Catecholaminergic modulation of meta-learning
Figures
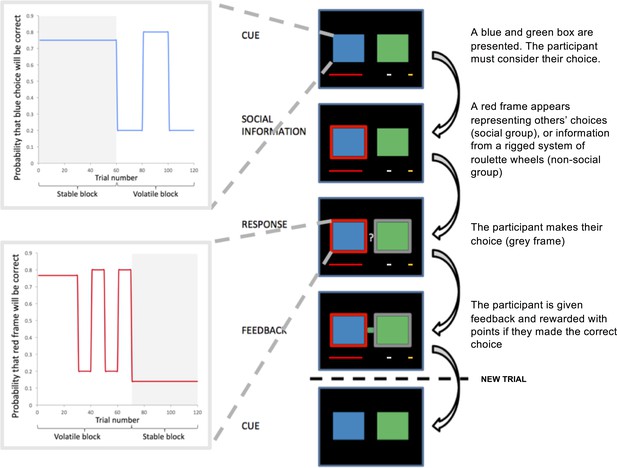
Task design.
Participants selected between two bandits (blue and green boxes) in order to win points. On each trial, participants saw the direct sources (boxes, 1–4 s), subsequently either the blue or green box was highlighted with a red frame (the indirect source, 1–4 s). Participants were instructed that the frame represented either the most popular choice made by a group of participants who had completed the task previously (Social Group) or the choice from rigged roulette wheels (Non-social Group). After participants had responded, their selected option was framed in grey. After 0.5–2 s participants received feedback in the form of a green or blue box between the two bandits. A new trial began after 1–3 s. Success resulted in the red reward bar progressing towards the silver and gold goals. The probability of reward associated with the blue and green boxes and the probability that the red frame surrounded the correct box varied according to probabilistic schedules which comprised stable and volatile phases.
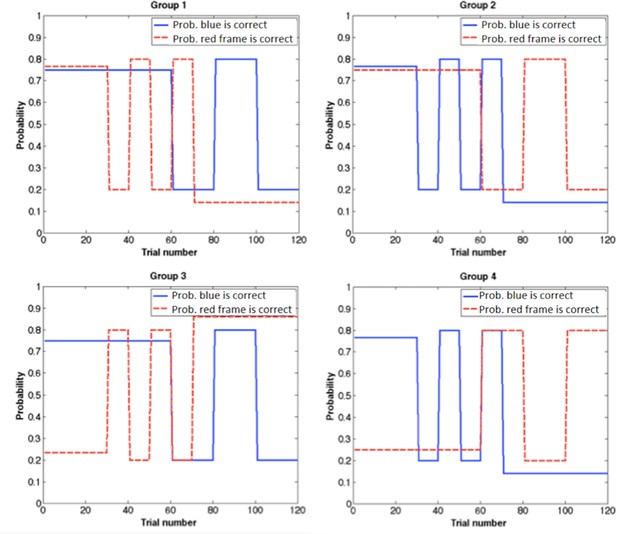
Probabilistic schedules.
Solid blue lines show the probability of blue being the correct choice, dashed red lines show the probability of the red frame information being correct. For the win-stay, lose-shift analysis the interaction between drug, learning type and volatility remained significant when probabilistic schedule group (1,2,3,4) was included as a factor in the ANOVA (F(1,94) = 7.935, p = 0.006). There was no significant main effect of schedule (F(3,94) = 0.906, p = 0.441) and no interactions involving both drug and schedule (all p>0.05). For the learning rate analysis, the interaction between drug, learning type and volatility remained significant when schedule (1,2,3,4) was included as a factor in the ANOVA (F(1,94) = 7.500, p = 0.007). There was no significant main effect of schedule (F(3,94) = 0.850, p = 0.470) and no drug x learning type x volatility x group x schedule interaction (F(3,94) = 0.404, p = 0.751). There was a significant drug x learning type x volatility x probabilistic schedule interaction (F(3,94) = 2.725, p = 0.049). Although the pattern of results was comparable, the interaction between drug x learning type x volatility was only statistically significant for schedule 2 (F(1,23) = 12.231, p = 0.002), and did not reach significance for schedules 1 (F(1,24) = 4.015, p = 0.057), 3 (F(1,22) = 2.881, p = 0.104) and 4 (F(1,25) = 0.501, p = 0.486), likely due to a lack of power with these reduced group sizes.
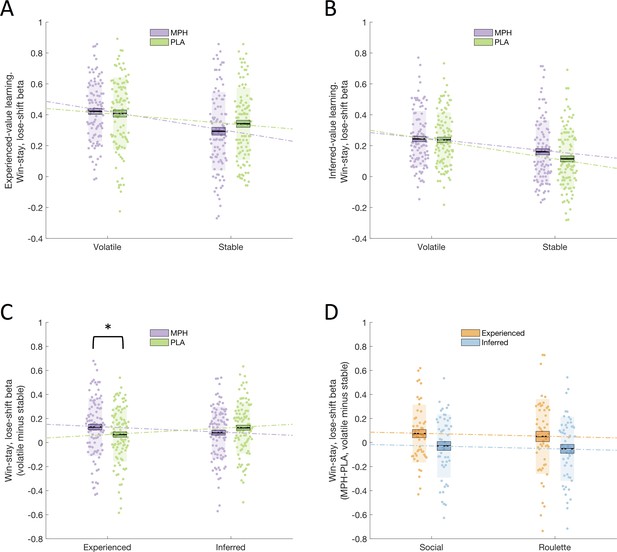
Drug effects on win-stay, lose-shift beta values.
(A) Win-stay, lose-shift betas, for experienced-value learning, in stable and volatile periods, under MPH (purple) and PLA (green). (B) Win-stay, lose-shift betas, for inferred-value learning, in stable and volatile periods, under MPH and PLA. (C) There was a significant interaction between drug and volatility for experienced-value learning, but not for inferred-value learning. (D) Drug effect on win-stay, lose-shift betas for volatile minus stable blocks, for experienced-value (orange) and inferred-value (blue) learning. There was no difference between the social and non-social (roulette) groups. Boxes = standard error of the mean, shaded region = standard deviation, individual datapoints are displayed, MPH = methylphenidate, PLA = placebo, * indicates statistical significance.
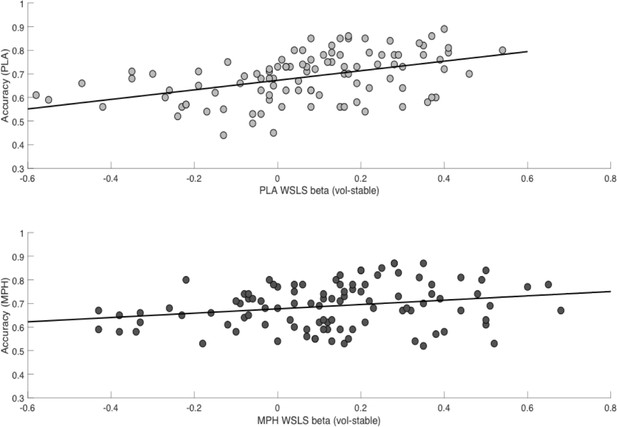
Illustration of relationship between experienced-value WSLS_vol-stable and accuracy.
Experienced-value WSLS_vol-stable was significantly positively correlated with accuracy under both PLA (Top: Pearson’s r = 0.453, p < 0.001) and MPH (Bottom: Pearson’s r = 0.236, p = 0.017).
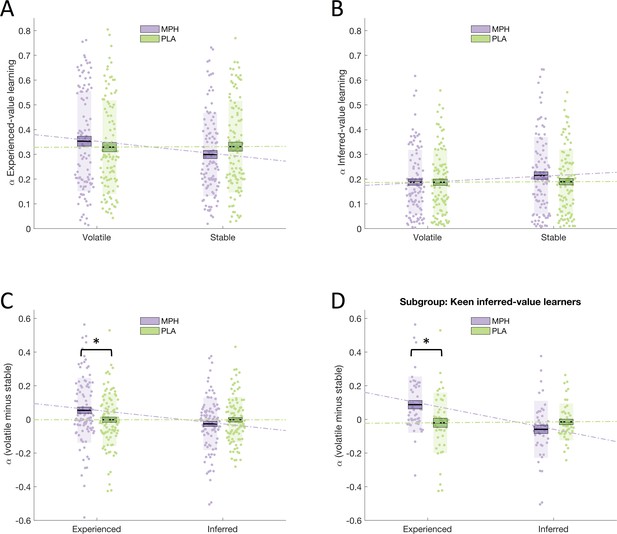
Drug effects on learning rates.
(A) Learning rate for experienced-value learning, in stable and volatile periods, under MPH (purple) and PLA (green). (B) Learning rate for inferred-value learning, in stable and volatile periods, under MPH and PLA. (C) There was a significant interaction between drug and volatility for experienced-value learning, but not for inferred-value learning. (D) The interaction between drug and volatility was specific to experienced-value learning even for a sub-sample of participants who were keen inferred-value learners. Boxes = standard error of the mean, shaded region = standard deviation, individual datapoints are displayed, MPH = methylphenidate, PLA = placebo, * indicates statistical significance.
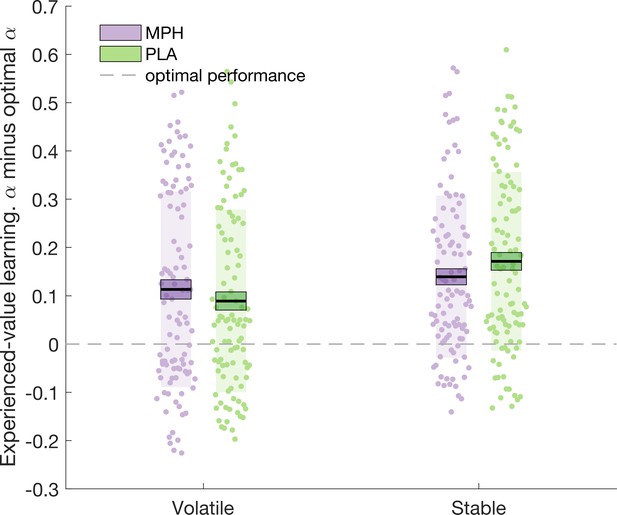
Experienced-value learning rates under PLA, but not MPH, were further from the optimal value in the stable compared to volatile phase.
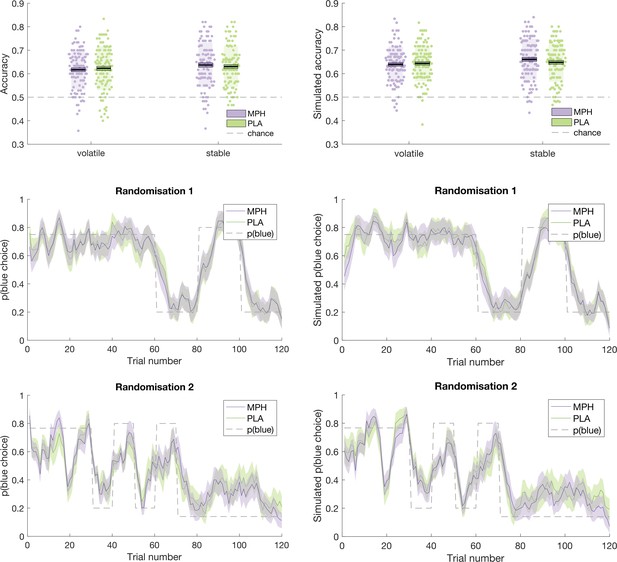
Participant data (left) juxtaposed against model simulations (right).
Top: Accuracy, in stable and volatile phases, under MPH (purple) and PLA (green). Boxes = standard error of the mean, shaded region = standard deviation, individual datapoints are displayed. Middle and Bottom: Running average, across 5 trials, of blue choices for probabilistic randomisation schedules 1 (middle) and 2 (bottom). Shaded region = standard error of the mean. MPH = methylphenidate, PLA = placebo.
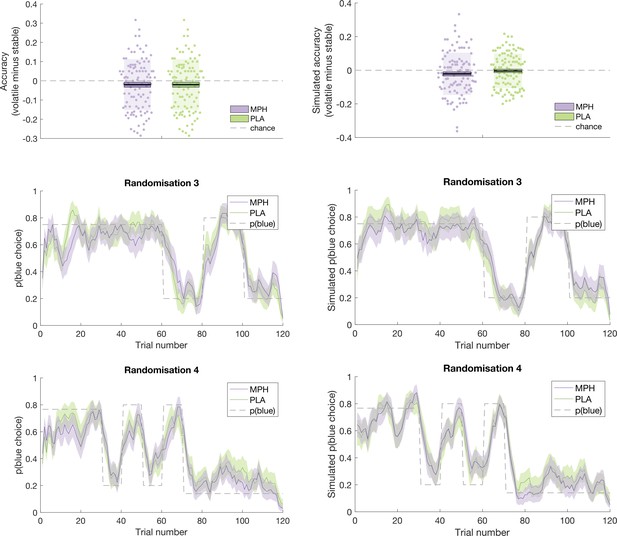
Participant data (left) juxtaposed against model simulations (right).
Top: Accuracy, in stable and volatile phases, under MPH (purple) and PLA (green). Boxes = standard error of the mean, shaded region = standard deviation, individual datapoints are displayed. Middle and Bottom: Running average, across 5 trials, of blue choices for probabilistic randomisation schedules 3 (middle) and 4 (bottom). Shaded region = standard error of the mean. MPH = methylphenidate, PLA = placebo.
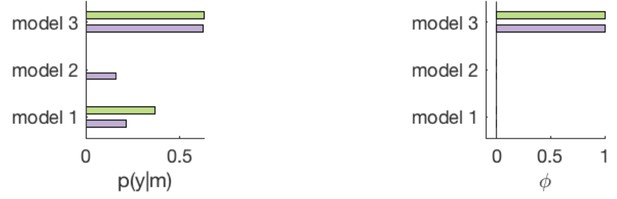
= posterior model probability, ϕ = exceedance probability, MPH = purple, PLA = green.
Tables
Coefficients from regression model with MPH-PLA accuracy as dependent variable.
(std. Err.) | standardised | t | p | ||
---|---|---|---|---|---|
constant | −0.008 | 0.006 | −1.3 | 0.197 | |
MPH-PLA | 0.014 | 0.002 | 0.36 | 5.763* | <0.001 |
ζMPH-PLA | −0.241 | 0.024 | −0.658 | −9.943* | <0.001 |
stable_experienced_MPH-PLA | 0.005 | 0.032 | 0.011 | 0.172 | 0.864 |
vol_experienced_MPH-PLA | 0.126 | 0.029 | 0.285 | 4.376* | <0.001 |
stable_inferred_MPH-PLA | 0.014 | 0.041 | 0.023 | 0.345 | 0.731 |
volatile_inferred_MPH-PLA | 0.02 | 0.041 | 0.03 | 0.483 | 0.63 |
-
* indicates statistical significance.
There were no significant differences between the social and non-social groups in age, gender or socioeconomic status as measured using the Barratt Simplified Measure of Social Status (Barratt, 2012).
Social | Non-social | ||
---|---|---|---|
Age in years () | 21.8 (2.6) | 21.3 (2.0) | t(100) = 1.1, p = 0.3 |
Socioeconomic status () | 60.4 (7.8) | 59.2 (8.4) | t(100) = 0.5, p = 0.6 |
N(Male:Female) | 50 (26:24) | 52 (25:27) | (1) = 0.16, p = 0.7 |
Win-stay, lose-shift analysis.
Main effects and interactions not involving the factor drug.
Main effect/interaction | Statistics | Further information |
---|---|---|
Learning type | F(1,100) = 58.595, p < 0.001 | WSLS for experienced ( = 0.366(0.017)) > inferred ( = 0.188(0.012)) |
Volatility | F(1,100) = 70.671, p < 0.001 | WSLS for volatile ( = 0.327(0.010)) > stable ( = 0.227(0.011)) |
Group | F(1,100) = 4.001, p = 0.048 | WSLS for non-social ( = 0.295(0.013)) > social ( = 0.259(0.013)) |
Learning type x group | F(1,100) = 7.729, p = 0.006 | WSLS for social ( = 0.138(0.017)) < non-social ( = 0.239(0.017)) for inferred (F(1,100) = 17.743, p < 0.001). No difference between groups for experienced (social = 0.381 (0.024), non-social = 0.352 (0.024), F(1,100) = 0.694, p = 0.407) |
Learning rate analysis.
Main effects and interactions not involving the factor drug. Note that the learning type x volatility interaction is consistent with previous studies of experienced-value learning which have reported higher learning rates in volatile relative to stable environments (Behrens et al., 2007; Behrens et al., 2008; Browning et al., 2015; Krugel et al., 2009; Nassar et al., 2010).
Main effect/interaction | Statistics | Further information |
---|---|---|
Learning type | F(1,100) = 65.409, p < 0.001 | direct ( = 0.328(0.014)) > inferred ( = 0.194(0.010)) |
Learning type x volatility | F(1,100) = 4.882, p = 0.029 | vol_experienced (= 0.341(0.017)) > stable_experienced ( = 0.315(0.015); F(1,100) = 3.916, p = 0.051). No significant difference between stable_inferred ( = 0.201(0.012)) and vol_inferred ( = 0.187(0.011); F(1,100) = 1.675, p = 0.199) |
Learning type x group | F(1,100) = 4.097, p = 0.046 | social ( = 0.161(0.014)) < non-social ( = 0.227(0.014)) for inferred (F(1,100) = 11.349, p = 0.001). No difference between groups for experienced (social 0.329(0.021), non-social 0.328(0.020); F(1,100) = 0.002, p = 0.966) |
Coefficients from regression models with MPH and PLA accuracy as dependent variables.
(std. Err.) | standardised | t | p | ||
---|---|---|---|---|---|
PLA | |||||
constant | 0.67 | 0.02 | 30.15* | <0.001 | |
PLA | −0.25 | 0.02 | −0.66 | −12.84* | <0.001 |
ζPLA | 0.03 | 0.00 | 0.43 | 8.53* | <0.001 |
stable_experienced_PLA | 0.05 | 0.04 | 0.09 | 1.35 | 0.18 |
vol_experienced_PLA | 0.10 | 0.04 | 0.18 | 2.67* | 0.01 |
stable_inferred_PLA | −0.03 | 0.04 | −0.03 | −0.56 | 0.58 |
volatile_inferred_PLA | 0.13 | 0.05 | 0.16 | 2.84* | 0.01 |
MPH | |||||
constant | 0.69 | 0.02 | 39.88* | <0.001 | |
MPH | −0.26 | 0.02 | −0.78 | −15.92* | <0.001 |
ζMPH | 0.02 | 0.00 | 0.44 | 9.15* | <0.001 |
stable_experienced_MPH | 0.03 | 0.03 | 0.06 | 1.05 | 0.30 |
vol_experienced_MPH | 0.10 | 0.03 | 0.22 | 4.05* | <0.001 |
stable_inferred_MPH | 0.05 | 0.03 | 0.08 | 1.49 | 0.14 |
volatile_inferred_MPH | 0.03 | 0.04 | 0.05 | 0.92 | 0.36 |
-
* indicates statistical significance at p < 0.05.
Mean(standard deviation) scores for neuropsychological tests and self-report questionnaires for the groups that received PLA and MPH on day 1.
Significance level for the between group differences are reported. Self-report questionnaires include the Barratt Impulsiveness Scale (BIS-11; Patton et al., 1995), the Behavioural Inhibition Scale/Behavioural Activation Scale (BISBAS) (Carver and White, 1994), Need for Cognition Scale (NCS) (Cacioppo et al., 1984), Multidimensional Scale of Perceived Social Support (MSPSS) (Zimet et al., 1988), Barratt Simplified Measure of Social Status (BSMSS) (Barratt, 2012), Sociable and Aggressive Dominance Questionnaire (SADQ) (Kalma et al., 1993), Beck Depression Inventory II (BDI-II) (Beck et al., 1996), Spielberger Trait Anxiety Inventory (STAI) (Spielberger et al., 1983).
Group 1 PLA Day 1 | Group 2 MPH Day 1 | |||
---|---|---|---|---|
Neuropsychological tests | Listening span | 5.0 (0.9) | 4.6 (1.2) | p = 0.160 |
NLV | 94.4 (7.6) | 92.6 (7.6) | p = 0.230 | |
Digit span – forward | 17.2 (3.7) | 16.2 (3.6) | p = 0.158 | |
Digit Span - backward | 14.7 (3.4) | 13.9 (2.7) | p = 0.219 | |
Self-report questionnaires | Impulsivity (BIS-11) | 63.5 (8.9) | 60.2 (7.9) | p = 0.052* |
Behavioural inhibition (BIS) | 16.4 (3.7) | 16.3 (3.5) | p = 0.899 | |
Behavioural activation (BAS) | 22.8 (3.9) | 23.9 (4.0) | p = 0.166 | |
Need for cognition (NCS) | 64.5 (10.5) | 62.2 (10.5) | p = 0.264 | |
Social support (MDSPSS) | 71.1 (10.1) | 69.3 (9.6) | p = 0.349 | |
Social status (BSMSS) | 49.8 (12.1) | 45.9 (12.7) | p = 0.114 | |
Social dominance (SADQ) | 4.1 (0.9) | 4.1 (0.8) | p = 0.823 | |
Aggressive dominance (SADQ) | 2.6 (0.6) | 2.6 (0.6) | p = 0.691 | |
Depressive symptoms (BDI) | 3.5 (3.7) | 3.6 (3.9) | p = 0.969 | |
Anxiety symptoms (STAI) | 32.4 (6.6) | 32.4 (7.2) | p = 0.995 |
-
*One participant had an outlying score on the BIS-11. Without outlier: p = 0.090.