRP1) (plasmid) | This paper – available from Christian Landry upon request | ||
Recombinant DNA reagent | pDN0502 (LEU2) (plasmid) | This paper – available from Christian Landry upon request | ||
Recombinant DNA reagent | pHMA1001 (TRP1)(plasmid) | This paper – available from Christian Landry upon request | ||
Recombinant DNA reagent | pHMA1003 (LEU2) (plasmid) | This paper – available from Christian Landry upon request | ||
Recombinant DNA reagent | pDEST-DHFR F[1,2] (TRP1) (plasmid) | This paper – available from Christian Landry upon request | ||
Recombinant DNA reagent | pDEST-DHFR F[1,2] (LEU2) (plasmid) | This paper – available from Christian Landry upon request | ||
Recombinant DNA reagent | pDEST-DHFR F[3] (TRP1) (plasmid) | This paper – available from Christian Landry upon request | ||
Recombinant DNA reagent | pDEST-DHFR F[3] (LEU2) (plasmid) | This paper – available from Christian Landry upon request | ||
Recombinant DNA reagent | pDONR201 (plasmid) | Invitrogen | Cat. #11798–014 | |
Recombinant DNA reagent | PacI | New England BioLabs Inc | Cat. #R0547S | |
Recombinant DNA reagent | SacI | New England BioLabs Inc | Cat. #R0156S | |
Recombinant DNA reagent | SpeI | New England BioLabs Inc | Cat. #R0133S | |
Recombinant DNA reagent | PI-PspI | New England BioLabs Inc | Cat. #R0695S | |
Sequence-based reagent | Oligonucleotides | This paper | PCR primers | See Supplementary file 2 Table S12 for the complete list |
Sequence-based reagent | DEY011 | Integrated DNA Technologies, Inc | gBlock | See Supplementary file 2 Table S12 for the sequence |
Commercial assay or kit | Presto Mini Plasmid Kit | Geneaid Biotech Ltd | Cat. #PDH300 | |
Commercial assay or kit | Lexogen Quantseq 3’ mRNA kit | D-Mark Biosciences | Cat. #012.24A | |
Commercial assay or kit | Gateway BP Clonase II enzyme mix | Thermo Fisher Scientific | Cat. #11789020 | |
Commercial assay or kit | Gateway LR Clonase II enzyme mix | Thermo Fisher Scientific | Cat. #11791020 | |
Commercial assay or kit | Gibson Assembly Master Mix | New England BioLabs Inc | Cat. # E2611L | |
Chemical compound, drug | Kanamycin | BioShop Canada, Inc | Cat. #KAN201.10 | |
Chemical compound, drug | Ampicillin | BioShop Canada, Inc | Cat. #AMP201 | |
Chemical compound, drug | Nourseothricin (NAT) | WERNER BioAgents GmbH | Cat. #5.010.000 | |
Chemical compound, drug | Hygromycin B (HygB) | BioShop Canada, Inc | Cat. #HYG003 | |
Chemical compound, drug | Methotrexate (MTX) | BioShop Canada, Inc | Cat. #MTX440 | |
Software, algorithm | MUSCLE v 3.8.31 | Edgar, 2004 | RRID:SCR_011812 | |
Software, algorithm | gitter (R package version 1.1.1) | Wagih and Parts, 2014 | ||
Software, algorithm | normalmixEM function (R mixtools package) | Benaglia et al., 2009 | ||
Software, algorithm | FastQC | Andrews, 2010 | RRID:SCR_014583 | |
Software, algorithm | cutadapt | Martin, 2011 | RRID:SCR_011841 | |
Software, algorithm | bwa | Li and Durbin, 2009 | RRID:SCR_010910 | |
Software, algorithm | HTSeq (Python package) | Anders et al., 2015 | RRID:SCR_005514 | |
Software, algorithm | BLASTP (version 2.6.0+) | Camacho et al., 2009 | RRID:SCR_001010 | |
Software, algorithm | FoldX suite version 4 | Guerois et al., 2002 and Schymkowitz et al., 2005 | RRID:SCR_008522 | |
Software, algorithm | FreeSASA | Mitternacht, 2016 | ||
Software, algorithm | Biopython | Cock et al., 2009 | RRID:SCR_007173 | |
Other, database | IntAct | Orchard et al., 2014 | RRID:SCR_006944 | https://www.ebi.ac.uk/intact/ |
Other, database | Yeast Gene Order Browser (YGOB) | Byrne and Wolfe, 2005 | http://ygob.ucd.ie/ | |
Other, database | PhylomeDB | Huerta-Cepas et al., 2008 | RRID:SCR_007850 | http://phylomedb.org/ |
Other, database | Protein Data Bank (PDB) | Berman et al., 2000 | RRID:SCR_012820 | https://www.rcsb.org/ |
Other, database | Ensembl | Zerbino et al., 2018 | RRID:SCR_002344 | http://useast.ensembl.org/info/data/ftp/index.html |
Other, database | TheCellMap (version of March 2016) | Usaj et al., 2017 | http://thecellmap.org/ | |
Other, database | Saccharomyces Genome Database (SGD) | Cherry et al., 2012 | RRID:SCR_004694 | https://www.yeastgenome.org/ |
Other, database | Complex Portal | Meldal et al., 2015 | RRID:SCR_015038 | https://www.ebi.ac.uk/complexportal/ |
Other, database | CYC2008 catalog | Pu et al., 2009Pu et al., 2007 | http://wodaklab.org/cyc2008/ | |
Other, database | YEASTRACT | Teixeira et al., 2018, Teixeira et al., 2006 | RRID:SCR_006076 | http://www.yeastract.com/ |
Other, database | Yeast GFP Fusion Localization Database (YeastGFP) | Huh et al., 2003 | https://yeastgfp.yeastgenome.org/ | |
Other, database | The Protein Families Database (Pfam) | El-Gebali et al., 2019 | RRID:SCR_004726 | https://pfam.xfam.org/ |
Other, database | UniprotKB database | The UniProt Consortium, 2019 | RRID:SCR_004426 | https://www.uniprot.org/ |
Other, database | BIOGRID-3.5.166 | Chatr-Aryamontri et al., 2017, Chatr-Aryamontri et al., 2013 | RRID:SCR_007393 | https://thebiogrid.org/ |
Other, database | Ohnologs | Singh et al., 2015 | http://ohnologs.curie.fr/ | |
Other, dataset | Supplementary materials of Benschop et al. (2010) | Benschop et al., 2010 | https://doi.org/10.1016/j.molcel.2010.06.002 | |
Other, dataset | Supplementary materials of Kim et al. (2019) | Kim et al., 2019 | https://doi.org/10.1101/gr.231860.117 | |
Other, dataset | Supplementary materials of Ihmels et al. (2004) | Ihmels et al., 2004 | https://doi.org/10.1093/bioinformatics/bth166 | |
Other, dataset | Supplementary materials of Gasch et al. (2017) | Gasch et al., 2017 | https://doi.org/10.1371/journal.pbio.2004050 | |
Other, dataset | Supplementary materials of Guan et al. (2007) | Guan et al., 2007 | https://doi.org/10.1534/genetics.106.064329 | |
Other, dataset | Supplementary materials of Tarassov et al. (2008) | Tarassov et al., 2008 | https://doi.org/10.1126/science.1153878 | |
Other, dataset | Supplementary materials of Stynen et al. (2018) | Stynen et al., 2018 | https://doi.org/10.1016/j.cell.2018.09.050 | |
Other, dataset | Supplementary materials of Lan and Pritchard (2016) | Lan and Pritchard, 2016 | https://doi.org/10.1126/science.aad8411 |
Additional files
-
Supplementary file 1
Supplementary text on the performance of PCA as compared to other methods and descriptions of the supplementary tables.
- https://doi.org/10.7554/eLife.46754.031
-
Supplementary file 2
Supplementary tables for this work.
Table descriptions can be found in Supplementary file 1.
- https://doi.org/10.7554/eLife.46754.032
-
Transparent reporting form
- https://doi.org/10.7554/eLife.46754.033