Microstructural organization of human insula is linked to its macrofunctional circuitry and predicts cognitive control
Figures
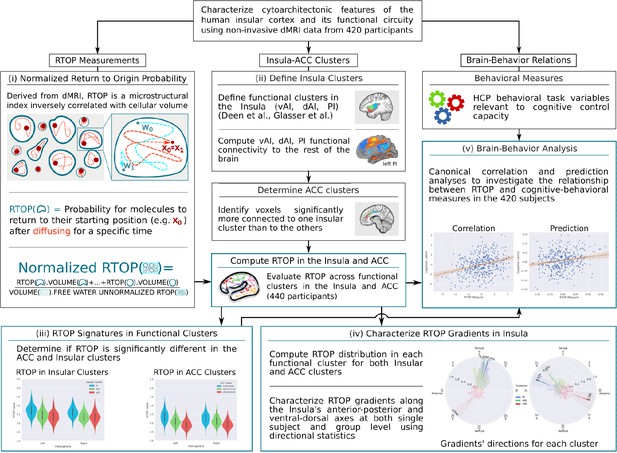
Flow chart illustrating data analysis pipeline.
(A) Main components of human insula microstructure analysis using diffusion magnetic resonance imaging (dMRI) data from 413 Human Connectome Project (HCP) participants. Key steps include: (i) measurement of microstructure features based on Return to Origin Probability (RTOP), the ratio between the probability of molecules returning to their starting position in biological tissue versus free diffusion, (ii) demarcation of functional subdivisions in insula and its interconnected anterior cingulate cortex (ACC) subdivisions, which together anchor the salience network, (iii) computation of microstructure features of the insula within its functional subdivisions and its interconnected ACC subdivisions, (iv) computation of microstructural gradients along the anterior-posterior and dorsal-ventral axes of the insula, and (v) analysis of relation between insula microstructural organization and cognitive control abilities.
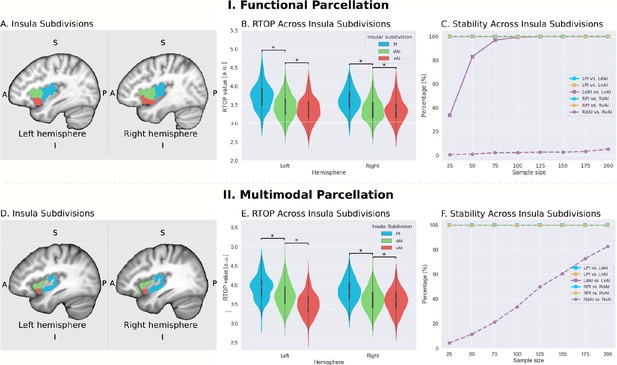
Microstructural properties of functional insular cortex subdivisions.
(A) Functional subdivisions spanning the posterior insula (PI), dorsal anterior insula (dAI) and ventral anterior insula (vAI) (Deen et al., 2011). (B) RTOP differs significantly between PI, dAI and vAI in the left hemisphere and between PI and dAI or vAI in the right hemisphere (p<0.001, Bonferroni corrected). The right vAI has the smallest RTOP value among all the subdivisions (all ps < 0.001, except p=0.036 for right vAI < dAI; Bonferroni corrected). (C) Stability of findings as a function of sample size. A sample size of N = 25 was sufficient to achieve a stable differentiation (p<0.01) between PI and vAI in both hemispheres, while differentiating the vAI and dAI required a larger sample size. a PI: posterior insula; dAI: dorsal anterior insula; vAI: ventral anterior insula. (D–F) Replication with an independent multimodal parcellation using HCP data (Glasser et al., 2016).
-
Figure 2—source data 1
Data for Figure 2 panel B.
- https://cdn.elifesciences.org/articles/53470/elife-53470-fig2-data1-v2.csv
-
Figure 2—source data 2
Data for Figure 2 panel E.
- https://cdn.elifesciences.org/articles/53470/elife-53470-fig2-data2-v2.csv
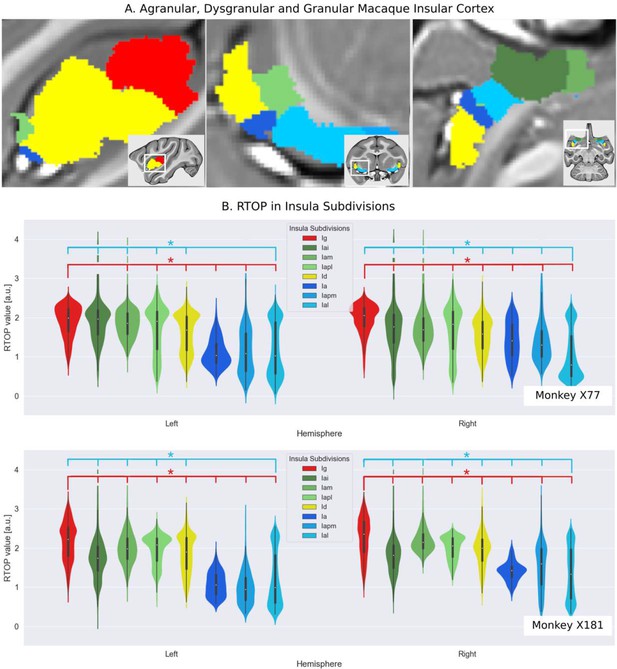
RTOP measurements validate the cytoarchitectonic organization of the macaque insular cortex in vivo.
(A) Cytoarchitectonic subdivisions of the insula in the macaque monkey: Granular (red), Dysgranular (yellow), and Agranular (divided in three green dorsal regions, and three blue ventral ones) (B) Distribution of RTOP values inside the cytoarchitectonic insular subdivisions for the two monkeys (X77 and X181), ordered based on their RTOP values. RTOP inversely correlates with expected average neuron size: RTOP is significantly lower in the lateral agranular insula (Ial) region (p<0.001, blue line) compared to other insular subdivisions (except adjoining ventral regions in left hemisphere: posteromedial agranular insula, Iapm, and agranular insula, Ia). Granular insula (Ig) region has significantly higher RTOP values than other insular subdivisions, except for the intermediate agranular insula (Iai) in monkey X77 (p<0.001, red line).
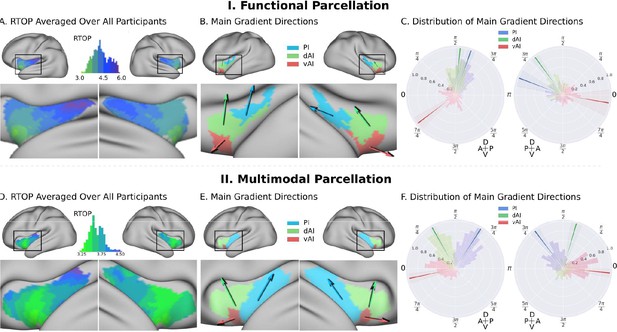
Insula microstructure gradients along its anterior-posterior and dorsal-ventral axes.
(A) RTOP averaged over all participants (N = 413) illustrates inhomogeneity in insula microstructure with a ventral anterior insula peak, and gradients along the anterior-posterior and dorsal-ventral gradients axes. Larger RTOP indicates smaller average compartments. There is a prominent gradient from the insular pole towards the posterior insular section. Note right hemisphere dominance. (B) Main gradient directions, computed using Rayleigh directional statistics in each functionally defined subdivision. The main directions show an anterior-to-posterior and inferior-to-superior RTOP organization in the left insular cortex and an anterior-to-posterior organization in the right insular cortex. The polar plots show the distribution of main gradient directions in each functional subdivision. (C) Gradient direction histograms. The mean direction is represented with solid lines on top of the distribution histogram; the shaded region represents the 95% confidence interval. For detailed statistics (Table S1). (D–F) Replication with an independent multimodal parcellation using HCP data (Glasser et al., 2016).
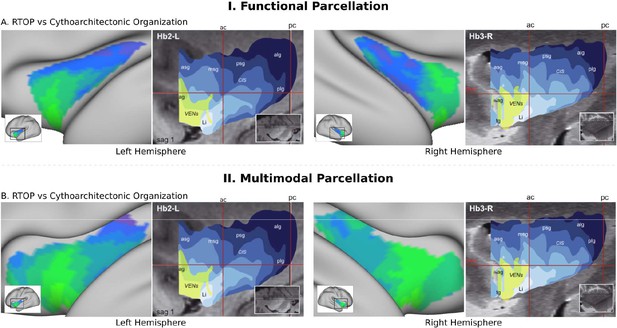
RTOP isocontours reflect insula cytoarchitectonic organization.
Isocontours of the population-average RTOP (left) are closely aligned to cytoarchitectonic organization of the insula and VEN expression from studies of post-mortem brains (right, based on Morel et al., 2013). (B) Replication with an independent.
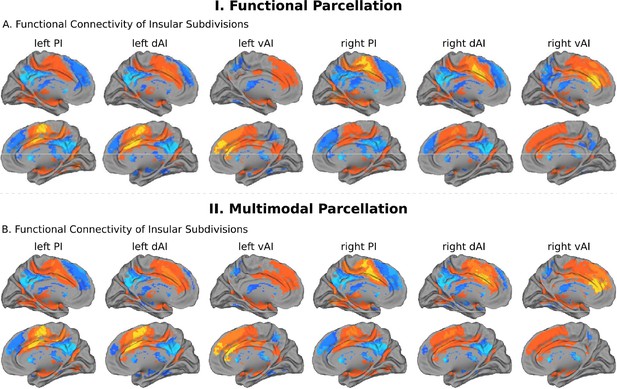
Intrinsic functional connectivity of insula subdivisions dAI, vAI and PI.
(A) Whole-brain resting-state functional connectivity analysis revealed distinct functional connectivity patterns of the insula subdivisions (Deen et al., 2011), bilateral posterior insula (PI), dorsal anterior insula (dAI) and ventral anterior insula (vAI) (all ps < 0.001, FDR corrected). (B) Replication with an independent multimodal parcellation using HCP data (Glasser et al., 2016).
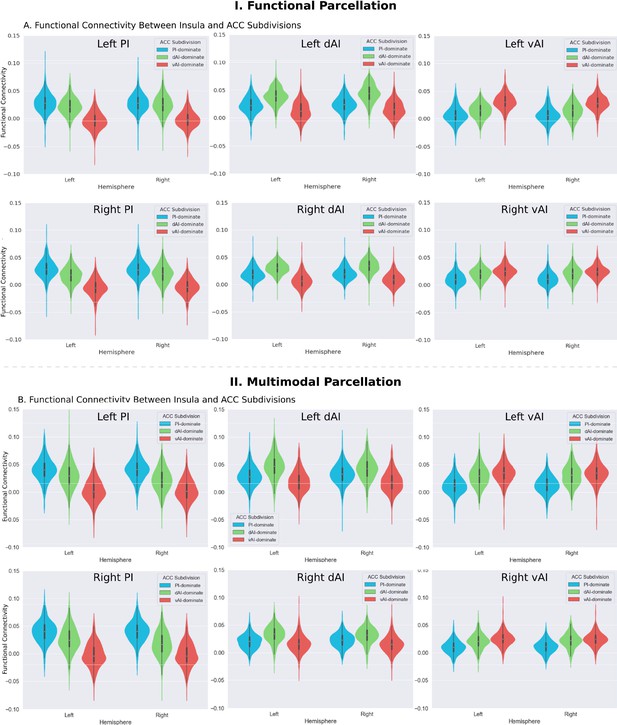
Functional connectivity between insula and ACC subdivisions.
(A) RTOP differs across ACC functional subdivisions differentially linked to the three insular subdivisions (Deen et al., 2011). (B) Replication with an independent multimodal parcellation using HCP data (Glasser et al., 2016).
-
Figure 6—source data 1
Data for Figure 6 panel A.
- https://cdn.elifesciences.org/articles/53470/elife-53470-fig6-data1-v2.csv
-
Figure 6—source data 2
Data for Figure 6 panel B.
- https://cdn.elifesciences.org/articles/53470/elife-53470-fig6-data2-v2.csv
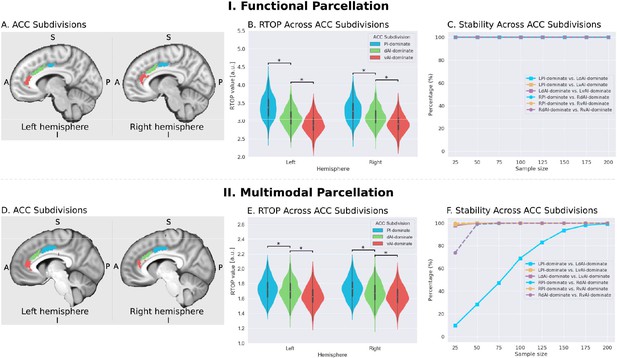
Microstructural properties of anterior cingulate cortex subdivisions mirror profiles in corresponding insula functional subdivisions.
(A) Illustration of ACC subdivisions. Each ACC subdivision preferentially connects to one of the three insular subdivisions defined using an independent functional parcellation (Deen et al., 2011). ACC subdivisions showed significantly greater functional connectivity with one insula subdivision over others (e.g. right PI > right dAI) and (right PI > right vAI) (all ps < 0.01, FDR corrected). (B) RTOP values were significantly different among the three ACC subdivisions in each hemisphere (p<0.001, Bonferroni corrected). The ACC subdivision differentially connected to vAI has smaller RTOP values than the other subdivisions (all ps < 0.001, Bonferroni corrected). (C) RTOP differences among three ACC subdivisions were robust and reliable at sample sizes of N = 25 or more. PI: posterior insula; dAI: dorsal anterior insula; vAI: ventral anterior insula. (D–E) Replication with an independent multimodal parcellation using HCP data (Glasser et al., 2016).
-
Figure 7—source data 1
Data for Figure 7 panel B.
- https://cdn.elifesciences.org/articles/53470/elife-53470-fig7-data1-v2.csv
-
Figure 7—source data 2
Data for Figure 7 panel E.
- https://cdn.elifesciences.org/articles/53470/elife-53470-fig7-data2-v2.csv
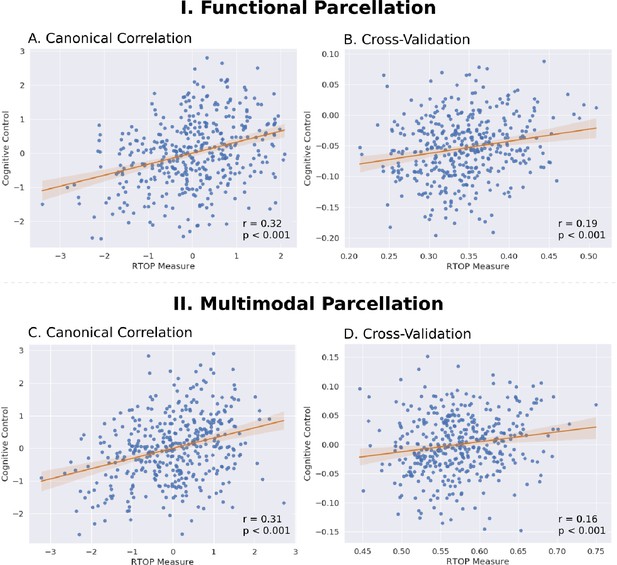
Insula microstructural features predict cognitive control abilities.
(A) Canonical correlation analysis (CCA) revealed a significant relationship between mean RTOP in each insular subdivision (Deen et al., 2011) and cognitive control measures. CCA weights of RTOP measures were significantly correlated with CCA weights of cognitive control measures in CCA Axis 1. (B) Cross-validation analysis revealed that CCA-derived weights of RTOP predicted cognitive control measures on unseen data. (C–D) Replication with an independent multimodal parcellation using HCP data (Glasser et al., 2016).
-
Figure 8—source data 1
Data for Figure 8 panel A.
- https://cdn.elifesciences.org/articles/53470/elife-53470-fig8-data1-v2.csv
-
Figure 8—source data 2
Data for Figure 8 panel B.
- https://cdn.elifesciences.org/articles/53470/elife-53470-fig8-data2-v2.csv
-
Figure 8—source data 3
Data for Figure 8 panel C.
- https://cdn.elifesciences.org/articles/53470/elife-53470-fig8-data3-v2.csv
-
Figure 8—source data 4
Data for Figure 8 panel D.
- https://cdn.elifesciences.org/articles/53470/elife-53470-fig8-data4-v2.csv
Tables
Reagent type (species) or resource | Designation | Source or reference | Identifiers | Additional information |
---|---|---|---|---|
Other (Human) | Human Connectome Project (HCP) | Glasser et al., 2013 (doi: 10.1016/j.neuroimage.2013.04.127) | Dataset: WU-Minn HCP Data - 1200 | |
Other (Macaque) | NKI PRIMatE Data Exchange database (PRIME) | Milham et al., 2018 (doi: 10.1016/j.neuron.2018.08.039) | Dataset: Princeton NA&P Lab | |
Software, algorithm | DIPY | Dipy Contributors et al., 2014 (doi:10.3389/fninf.2014.00008) |
Additional files
-
Supplementary file 1
Contains the von Mises-Fisher statistics for the three insula subdivisions dAI, vAI, and PI for each functional parcellation-derived subdivision (Deen et al., 2011) in Supplementary Table 1; as well as for each multimodal parcellation-derived subdivision (Glasser et al., 2016) in Supplementary Table 2.
It also contains the Microstructural properties of insular subdivisions in the macaque brain in Supplementary Table 3. Finally, the sorted weights of the first component in brain-behavior canonical correlation analysis for the analysis using functional parcellations of the insula (Deen et al., 2011) is included in Supplementary Table 4; and the result of the same analysis using multimodal parcellations of the insula (Glasser et al., 2016) is included in Supplementary Table 5.
- https://cdn.elifesciences.org/articles/53470/elife-53470-supp1-v2.docx
-
Transparent reporting form
- https://cdn.elifesciences.org/articles/53470/elife-53470-transrepform-v2.docx