Computational Neuroscience: Finding patterns in cortical responses
Any system, including biological systems, can be said to perform a computation when it transforms input information to generate an output. It is thought that many brain computations are performed by neurons (or groups of neurons) receiving input signals that they process to produce output activity, which then becomes input for other neurons. Many computations that brains can perform could, in principle, be carried out through feedforward processes (Yamins et al., 2014). In simple terms, feedforward means that the signals always travel in one direction – forward to the next neuron or network of neurons – and they never travel backwards or sideways to other neurons within a neuron group. In the cortex, however, networks of neurons have substantial 'recurrent' connectivity. Most cortical neurons are connected to other nearby cortical neurons, and therefore, signals can travel sideways due to these recurrent, local connections.
One property of networks with recurrent connectivity is that they can amplify certain inputs to produce larger outputs, while suppressing other inputs or amplifying them by a smaller factor. However, it has been challenging to understand how this can happen without the system displaying unstable or runaway activity, which is undesirable in the brain because it can lead to epileptic seizures. One plausible mechanism for recurrent amplification is known as 'balanced amplification' (Murphy and Miller, 2009). In mathematical network models that support balanced amplification, recurrent connectivity allows certain inputs to produce large outputs, yet the networks still exhibit other properties that are consistent with experimental data (such as fast responses to inputs). Recurrent connections can also influence the timing of neurons’ responses, allowing shorter inputs to create long-lasting, or time-varying outputs (Hennequin et al., 2014).
Neurons can be excitatory or inhibitory: when an excitatory neuron fires, the neuron receiving that input becomes more likely to fire as well, and when an inhibitory neuron fires, the opposite occurs, and the recipient neuron is suppressed. A network of excitatory and inhibitory cells must possess strong recurrent connectivity to support many recurrent computations, including balanced amplification. Here 'strong' means that recurrent connections are sufficiently dense to allow excitatory neurons to amplify other excitatory neurons’ activity, and in this situation, strong inputs from inhibitory neurons are required to stop the network from becoming unstable. More precisely, inhibitory-stabilized network models are those where, if the activity of inhibitory neurons could be locked to a fixed level, the excitatory neurons in the network would then become unstable (Tsodyks et al., 1997). Inhibitory-stabilized networks have been found in several cortical areas, and are seen across a range of levels of network activity – both when sensory stimulation is present, and when it is absent (Ozeki et al., 2009; Li et al., 2019; Sanzeni et al., 2019, but see Mahrach et al., 2020).
The simplest form of strong connectivity amongst excitatory neurons in a network is where the whole excitatory network is unstable. This is the standard inhibitory-stabilized network. But complex neural networks can have multiple unstable excitatory modes, where subgroups of excitatory neurons are unstable and would display runaway behavior if they were not stabilized by inhibition. Networks in which inhibition stabilizes multiple excitatory modes or subgroups are said to be in detailed balance (Vogels and Abbott, 2009; Hennequin et al., 2014; Litwin-Kumar and Doiron, 2014), while those in which inhibition stabilizes a single group of excitatory cells, typically the group of all excitatory cells, are in global balance. As a general rule, networks in detailed balance are also in global balance.
Now, in eLife, Sadra Sadeh and Claudia Clopath from Imperial College London report the result of simulations that show that networks in detailed balance have properties that extend the basic inhibitory-stabilized network (Sadeh and Clopath, 2020). In globally-balanced networks, when inhibitory neurons are stimulated uniformly (all of the neurons across the network receive an input of the same strength) a distinctive ‘paradoxical’ response, where adding input reduces activity, can be observed (Figure 1C). These paradoxical responses can be used as a signature to determine whether the network is an inhibitory-stabilized network (Tsodyks et al., 1997). Sadeh and Clopath extend this idea to detailed-balance networks with multiple unstable excitatory modes. They show that if the inhibitory neurons in these networks receive more complex, patterned stimulation (that is, certain neurons receive a stronger input than others) a predictable paradoxical signature can be observed (Figure 1D). Sadeh and Clopath call networks in which this happens ‘specific inhibitory-stabilized networks’. The connectivity patterns between neurons in their models are consistent with anatomical evidence of structured network connectivity in the cortex (Ko et al., 2013; Znamenskiy et al., 2018). Further, the existence of multiple excitatory submodes in the cortex is suggested by recent experiments that have found preferential amplification of specific patterns of input (Marshel et al., 2019; Peron et al., 2020).
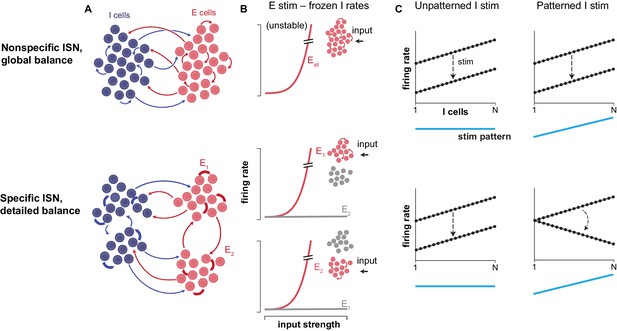
Inhibitory structure revealed by patterned stimulation.
(A) Two possible network structures create two types of inhibitory-stabilized networks (ISNs). In a non-specific network (top), any excitatory (or inhibitory) neuron (E cell or I cell) has the same probability of connecting with other excitatory (inhibitory) neurons. In a specific network, subgroups of neurons connect preferentially to other neurons within the subgroup. (B) The two types of network require two different kinds of inhibitory balance. These types of balance are illustrated here conceptually, via a thought experiment where one imagines inhibitory neurons’ (I cells’) activity is frozen at a fixed level. For non-specific networks (top), if inhibitory neurons’ activity could be frozen, a single group of excitatory cells would respond to input (x-axis) by entering runaway behavior together. Thus, during normal network operation, feedback from inhibitory neurons is required to stabilize this single excitatory mode. The network is then said to be in global balance. For specific networks (bottom), multiple excitatory modes (subgroups of E neurons) are unstable when inhibition is frozen. During normal network operation, the inhibitory network must be connected in such a way as to stabilize these multiple excitatory modes, and these networks are said to be in detailed balance. (C) Sadeh and Clopath examine how firing rates (y-axis) of different inhibitory cells (x-axis) change when stimulated, depending on whether the stimulation pattern (blue line) was uniform (left panels) or patterned (right panels) in non-specific (top) or specific (bottom) networks (note that the specific networks that Sadeh and Clopath simulated have even more than two excitatory modes; see their work for details). The dotted lines show inhibitory firing rates, before (upper line) and after (lower line) stimulation, with the change in firing rates induced by stimulation indicated by the arrow. In both specific and non-specific networks, stimulation that excites the inhibitory neurons uniformly (left) paradoxically leads to a decrease in their firing rates. Patterned stimulation of inhibitory cells (right) in non-specific networks (top) leads to a similar response as with uniform inputs. However, in specific networks (bottom), patterned stimulation adds another effect: the inhibitory neurons that receive the strongest stimulation decrease their activity the most.
Sadeh and Clopath thus make a concrete prediction: that this “specific paradoxical effect” will be seen in networks where the connectivity between neurons is strong and structured. This prediction can now be tested using a technique called two-photon optogenetics that allows patterned input to be provided to neural networks in vivo with single-cell resolution, both for excitatory and inhibitory neurons (for example, Marshel et al., 2019; Forli et al., 2018).
The article by Sadeh and Clopath also takes a conceptual step forward by considering the information that can be gained about network structure and function by providing each neuron with an input of different strength. This conceptual framework is timely, as two-photon stimulation has this ability to vary the strength of the input to selected neurons. Specifically, Sadeh and Clopath predict that a pattern of input across inhibitory neurons will generate a response that is similar to the input pattern but with opposite sign. These predictions should shape future experiments, yielding new information about a key element of cortical function: how the recurrent connectivity in cortical networks is used for computation.
References
-
Formation and maintenance of neuronal assemblies through synaptic plasticityNature Communications 5:5319.https://doi.org/10.1038/ncomms6319
-
Paradoxical effects of external modulation of inhibitory interneuronsThe Journal of Neuroscience 17:4382–4388.https://doi.org/10.1523/JNEUROSCI.17-11-04382.1997
Article and author information
Author details
Publication history
Copyright
© 2020, Sanzeni and Histed
This article is distributed under the terms of the Creative Commons Attribution License, which permits unrestricted use and redistribution provided that the original author and source are credited.
Metrics
-
- 1,657
- views
-
- 188
- downloads
-
- 3
- citations
Views, downloads and citations are aggregated across all versions of this paper published by eLife.
Download links
Downloads (link to download the article as PDF)
Open citations (links to open the citations from this article in various online reference manager services)
Cite this article (links to download the citations from this article in formats compatible with various reference manager tools)
Further reading
-
- Neuroscience
Life histories of oviparous species dictate high metabolic investment in the process of gonadal development leading to ovulation. In vertebrates, these two distinct processes are controlled by the gonadotropins follicle-stimulating hormone (FSH) and luteinizing hormone (LH), respectively. While it was suggested that a common secretagogue, gonadotropin-releasing hormone (GnRH), oversees both functions, the generation of loss-of-function fish challenged this view. Here, we reveal that the satiety hormone cholecystokinin (CCK) is the primary regulator of this axis in zebrafish. We found that FSH cells express a CCK receptor, and our findings demonstrate that mutating this receptor results in a severe hindrance to ovarian development. Additionally, it causes a complete shutdown of both gonadotropins secretion. Using in-vivo and ex-vivo calcium imaging of gonadotrophs, we show that GnRH predominantly activates LH cells, whereas FSH cells respond to CCK stimulation, designating CCK as the bona fide FSH secretagogue. These findings indicate that the control of gametogenesis in fish was placed under different neural circuits, that are gated by CCK.
-
- Neuroscience
Chronic back pain (CBP) is a global health concern with significant societal and economic burden. While various predictors of back pain chronicity have been proposed, including demographic and psychosocial factors, neuroimaging studies have pointed to brain characteristics as predictors of CBP. However, large-scale, multisite validation of these predictors is currently lacking. In two independent longitudinal studies, we examined white matter diffusion imaging data and pain characteristics in patients with subacute back pain (SBP) over 6- and 12-month periods. Diffusion data from individuals with CBP and healthy controls (HC) were analyzed for comparison. Whole-brain tract-based spatial statistics analyses revealed that a cluster in the right superior longitudinal fasciculus (SLF) tract had larger fractional anisotropy (FA) values in patients who recovered (SBPr) compared to those with persistent pain (SBPp), and predicted changes in pain severity. The SLF FA values accurately classified patients at baseline and follow-up in a third publicly available dataset (Area under the Receiver Operating Curve ~0.70). Notably, patients who recovered had FA values larger than those of HC suggesting a potential role of SLF integrity in resilience to CBP. Structural connectivity-based models also classified SBPp and SBPr patients from the three data sets (validation accuracy 67%). Our results validate the right SLF as a robust predictor of CBP development, with potential for clinical translation. Cognitive and behavioral processes dependent on the right SLF, such as proprioception and visuospatial attention, should be analyzed in subacute stages as they could prove important for back pain chronicity.