Learning improves decoding of odor identity with phase-referenced oscillations in the olfactory bulb
Figures
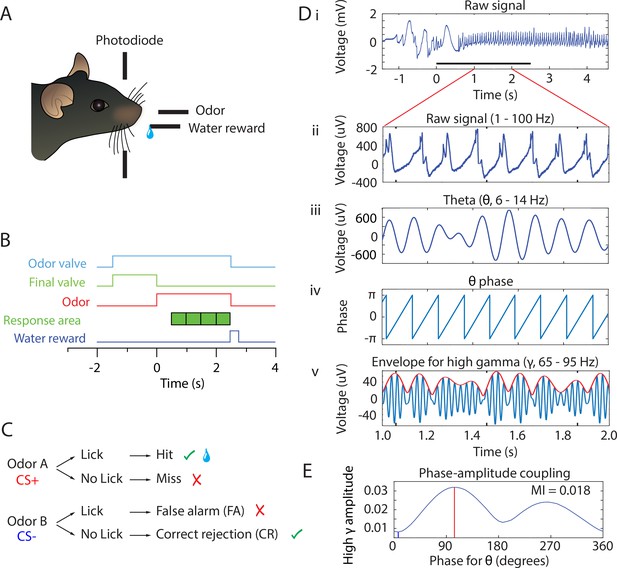
Behavioral task and PAC analysis.
(A) Behavioral apparatus. Mouse self-initiates trials by breaking a photodiode beam. Odorants and water are delivered according to the timeline in B and the decision tree in C. (B) Timeline for a single trial. When the animal enters the port the air flow is diverted by turning the final valve output to the exhaust and the odorant valve is turned on. At this time the odor builds up to steady-state concentration flowing away from the animal for 1–1.5 s. At time 0 the final valve air flow is turned towards the odor port for 2.5 s resulting in odor onset 100 ms after the valve is actuated. In order to trigger water reward for the rewarded odorant the mouse must lick at least once during each 0.5 s block for four blocks in the response area. If the stimulus is rewarded and the animal licked appropriately, a water reward is delivered. (C) Decision tree for each stimulus. Green check mark means the correct decision was made. Red ‘X’ mark indicates an incorrect decision. Water reward is represented by the water droplet symbol in the case of a hit. (D) PAC data analysis of the LFP. For each electrode, raw signal collected at 20 kHz (i) is bandpass filtered to 1–100 Hz for broadband LFP (ii) or filtered into different frequency bands as needed (e.g. theta 6–14 Hz in iii or high gamma 65–95 Hz in v, blue line). Hilbert transform is used to calculate the theta phase (iv) and the amplitude envelope of higher oscillations such as high gamma (65–95 Hz) (red line in v). Theta phase and the envelope of the amplitude of high gamma are then used to calculate the probability for high gamma amplitude at specific phases of theta (PAC, shown in E). (E) Probability for high gamma amplitude at specific phases of theta. In this example, PAC is strongest at ~100° and 270°. The coupling strength is quantified by the modulation index, MI. Peak phase (the phase of theta with the highest amplitude in high gamma) is indicated with a red line and trough phase is indicated with a blue line.
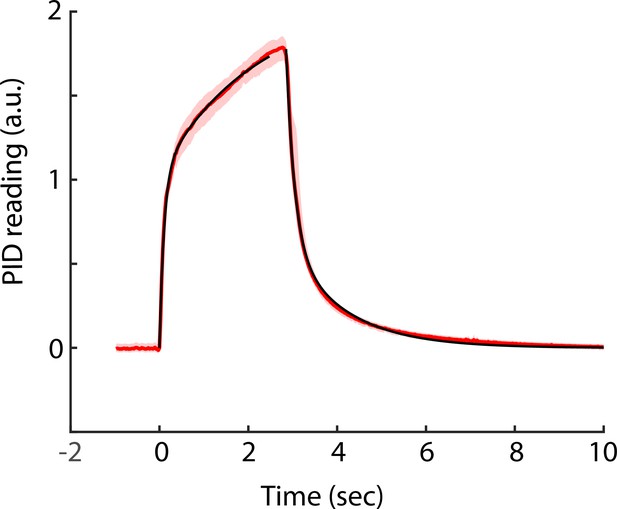
Time course for odorant concentration measured at the odor spout in the olfactometer.
A miniPID (Aurora Scientific) photoionization detector was used to measure the concentration of odorant at the odor spout of the olfactometer. The odorant was air equilibrated with Sharpie black ink. The red line is the concentration of the odorant in arbitrary units bounded by the bootstrapped confidence interval (n = 10 traces). Time was aligned to the onset of the increase in odorant concentration that took place 104.5 ± 7 msec (mean ± SD, n = 10) after the final valve was diverted to deliver the odorant to the odorant spout. The black lines are regression fits of exponential increase and decrease lines with time constants of 0.12 and 2.16 s (increase, two exponential terms) and 0.19 and 1.28 s (decrease, two exponential terms).
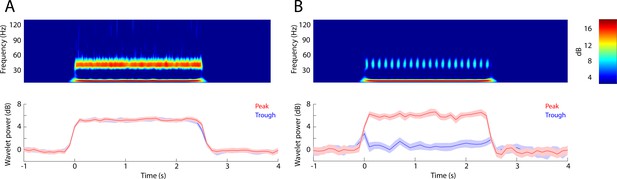
Simulation: PRP wavelet analysis for theta-gamma waves with/without PAC.
LFP data were generated by sinusoids supplemented with gaussian random noise. The data were analyzed for PRP as described in the text. (A) Example with no theta/gamma PAC generated by input of two continuous cosine waves of 8 Hz and 40 Hz. Top: Morlet wavelet spectrogram shows a continuous increase in power at the two frequencies. Bottom: peak (red) and trough (blue) PRP do not differ. MI = 0.0004. (B) Example of theta/gamma PAC generated with input of 40 Hz gamma bursts placed at the 180° phase of an 8 Hz theta sine wave (the bursts take place at the peak of PAC). Top: Morlet wavelet spectrogram shows discrete increases of gamma power and a continuous increase in power at 8 Hz. Bottom: peak (red) PRP shows a large increase in power while trough PRP (blue) does not increase. MI = 0.01. .
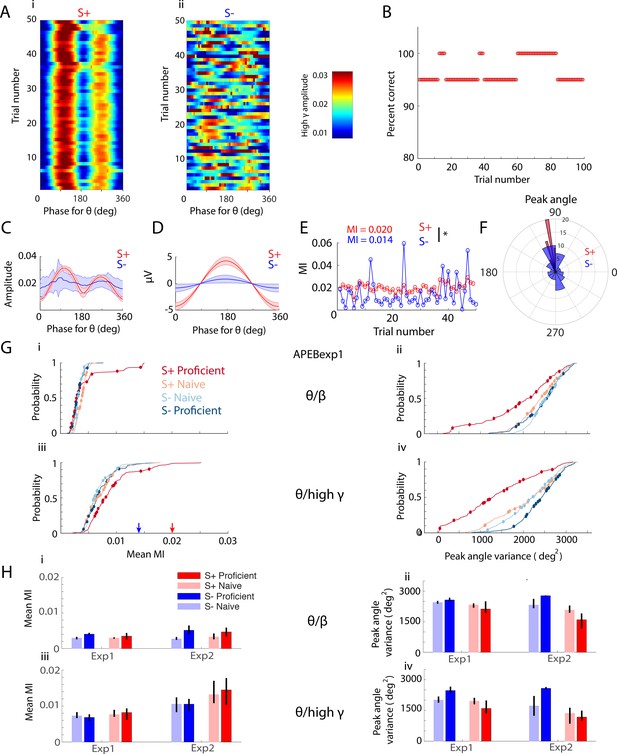
The peak angle variance of PAC changes as the animal learns to discriminate odorants.
(A-F) Example of theta/high gamma phase amplitude coupling for a proficient animal (IAAPexp1 odorant pair). (A) Pseudocolor plots showing the phase amplitude relationship for S+ (left) and S- (right) for an example go no-go session. PAC between theta (6–14 Hz) phase and high gamma (65–95 Hz) amplitude was more variable for S- compared to S+. (B) Behavioral performance for this session. Over 100 trials, this trained animal discriminated between isoamyl acetate and acetophenone with 95–100% accuracy. A sliding window of 20 trials with a step of one trial was used to calculate percent correct behavioral performance. (C) Theta phase distribution for high gamma amplitude (mean ± CI) for S+ (red) and S- (blue). (D) Mean theta LFP (mean ±CI) for one cycle for S+ (red) and S- (blue). (E) MI per trial for S+ (red) and S- (blue) as a function of trial number. The difference in MI between S+ and S- is statistically significant (ranksum p value < 0.001, n = 50 s- trials, 49 S+ trials). (F) Polar histograms for high gamma peak theta angles for S+ (red) and S- (blue). (G) Cumulative histograms showing the differences in mean MI (i,iii) and peak angle variance (ii,iv) for theta/beta PAC (i,ii) and theta/high gamma PAC (iii,iv) for the APEB odorant in Exp1 for the S+ and S- odorants for naïve and proficient trial ranges. Peak angle variance for S+ displays a substantial decrease with learning, while mean MI shows small changes. Changes with learning and between events for mean MI and peak angle variance are statistically significant (GLM p value < 0.001, 896 d.f. for theta/beta and theta/high gamma, 16 electrodes per mouse, 14 mice). Blue and red arrows in Giii are MIs derived from the single-session example in C. (H) Bar graph displaying mean MI (i,iii) and peak angle variance (ii,iv) for naïve and proficient trial ranges and S+ and S- events for mean values calculated per experiment for theta/beta (i,ii) and theta/high gamma PAC (iii,iv). GLM yielded significant p values for peak angle variance for theta/high gamma PAC for S+ vs. S- (<0.01, 16 d.f.) and for the interaction between S+ vs. S- and proficiency (p<0.05, 16 d.f.). GLM yielded significant p values for peak angle variance for theta/beta PAC for S+ vs. S- and experiment and for the interaction between S+ vs. S- and experiment (<0.01, 16 d.f.). For mean MI theta/high gamma PAC GLM yields significant difference for experiments (p<0.01, 16 d.f.). Finally, there are no significant effects for mean MI for theta/beta PAC (p>0.05, 16 d.f.) and no post hoc tests yield significant differences (p>pFDR).
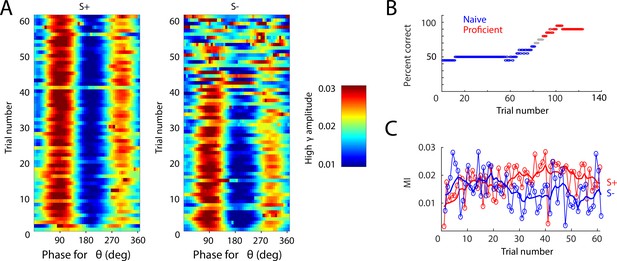
Example of PAC changes over learning in one session.
(A) PAC for all S+ trials (left) and S- trials (middle), with high γ amplitude color bar quantified at right. (B) Behavioral performance. Mouse starts the session performing at chance, then learns the task, acquiring proficiency by the end. Blue: naïve, performance ≤65%. Red: proficient, performance ≥80%. (C) PAC quantified as mean MI for S+ (red) and S- (blue). With learning, PAC increases for S+ and decreases for S-.
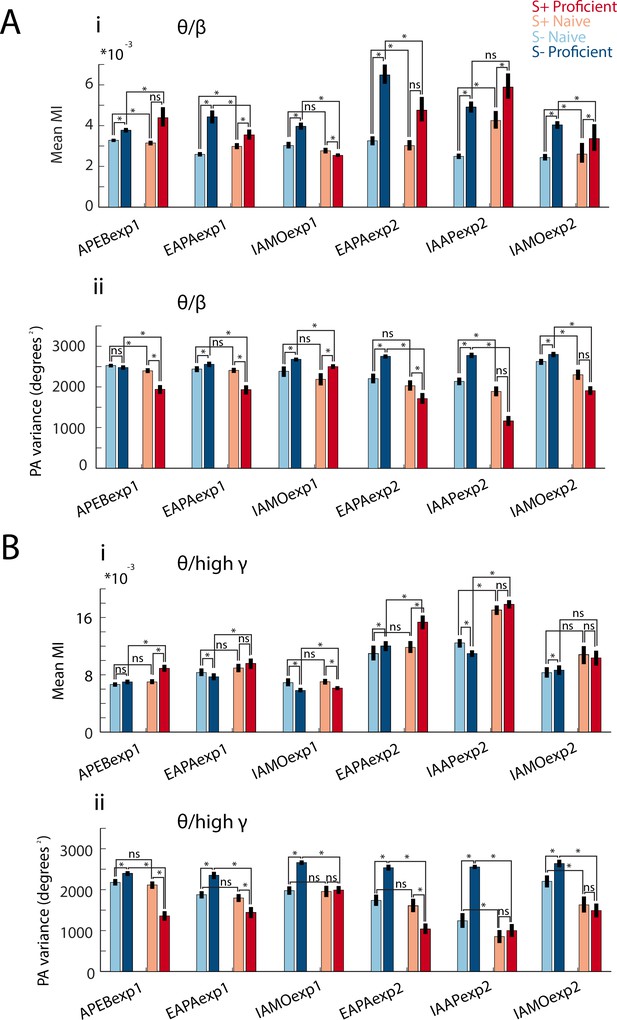
Modulation index and peak angle variance shown for each odorant pair.
Bar graphs displaying for theta/beta (A) and theta/high gamma (B) PAC differences in mean MI (Ai and Bi) and peak angle variance (Aii and Bii) between naïve and proficient trial ranges and S+ and S- events for all sessions for all the odorant pairs. GLM yielded significant p values (<0.001) for both mean MI and peak angle variance for event, proficiency and experiment, with the exception that p<0.01 for proficiency for theta/high gamma (3255 d.f., number of mice is in Supplementary file 1-Table S1). Asterisks denote a significant post-hoc difference evaluated with either a t-test or a ranksum test (p<pFDR, for the number of mice per odorant, see Supplementary file 1-Table S1). Changes in mean MI were variable for different odorants. MI displays differences between events and learning states that differ in magnitude between odorant pairs. Peak angle variance (at right) increases with task proficiency for S- and decreases for S+ for all odorants.
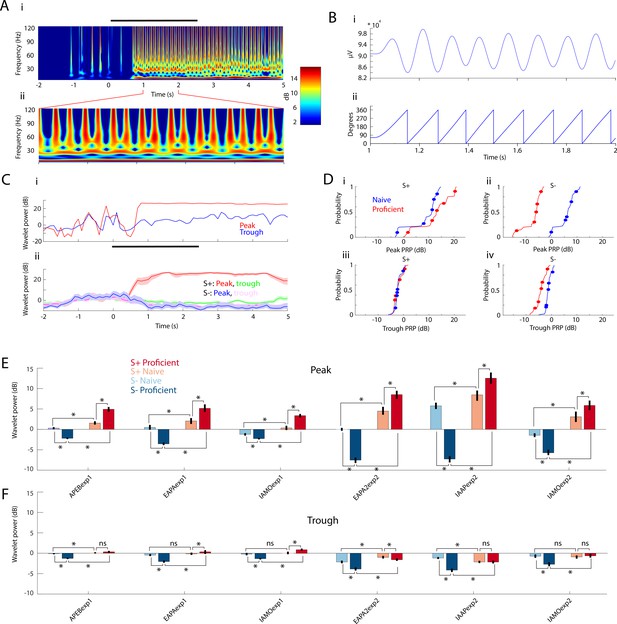
PRP increases for S+ and decreases for S- over learning.
(A) Example wavelet broadband LFP spectrogram for one S+ trial. Right: Pseudocolor scale for LFP power (in dB). Black bar represents the odor stimulation epoch (2.5 s). i: Full trial length demonstrating pre-odor baseline LFP, odor driven LFP, and reward epoch. ii: One-second epoch during odor stimulation. (B) i: Theta waveform (6–14 Hz) during odor stimulation. ii: Theta phase, extracted with Hilbert transform. (C) i: Single trial wavelet power referenced to either peak (108°, red) or trough (0°, blue) of the high gamma amplitude distribution along the theta phase. ii: PRP (± CI) averaged across the entire session for S+ and S-. Peak PRP for S+ displays the largest odor-induced increase in power that is sustained after the end of odor stimulation. (D) The thin lines are cumulative histograms for PRP for the LFP recorded in each electrode averaged for theta/high gamma for all proficient or naïve trials for mice performing to differentiate odorants in the IAAPexp2 odorant pair. i and ii are peak PRP and iii and iv are trough PRP. i and iii are S+; ii and iv are S-. As the animals learn the task, peak PRP (i and ii) increases for S+ and decreases for S-, whereas trough PRP (iii and iv) is relatively stable. The differences were statistically significant between peak and trough, S+ and S- and naïve and proficient (GLM p values < 0.001, 1016 d.f.). Circles are per mouse averages for PRP. (E–F) Odor-induced changes in high gamma PRP averages for all odorant pairs evaluated per electrode during the odor delivery epoch (0.5–2.5 s). Peak PRP (E) increases for S+ and decreases for S- when the mice become proficient. Trough PRP changes (F) are markedly weaker, yet they follow a similar trend (GLM p value < 0.001, 6386 d.f. for S+ vs. S-, peak vs. trough, naïve vs. proficient). In addition, the learning-induced changes in PRP are larger in Exp2 (GLM p value < 0.001 for experiment, 6386 d.f.).
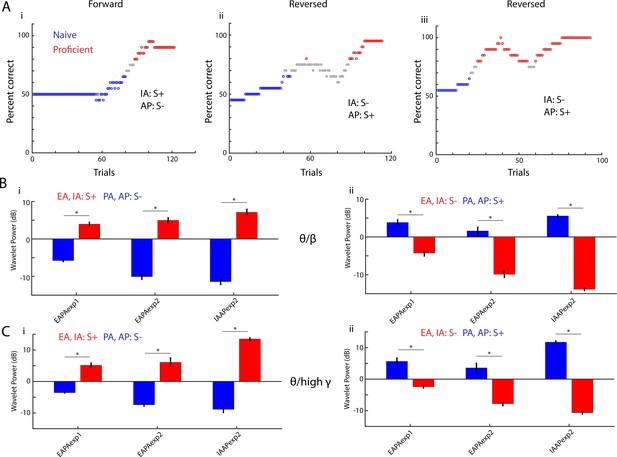
Peak theta-phase referenced power switches when odorant valence is reversed.
(A) Behavioral performance in three go-no go sessions for a mouse learning to differentiate the IAAPexp1 odorant pair. In the first session the rewarded odorant (S+) was isoamyl acetate (IA) and the unrewarded odorant (S-) was acetophenone (AP). In the other two sessions the valence of the odorant was reversed. Blue:≤65% percent correct performance (naïve) and red:≥80% (proficient). (B–C) Theta/beta (B) and theta/high gamma (C) peak PRP, calculated for trials when the mouse is proficient, switches when odorant valence is reversed. The data are shown for three odor pairs: EAPAexp1 (11 mice), EAPAexp2 (nine mice), IAAPexp2 (three mice). GLM analysis yields significant changes for odorant, reversal and the interaction of reversal and odorant (p<0.001, 1051 d.f.). Asterisks denote post hoc significant differences (p<pFDR = 0.04).
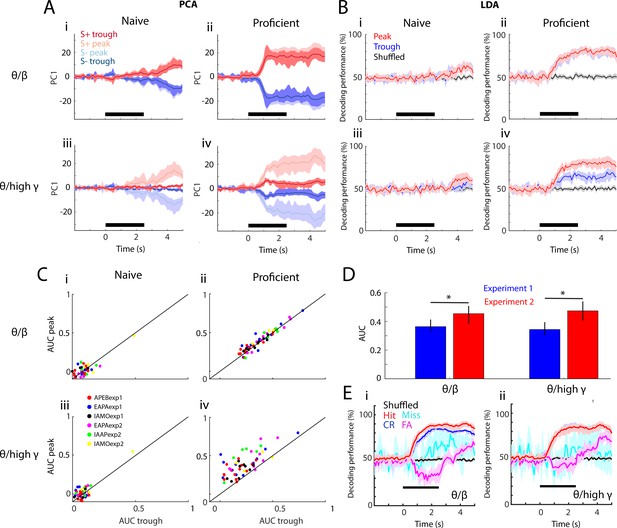
Linear discriminant analysis classifies stimuli using peak PRP.
(A–B) PCA (in panel A) and linear discriminant analysis (LDA; in panel B) of averaged PRP data for one example odorant pair, APEBexp1. (A) PCA of PRP data from naïve (i,iii) to proficient (ii,iv)—(i,ii): theta/beta, (iii,iv): theta/high gamma. Both PRPs for S+ and S- are discriminable with learning. (B) Decoding performance for the LDA of PRP data over learning (i,iii: naïve, ii,iv: proficient). i,ii: theta/beta, iii,iv: theta/high gamma. In the proficient state, LDA can decode stimulus identity using both PRPs (theta/beta PRP GLM naïve-proficient p value < 0.001, 104 d.f.; theta/high gamma PRP GLM naïve-proficient p value < 0.001, 104 d.f.). Peak and trough carry similar information for theta/beta; both peak- and trough-referenced beta power can be used to decode stimulus identity with learning. In theta/high gamma, peak PRP is significantly better at decoding the stimulus identity than trough PRP (GLM p value < 0.001, 104 d.f.), which is still better than shuffled data. (C) Area under the curve (AUC) for peak versus trough PRP over task learning (i,iii: naïve, ii,iv: proficient) for all odorant pairs and experiments. i,ii: Theta/beta PRP. iii,iv: For theta/high gamma PRP GLM statistics show that peak AUC is significantly higher than trough (p value < 0.001 for six odorant pairs, 184 d.f.) and that there are significant changes over learning (p value < 0.001 for six odorant pairs, 184 d.f.). These data suggest that peak PRP decodes stimulus identity better than trough PRP. (D) Average peak AUC (±CI) for all odorant pairs for theta/beta and theta/high gamma PRP for proficient mice for the two experimental settings. The learning-induced changes in PRP are larger in Exp2 (GLM p value < 0.05 for experiment, 102 d.f., *post hoc p<pFDR = 0.03). (E) Decoding performance for the LDA of PRP data for proficient mice calculated for the different behavioral outcomes of the go-no go task (Hit: red, Miss: cyan, CR: blue, FA: magenta, shuffled: black). i: Theta/beta PRP, ii: Theta/high gamma PRP. A GLM analysis indicates that there are significant differences between all behavioral outcomes except for Hit vs. CR for theta/high gamma (p<0.001 for all outcome pairs, except for Hit vs CR for theta/beta PRP that has p<0.01 and Hit vs. CR for theta/high gamma that has p>0.05, 2110 d.f.). There is no significant difference between experiments (GLM p>0.05, 2110 d.f.). The lines in A,B and E are bounded by the bootstrapped 95% CI..
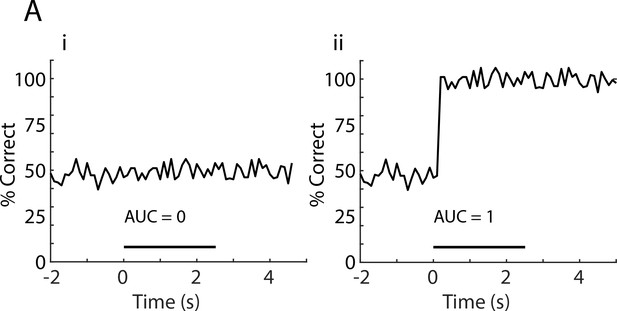
Simulated LDA performance data illustrating AUC zero and one.
(A) Simulated data illustrating the time course for decoding performance for LDA for discrimination of odor identity with: (i) The area under the curve (AUC) = 0, LDA was not able to identify the stimuli, and (ii) AUC = 1, perfect identification of the stimulus after odor application. AUC was calculated as the area under the curve during odorant stimulation normalized to 1.
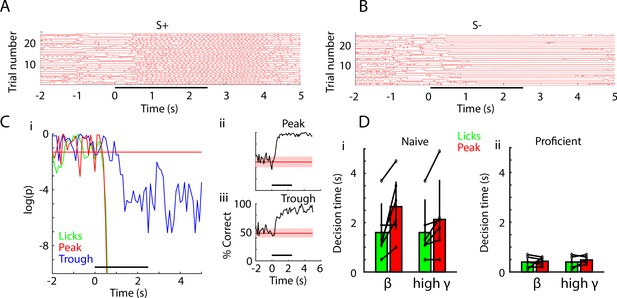
Decision-making time does not differ between licks and peak PRP LDA decoding of odorant identity.
(A) Lick time course for 25 S+ trials in one session of EAPAexp2 for one proficient mouse. Horizontal black bar indicates odor delivery epoch for all time course subpanels. (B) Lick time course for 25 S- trials in the same session as in A. Mouse refrains from licking in the presence of the unrewarded odorant. (C) i: p value time courses for difference between S+ and S- for licks and peak PRP LDA predictions. ii and iii: decoding performance for PRP LDA. Decision making times for both licks and PRP LDA were calculated using data pooled for all mice performing odorant discrimination with the EAPAexp2 odorant pair. i: p value for the ranksum test compares the time-course for decision-making using licks for S+ vs. S- (green) or LDA prediction of odorant identity with peak (red) or trough (blue) PRP. This example illustrates that that peak PRP LDA prediction and lick divergence perform similarly, while LDA prediction with trough PRP is significantly slower in stimulus decoding. Horizontal red line indicates p=0.05. ii and iii: LDA decoding performance appears to be faster and more accurate using peak PRP (ii) vs. trough PRP (iii). (D) Decision times for licks and for pooled animal peak theta/beta and theta/high gamma PRP LDA in naïve (i) and proficient (ii) mice. Decision times were significantly reduced with task learning (GLM p value < 0.001, 44 d.f.). Decision times for lick vs. PRP LDA and theta/beta vs. theta/high gamma did not differ (GLM p values > 0.05, 44 d.f.).
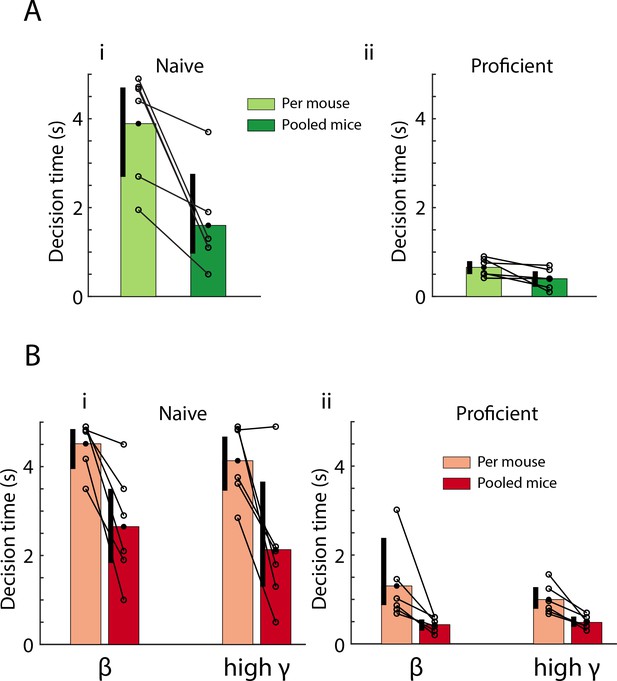
Decision times are faster for peak PRP LFP LDA computed when data are pooled across mice.
(A) Comparison of lick decision times between per mouse (light green) vs. pooled mouse (dark green) p value estimates. GLM p value for the difference in lick decision times between per-mouse vs. pooled GLM <0.01, 20 d.f. (B) Decision times for peak PRP decoding of stimulus identity for the per mouse (light red) vs. pooled mouse (red) LDA for naïve (i) vs. proficient (ii) mice. For both theta/beta and theta/high gamma decoding of stimulus identity via peak PRP is faster using data pooled across mice than the per mouse estimate (GLM p values < 0.001, 20 d.f.). There is no difference in decision-making times between theta/beta and theta/high gamma (GLM p values > 0.05, 20 d.f.). Vertical black bars represent the 95% bootstrap confidence interval of the data in each condition; they are shifted to the left to better display the mean and individual odorant pair values.
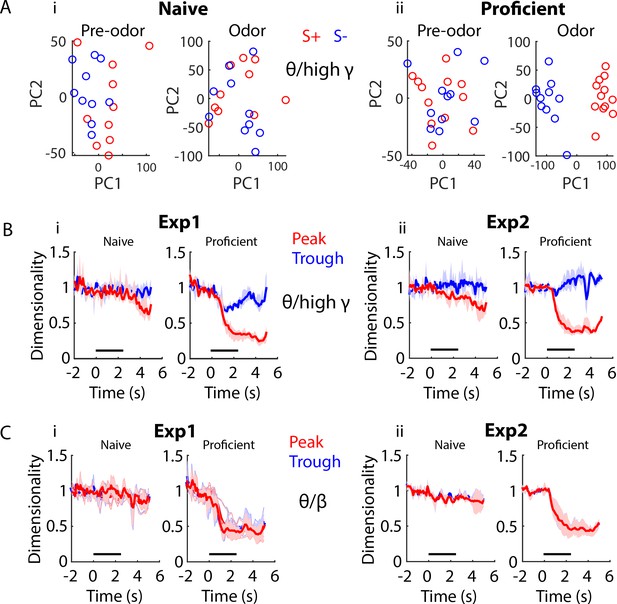
Dimensionality changes with learning and during the time course of the trial.
(A) Scatter plot showing the first two principal components for twenty trials of a PCA for peak theta/high gamma PRP recorded in mice learning to differentiate the odorants in the EAPAexp1 odorant pair. The input to the PCA was the peak PRP recorded in 16 electrodes pooled for all mice. Principal components are intermingled between S+ (red) and S- (blue) trials in the naïve state (left) in pre-odor and odor-delivery epochs, yet they separate during odor delivery upon task acquisition (right). i. Naïve animals. ii. Proficient animals. (B) Within-trial peak (red) and trough (blue) theta/high gamma PRP dimensionality time course for data pooled across mice is shown for Exp1 (i) and Exp2 (ii), normalized to pre-trial dimensionality. A GLM analysis indicates that there are significant differences for time course, proficient vs. naïve, experiments and peak vs. trough, and for pair interactions of these factors (GLM p values < 0.001, 1688 d.f.). (C) Within-trial peak (red) and trough (blue) theta/beta PRP dimensionality time course for data pooled across mice is shown for Exp1 (i) and Exp2 (ii), normalized to pre-trial dimensionality. GLM analysis found statistically significant differences for time course, and proficient vs. naïve, and for interaction of these factors (GLM p values < 0.001, 1688 d.f.), and there was a statistically significant difference between experiments (GLM p value < 0.01, 1688 d.f.). However, there was no difference between peak and trough (GLM p value > 0.05, 1688 d.f).
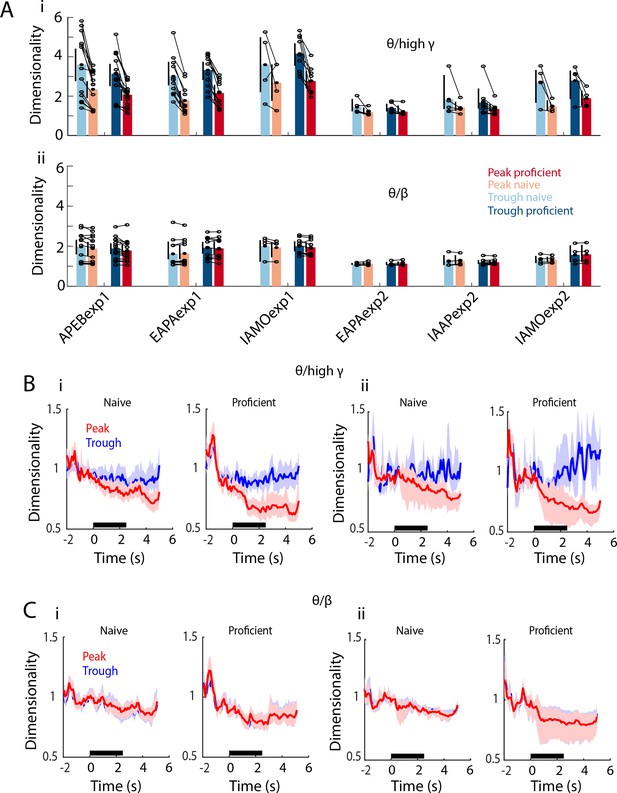
PRP dimensionality estimated per mouse.
(A) Per mouse theta/high gamma (i) and theta/beta (ii) PRP dimensionality summary for all six odorant pairs calculated before odorant delivery. GLM p value < 0.001 for Exp1 vs. Exp2 and >0.05 for peak vs. trough, 379 d.f. (B) Per mouse examples of the time course of theta/high gamma PRP dimensionality for APEBexp1 (i) and IAMOexp2 (ii). A GLM analysis of the dimensionality for peak PRP LFP calculated on a per mouse basis for all odorant pairs indicates that there are significant differences in theta/high gamma peak PRP LFP dimensionality for proficient vs. naïve, time, Exp1 vs. Exp2 and for interactions of these factors (except for the interaction of Exp1 vs. Exp2 x proficient vs. naïve) (GLM p values < 0.001, 6098 d.f.). (C) Per mouse examples of the time course of theta/beta PRP dimensionality for APEBexp1 (i) and IAMOexp2 (ii). A GLM analysis of the dimensionality for theta/beta peak PRP LFP calculated on a per mouse basis for all odorant pairs indicates that there are significant differences for proficient vs. naïve, time, Exp1 vs. Exp2 and for interactions of these factors (GLM p values < 0.01, 6098 d.f.). For theta/high gamma peak PRP LFP dimensionality there was a statistically significant difference between peak and trough (GLM p value < 0.0001, 12196 d.f.). and for theta/beta peak PRP LFP dimensionality there was no difference between peak and trough (GLM p value > 0.05, 12196 d.f.).
Tables
Reagent type | Reagent or resource | Source | Identifier | Additional information |
---|---|---|---|---|
Chemical compound, drug | Isoamyl acetate | Sigma-Aldrich | Cat#123-92-2 | Odorant |
Chemical compound, drug | Ethyl acetate | Sigma-Aldrich | Cat# 141-78-6 | Odorant |
Chemical compound, drug | Propyl acetate | Sigma-Aldrich | Cat# 109-60-4 | Odorant |
Chemical compound, drug | Mineral oil | Sigma-Aldrich | Cat# 8042-47-5 | Odorant |
Chemical compound, drug | Acetophenone | Sigma-Aldrich | Cat# 98-86-2 | Odorant |
Chemical compound, drug | Ethyl benzoate | Sigma-Aldrich | Cat# 93-89-0 | Odorant |
Other | Nickel-chrome wire | Sandvik | Cat#PX000002 | Tetrode fabrication |
Other | 16 Channel Electrode Interface Board | Neuralynx | EIB-16 | Tetrode fabrication |
Other | LFP Data | GigaDB | http://doi.org/10.5524/100699 | Losacco et al., 2020 |
Software, algorithm | Analysis code | This paper | Available on github and by request | https://github.com/restrepd/drgMaster |
Strain, strain background | Mouse: C57BL/6J | Jackson Lab | RRID: IMSR_JAX:000664 | Male mice |
Strain, strain background | Mouse: Tg(Dbh-cre)KH212Gsat/Mmucd | Mutant Mouse Resource and Research Center | 032081-UCD, RRID:MMRRC | All sexes used |
Strain, strain background | Mouse: eNpHR3.0, 129S-Gt(ROSA)26Sortm39(CAG-hop/EYFP) Hze/J | Jackson Lab | 014539, RRID:SCR_004633 | All sexes used |
Software, algorithm | MATLAB_R2018a | Mathworks | RRID: SCR_001622 | |
Software, algorithm | Illustrator | Adobe | RRID: SCR_010279 | |
Software, algorithm | Photoshop | Adobe | RRID: SCR_014199 | |
Strain, strain background | Mouse: OMP-hChR2V | In house | OMP-hChR2V | Li et al., 2014 |
Additional files
-
Supplementary file 1
This file includes two tables: Table S1. Odorant pairs, implant device, mouse genotype and electrode locations for each experiment Odorant identities for each pair are listed along with concentrations represented as volume/volume dilutions in mineral oil (MO). In the case of EAPAexp1, EA is 0.1% ethyl acetate and PA is a mixture of 0.05% ethyl acetate + 0.05% propyl acetate. The two recording locations (Exp1 and Exp2) are listed in millimeters with respect to bregma. Note: Cre expression can affect physiological parameters and therefore, the differences found between experiments in this publication can be due to Cre expression (Harno et al., 2013). However, it is not evident to us which of the methodological differences between experiments contributes to statistical differences in measured parameters. Table S2. Total sessions per mouse, experiment and odorant pair. Odorant pair abbreviations derived from Table S1. The number of sessions are in the 'odorant pair' columns. Each row represents one animal.
- https://cdn.elifesciences.org/articles/52583/elife-52583-supp1-v1.docx
-
Transparent reporting form
- https://cdn.elifesciences.org/articles/52583/elife-52583-transrepform-v1.pdf