Predictors of SIV recrudescence following antiretroviral treatment interruption
Figures
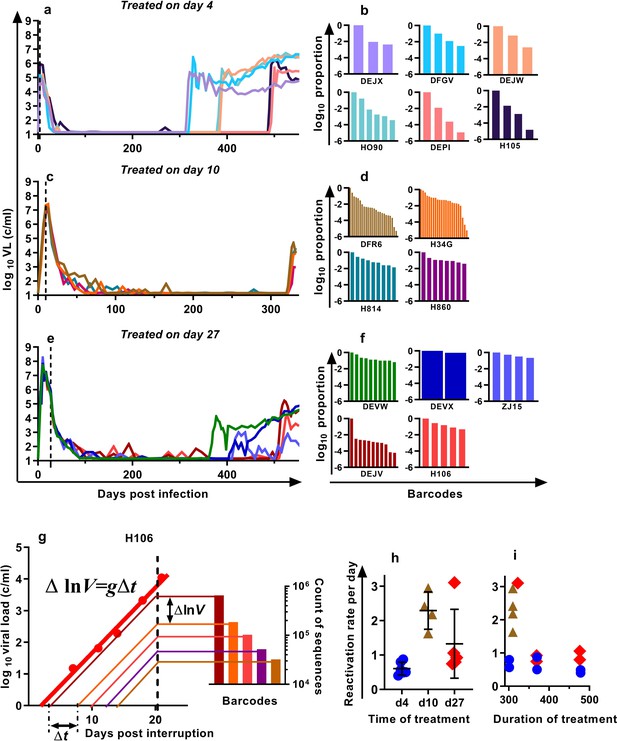
Viral load and frequency of reactivation after treatment interruption.
The viral load in individual animals infected with SIVmac239M and (a) treated on day four and interrupted on days 300 (n = 2), 370 (n = 2), or 478 (n = 2) and reproduced from Fennessey et al. (2017), or (c) treated day 10 and interrupted on day 320 (n = 4), or (e) treated on day 27, and interrupted on days 349 (n = 1), 396 (n = 2), or 503 (n = 2). Vertical dashed line is the time of treatment. (b,d,f) Frequency of barcode clonotypes identified by high throughput sequencing of plasma virus in individual monkeys during rebound. (g) Schematic of the method for estimation of the frequency of reactivation from latency, detailed in Fennessey et al. (2017). Red dots – measurements of total viral load. Thick red line is the trajectory of growth of total viral load. Coloured thin lines are the theoretical trajectories of growth of individual clonotypes based on the proportion of individual barcode sequences in the rebound plasma (bar graph at the right-hand side). Time between reactivations Δt is proportional to difference between logarithms of the frequency of barcodes. (h) The frequency of reactivation for the three cohorts initiating treatment on different days post-infection (bars indicate mean and SD) and (i) treated for different lengths of time.
-
Figure 1—source data 1
Viral kinetics, clonotype proportions, and reactivation frequencies for SIVmac239M infected animals.
- https://cdn.elifesciences.org/articles/49022/elife-49022-fig1-data1-v2.xlsx
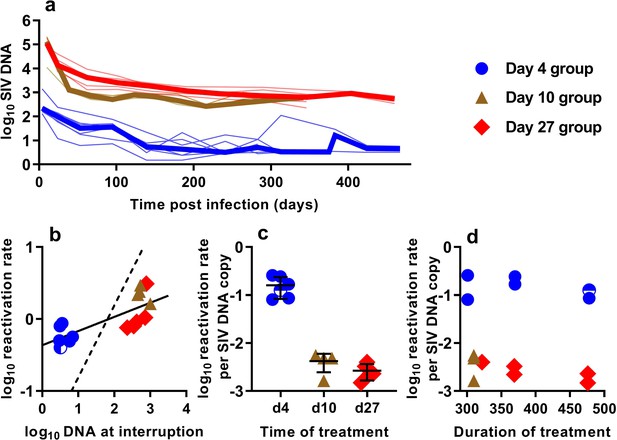
The relationship between SIV DNA and frequency of reactivation.
(a) The levels of SIV CA-DNA in individual animals (thin lines) were measured in peripheral blood. Median is shown as thick line. (b) The relationship between frequency of reactivation from latency and SIV DNA levels at cART interruption, for individual animals treated at different times after infection. Linear regression line fitted to log-log transformed data, dashed line is the regression with the fixed slope = 1. (c) The frequency of reactivation per DNA copy for animals treated on different days post-infection, and (d) the relationship between duration of treatment and reactivation per DNA copy. Some animals treated at day four had undetectable DNA levels at interruption. In this case, we can only estimate an upper/lower bound and the circle is only shaded at the side of lower DNA values.
-
Figure 2—source data 1
Kinetics of cell-associated SIV DNA and its relationship to SIV reactivation frequency.
- https://cdn.elifesciences.org/articles/49022/elife-49022-fig2-data1-v2.xlsx
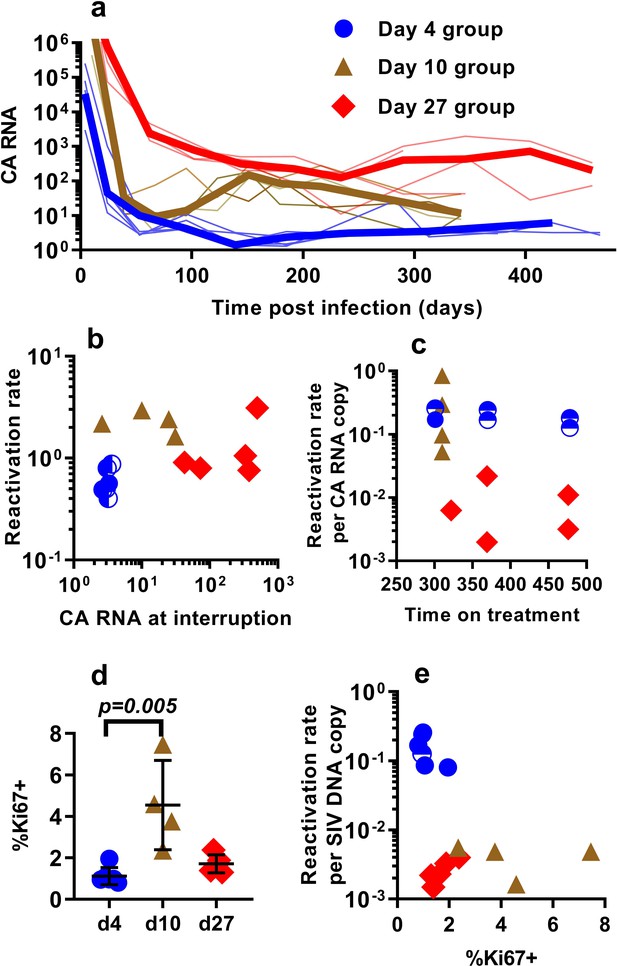
The relationship between CA-SIV-RNA, CD4+ T cell activation, and frequency of reactivation.
(a) The levels of CA-SIV RNA were measured over time in peripheral blood (thick line is the median). (b) The relationship between frequency of reactivation from latency and SIV RNA levels at interruption for individual animals treated at different times after infection. (c) The frequency of reactivation per RNA copy for animals treated on different days post-infection, according to the duration of treatment. (d) Immune activation was assessed based on expression of Ki67 in CD4+ T cells in whole blood just prior to interruption. There was a trend to higher Ki67 expression in animals treated on day 10 and 27, and (e) reactivation per SIV DNA copy was negatively correlated with expression of Ki67 (Spearman r −0.56, p=0.034). Some animals treated at day four had undetectable RNA levels at interruption. In this case, we can only estimate an upper/lower bound and the circle is only shaded at the side of possible lower DNA values. Ki67 analysis was performed on fresh cells for animals in the day 4 and day 27 groups, and frozen cells for animals in the days 10 group.
-
Figure 3—source data 1
Levels of cell-associated RNA and Ki67 expression and their association with reactivation frequency.
- https://cdn.elifesciences.org/articles/49022/elife-49022-fig3-data1-v2.xlsx
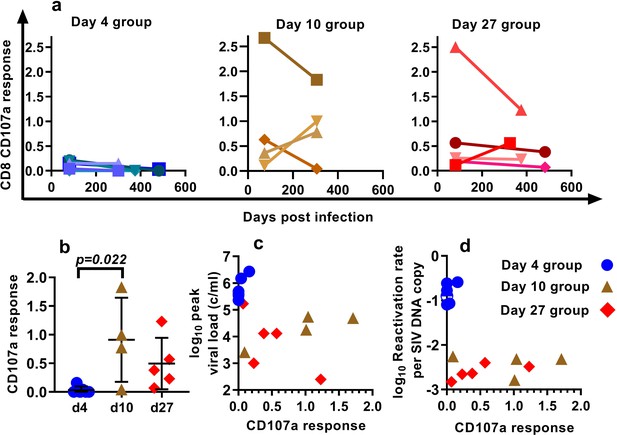
Effects of CD8+ T cell response to SIV peptides.
(a) The kinetics of the CD107a+ response in CD8+ T cells to pooled SIV peptides was measured in vitro using ICS (the sum of the responses to peptides from SIV ENV, GAG, POL and accessory (ACC) proteins is shown at the times indicated). (b) Animals treated beginning on day 10 and 27 have higher CD8+ T cell responses to pooled peptides (%CD107a+ cells shown) (Dunn's multiple comparisons test’s p=0.022, for day 4 (n = 6) vs. day 10 (n = 4); p=0.053, for day 4 (n = 6) vs day 27 (n = 5)). (c) Relationship between peak viral load after treatment interruption and the level of CD107a response to pooled peptides (Spearman r = −0.62, p=0.015, n = 15). For other responses and peptides see Figure 4—figure supplement 1. (d) The dependence of reactivation rate per SIV DNA at time of interruption on the level CD107a response to pooled peptides, for other responses and peptides see Figure 4—figure supplement 2, for reactivation rate vs. immune response see Figure 4—figure supplement 3.
-
Figure 4—source data 1
Relationship between immune response (CD8 cells expressing CD107a) and SIV reactivation.
- https://cdn.elifesciences.org/articles/49022/elife-49022-fig4-data1-v2.xlsx
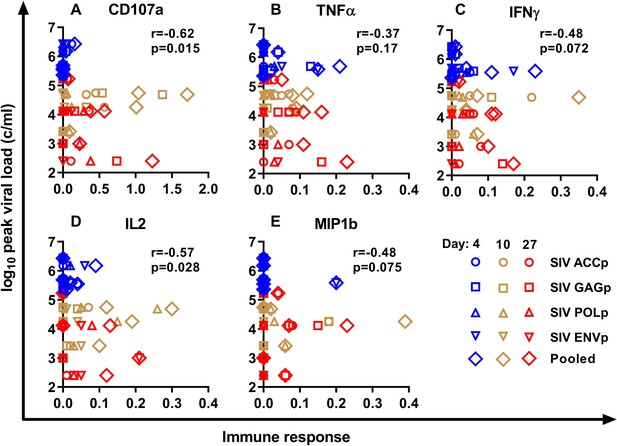
Effects of T cell response to SIV peptides on peak viremia after rebound.
Relationship between peak viral load after treatment interruption and the level of immune response to different peptides. Spearman r and p-value as a measure of correlation between response to pooled peptides and peak viral load presented in the corner of each panel.
-
Figure 4—figure supplement 1—source data 1
Relationship between T cell response and peak SIV viremia after rebound.
- https://cdn.elifesciences.org/articles/49022/elife-49022-fig4-figsupp1-data1-v2.xlsx
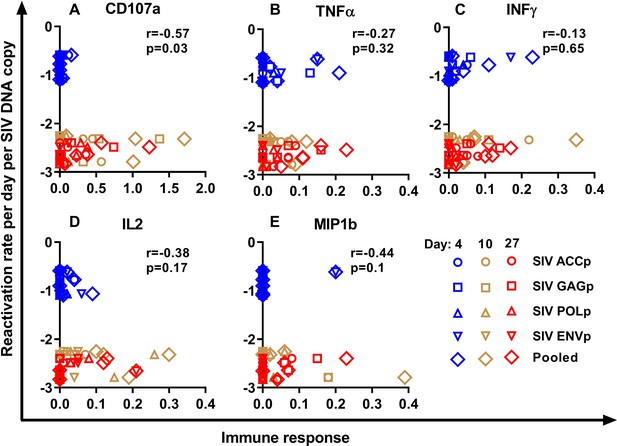
Effects of T cell response to SIV peptides on reactivation rate per SIV DNA copy.
Relationship between reactivation rate per SIV DNA at time of interruption and the level of immune response to different peptides. Spearman r and p-value as a measure of correlation between response to pooled peptides and reactivation rate per SIV DNA presented in the corner of each panel.
-
Figure 4—figure supplement 2—source data 1
Relationship between T cell response and SIV reactivation frequency per SIV DNA copy.
- https://cdn.elifesciences.org/articles/49022/elife-49022-fig4-figsupp2-data1-v2.xlsx
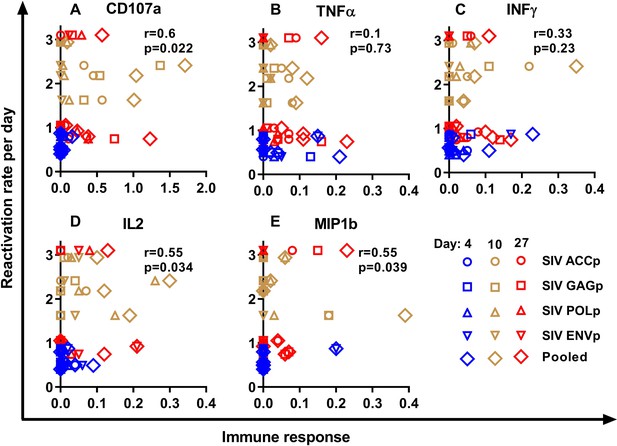
Effects of T cell response to SIV peptides on reactivation rate.
Relationship between reactivation rate at time of interruption and the level of immune response to different peptides. Spearman r and p-value as a measure of correlation between response to pooled peptides and reactivation rate presented in the corner of each panel. Higher immune response leads to higher reactivation rate since both are consequences of higher exposure to infection.
-
Figure 4—figure supplement 3—source data 1
Relationship between T cell response and SIV reactivation frequency.
- https://cdn.elifesciences.org/articles/49022/elife-49022-fig4-figsupp3-data1-v2.xlsx
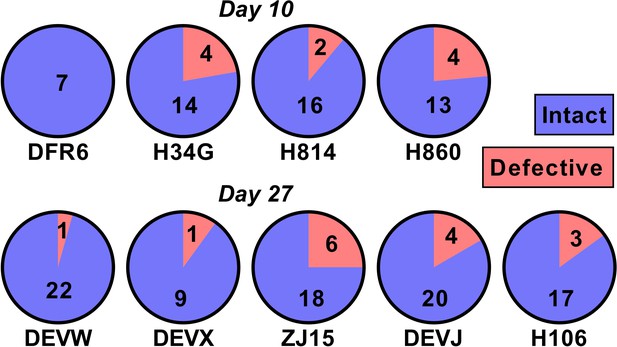
The majority of SIV DNA is intact.
Full length SIV DNA was sequenced from PBMC of macaques just prior to treatment interruption. The number of intact versus defective sequences observed in each animal is indicated. The proportion of intact sequences was similar for animals treated on day 10 (top row) versus day 27 (bottom row). Overall 84% of SIV genomes were found to be intact.
-
Figure 5—source data 1
Proportion of intact and defective SIV DNA.
- https://cdn.elifesciences.org/articles/49022/elife-49022-fig5-data1-v2.xlsx
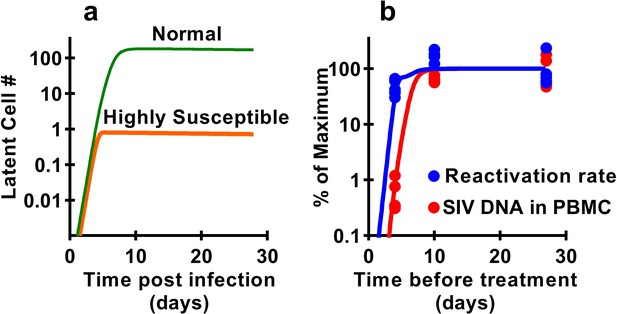
Modelling a subset of susceptible cells that are the major drivers of SIV reactivation.
The frequency of reactivation from latency has reached about half of its maximum in animals treated 4 days post-infection, whereas the level of SIV DNA continues to increase around 100-fold between day 4 and day 10 post-infection. We use a modelling approach to explore the dynamics of early infection and consider the possibility that there are different subsets of infected cells. For example, if we had a subset of cells which is both highly susceptible to infection, and has a high frequency of reactivation from latency, then these cells would both be infected first (orange curve in panel a), and would also make an out-sized contribution to the overall frequency of reactivation from latency. The less susceptible and quiescent pool (green curve in panel a) continues to ‘fill’ over time, but this has relatively little impact on later reactivation rates The frequency of reactivation and SIV DNA levels include contributions from both subsets. However, the small subset of highly active cells can make a major contribution to the frequency of reactivation, while having little effect on total DNA levels. For example, panel b shows experimental data (dots) and along with panel a illustrates that a theoretical subset of only 0.4% of cells that is 100-fold more susceptible to infection, and 500-fold more prone to reactivation, could explain the data on SIV DNA and SIV reactivation frequency.
Tables
Variables used in the multiple linear regression model.
Variable number | Variable name | Allowed sign of coeffiecient |
---|---|---|
1 | Treatment Duration | -ve |
2 | Log10(DNA at treatment) | +ve |
3 | Log10(DNA at interruption) | +ve |
4 | Log10(RNA at treatment) | +ve |
5 | Log10(RNA at interruption) | +ve |
6 | Log10(Viral Load at treatment) | -ve or +ve |
7 | Log10(Area Under curve of Viral load at treatment) | -ve or +ve |
8 | Log10(Maximum Viral Load) | -ve or +ve |
9 | CD107a | -ve |
10 | TNF | -ve |
11 | IFN | -ve |
12 | IL2 | -ve |
13 | MIP1β | -ve |
14 | Ki67 | +ve |
15 | HLA-DR | +ve |
16 | CD38 | +ve |