Robust and distributed neural representation of action values
Figures
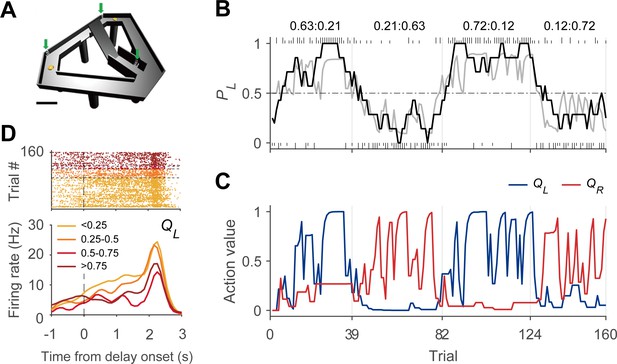
Dynamic foraging task.
(A) Modified T-maze. Rats chose freely between two targets (orange circles) to obtain water reward. Rats navigated from the central stem to either target and returned to the central stem via the lateral alley to start a new trial. A delay (2–3 s) was imposed at the beginning of a new trial by raising the central bridge. Green arrows, photobeam sensors. Scale bar, 10 cm. (B) Behavioral data from a sample session (Kim et al., 2013). The black curve shows the probability to choose the left target (PL) in moving average of 10 trials. The gray curve denotes the probability to choose the left target predicted by the Q-learning model. Tick marks denote trial-by-trial choices of the rat (upper, left choice; lower, right choice; long, rewarded trial; short, unrewarded trial). Vertical gray lines denote block transitions and numbers above indicate reward probabilities of the left and right targets in each block. (C) Trial-by-trial action values of the sample session computed with the Q-learning model. Blue, left-choice action value (QL); Red, right-choice action value (QR). (D) An example DMS unit showing activity correlated with left-choice action value. Trials were grouped into quartiles of left-choice action value. Delay onset is when the rat broke the photobeam sensor on the central stem.
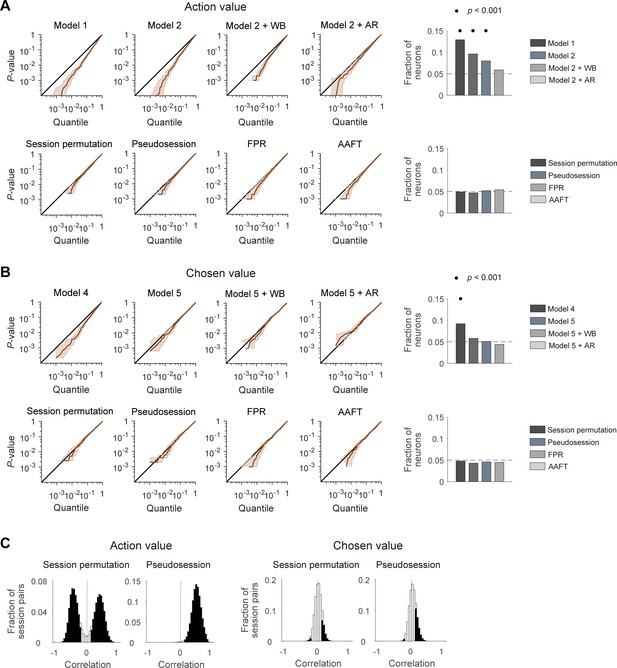
Performances of different statistical tests for action-value and chosen-value signals.
(A) Left, cumulative density functions (CDFs) of p-values for the neural activity related to action value were determined with different analysis methods using null neural data to assess false positive rates. Results obtained with the methods used in previous studies (top), including the t-test in two different regression models (models 1 and 2), within-block permutation applied to model 2 (model 2 + WB), and model 2 with autoregressive terms (model 2 + AR), as well as those obtained with the methods based on surrogate data (bottom) are shown. Right, fractions of neurons significantly responsive to either action value (p<0.025 for QL or QR). Horizontal dotted lines denote 5%. Significant fractions (binomial test) are indicated by black filled circles. (B) Left, CDFs of p-values for the neural activity related to chosen value. Right, fractions of neurons significantly responsive to chosen value (p<0.05 for Qc). The same format as in B, but models 4 and 5 were used instead of models 1 and 2, respectively. (C) Correlation between action values (left) or chosen values (right) calculated from the original and resampled behavioral data either from other sessions (session permutation, n = 382) or simulated behavioral sessions (pseudosession, n = 500). Filled bars indicate significant (t-test, p<0.05) correlations.
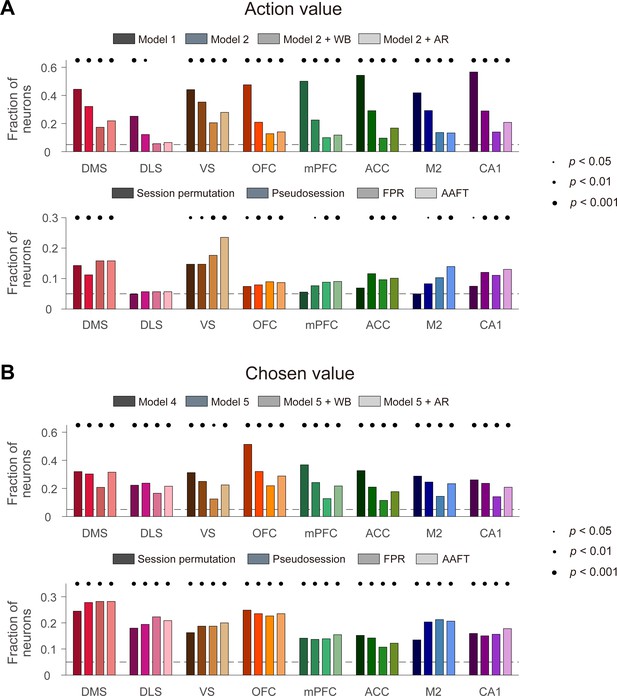
Action-value and chosen-value signals in multiple brain regions.
Action-value and chosen-value neurons were determined based on actual behavioral data and actual neural data recorded from several different areas of the rat brain. Shown are fractions of neurons significantly responsive to either action value (p<0.025 for QL or QR; A) or chosen value (p<0.05 for Qc; B) determined with the previous methods (top) or resampling-based methods (bottom). Significant fractions (binomial test) are indicated by black filled circles.
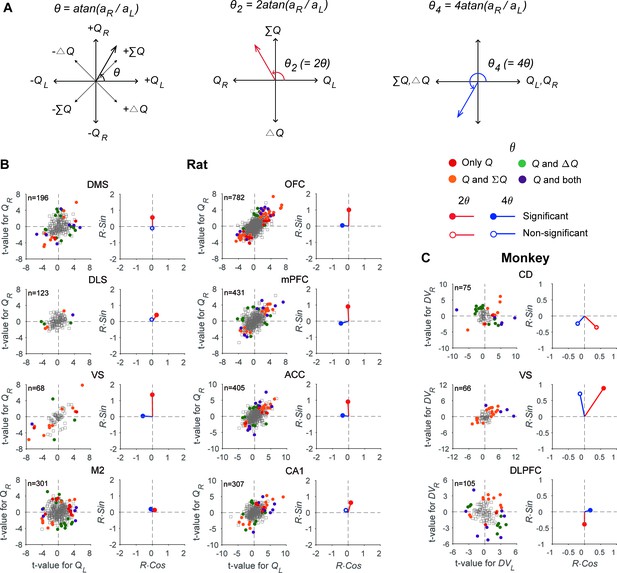
Neural signals related to action value, policy, and state value.
(A) Transformations applied to the angle defined by the original regression coefficients (θ) to examine multiple types of value signals (θ2 for signals related to policy vs. state value; θ4 for action values vs. other value signals). (B) The scatter plots show t-values for the left and right action values (abscissa and ordinate, respectively) estimated from neural activity recorded in different areas of the rat brain. Filled circles denote those neurons significantly responsive to one or more of the decision variables tested (QL, QR, ΔQ, and ΣQ). Q, those neurons significantly responsive to either action value (p<0.025 for QL or QR). The vectors on the right panel for each area show mean vectors computed after doubling (2θ, red) or quadrupling (4θ, blue) the angle of each data point in the scatter plots. Red filled circles, the Y-component of the mean vector is significantly different from 0; blue filled circles, the X-component of the mean vector is significantly different from 0 (Wilcoxon rank-sum test, p<0.05). (C) The same scatter and vector plots for monkey striatal and DLPFC neurons. DVL, left action value; DVR, right action value.
Additional files
-
Supplementary file 1
Statistical test results for 2θ and 4θ plots.
Top, statistical test results for 2θ plots. Orange shading, Y-component of the mean vector was tested for significant deviation from 0 (Wilcoxon rank-sum test, red indicates p-values <0.05). No shading, Y-component of the mean vector was compared across regions using one-way ANOVA (rat, F(7,2587) = 12.64, p=4.9 × 10−16; monkey, F(2,247) = 10.75, p=3.4 × 10−5) followed by Bonferroni post hoc tests. Significant differences (p-values <0.05) between regions are indicated in red. Bottom, statistical test results for 4θ plots. Orange shading, X-component of the mean vector was tested for significant deviation from 0 (Wilcoxon rank-sum test, red indicates p-values<0.05). No shading, X-component of the mean vector was compared across regions using one-way ANOVA (rat, F(7,2587) = 3.79, p=4.3 × 10−4; monkey, F(2,247) = 0.95, p=0.387) followed by Bonferroni post hoc tests. Significant differences (p-values <0.05) between regions are indicated in red.
- https://cdn.elifesciences.org/articles/53045/elife-53045-supp1-v2.docx
-
Transparent reporting form
- https://cdn.elifesciences.org/articles/53045/elife-53045-transrepform-v2.docx