Deciphering the regulatory genome of Escherichia coli, one hundred promoters at a time
Figures
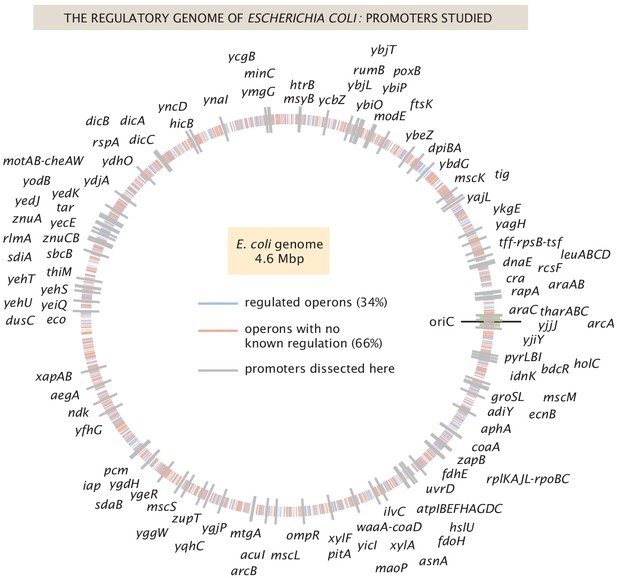
The E. coli regulatory genome.
Illustration of the current ignorance with respect to how genes are regulated in E. coli. Genes with previously annotated regulation (as reported on RegulonDB [Gama-Castro et al., 2016]) are denoted with blue ticks and genes with no previously annotated regulation denoted with red ticks. The 113 genes explored in this study are labeled in gray, and their precise genomic locations can be found in Figure 1—source data 1.
-
Figure 1—source data 1
Locations of TSS for all promoters in Figure 1.
In Figure 1 the locations of all promoters studied in Reg-Seq are displayed along the E. coli genome. The source data contains the exact position of the '0' position of each mutagenized promoter region.
- https://cdn.elifesciences.org/articles/55308/elife-55308-fig1-data1-v2.csv
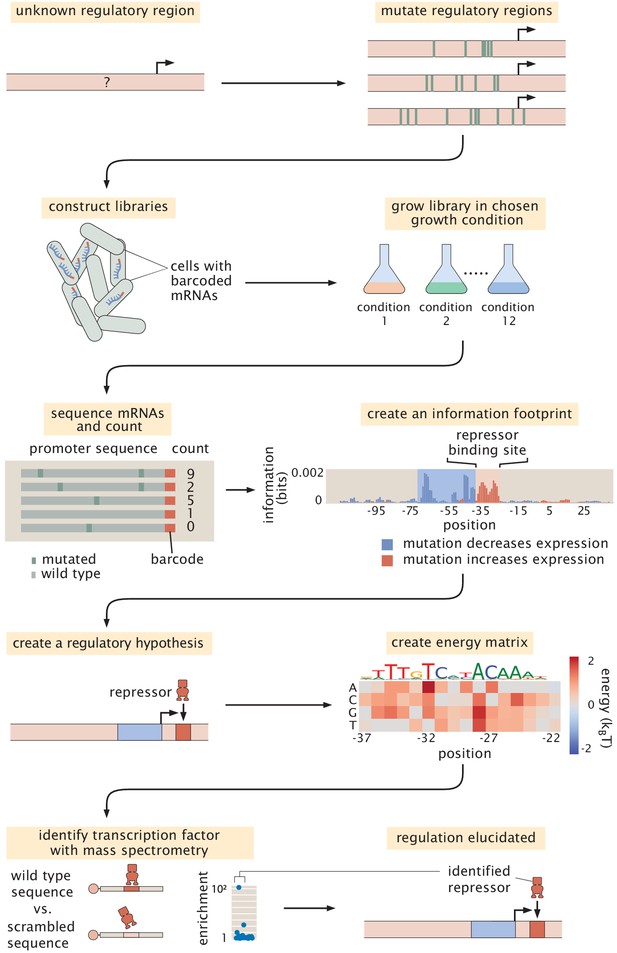
Schematic of the Reg-Seq procedure as used to recover a repressor-binding site.
The process is as follows: After constructing a promoter library driving expression of a randomized barcode (an average of five barcodes for each promoter), RNA-Seq is conducted to determine the frequency of these mRNA barcodes across different growth conditions (list included in Appendix 1 Section 'Growth conditions'). By computing the mutual information between DNA sequence and mRNA barcode counts for each base pair in the promoter region, an 'information footprint' is constructed that yields a regulatory hypothesis for the putative binding sites (with the RNAP-binding region highlighted in blue and the repressor-binding site highlighted in red). Energy matrices, which describe the effect that any given mutation has on DNA-binding energy, as well as sequence logos, are inferred for the putative transcription-factor-binding sites. Next, we identify which transcription factor preferentially binds to the putative binding site via DNA-affinity chromatography followed by mass spectrometry. This procedure culminates in a coarse-grained, cartoon-level view of our regulatory hypothesis for how a given promoter is regulated.
-
Figure 2—source data 1
Information footprint data displayed in Figure 2.
- https://cdn.elifesciences.org/articles/55308/elife-55308-fig2-data1-v2.xlsx
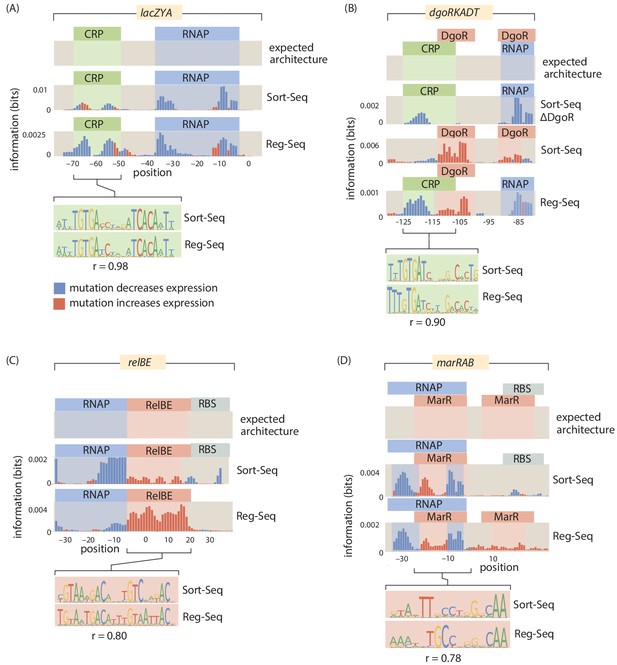
A summary of four direct comparisons of measurements from Sort-Seq and Reg-Seq.
We show the identified regulatory regions as well as quantitative comparisons between inferred position weight matrices. (A) CRP binds upstream of RNAP in the lacZYA promoter. Despite the different measurement techniques for the two inferred position weight matrices, the CRP-binding sites have a Pearson correlation coefficient of . (B) The dgoRKADT promoter is activated by CRP in the presence of galactonate and is repressed by DgoR. For Sort-Seq and Reg-Seq, type II activator-binding sites can be identified based on the signals in the information footprint in the area indicated in green. Additionally, the quantitative agreement between the CRP position weight matrices are strong, with . (C) The relBE promoter is repressed by RelBE as can be identified algorithmically in both Sort-Seq and Reg-Seq. The inferred logos for the two measurement methods have . (D) The marRAB promoter is repressed by MarR. The inferred energy matrices (data not shown) and sequence logos shown have . The right most MarR site overlaps with a ribosome-binding site. The overlap has a stronger obscuring effect on the sequence specificity of the Sort-Seq measurement, which measures protein levels directly, than it does on the output of the Reg-Seq measurement. Numeric values for the displayed data can be found in Figure 3—source data 1.
-
Figure 3—source data 1
Data for information footprints and PWMs in Figure 3.
- https://cdn.elifesciences.org/articles/55308/elife-55308-fig3-data1-v2.xlsx
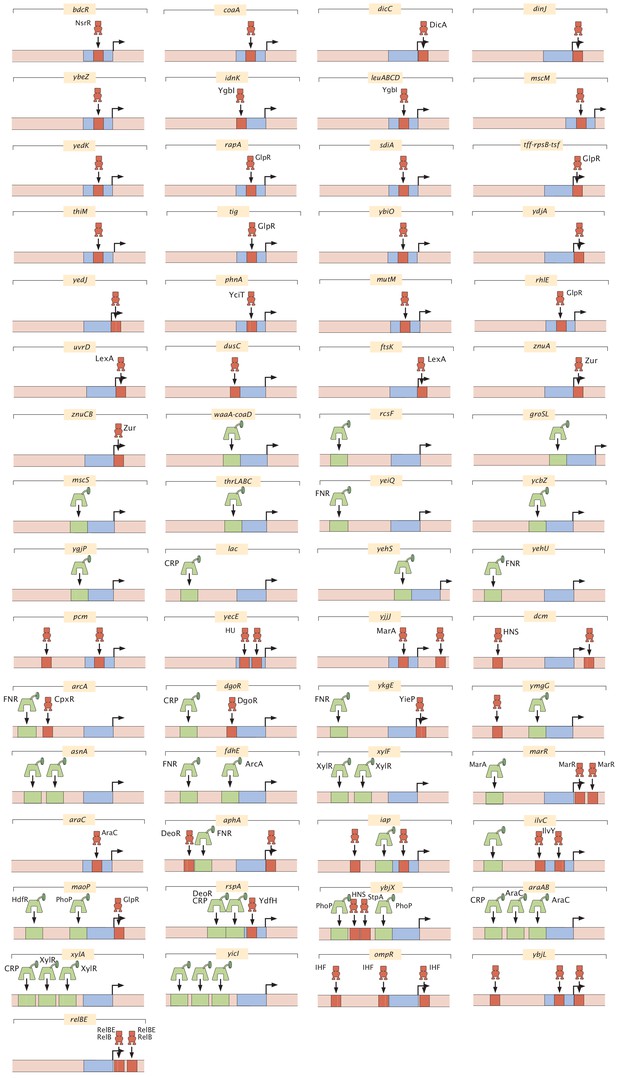
All regulatory architectures uncovered in this study.
For each regulated promoter, activators and their binding sites are labeled in green, repressors and their binding sires are labeled in red, and RNAP-binding sites are labeled in blue. All cartoons are displayed with the transcription direction to the right. Only one RNAP site is depicted per promoter. The transcription-factor-binding sites displayed have either been identified by the method described in the Section 'Automated putative binding site algorithm' or have additional evidence for their presence as described in Table 2. Binding sites found for these promoters in the EcoCyc or RegulonDB databases are only depicted in these cartoons if the sites are within the 160 bp mutagenized region studied, and are detected by Reg-Seq.
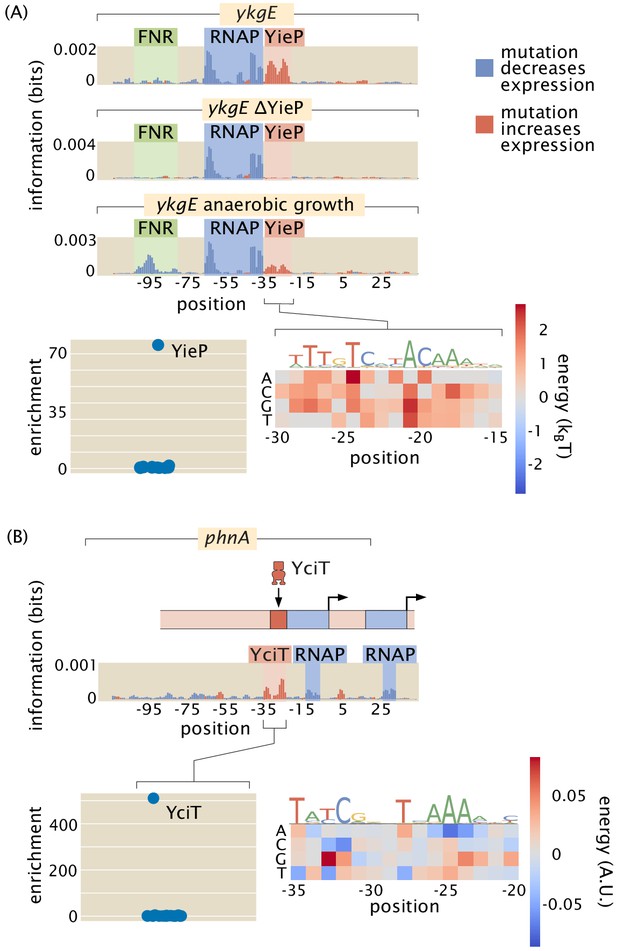
Examples of the insight gained by Reg-Seq in the context of promoters with no previously known regulatory information.
Activator-binding regions are highlighted in green, repressor binding regions in red, and RNAP binding regions in blue. (A) From the information footprint of the ykgE promoter under different growth conditions, we can identify a repressor-binding site downstream of the RNAP-binding site. From the enrichment of proteins bound to the DNA sequence of the putative repressor as compared to a control sequence, we can identify YieP as the transcription factor bound to this site as it has a much higher enrichment ratio than any other protein. Lastly, the binding energy matrix for the repressor site along with corresponding sequence logo shows that the wild-type sequence is the strongest possible binder and it displays an imperfect inverted repeat symmetry. (B) Illustration of a comparable dissection for the phnA promoter. Numeric values for the displayed data can be found in Figure 5—source data 1.
-
Figure 5—source data 1
Data for information footprints, energy matrices, PWMs, and mass spectrometry in Figure 5.
- https://cdn.elifesciences.org/articles/55308/elife-55308-fig5-data1-v2.xlsx
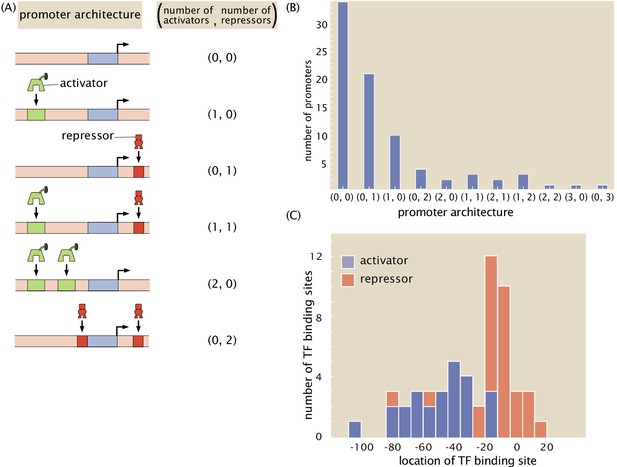
A summary of regulatory architectures discovered in this study.
(A) The cartoons display a representative example of each type of architecture, along with the corresponding shorthand notation. (B) Counts of the different regulatory architectures discovered in this study. We exclude the 'gold-standard' promoters (listed in Appendix 2—table 1) unless new transcription factors are also discovered in the promoter. If, for example, one repressor was newly discovered and two activators were previously known, then the architecture is still counted as a (2,1) architecture. (C) Distribution of positions of binding sites discovered in this study for activators and repressors. Only newly discovered binding sites are included in this figure. The position of the transcription-factor-binding sites are calculated relative to the estimated TSS location, which is based on the location of the associated RNAP site. Numeric values for the binding locations can be found in Figure 6—source data 1.
-
Figure 6—source data 1
Data for binding site locations in Figure 6.
- https://cdn.elifesciences.org/articles/55308/elife-55308-fig6-data1-v2.xlsx
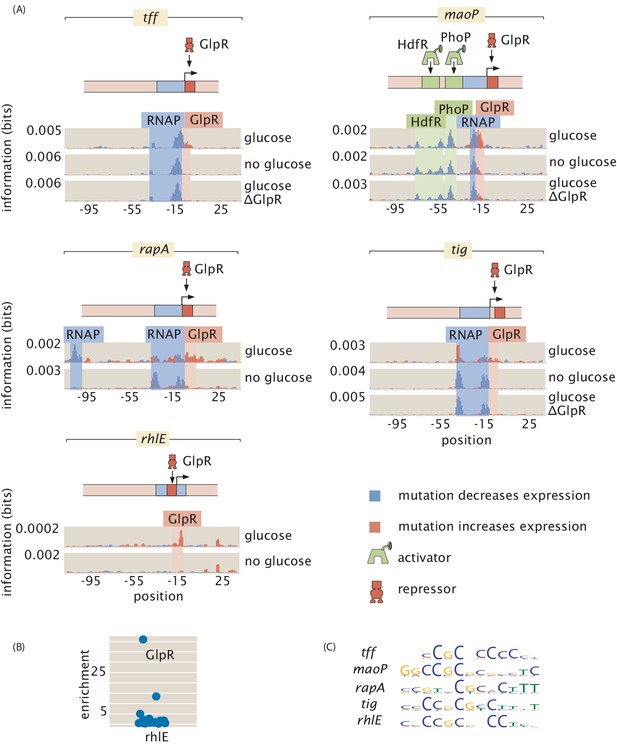
GlpR as a widely acting regulator.
(A) Information footprints for the promoters which we found to be regulated by GlpR, all of which were previously unknown. Activator-binding regions are highlighted in green, repressor-binding regions in red, and RNAP-binding regions in blue. (B) GlpR was demonstrated to bind to rhlE by mass spectrometry. (C) Sequence logos for GlpR-binding sites. Binding sites in the promotes of tff, tig, maoP, rhlE, and rapA have similar DNA binding preferences as seen in the sequence logos and each transcription-factor-binding site binds strongly only in the presence of glucose (As shown in (A)). These similarities suggest that the same transcription factor binds to each site. To test this hypothesis, we knocked out GlpR and ran the Reg-Seq experiments for tff, tig, and maoP. In (A), we see that knocking out GlpR removes the binding signature of the transcription factor. Numeric values for the binding locations can be found in Figure 7—source data 1.
-
Figure 7—source data 1
Data for information footprints, PWMs, and mass spectrometry in Figure 7.
- https://cdn.elifesciences.org/articles/55308/elife-55308-fig7-data1-v2.xlsx
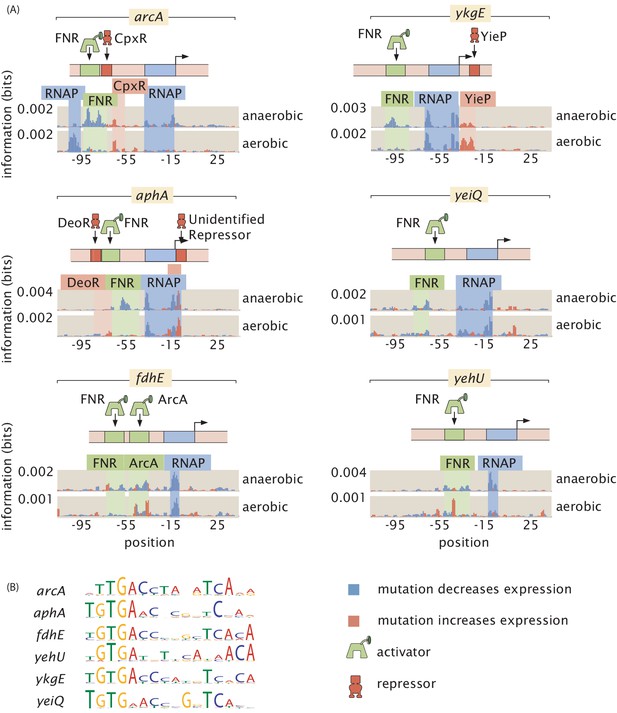
FNR as a global regulator.
FNR is known to be upregulated in anaerobic growth, and here we found it to regulate a suite of six genes. In aerobic growth conditions, the putative FNR sites are weakened. (A) Information footprints for the six regulated promoters. Activator binding regions are highlighted in green, repressor-binding regions in red, and RNAP binding regions in blue. (B) Sequence logos for the FNR-binding sites displayed in (A). The DNA binding preference of the six sites are shown to be similar from their sequence logos. Numeric values for the binding locations can be found in Figure 8—source data 1.
-
Figure 8—source data 1
Data for information footprints and PWMs in Figure 8.
- https://cdn.elifesciences.org/articles/55308/elife-55308-fig8-data1-v2.xlsx
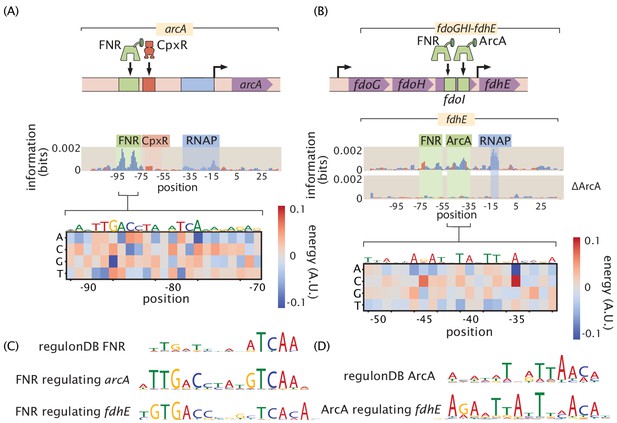
Inspection of a genetic circuit.
(A) Here, the information footprint of the arcA promoter is displayed along with the energy matrix describing the discovered FNR-binding site. (B) Intra-operon regulation of fdhE by both FNR and ArcA. The information footprint of fdhE is displayed. The discovered sites for FNR and ArcA are highlighted and the energy matrix for ArcA is displayed. A TOMTOM (Gupta et al., 2007) search of the binding motif found that ArcA was the most likely candidate for the transcription factor. The displayed information footprint from a knockout of ArcA demonstrates that the binding signature of the site, and its associated RNAP site, are no longer determinants of gene expression. (C) Sequence logos for FNR generated from both the sites cataloged in RegulonDB, as well as the discovered sites regulating arcA and fdhE. (D) Sequence logos for ArcA from sites contained in RegulonDB and the ArcA site regulating fdhE. Numeric values for the binding locations can be found in Figure 9—source data 1.
-
Figure 9—source data 1
Data for information footprints, energy matrices, and PWMs in Figure 9B.
- https://cdn.elifesciences.org/articles/55308/elife-55308-fig9-data1-v2.xlsx
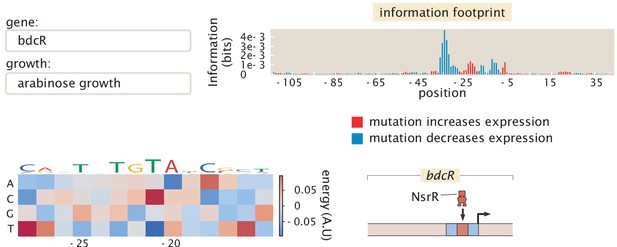
Representative view of the interactive figure that is available online.
This interactive figure captures the entirety of our dataset. Each figure features a drop-down menu of genes and growth conditions. For each such gene and growth condition, there is a corresponding information footprint revealing putative binding sites, an energy matrix that shows the strength of binding of the relevant transcription factor to those binding sites and a cartoon that schematizes the newly-discovered regulatory architecture of that gene. Numeric values for the binding locations can be found in Figure 10—source data 1.
-
Figure 10—source data 1
Data for information footprints, energy matrices, and PWMs in Figure 10.
- https://cdn.elifesciences.org/articles/55308/elife-55308-fig10-data1-v2.xlsx
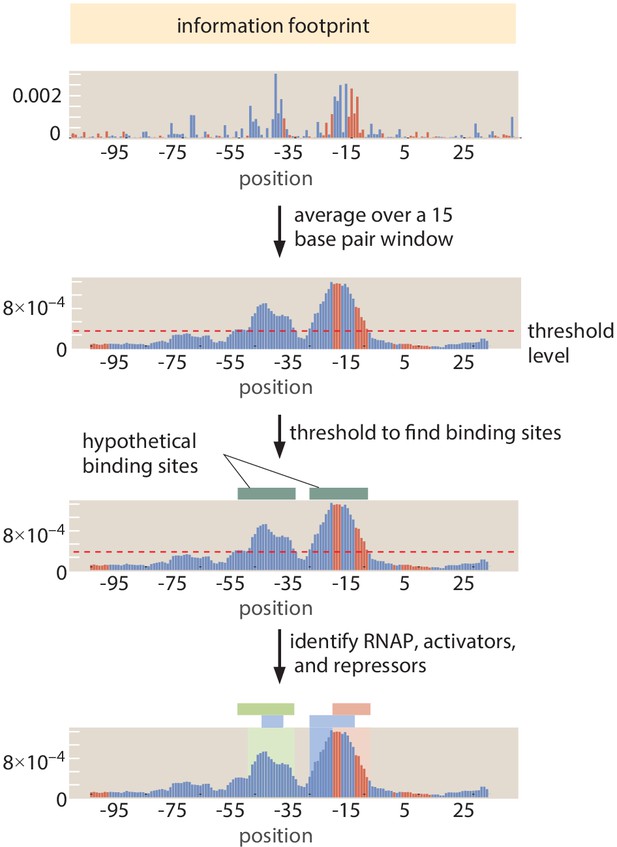
Procedure to identify binding site regions automatically.
First, an information footprint is generated for the target region. Next, the information footprint is smoothed over a 15 base pair sliding window and a threshold of bits is applied to identify regions of interest. RNAP-binding sites are first identified (in blue), and the remainder of the regulatory regions are identified as repressor-binding sites (if they tend to increase expression on mutation from wild type) or activator-binding sites (if they tend to decrease expression upon mutation).
-
Figure 11—source data 1
Information footprint data displayed in Figure 11.
- https://cdn.elifesciences.org/articles/55308/elife-55308-fig11-data1-v2.xlsx
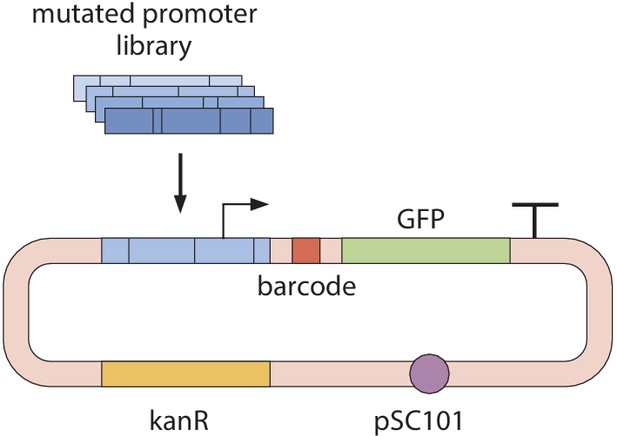
Schematic of the genetic construct used in this study.
Mutated DNA libraries for each regulatory region were expressed from a pSC101 plasmid with kanamycin resistance (kanR). Each mutated sequence is 160 bp in length, which includes 45 bp downstream and 115 bp upstream of a given TSS. Each mutated sequence is flanked by primer-binding sites to facilitate cloning. The genetic construct also contains a random barcode, a ribosome-binding site (RBS), a GFP gene, and a terminator labeled with a large 'T'.
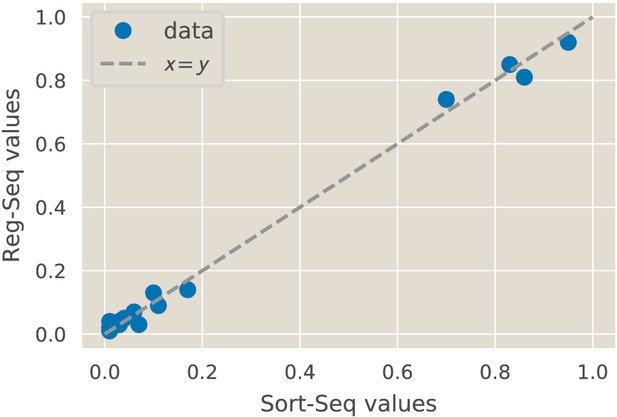
Mock data comparing Sort-Seq and Reg-Seq sequence logo values.
These data have a Pearson correlation coefficient of . This high correlation is also indicated by the data deviating little from the line.
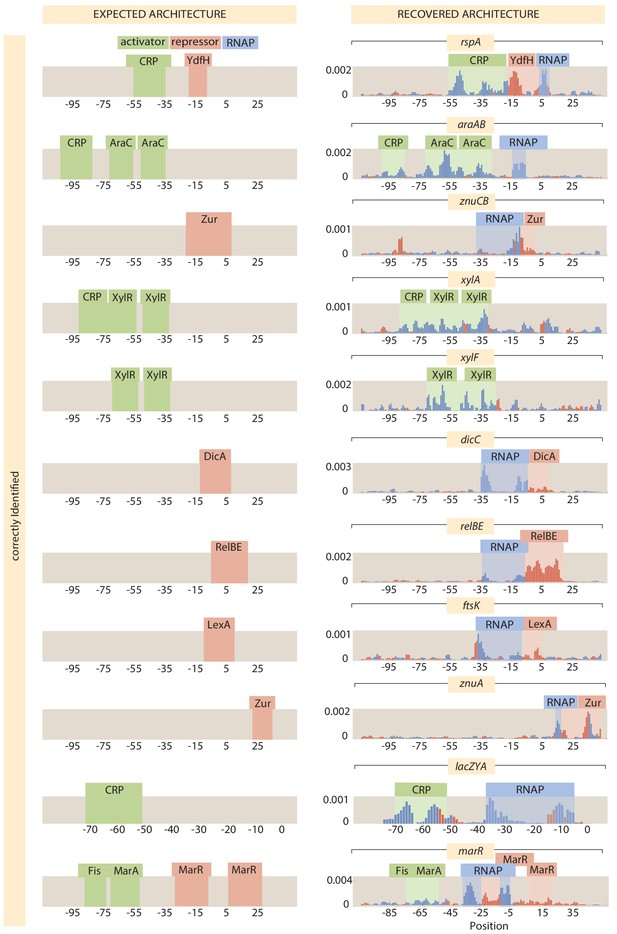
A visual comparison of the literature binding sites (left panel) and the extent of the binding sites discovered by our algorithmic approach (right panel).
RNAP-binding sites are also labeled in the right panel, but RNAP-binding sites are not included in the false positive analysis. Numeric values for the displayed data can be found in Appendix 2—figure 2—source data 1.
-
Appendix 2—figure 2—source data 1
Data for information footprints and identified regions in Appendix 2—figure 2.
- https://cdn.elifesciences.org/articles/55308/elife-55308-app2-fig2-data1-v2.xlsx
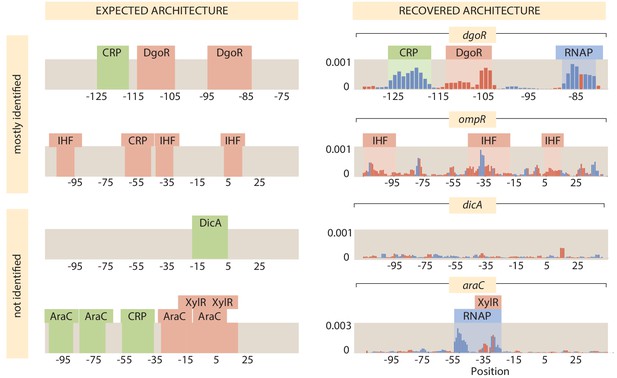
A continuation of the visual comparison of the literature binding sites (left panel) and the binding sites discovered by our algorithmic approach (right panel) begun in Appendix 2—figure 2.
-
Appendix 2—figure 3—source data 1
Data for information footprints and identified regions in Appendix 2—figure 3.
- https://cdn.elifesciences.org/articles/55308/elife-55308-app2-fig3-data1-v2.xlsx
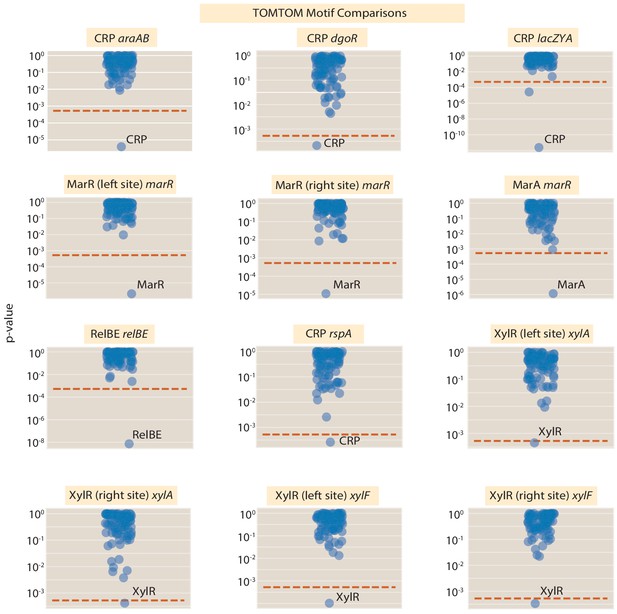
A visual display of the results of the TOMTOM motif comparison between the discovered binding sites and known sequence motifs from RegulonDB and our prior Sort-Seq experiment (Belliveau et al., 2018).
Each dot in a given panel represents a comparison between the target position weight matrix (given in the figure title) and a position weight matrix for a given transcription factor. The p-value is calculated using the null hypothesis, that both motifs are drawn independently from the same underlying probability distribution. The red dotted line is displayed at a p-value of . The line represents a p-value threshold of 0.05 that has been corrected for multiple hypothesis testing using the Bonferroni correction (95 motifs were compared against the target for a p-value threshold of ). Numeric values for the displayed data can be found in Appendix 2—figure 4-source data 1.
-
Appendix 2—figure 4—source data 1
All p-values displayed in Appendix 2—figure 4.
- https://cdn.elifesciences.org/articles/55308/elife-55308-app2-fig4-data1-v2.csv
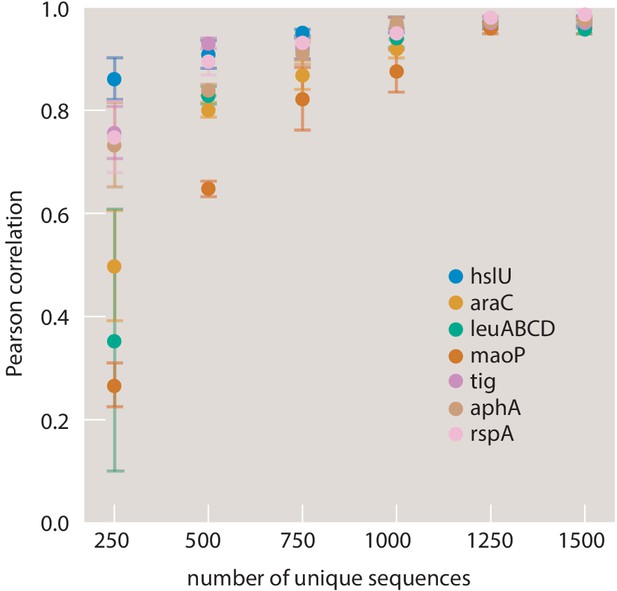
Pearson correlation as a function of the number of unique DNA sequences (as explained in Appendix 2 Section 'Comparison between Reg-Seq by RNA-Seq and 2uorescent sorting').
For seven different genes, we studied how the number of mutated DNA sequences affects the reproducibility of our MCMC inference models. As the number of unique sequences increases, so too does the Pearson correlation value, approaching 1.0. Numeric values for the displayed data can be found in Appendix 3—figure 1—source data 1.
-
Appendix 3—figure 1—source data 1
Pearson correlation values for Appendix 3—figure 1.
- https://cdn.elifesciences.org/articles/55308/elife-55308-app3-fig1-data1-v2.txt
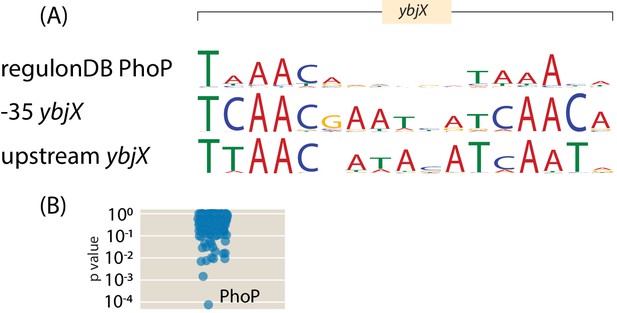
Motif comparison using TOMTOM for the two PhoP-binding sites in the ybjX promoter.
Searching our energy motifs against the RegulonDB database using TOMTOM allowed us to guide our transcription factor knockout experiments. Here, we show the sequence logos of the PhoP transcription factor from RegulonDB (top) and the ones generated from the ybjX promoter energy matrix. E-value = 0.01 using Euclidean distance as a similarity matrix.
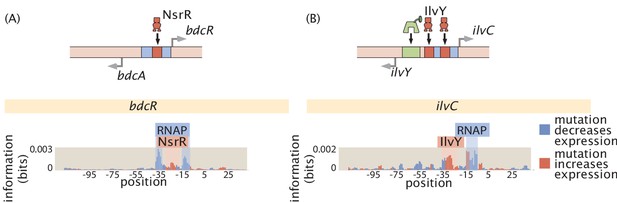
Two cases in which we see transcription-factor-binding sites that we have found to regulate both of the two divergently transcribed genes.
(A) An information footprint and regulatory cartoon for the divergently transcribed bdcA and bdcR promoters. A single NsrR site regulates both promoters. (B) An information footprint and regulatory cartoon for the ilvC and ilvY promoters. Both promoters are repressed by IlvY when grown without acetolactate. Only the IlvY site is labeled on the information footprint.
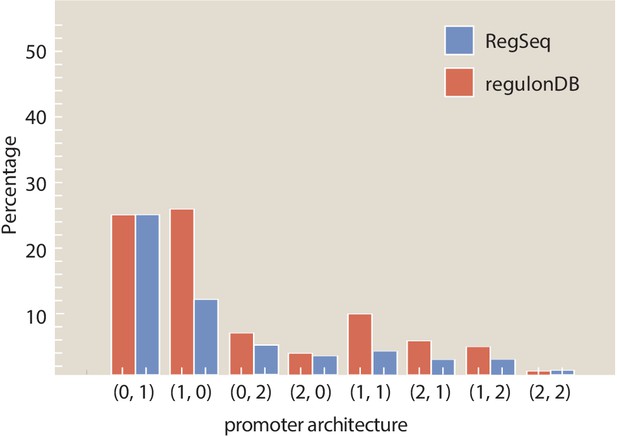
A comparison of the types of architectures found in RegulonDB (Santos-Zavaleta et al., 2019) to the architectures with newly discovered binding sites found in the Reg-Seq study.
For each type of architecture, labeled as (number of activators, number of repressors), the fraction that architecture comprises of the total number of operons is given both for the data found in RegulonDB and from the results of the Reg-Seq experiment. Numeric values for the displayed data can be found in Appendix 4—figure 2—source data 1.
-
Appendix 4—figure 2—source data 1
Source data for the percentage composition of regulatory architectures.
The source data file contains the percentage composition of each regulatory architecture displayed in the Figure. This includes architectures more complicated than those displayed in the histogram.
- https://cdn.elifesciences.org/articles/55308/elife-55308-app4-fig2-data1-v2.csv
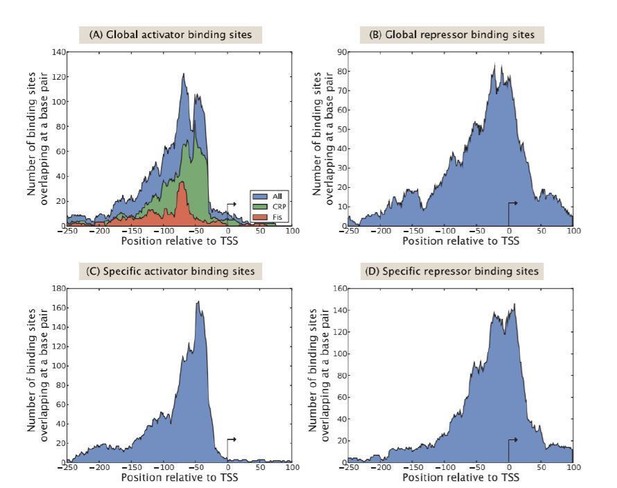
Figure 10 from Rydenfelt et al., 2014b.
Distribution of activating and repressing binding sites bound by global TFs and specific TFs, respectively. The y-axis shows the number of binding sites overlapping each nucleotide position, after aligning all promoters with respect to their transcription start site (TSS) for the different kinds of TFs.
Tables
All promoters examined in this study, categorized according to type of regulatory architecture.
Those promoters which have no recognizable RNAP site are labeled as inactive rather than constitutively expressed (0, 0).
Architecture | Total number of promoters | Number of promoters with at least one newly discovered binding site |
---|---|---|
All Architectures | 113 | 48 |
(0,0) | 34 | 0 |
(0,1) | 26 | 21 |
(1,0) | 11 | 10 |
(1,1) | 4 | 3 |
(0,2) | 4 | 4 |
(2,0) | 3 | 2 |
(1,2) | 4 | 3 |
(2,1) | 2 | 2 |
(2,2) | 1 | 1 |
(3,0) | 3 | 1 |
(0,3) | 2 | 1 |
(0,4) | 1 | 0 |
inactive | 18 | 0 |
All genes investigated in this study categorized according to their regulatory architecture, given as (number of activators, number of repressors).
The regulatory architectures as listed reflect only the binding sites that would be able to be recovered within our 160 bp constructs, but include both newly discovered and previously known binding sites. In those cases where binding sites that appear in RegulonDB or Ecocyc are omitted from this tally, the Section 'Explanation of included binding sites' in Appendix 4 has the reasoning, for each relevant gene, why the binding sites are not shown. The table also lists the number of newly discovered binding sites, previously known binding sites, and number of identified transcription factors. The evidence used for the transcription factor identification is given in the final column. 'Bioinformatic' evidence implies that discovered position weight matrices were compared to known transcription factor position weight matrices. The literature sites column contains only those sites that are both expected to be and are, in actuality, observed in the Reg-Seq data.
Architecture | Promoter | Newly discovered binding sites | Literature binding sites | Identified binding sites | Evidence |
---|---|---|---|---|---|
(0, 0) | acuI | 0 | 0 | 0 | |
aegA | 0 | 0 | 0 | ||
arcB | 0 | 0 | 0 | ||
cra | 0 | 0 | 0 | ||
dnaE | 0 | 0 | 0 | ||
ecnB | 0 | 0 | 0 | ||
fdoH | 0 | 0 | 0 | ||
holC | 0 | 0 | 0 | ||
hslU | 0 | 0 | 0 | ||
htrB | 0 | 0 | 0 | ||
minC | 0 | 0 | 0 | ||
modE | 0 | 0 | 0 | ||
ycgB | 0 | 0 | 0 | ||
mscL | 0 | 0 | 0 | ||
pitA | 0 | 0 | 0 | ||
poxB | 0 | 0 | 0 | ||
rlmA | 0 | 0 | 0 | ||
rumB | 0 | 0 | 0 | ||
sbcB | 0 | 0 | 0 | ||
sdaB | 0 | 0 | 0 | ||
tar | 0 | 0 | 0 | ||
ybdG | 0 | 0 | 0 | ||
ybiP | 0 | 0 | 0 | ||
ybjT | 0 | 0 | 0 | ||
yehT | 0 | 0 | 0 | ||
yfhG | 0 | 0 | 0 | ||
ygdH | 0 | 0 | 0 | ||
ygeR | 0 | 0 | 0 | ||
yggW | 0 | 0 | 0 | ||
ynaI | 0 | 0 | 0 | ||
yqhC | 0 | 0 | 0 | ||
zapB | 0 | 0 | 0 | ||
zupT | 0 | 0 | 0 | ||
amiC | 0 | 0 | 0 | ||
(0, 1) | araC | 0 | 1 | 0 | |
bdcR | 1 | 0 | 1 | Known binding location (NsrR) (Partridge et al., 2009) | |
coaA | 1 | 0 | 0 | ||
dicC | 0 | 1 | 0 | ||
dinJ | 1 | 0 | 0 | ||
ybeZ | 1 | 0 | 0 | ||
idnK | 1 | 0 | 1 | Mass- Spectrometry (YgbI) | |
leuABCD | 1 | 0 | 1 | Mass- Spectrometry (YgbI) | |
mscM | 1 | 0 | 0 | ||
yedK | 1 | 0 | 1 | Mass- Spectrometry (TreR) | |
rapA | 1 | 0 | 1 | Growth condition Knockout (GlpR), Bioinformatic (GlpR) | |
sdiA | 1 | 0 | 0 | ||
tff-rpsB-tsf | 1 | 0 | 1 | Growth condition Knockout (GlpR), Bioinformatic (GlpR), Knockout (GlpR) | |
thiM | 1 | 0 | 0 | ||
tig | 1 | 0 | 1 | Growth condition Knockout (GlpR), Bioinformatic (GlpR), Knockout (GlpR) | |
ybiO | 1 | 0 | 0 | ||
ydjA | 1 | 0 | 0 | ||
yedJ | 1 | 0 | 0 | ||
phnA | 1 | 0 | 1 | Mass- Spectrometry (YciT) | |
mutM | 1 | 0 | 0 | ||
rhlE | 1 | 0 | 1 | Growth condition Knockout (GlpR), Bioinformatic (GlpR), Mass- Spectrometry (GlpR) | |
uvrD | 1 | 0 | 1 | Bioinformatic (LexA) | |
dusC | 1 | 0 | 0 | ||
ftsK | 0 | 1 | 0 | ||
znuA | 0 | 1 | 0 | ||
znuCB | 0 | 1 | 0 | ||
(1, 0) | waaA-coaD | 1 | 0 | 0 | |
rcsF | 1 | 0 | 0 | ||
groSL | 1 | 0 | 0 | ||
mscS | 1 | 0 | 0 | ||
thrLABC | 1 | 0 | 0 | ||
yeiQ | 1 | 0 | 1 | Growth condition Knockout (FNR), Bioinformatic (FNR) | |
ycbZ | 1 | 0 | 0 | ||
ygjP | 1 | 0 | 0 | ||
lac | 0 | 1 | 0 | Bioinformatic (CRP) | |
yehS | 1 | 0 | 0 | ||
yehU | 1 | 0 | 1 | Growth condition Knockout (FNR), Bioinformatic (FNR) | |
(0, 2) | pcm | 2 | 0 | 0 | |
yecE | 2 | 0 | 1 | Mass- Spectrometry (HU) | |
yjjJ | 2 | 0 | 1 | Growth condition Knockout (MarA), Bioinformatic (MarA) | |
dcm | 2 | 0 | 1 | Mass- Spectrometry (HNS) | |
(1, 1) | arcA | 2 | 0 | 2 | Growth condition Knockout (FNR), Bioinformatic (FNR), Mass- Spectrometry (FNR, CpxR) |
dgoR | 0 | 2 | 0 | Bioinformatic (CRP) Bioinformatic (DgoR) | |
ykgE | 2 | 0 | 2 | Growth condition Knockout (FNR), Bioinformatic (FNR), Mass- Spectrometry(YieP) Knockout (YieP) | |
ymgG | 2 | 0 | 0 | ||
(2, 0) | asnA | 2 | 0 | 0 | |
fdhE | 2 | 0 | 2 | Growth condition Knockout (FNR, ArcA), Bioinformatic (FNR, ArcA), Knockout (ArcA) | |
xylF | 0 | 2 | 0 | ||
(1, 2) | marR | 0 | 3 | 0 | Mass- Spectrometry (MarR) |
aphA | 3 | 0 | 2 | Growth condition Knockout (FNR), Bioinformatic (FNR), Mass- Spectrometry (DeoR) | |
iap | 3 | 0 | 0 | ||
ilvC | 3 | 0 | 1 | Mass- Spectrometry (IlvY) (Rhee et al., 1998) | |
(2, 1) | maoP | 3 | 0 | 3 | Growth condition Knockout (GlpR), Bioinformatic (GlpR), Knockout (PhoP, HdfR, GlpR) |
rspA | 1 | 2 | 1 | Mass- Spectrometry (DeoR) | |
(2, 2) | ybjX | 4 | 0 | 4 | Bioinformatic (2 PhoP sites), Mass- Spectrometry (HNS, StpA) |
(3, 0) | araAB | 0 | 3 | 0 | |
xylA | 0 | 3 | 0 | ||
yicI | 3 | 0 | 0 | ||
(0, 3) | ompR | 0 | 3 | 0 | |
ybjL | 3 | 0 | 0 | ||
(0, 4) | relBE | 0 | 4 | 0 | Mass- Spectrometry (RelBE) |
All growth conditions used in the Reg-Seq study.
Heat shocked cells were exposed to 42°C for 5 min upon reaching OD 0.3 as this is known to induce transcription by (Arsène et al., 2000). Low oxygen growth cells were grown in a flask sealed with parafilm with minimal oxygen, although some was present as no anaerobic chamber was used. This level of oxygen stress was still sufficient to activate FNR binding, thus activating anaerobic metabolism. For cells grown with iron, upon reaching OD of 0.3 iron was added and cells were incubated for 10 min before harvesting RNA. Growth without cAMP was accomplished by the use of the JK10 strain (Kinney et al., 2010) which does not maintain its cAMP levels.
Growth conditions |
---|
M9 with glucose (0.5%) |
M9 with acetate (0.5%) |
M9 with arabinose (0.5%) |
M9 with xylose (0.5%) and arabinose (0.5%) |
M9 with succinate (0.5%) |
M9 with trehalose (0.5%) |
M9 with glucose (0.5%) and 5 mM sodium salycilate |
LB |
heat shock in M9 with glucose (0.5%) |
LB in low oxygen |
zinc, 5 mM ZnCl in M9 with glucose (0.5%) |
iron, 5 mM FeCL in M9 with glucose (0.5%) |
no cAMP in M9 with glucose (0.5%) |
A suite of experimentally validated and high-evidence binding sites used to test our automated binding site finding algorithm.
Specifically, this list of genes was used to test the false negative rate of our Reg-Seq method by examining what fraction of high-evidence sites were also identified with Reg-Seq.
Gene | Transcription factor | Transcription factor type |
---|---|---|
rspA | CRP | activator |
rspA | YdfH | repressor |
araAB | AraC (two sites) | activator |
znuCB | Zur | repressor |
xylA | CRP | activator |
xylA | XylR (two sites) | activator |
xylF | XylR (two sites) | activator |
dicC | DicA | repressor |
relBE | RelBE | repressor |
ftsK | LexA | repressor |
znuA | Zur | repressor |
lac | CRP | activator |
marR | Fis | activator |
marR | MarA | activator |
marR | MarR (two sites) | repressor |
dgoR | CRP | activator |
dgoR | DgoR (right site) | repressor |
ompR | IHF (three sites) | repressor |
ompR | CRP | repressor |
dicA | DicA | repressor |
araC | AraC (two sites) | repressor |
araC | AraC (two sites) | activator |
araC | CRP | activator |
araC | XylR (two sites) | repressor |
The results of the comparison between experimentally verified, high-evidence binding sites and Reg-Seq-binding sites.
A visual illustration of the comparison can be found in Appendix 2—figures 2 and 3.
Gene | Transcription factor | Was the region classified correctly? |
---|---|---|
rspA | CRP | Yes |
rspA | YdfH | Yes |
araAB | AraC (two sites) | Yes |
znuCB | Zur | Yes |
xylA | CRP | Yes |
xylA | XylR (two sites) | Yes |
xylF | XylR (two sites) | Yes |
dicC | DicA | Yes |
relBE | RelBE | Yes |
ftsK | LexA | Yes |
znuA | Zur | Yes |
lac | CRP | Yes |
marR | Fis | No |
marR | MarA | Yes |
marR | MarR (two sites) | Yes |
dgoR | CRP | Yes |
dgoR | DgoR (right site) | No |
ompR | IHF (three sites) | Yes |
ompR | CRP | No |
dicA | DicA | No |
araC | AraC (four sites) | one site identified |
araC | CRP | No |
araC | XylR (two sites) | No |
Example dataset of four nucleotide sequences, and the corresponding counts from the plasmid library and mRNAs.
Sequence | Library sequencing counts | mRNA counts |
---|---|---|
ACTA | 5 | 23 |
ATTA | 5 | 3 |
CCTG | 11 | 11 |
TAGA | 12 | 3 |
GTGC | 2 | 0 |
CACA | 8 | 7 |
AGGC | 7 | 3 |
Global, absolute quantification for most transcription factors identified in this study, as determined for E. coli K12 grown in both glucose (5 g/L concentration in M9 minimal media) and LB medias.
The values in this table are reprinted from Schmidt et al., 2016 Supplemental Table S6.
Transcription factor name | Glucose | LB |
---|---|---|
FNR | 609 | 1101 |
YieP | 158 | 261 |
YciT | 82 | 104 |
NsrR | 872 | 136 |
LexA | 560 | 1027 |
DeoR | 26 | 34 |
CRP | 2048 | 3450 |
YdfH | 96 | 154 |
ArcA | 3367 | 5464 |
Zur | 70 | 130 |
GlpR | 75 | 145 |
PhoP | 2967 | 3132 |
HNS | 22541 | 47133 |
StpA | 6863 | 5241 |
DicA | 20 | 25 |
YgbI | 2 | 6 |
XylR | 1 | 8 |
Example energy matrix.
This matrix is in arbitrary units, and the process to obtain absolute units (in ) is described in Appendix 3 Section 'Inference of scaling factors for energy matrices'.
Pos | A | C | G | T |
---|---|---|---|---|
0 | −0.01 | −0.01 | −0.01 | 0.03 |
1 | 0.002 | 0.05 | −0.06 | 0.008 |
2 | −0.0002 | −0.04 | 0.008 | 0.03 |
3 | −0.02 | 0.02 | −0.01 | 0.01 |
Example dataset with energy predictions.
Energy predictions are made by applying the example energy matrix in Appendix 3—table 3 to the example dataset in Appendix 3—table 1 according to Equation (26).
Energy ( | ||
---|---|---|
5 | 23 | 0.05 |
5 | 3 | 0.008 |
11 | 11 | 0.09 |
12 | 3 | −0.03 |
2 | 0 | 0.03 |
8 | 7 | −0.07 |
7 | 3 | −0.04 |
A table showing scaling factors to convert arbitrary units to absolute units in .
Growth conditions indicate the energy matrix and dataset used in the fit. In some growth condition additional regulatory features will be present, meaning specify condition is important.
Gene | Growth | Scaling factor A |
---|---|---|
tff-rpsB-tsf | Heat shock | |
tig | Heat shock | |
yjjJ | Heat shock | |
bdcR | Heat shock | |
fdhE | Anaerobic growth | |
ykgE | Arabinose | |
dicC | Arabinose | |
rspA | Arabinose |
Reagent type (species) or resource | Designation | Source or reference | Identifiers | Additional information |
---|---|---|---|---|
Cell line (Escherichia coli) | E. coli K12 | E. coli Stock Center | ||
Cell line (Escherichia coli) | E. coli ΔYieP | E. coli Stock Center | ||
Cell line (Escherichia coli) | E. coli ΔGlpR | E. coli Stock Center | ||
Cell line (Escherichia coli) | E. coli ΔArcA | E. coli Stock Center | ||
Cell line (Escherichia coli) | E. coli ΔLrhA | E. coli Stock Center | ||
Cell line (Escherichia coli) | E. coli ΔPhoP | E. coli Stock Center | ||
Cell line (Escherichia coli) | E. coli ΔHdfR | E. coli Stock Center | ||
Strain, strain background (Escherichia coli) | E. coli ΔGlpR in K12 strain | This paper | Knockout transferred to E. coli K12 | |
Strain, strain background (Escherichia coli) | E. coli ΔArcA in K12 strain | This paper | Knockout transferred to E. coli K12 | |
Strain, strain background (Escherichia coli) | E. coli ΔLrhA in K12 strain | This paper | Knockout transferred to E. coli K12 | |
Strain, strain background (Escherichia coli) | E. coli ΔPhoP in K12 strain | This paper | Knockout transferred to E. coli K12 | |
Strain, strain background (Escherichia coli) | E. coli ΔHdfR in K12 strain | This paper | Knockout transferred to E. coli K12 | |
Chemical compound, drug | Q5 Polymerase | Qiagen | Cat. : M0491L | |
Chemical compound, drug | qPCR master mix | QuantaBio | Cat. : 101414–166 | |
Chemical compound, drug | Lysyl Endopeptidase | Wako Chemicals | Cat. : 125–05061 | |
Commercial assay or kit | RNEasy Mini kit | Qiagen | Cat. : 74104 | |
Chemical compound, drug | RNAprotect bacteria reagent | Qiagen | Cat. : 76506 | |
Software, algorithm | mpathic | Kinney Lab Ireland and Kinney, 2016 | ||
Software, algorithm | FastX | Hannon Lab | RRID:SCR_005534 | |
Software, algorithm | FLASH | CBCB | RRID:SCR_005531 | |
Other | Oligo Pool | Twist Bioscience | ||
Sequence-based reagent | fwd oligo 101 | IDT | TTCGTCTTCACCT CGAGCACGCTTATT CGTGCCGTGTTAT | |
Sequence-based reagent | fwd oligo 102 | IDT | TTCGTCTTCACCTC GAGCACTTTGCTT CAGTCAGATTCGC | |
Sequence-based reagent | fwd oligo 103 | IDT | TTCGTCTTCACCT CGAGCACGTCGAGT CCTATGTAACCGT | |
Sequence-based reagent | fwd oligo 104 | IDT | TTCGTCTTCACCT CGAGCACGTAAGAT GGAAGCCGGGATA | |
Sequence-based reagent | fwd oligo 105 | IDT | TTCGTCTTCACCT CGAGCACGGTGTCGC AACATGATCTAC | |
Sequence-based reagent | fwd oligo 106 | IDT | TTCGTCTTCACCT CGAGCACGTGCTAAG TCACACTGTTGG | |
Sequence-based reagent | fwd oligo 107 | IDT | TTCGTCTTCACCT CGAGCACTCTAAACA GTTAGGCCCAGG | |
Sequence-based reagent | fwd oligo 108 | IDT | TTCGTCTTCACCT CGAGCACGTCTTTAT ACTTGCCTGCCG | |
Sequence-based reagent | fwd oligo 109 | IDT | TTCGTCTTCACCT CGAGCACCACCGCGA TCAATACAACTT | |
Sequence-based reagent | fwd oligo 110 | IDT | TTCGTCTTCACCT CGAGCACTTCGGATA GACTCAGGAAGC | |
Sequence-based reagent | fwd oligo 111 | IDT | TTCGTCTTCACCT CGAGCACCCATTGAT AGATTCGCTCGC | |
Sequence-based reagent | fwd oligo 112 | IDT | TTCGTCTTCACCT CGAGCACTTTTCTAC TTTCCGGCTTGC | |
Sequence-based reagent | fwd oligo 113 | IDT | TTCGTCTTCACCT CGAGCACATGACTAT TGGGGTCGTACC | |
Sequence-based reagent | fwd oligo 114 | IDT | TTCGTCTTCACCT CGAGCACTCGACAAT AGTTGAGCCCTT | |
Sequence-based reagent | fwd oligo 115 | IDT | TTCGTCTTCACCT CGAGCACGAGCCATG TGAAATGTGTGT | |
Sequence-based reagent | fwd oligo 116 | IDT | TTCGTCTTCACCT CGAGCACCGTATACG TAAGGGTTCCGA | |
Sequence-based reagent | fwd oligo 117 | IDT | TTCGTCTTCACCT CGAGCACTTATGATG TCCGGATACCCG | |
Sequence-based reagent | fwd oligo 118 | IDT | TTCGTCTTCACCT CGAGCACTCTTAGAA ATCCACGGGTCC | |
Sequence-based reagent | rev oligo 101 | IDT | TGTAAAACGACGG CCAGTGACTAGCGC TGAGGAGAAGCCT AATAGGGCACAGC AATCAAAAGTA | |
Sequence-based reagent | rev oligo 102 | IDT | TGTAAAACGACG GCCAGTGAGGAGCGC TGAGGAGAAGCC TAATACCGGGATT CAGTGATTGAAC | |
Sequence-based reagent | rev oligo 103 | IDT | TGTAAAACGACG GCCAGTGAGTCCC GCTGAGGAGAAG CCTAATATGAAGAT ATGACGACCCCTG | |
Sequence-based reagent | rev oligo 104 | IDT | TGTAAAACGACGG CCAGTGACCGACGCT GAGGAGAAGCCTAA TATTCCACAGCTC TATGAGGTG | |
Sequence-based reagent | rev oligo 105 | IDT | TGTAAAACGACGG CCAGTGATTGGCGCT GAGGAGAAGCCTA ATAGCAAACATGA CTAGGAACCG | |
Sequence-based reagent | rev oligo 106 | IDT | TGTAAAACGACGG CCAGTGAGATACGC TGAGGAGAAGCC TAATACCGGGACG AGATTAGTACAA | |
Sequence-based reagent | rev oligo 107 | IDT | TGTAAAACGACGGC CAGTGAACTCCGCT GAGGAGAAGCCTA ATACACGCCAGTT GTGAACATAA | |
Sequence-based reagent | rev oligo 108 | IDT | TGTAAAACGACG GCCAGTGATACTCGC TGAGGAGAAGC CTAATACAAAGGC CAAATCAGTTCCA | |
Sequence-based reagent | rev oligo 109 | IDT | TGTAAAACGACGGC CAGTGACCAACGCT GAGGAGAAGCCT AATAGGTGCATGGG AGGAACTATA | |
Sequence-based reagent | rev oligo 110 | IDT | TGTAAAACGACG GCCAGTGAAGGCCGC TGAGGAGAAGCCT AATATGCATGGGT CTGTCTATTGT | |
Sequence-based reagent | rev oligo 111 | IDT | TGTAAAACGACGGC CAGTGAAATTCGC TGAGGAGAAGCCT AATACTCCTATGCT AGCTCGACTC | |
Sequence-based reagent | rev oligo 112 | IDT | TGTAAAACGACG GCCAGTGATTGT CGCTGAGGAGAAG CCTAATAATGGTA AGAAGCTCCCACAA | |
Sequence-based reagent | rev oligo 113 | IDT | TGTAAAACGACGGC CAGTGATTTACGCT GAGGAGAAGCCTA ATACTATGGTCA TTCCCGTACGA | |
Sequence-based reagent | rev oligo 114 | IDT | TGTAAAACGACGGC CAGTGAACCGCGCT GAGGAGAAGCCTA ATATAATCGGCT ACGTTGTGTCT | |
Sequence-based reagent | rev oligo 115 | IDT | TGTAAAACGACGGC CAGTGATGGCCGC TGAGGAGAAGC CTAATATGACTCGA TCCTTTAGTCCG | |
Sequence-based reagent | rev oligo 116 | IDT | TGTAAAACGACGG CCAGTGAGGCCCGC TGAGGAGAAGC CTAATAACGCTTT GTGTTATCCGATG | |
Sequence-based reagent | rev oligo 117 | IDT | TGTAAAACGACGG CCAGTGAGGTGCG CTGAGGAGAAG CCTAATAACCACG GTGGAGTATACATC | |
Sequence-based reagent | rev oligo 118 | IDT | TGTAAAACGACG GCCAGTGACAATCG CTGAGGAGAAGC CTAATAGGCACCA GGTACATATCTCA | |
Sequence-based reagent | mRNA rev | IDT | GCAGGGGATAA TATTGCCCA | |
Sequence-based reagent | fwd sequencing 94 | IDT | AATGATACGGCGACCAC CGAGATCT ACACTCTT TCCCTACACGACGC TCTTCCGATCTGACC TATTAGGCTT CTCCTCAGCG | |
Sequence-based reagent | fwd sequencing 95 | IDT | AATGATACGGCGACCAC CGAGATCT ACACTCTT TCCCTACACGACGC TCTTCCGATCTCAGT TATTAGGCTT CTCCTCAGCG | |
Sequence-based reagent | fwd sequencing 96 | IDT | AATGATACGGCGACCAC CGAGATCT ACACTCTT TCCCTACACGACGC TCTTCCGATCTTCTA TATTAGGCTT CTCCTCAGCG | |
Sequence-based reagent | fwd sequencing 97 | IDT | AATGATACGGCGACCAC CGAGATCT ACACTCTT TCCCTACACGACGC TCTTCCGATCTAGAG TATTAGGCTT CTCCTCAGCG | |
Sequence-based reagent | fwd sequencing 98 | IDT | AATGATACGGCGACCAC CGAGATCT ACACTCTT TCCCTACACGACGC TCTTCCGATCTGCAT TATTAGGCTT CTCCTCAGCG | |
Sequence-based reagent | fwd sequencing 99 | IDT | AATGATACGGCGACCAC CGAGATCT ACACTCTT TCCCTACACGACGC TCTTCCGATCTCTTA TATTAGGCTT CTCCTCAGCG | |
Sequence-based reagent | fwd sequencing 100 | IDT | AATGATACGGCGACCAC CGAGATCT ACACTCTT TCCCTACACGACGC TCTTCCGATCTTAGC TATTAGGCTT CTCCTCAGCG | |
Sequence-based reagent | fwd sequencing 101 | IDT | AATGATACGGCGACCAC CGAGATCT ACACTCTT TCCCTACACGACGC TCTTCCGATCTCAAG TATTAGGCTT CTCCTCAGCG | |
Sequence-based reagent | fwd sequencing 102 | IDT | AATGATACGGCGACCAC CGAGATCT ACACTCTT TCCCTACACGACGC TCTTCCGATCTGTAC TATTAGGCTT CTCCTCAGCG | |
Sequence-based reagent | fwd sequencing 103 | IDT | AATGATACGGCGACCAC CGAGATCT ACACTCTT TCCCTACACGACGC TCTTCCGATCTTGAA TATTAGGCTT CTCCTCAGCG | |
Sequence-based reagent | fwd sequencing 104 | IDT | AATGATACGGCGACCAC CGAGATCT ACACTCTT TCCCTACACGACGC TCTTCCGATCTTCGT TATTAGGCTT CTCCTCAGCG | |
Sequence-based reagent | fwd sequencing 105 | IDT | AATGATACGGCGACCAC CGAGATCT ACACTCTT TCCCTACACGACGC TCTTCCGATCTATGC TATTAGGCTT CTCCTCAGCG | |
Sequence-based reagent | fwd sequencing 106 | IDT | AATGATACGGCGACCAC CGAGATCT ACACTCTT TCCCTACACGACGC TCTTCCGATCTGTCA TATTAGGCTT CTCCTCAGCG | |
Sequence-based reagent | fwd sequencing 107 | IDT | AATGATACGGCGACCAC CGAGATCT ACACTCTT TCCCTACACGACGC TCTTCCGATCTCTCA TATTAGGCTT CTCCTCAGCG | |
Sequence-based reagent | fwd sequencing 108 | IDT | AATGATACGGCGACCAC CGAGATCT ACACTCTT TCCCTACACGACGC TCTTCCGATCTAGTA TATTAGGCTT CTCCTCAGCG | |
Sequence-based reagent | rev sequencing | IDT | AAGCAGAAGACGGCAT ACGAGATCGGT CTCG GCATTCCTGCTGAACC GCTCTTCCGATCTCAAA GCAGGGGATAA TATTGCCCA | |
Other | Streptavin coated dynabeads | Thermo Fisher | Cat. : 65601 | |
Database | RegulonDB | RRID:SCR_003499 | ||
Database | EcoCyc | RRID:SCR_002433 |
pos | A | C | G | T |
---|---|---|---|---|
0 | -0.005387 | -0.011758 | -0.010176 | 0.027322 |
1 | 0.002338 | 0.049826 | -0.058030 | 0.005866 |
2 | -0.000259 | -0.037224 | 0.008021 | 0.029461 |
3 | -0.017494 | 0.015760 | -0.012184 | 0.013918 |
… | … | … | … | … |
Additional files
-
Supplementary file 1
This file contains the presumed location of the TSS for each promoter region in Reg-Seq.
It additionally contains the logic behind the choice of TSS when there are multiple options.
- https://cdn.elifesciences.org/articles/55308/elife-55308-supp1-v2.xlsx
-
Supplementary file 2
This file contains all primers used in the Reg-Seq experiment.
Additionally, it contains the flanking sequences of the mutated inserts and the barcodes used to label the growth conditions in the Reg-Seq experiment.
- https://cdn.elifesciences.org/articles/55308/elife-55308-supp2-v2.xlsx
-
Supplementary file 3
This file contains all transcription-factor-binding sites identified either through the automated binding site algorithm or which were identified manually and have additional evidence for binding.
The starting and ending base pairs for each binding site, and whether the transcription factor acts as an activator or repressor are listed.
- https://cdn.elifesciences.org/articles/55308/elife-55308-supp3-v2.xlsx
-
Source code 1
This file contains custom python scripts used in the processing and analysis of sequencing data.
- https://cdn.elifesciences.org/articles/55308/elife-55308-code1-v2.tar.gz
-
Transparent reporting form
- https://cdn.elifesciences.org/articles/55308/elife-55308-transrepform-v2.docx
-
Appendix 2—figure 2—source data 1
Data for information footprints and identified regions in Appendix 2—figure 2.
- https://cdn.elifesciences.org/articles/55308/elife-55308-app2-fig2-data1-v2.xlsx
-
Appendix 2—figure 3—source data 1
Data for information footprints and identified regions in Appendix 2—figure 3.
- https://cdn.elifesciences.org/articles/55308/elife-55308-app2-fig3-data1-v2.xlsx
-
Appendix 2—figure 4—source data 1
All p-values displayed in Appendix 2—figure 4.
- https://cdn.elifesciences.org/articles/55308/elife-55308-app2-fig4-data1-v2.csv
-
Appendix 3—figure 1—source data 1
Pearson correlation values for Appendix 3—figure 1.
- https://cdn.elifesciences.org/articles/55308/elife-55308-app3-fig1-data1-v2.txt
-
Appendix 4—figure 2—source data 1
Source data for the percentage composition of regulatory architectures.
The source data file contains the percentage composition of each regulatory architecture displayed in the Figure. This includes architectures more complicated than those displayed in the histogram.
- https://cdn.elifesciences.org/articles/55308/elife-55308-app4-fig2-data1-v2.csv