Humans can efficiently look for but not select multiple visual objects
Figures
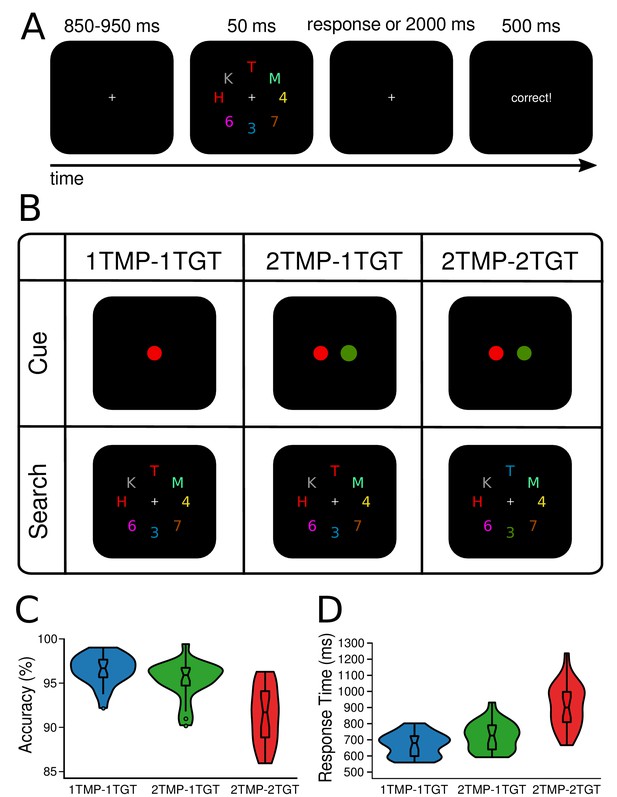
Design and behavioral results.
In all conditions, observers were required to select two target characters and determine whether they were of the same (i.e., both letters or both digits) or different category (i.e., letter and digit). (A) The target colors were cued once in the beginning of a block and stayed constant for the rest of the block (64 trials). A trial started with a fixation screen for a jittered interval of 850 to 950 ms, followed by the search display, presented for 50 ms and another fixation screen that lasted for up to 2000 ms or until participants responded. Depending on the response, feedback was presented for 500 ms (‘correct’, or ‘wrong’). If no response was given, a 10 s time out occurred and participants were urged to try responding quicker. (B) Task design. Depending on the condition, either one or two colors were cued to be task-relevant in the beginning of a block (creating one vs. two unique templates). Similarly, whenever two colors were cued, search displays could contain either one of them, or both (one vs. two unique target features). Thus, in the one-template-one-target-feature condition (1TMP–1TGT) one color was cued, and both targets carried this color in the search display, in the two-templates-one-target-feature condition (2TMP–1TGT) two colors were cued but only one of these colors was present in the search display with both targets carrying that color, and in the two-template-two-target-feature condition (2TMP–2TGT) two colors were cued and both colors were present in the search displays. One target always appeared on the horizontal meridian (above or below fixation), and the other target on the vertical meridian (to the left or right of fixation). (C) and (D) Behavioral results. The violin plots depict the distribution of (C) accuracy (see Figure 1—source data 1) and (D) response times (see Figure 1—source data 2) across participants, separately for the 1TMP–1TGT, 2TMP–1TGT, and 2TMP–2TGT conditions. The horizontal lines in the box plots represent quartiles. The vertical line represents the minimum (lower quartile - 1.5 * interquartile range) and maximum (upper quartile + 1.5 * interquartile range) while single dots beyond that range indicate individual outliers.
-
Figure 1—source data 1
Accuracy data across participant that are represented in Figure 1C.
- https://doi.org/10.7554/eLife.49130.003
-
Figure 1—source data 2
Response time data across participant that are represented in Figure 1D.
- https://doi.org/10.7554/eLife.49130.004
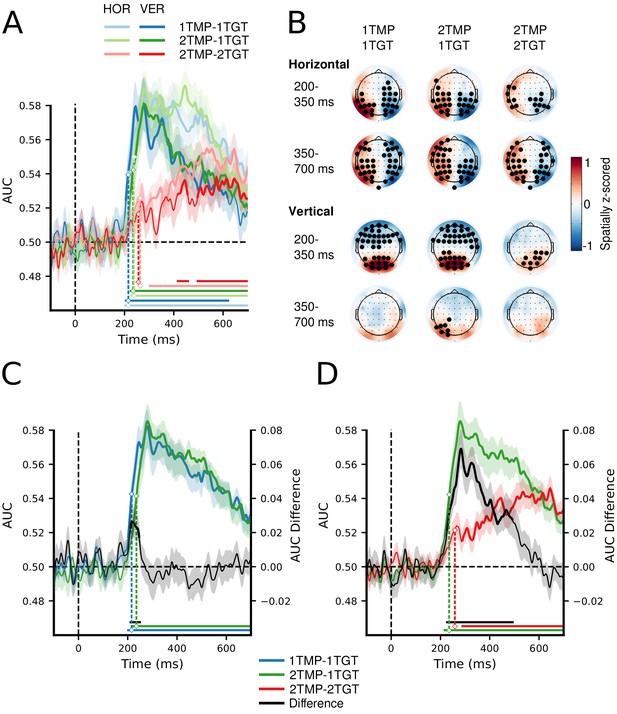
MVPA decoding performance for target position.
(A) Decoding performance expressed as Area Under the Curve (AUC) for target position on the horizontal (left vs. right) and on the vertical meridian (top vs. bottom) separately, as a function of number of templates and number of target features. See also Figure 2—source data 1 (B) Topographical activation maps for horizontal and vertical position decoding averaged over the typical N2pc time window (200–350 ms) and the typical SPCN/CDA time window (350–700 ms). (C) Decoding performance collapsed across the horizontal and vertical dimensions, comparing the 1TMP–1TGT and 2TMP–1TGT conditions, with the difference score thus showing the effect of the number of templates. See also Figure 2—source data 2. (D) The same, now comparing the 2TMP–1TGT and 2TMP–2TGT conditions, thus showing the effect of multiple different target features in the display. See also Figure 2—source data 2. The shaded area represents 1 SEM above and below the mean for every time point. Thick lines as well as horizontal bars indicate significant clusters (at α = 0.05) as produced by cluster-based permutation testing (5000 permutations). For visualization purposes only, the classification scores over time were fitted with a cubic spline (λ = 15, comparable to a 30 Hz low-pass filter) to achieve temporal smoothing. Note the statistical analyses and estimation of the onset latencies were done on unsmoothed data. The marked time points indicate the latency of 50% maximum amplitude as estimated using a jackknife approach, as a measure of the onset of selection (Miller et al., 1998; Luck, 2014; Liesefeld, 2018). The zero points on the x-axis of panels A,C and D represent search display onsets.
-
Figure 2—source data 1
Time series data of classification AUC scores, separately for each condition and horizontal and vertical targets, as shown in Figure 2A.
- https://doi.org/10.7554/eLife.49130.009
-
Figure 2—source data 2
Time series data of classification AUC scores, separately for each condition (incl. separately for 21TGT switch and repeat trials), but collapsed across target position, as shown in Figure 2C, D and Figure 2—figure supplement 2.
- https://doi.org/10.7554/eLife.49130.010
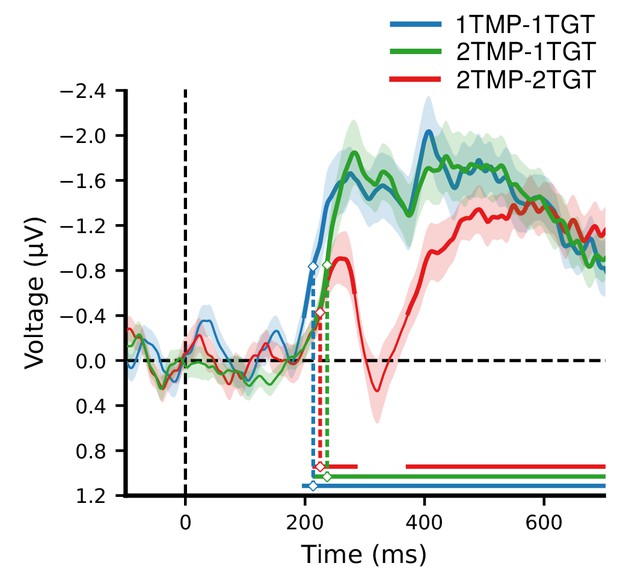
Average N2pc difference waves for targets on the horizontal (left vs. right) meridian as a function of number of templates and number of target features.
N2pc components were computed using the electrodes PO7 and PO8, by subtracting ERPs ipsilateral to the visual field of lateral targets from contralateral ERPs. The shaded area represents 1 SEM above and below the mean for every time point. Thick lines and horizontal bars indicate significant clusters (at α = 0.05) as produced by cluster-based permutation testing (5000 permutations). For visualization purposes only, the contra-ipsilateral difference waves over time were fitted with a cubic spline (λ = 15, comparable to a 30 Hz low-pass filter) to achieve temporal smoothing. Note the statistical analyses and estimation of the onset latencies were done on unsmoothed data. The marked time points indicate the latency of 50% maximum amplitude as estimated using a jackknife approach, as a measure of the onset of selection (Miller et al., 1998; Luck, 2014; Liesefeld, 2018). Reliable N2pc and sustained posterior contralateral negativity (SPCN; Mazza et al., 2007; Jolicoeur et al., 2008; Eimer, 2014; Grubert et al., 2016) components were identified in all three conditions. Onset latency was fastest for the 1TMP–1TGT condition (214 ms), followed by the 2TMP-2TGT condition (225 ms), and, surprisingly, the 2TMP–1TGT condition (237 ms, all ts > 2.2, ps<0.05). Although overall patterns are similar, these findings indicate that our main classification analyses reflect more information than is present in just the N2pc, which is subject to inherent electrode selection. See also Figure 2—figure supplements 1—source data 1.
-
Figure 2—figure supplement 1—source data 1
Time series data of N2pc difference wave locked to stimulus display onset for each condition separately.
- https://doi.org/10.7554/eLife.49130.007
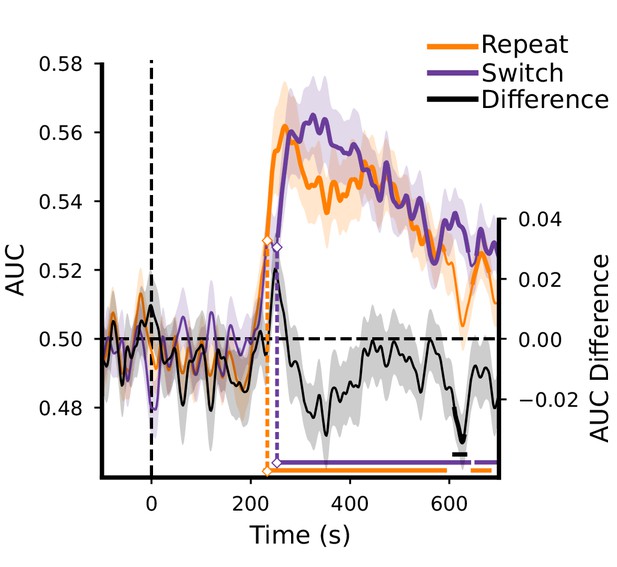
MVPA decoding performance for target position separately for switch and repeat trials in the 2TMP-1TGT condition.
The shaded area represents 1 SEM above and below the mean for every time point. Thick lines as well as horizontal bars indicate significant clusters (at α = 0.05) as produced by cluster-based permutation testing (5000 permutations). For visualization purposes only, the classification scores over time were fitted with a cubic spline (λ = 15, comparable to a 30 Hz low-pass filter) to achieve temporal smoothing. Note the statistical analyses and estimation of the onset latencies were done on unsmoothed data. The marked time points indicate the latency of 50% maximum amplitude as estimated using a jackknife approach (Miller et al., 1998; Luck, 2014; Liesefeld, 2018). See also Figure 2—source data 2.
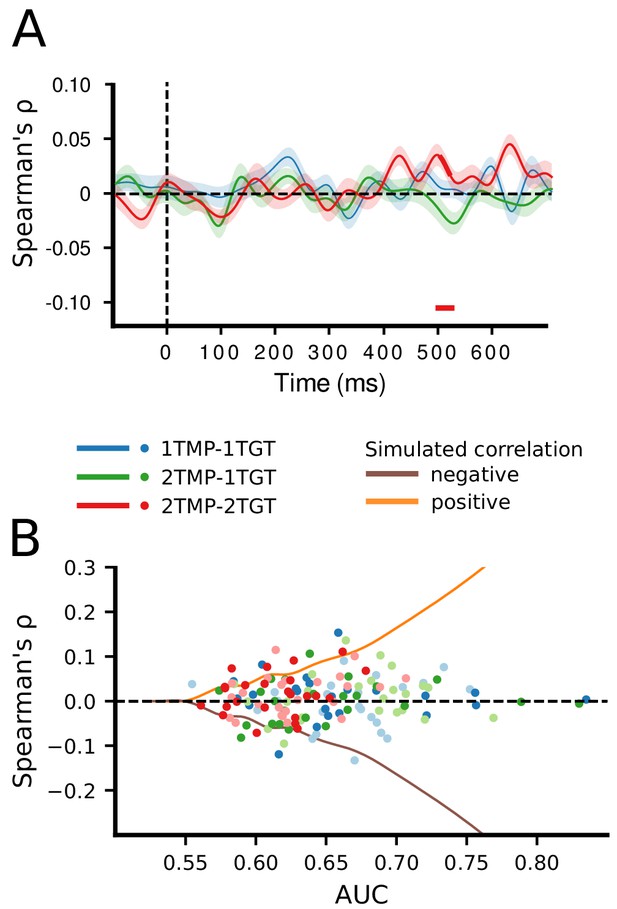
Correlation of classifier confidence scores.
(A) For each condition, and trial, the classification confidence scores per time point and subject were extracted for horizontal and vertical classifiers and then correlated (Spearman’s ρ) between these dimensions, across trials. (B) To examine whether a non-zero correlation would be present for individuals who show high AUC scores, we plotted the individual correlation scores for those time points at which individual classification was maximal, separately for the horizontal dimension (less saturated dots) and the vertical dimension (more saturated dots). The curves represent the correlation strengths that can be expected for a certain decoding strength (AUC, corresponding to SNR) as based on our simulated data set (the simulated negative correlation being the mirrored version of the positive correlation).
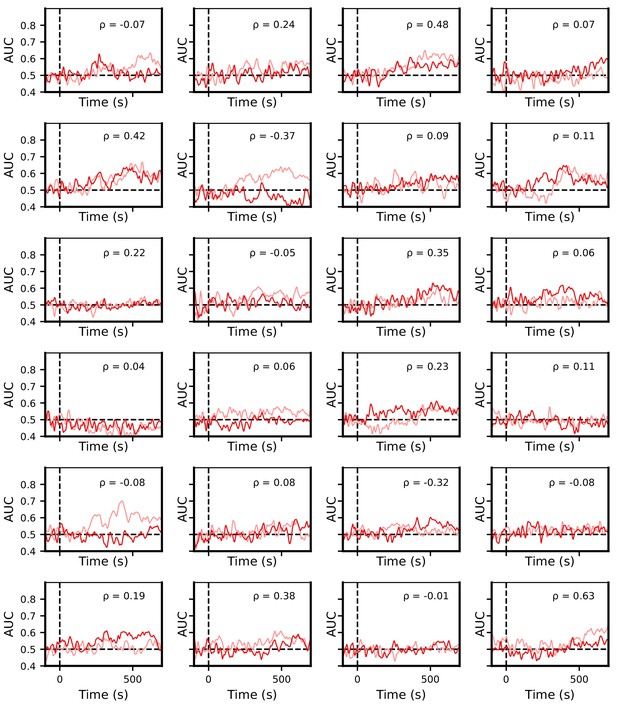
Classification performance in the 2TMP-2TGT condition, separately for horizontal and vertical targets for each participant and individual correlation scores of classifier confidence scores between vertical and horizontal targets.
Individual MVPA decoding performance for target positions on the horizontal (left vs. right, less saturated red line) and on the vertical meridian (top vs. bottom, more saturated red line) in the 2TMP-2TGT condition (which is the condition where we expected serial processing, if any, to be most prominent). For each individual, we computed Spearman’s ρ correlation between the classifier performances in the time window from 150 ms to 700 ms post stimulus (the values of which are shown in each plot). For visualization purposes only, the classification scores over time were fitted with a cubic spline (λ = 15, comparable to a 30 Hz low-pass filter) to achieve temporal smoothing. Note that the correlations were performed on unsmoothed data. See also Figure 3—figure supplement 1—source data 1 and 2.
-
Figure 3—figure supplement 1—source data 1
Time series data of classifier confidence for horizontal targets in the 2TMP-2TGT condition per participant, as shown in Figure 3—figure supplement 1.
- https://doi.org/10.7554/eLife.49130.013
-
Figure 3—figure supplement 1—source data 2
Time series data of classifier confidence for vertical targets in the 2TMP-2TGT condition per participant, as shown in Figure 3—figure supplement 1.
- https://doi.org/10.7554/eLife.49130.014
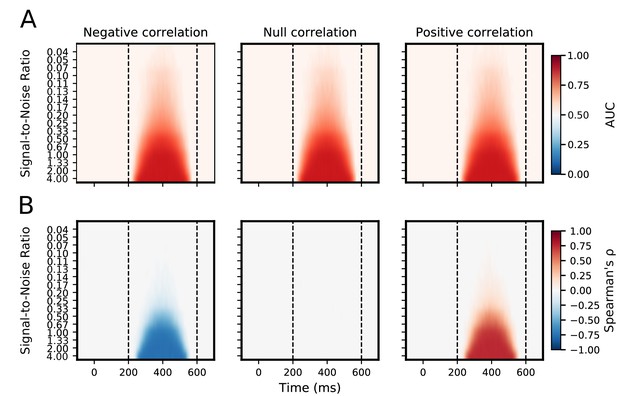
Results of location decoding and correlation analysis of a simulated dataset across several signal-to-noise ratios (SNRs).
We simulated ERPs for targets at all four target positions. These artificial ERPs were either positively, negatively, or not correlated between vertical and horizontal target position. The SNR of the simulated dataset was then manipulated by adding random noise of various levels (relative to the peak amplitude of the ERP) to the data. (A) AUC scores (color coded) are shown for a location decoding analysis, separately for the injected correlation and SNR across time. (B) Spearman’s ρ correlation (color coded) between confidence scores of the vertical and horizontal classifiers, separately for the injected correlation and SNR across time. For both A and B, only those time points were colored that survived cluster-based permutation testing (α = 0.05, 5000 permutations). Dashed vertical lines indicate the time window in which the ERP was injected. The figure shows that both location decoding and the retrieving of the injected correlation declined with decreasing SNR. Importantly, reliable correlations disappear before classification itself, indicating that for the average group classification levels as observed in our data, any correlation present may have been too low to be detected.
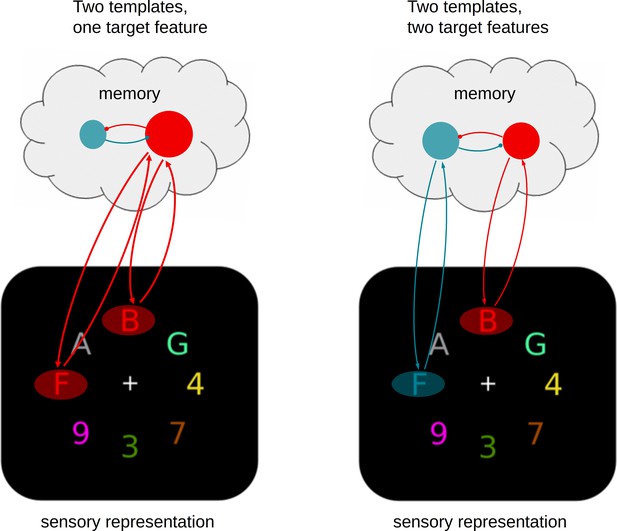
A limited parallel model.
Attentional templates in memory engage in recurrent feedback loops with matching sensory representations, resulting in target enhancement. Multiple templates can be activated in parallel and may be equally active prior to search, but they compete through mutual suppression, which has consequences during search. (A) The presence of a single target feature in the sensory input will unequivocally trigger one of the active templates, eventually resulting in as strong selection as when there is only one template (not shown), albeit at a short delay. (B) When both templates are activated the mutual suppression will prevent strong activation of either, resulting in substantially weakened and delayed selection of both targets.
Additional files
-
Transparent reporting form
- https://doi.org/10.7554/eLife.49130.017