Heterogeneous contributions of change in population distribution of body mass index to change in obesity and underweight
Abstract
From 1985 to 2016, the prevalence of underweight decreased, and that of obesity and severe obesity increased, in most regions, with significant variation in the magnitude of these changes across regions. We investigated how much change in mean body mass index (BMI) explains changes in the prevalence of underweight, obesity, and severe obesity in different regions using data from 2896 population-based studies with 187 million participants. Changes in the prevalence of underweight and total obesity, and to a lesser extent severe obesity, are largely driven by shifts in the distribution of BMI, with smaller contributions from changes in the shape of the distribution. In East and Southeast Asia and sub-Saharan Africa, the underweight tail of the BMI distribution was left behind as the distribution shifted. There is a need for policies that address all forms of malnutrition by making healthy foods accessible and affordable, while restricting unhealthy foods through fiscal and regulatory restrictions.
Introduction
Underweight as well as obesity can lead to adverse health outcomes (Prospective Studies Collaboration et al., 2009; Global BMI Mortality Collaboration, 2016; Emerging Risk Factors Collaboration et al., 2011). For at least four decades, the prevalence of underweight has decreased, and that of obesity has increased, in most countries with significant variation in the magnitude of these changes across regions of the world (NCD Risk Factor Collaboration (NCD-RisC), 2017a; NCD Risk Factor Collaboration (NCD-RisC), 2019).
A shift in the whole distribution of body mass index (BMI) would simultaneously affect mean BMI as well as the prevalence of underweight and obesity (Razak et al., 2018; Rose and Day, 1990). In contrast, changes in the shape of BMI distribution – for example, widening or narrowing of the BMI distribution, becoming more or less skewed, or having a thinner or thicker tail – would affect the prevalence of underweight and obesity with only small impacts on the population mean, as shown schematically in Figure 1. Understanding these two mechanisms is essential as they may require different public health and clinical responses (Penman and Johnson, 2006). But it is unclear how much the two mechanisms have contributed to the observed decline in underweight and rise in obesity in different world regions.
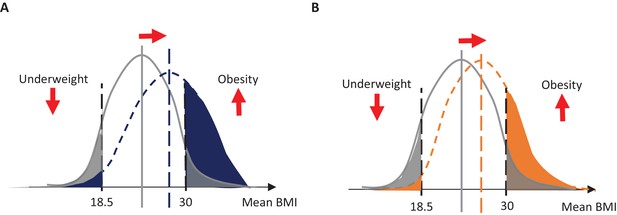
Schematic diagram of contribution of change in mean body mass index (BMI) to change in total prevalence of underweight or obesity.
(A) Change in the prevalence of underweight and obesity if the distribution shifts, represented by a change in its mean and its shape. In this example, the change (shown as the difference between blue and gray) results in a small decrease of underweight and a large increase in obesity. (B) Change in the prevalence of underweight and obesity when only mean BMI changes (shown as the difference between orange and gray), without a change in the shape of the distribution.
Some studies have investigated whether the rise in obesity or the decrease of underweight over time, or differences across countries, were due to a shift in BMI distribution versus changes in the low- or high-BMI tails of the distribution (Razak et al., 2018; Wang et al., 2007; Wagner et al., 2019; Vaezghasemi et al., 2016; Razak et al., 2013; Popkin and Slining, 2013; Popkin, 2010; Peeters et al., 2015; Ouyang et al., 2015; Monteiro et al., 2002; Midthjell et al., 2013; Lebel et al., 2018; Khang and Yun, 2010; Helmchen and Henderson, 2004; Hayes et al., 2015; Green et al., 2016; Flegal and Troiano, 2000; Stenholm et al., 2015; Hayes et al., 2017; Flegal et al., 2012; Bovet et al., 2008). Most of these studies focused on a single or small number of countries over relatively short durations or covered only one sex, a narrow age group, or specific social groups. To understand whether weight gain occurs across all BMI levels or disproportionately affects the underweight or obese segments of the distribution, and how this phenomenon varies geographically, there is a need for a population-based study that simultaneously investigates both underweight and obesity in relation to mean BMI in different regions of the world. We used a comprehensive global database to investigate how much change in mean BMI can explain the corresponding changes in prevalence of adults with underweight (defined as BMI <18.5 kg/m2), total obesity (BMI ≥30 kg/m2), and severe obesity (BMI ≥35 kg/m2) over three decades from 1985 to 2016 in different regions of the world.
Results
Data sources
The Non-Communicable Disease Risk Factor Collaboration (NCD-RisC) database contains 2896 population-based studies conducted from 1985 to 2019 with height and weight measurements of 187 million participants. Of these, 2033 studies had measurements of height and weight on 132.6 million participants aged 20–79 years. The number of studies with participants aged 20–79 years in different regions ranged from 53 in Oceania to 637 in the high-income western region. The number of data sources by country is shown in Figure 2. The list of data sources and their characteristics is provided in Supplementary file 4.
Change in mean BMI and prevalence of underweight, obesity, and severe obesity by region
In 2016, the age-standardised prevalence of underweight was highest (>16% in different sex-age groups) in South Asia; it was <2.5% in Central and Eastern Europe; the high-income western region; Latin America and the Caribbean; Oceania; and Central Asia, the Middle East, and North Africa for most age and sex groups. The age-standardised prevalence of obesity was highest (>24%) in these same regions for most age and sex groups. It was lowest (<7%) in men and women from South Asia; the high-income Asia Pacific region; and men from sub-Saharan Africa. The age-standardised prevalence of severe obesity was highest (12–18%) in women aged 50–79 years from Central Asia, the Middle East, and North Africa; the high-income western region; Central and Eastern Europe; and Latin America and the Caribbean. It was lowest (<2%) in South Asia; East and Southeast Asia; the high-income Asia Pacific region; and men in sub-Saharan Africa.
From 1985 to 2016, age-standardised mean BMI increased by 1–4 kg/m2 in all regions, with the exception of women in the high-income Asia Pacific region and Central and Eastern Europe whose mean BMI changed by less than 1 kg/m2 (Figure 3). The prevalence of underweight decreased or stayed unchanged and that of obesity and severe obesity increased from 1985 to 2016 in all regions, with the exception of an increase in the prevalence of underweight in younger women in the high-income Asia Pacific region. The largest absolute decrease in underweight prevalence from 1985 to 2016 was seen in South Asia; East and Southeast Asia; and sub-Saharan Africa, where it declined by 14–35 percentage points in different age–sex groups (Figure 4). Nonetheless, underweight prevalence remained higher in these three regions than elsewhere in 2016. Prevalence of underweight changed only marginally in regions such as Central and Eastern Europe and the high-income western region, where prevalence was already low in 1985.
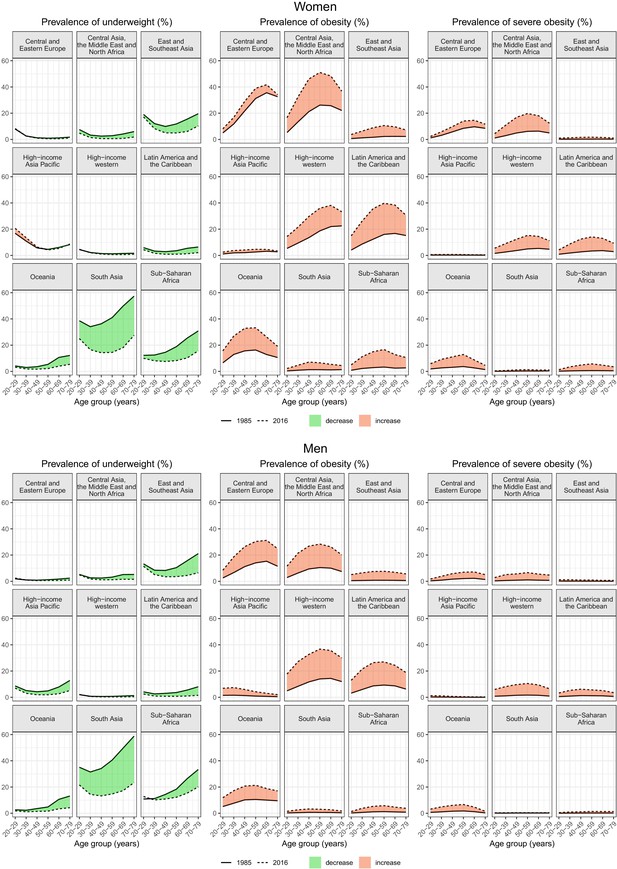
Change in prevalence of underweight, obesity, and severe obesity from 1985 to 2016 by region, sex, and age group.
The largest absolute increase in obesity prevalence from 1985 to 2016 occurred in Central Asia, the Middle East, and North Africa; the high-income western region; Latin America and the Caribbean; Oceania (women); and Central and Eastern Europe (men) (Figure 4). Women in these regions also experienced the largest increase in severe obesity prevalence, along with men in the high-income western region. In these regions and sexes, obesity prevalence increased by 16–24 percentage points in different age groups, and severe obesity increased by 5–13 percentage points. The increase in obesity was less than five percentage points in the high-income Asia Pacific region; South Asia; and in men in sub-Saharan Africa; in the same regions, along with East and Southeast Asia, the increase in severe obesity was less than two percentage points.
Associations of underweight, obesity, and severe obesity prevalence with mean BMI
There was a strong association between the prevalence of underweight, obesity, and severe obesity with mean BMI as measured by R-squared of the regressions of prevalence on mean (Supplementary files 1 and 2). These indicate that 93% (men) and 96% (women) of variation in obesity, and between 83% and 92% of variation in underweight and severe obesity, were explained by mean BMI and other variables (year, region, and age group) in the regression models. The coefficients of the mean BMI terms represent the changes in (probit-transformed) prevalence associated with a unit change in mean BMI, and their interactions with region represent variations in this association across regions. For all three outcomes, the association of prevalence with mean BMI varied across regions.
The inter-regional variation in the prevalence–mean association was stronger for obesity and severe obesity than underweight, as seen in larger inter-regional range of the interaction terms. The extent to which prevalence changes with any variation in mean BMI in each region is an outcome of the main BMI term and its interaction with region; to be epidemiologically interpretable, this will have to be converted from probit-transformed to original prevalence scale. For example, in the year 2016, for women aged 50–59 years, at a mean BMI of 25 kg/m2 (which was approximately the global age-standardised mean level of BMI) (NCD Risk Factor Collaboration (NCD-RisC), 2017a), prevalence of underweight would have varied by seven percentage points across regions, being lowest in Central and Eastern Europe and highest in sub-Saharan Africa; a unit increase in mean BMI would have been associated with a relative change in prevalence ranging from −49% in the high-income Asia Pacific region to −14% in Oceania. Also for women aged 50–59 years and a mean BMI of 25 kg/m2, the prevalence of obesity and severe obesity would both have been the highest in Oceania and the lowest in the high-income Asia Pacific region, with a difference of 12 and 6 percentage points, respectively, for the two outcomes; a unit increase in mean BMI would have been associated with a relative change ranging from 21% to 46% for obesity and from 30% to 59% for severe obesity, the smallest change for both being in Oceania and the largest in East and Southeast Asia. There was similar inter-regional variation in the other year–age–sex strata.
Contribution of mean BMI to changes in underweight and obesity prevalence
The rise in mean BMI accounted for >82% of the decline in underweight in different age–sex groups in South Asia, where underweight prevalence declined by over 16 percentage points for all age–sex groups (Figure 5). The remainder of the decline was due to change in the shape of the BMI distribution which reduced underweight prevalence beyond the effects of the population mean. In contrast, in sub-Saharan Africa and East and Southeast Asia, the total change in prevalence of underweight (3–12 percentage points) was 20–80% less than what was expected based on the increase in mean BMI (Figure 5). In other words, in these regions the underweight tail of the BMI distribution was left behind as the distribution shifted.
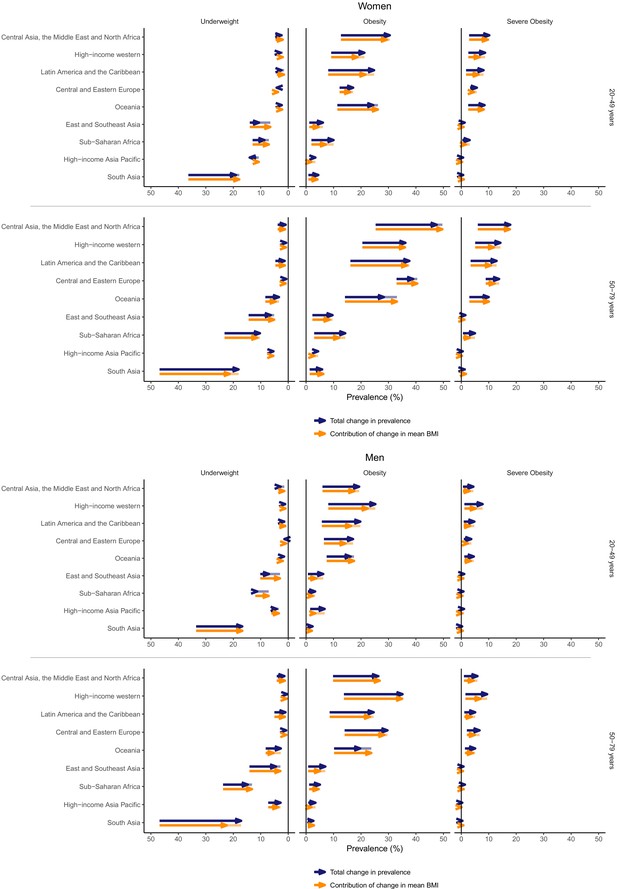
Contribution of change in mean body mass index (BMI) to total change from 1985 to 2016 in prevalence of underweight, obesity, or severe obesity by region, sex, and age group.
Blue arrows show the total change in prevalence of underweight, obesity, or severe obesity. Orange arrows show the contribution of change in mean BMI to the change in prevalence. The difference between these two arrows is shown with a line, whose colour follows the shorter arrow.
Where obesity increased the most – Central Asia, the Middle East, and North Africa; Latin America and the Caribbean; and the high-income western region – the rise in mean BMI accounted for over three quarters of the increase in different age–sex groups (Figure 5). In Oceania, the actual rise in prevalence of obesity (8–14 percentage points for all age–sex groups) was about two-thirds to one-half of what would have been expected by the observed increase in mean BMI in men and women (Figure 5). Change in mean BMI consistently accounted for a smaller share of the change in severe obesity than it did for change in total obesity. Specifically, in regions where prevalence of severe obesity changed by more than one percentage point, the contribution of change in mean BMI to change in severe obesity in different regions was 53–90% of the corresponding contribution for total obesity (Figure 5).
In other regions, the change in the prevalence of underweight, obesity, or severe obesity was too small for the contribution of change in mean BMI to be epidemiologically relevant (Figure 5).
Discussion
We found that the trends in the prevalence of underweight, total obesity, and, to a lesser extent, severe obesity are largely driven by shifts in the distribution of BMI, with smaller contributions from changes in the shape of the distribution. The notable exceptions to this pattern were the decline in the prevalence of underweight in East and Southeast Asia and sub-Saharan Africa and the rise of obesity in Oceania, which were both smaller than expected based on change in mean BMI.
Our results are consistent with a recent cross-sectional study (Razak et al., 2018) using data from women in low- and middle-income countries that found a strong association between mean BMI and prevalence of obesity, and a moderate association between mean BMI and prevalence of underweight. Being cross-sectional, this study did not consider changes over time, as we have. Our results are also consistent with another study which found that changes in median BMI contributed more than 75% to the increase in obesity in the USA from 1980 to 2000 (Helmchen and Henderson, 2004).
Previous studies used one or more approaches to investigate changes in population BMI distribution: some analysed percentiles of the BMI distribution (Wagner et al., 2019; Vaezghasemi et al., 2016; Razak et al., 2013; Popkin and Slining, 2013; Popkin, 2010; Peeters et al., 2015; Ouyang et al., 2015; Lebel et al., 2018; Hayes et al., 2015), others focused on the change in prevalence above or below pre-specified BMI thresholds (Wang et al., 2007; Razak et al., 2013; Popkin, 2010; Peeters et al., 2015; Ouyang et al., 2015; Khang and Yun, 2010; Flegal and Troiano, 2000), or evaluated how the shape of the BMI distribution has changed via examining metrics such as standard deviation and skewness (Peeters et al., 2015; Ouyang et al., 2015; Lebel et al., 2018; Khang and Yun, 2010; Hayes et al., 2015; Flegal and Troiano, 2000). Most of these studies reached the same conclusion as our study that, as the BMI distribution shifts upwards, the prevalence of underweight declines somewhat more slowly than the prevalence of obesity rises.
Our study has strengths in scope, data, and methods: the strengths of our study include presenting the first global analysis of how much the rise in mean BMI versus changes in the shape of its distribution influenced changes in both underweight and obesity prevalence. We used an unprecedented amount of data from different regions covering three decades and used only measured data on height and weight to avoid biases in self-reported data.
As with all global analyses, our study has some limitations. Despite using the most comprehensive global collection of population-based studies to date, some regions, especially Oceania and sub-Saharan Africa, had less data, especially early in our analysis period. Further, given the large number of age, sex, and region subgroups of population in our analysis, and its long duration, it was not possible to visually explore how the shape of BMI distribution has changed in the underweight and obesity ranges where changes in the mean did not fully explain change in prevalence. Finally, there are variations in characteristics such as response rate and measurement protocol across studies. Some of these, such as exclusion of studies with self-reported height and weight, were a part of our inclusion and exclusion criteria. Others may affect population mean or prevalence.
The finding that the majority of the rise in the prevalence of obesity from 1985 to 2016 is mostly the result of a distributional shift points towards an important role for societal drivers, including lower availability and higher price of healthy and fresh foods compared to caloric-dense and nutrient-deficient foods (Swinburn et al., 2011), and mechanisation of work and motorisation of transport throughout the world that have reduced energy expenditure in populations around the world (NCD Risk Factor Collaboration (NCD-RisC), 2019; Ng and Popkin, 2012). First, although there is a genetic component to BMI at the individual level (Silventoinen et al., 2017; Silventoinen et al., 2016; Locke et al., 2015; Brandkvist et al., 2019), genetics explain only a small part of changes over time, especially when people have access to healthy food and living environment. When the environment becomes more obesogenic, some people or population subgroups may gain more weight than others, implying that the environment remains the main contributor (Brandkvist et al., 2019). This interplay of genetic predisposition and changes in the environment might account for some of the excess rise in obesity and severe obesity beyond the effect of distributional shift alone (Brandkvist et al., 2019). The exception observed in Oceania is possibly because in 1985 obesity prevalence in this region was already so high (NCD Risk Factor Collaboration (NCD-RisC), 2017a) that the rise in BMI did not change overall obesity status (but there was a substantial increase in those with severe obesity, mostly accounted for by the change in mean BMI). The smaller decline in underweight than expected in sub-Saharan Africa and East and Southeast Asia may be because underweight is associated with lower socioeconomic status, food insecurity, and for sub-Saharan Africa widening difference between rural and urban BMI levels which is different from other regions (NCD Risk Factor Collaboration (NCD-RisC), 2019; Brandkvist et al., 2019; Di Cesare et al., 2015; Subramanian and Smith, 2006; Di Cesare et al., 2013). If the benefits of economic development do not sufficiently reach the poor, they remain nutritionally vulnerable, as has been seen for height and weight during childhood and adolescence (NCD Risk Factor Collaboration (NCD-RisC), 2020; Subramanyam et al., 2011; Sanchez and Swaminathan, 2005; Pongou et al., 2006; Haddad, 2003; Stevens et al., 2012). Together with the rise in mean BMI and obesity (and short stature which is not a topic of this paper but addressed in other studies) (NCD Risk Factor Collaboration (NCD-RisC), 2020; Stevens et al., 2012; NCD Risk Factor Collaboration (NCD-RisC), 2016a), this creates a double burden of malnutrition (Popkin et al., 2020).
In summary, we found that the worldwide rise in obesity and the decline in underweight are primarily driven by the shift in the population distribution of BMI. At the same time, there is an evidence of both excess obesity, and especially severe obesity, and persistent underweight beyond the distributional shift in some regions, which may be related to growing social inequalities that restrict access to healthy foods in those at highest risk of undernutrition (Popkin et al., 2020; Wells et al., 2020; Darmon and Drewnowski, 2015). The response to these trends must motivate ‘double-duty actions’ that prevent and tackle all forms of malnutrition through both fiscal and regulatory restrictions on unhealthy foods, and making healthy foods available, accessible, and affordable especially to those at high risks of underweight and obesity (Powell et al., 2013; Hawkes et al., 2020; Bleich et al., 2017).
Materials and methods
Study design
Request a detailed protocolOur aim was to quantify, for all regions of the world, how much of the change in prevalence of underweight (defined as BMI <18.5 kg/m2), (total) obesity (BMI ≥30 kg/m2), and severe obesity (BMI ≥35 kg/m2) among men and women aged 20–79 years from 1985 to 2016 could be accounted for by change in mean BMI. In the first step, we used data from a global database of human anthropometry to estimate the associations of the prevalence of underweight, prevalence of obesity, or prevalence of severe obesity with population mean BMI, including how the association varies in relation to age group and region. We then used the fitted association to estimate the contribution of change in the population mean BMI to change in the prevalence of underweight, obesity, or severe obesity in different regions.
Data sources
Request a detailed protocolIn the first step of the analysis, we estimated the prevalence-mean associations, using data from a comprehensive database on cardiometabolic risk factors collated by NCD-RisC as described below. In the second step, we needed consistent estimates of mean BMI for all regions. For this purpose, we used data from a recent comprehensive analysis of worldwide trends in mean BMI from 1985 to 2016 (NCD Risk Factor Collaboration (NCD-RisC), 2017a) which had fitted a Bayesian hierarchical model to the NCD-RisC data.
Data in the NCD-RisC database were obtained from publicly available multi-country and national measurement surveys (e.g., Demographic and Health Surveys, WHO-STEPwise approach to Surveillance [STEPS] surveys, and those identified via the Inter-University Consortium for Political and Social Research and European Health Interview and Health Examination Surveys Database). With the help of the World Health Organization (WHO) and its regional and country offices as well as the World Heart Federation, we identified and accessed population-based survey data from national health and statistical agencies. We searched and reviewed published studies as detailed previously (NCD Risk Factor Collaboration (NCD-RisC), 2017a) and invited eligible studies to join NCD-RisC, as we did with data holders from earlier pooled analysis of cardiometabolic risk factors (Finucane et al., 2011; Farzadfar et al., 2011; Danaei et al., 2011a; Danaei et al., 2011b).
Data inclusion and exclusion
Request a detailed protocolWe carefully checked that each data source met our inclusion criteria as listed below:
measurement data on height and weight were available;
study participants were 5 years of age and older (as described earlier data used here were for those 20–79 years);
data were collected using a probabilistic sampling method with a defined sampling frame;
data were from population samples at the national, sub-national (i.e., covering one or more sub-national regions, with more than three urban or five rural communities), or community level; and
data were from the countries and territories listed in Supplementary file 3.
We excluded all data sources that only used self-reported weight and height without a measurement component because these data are subject to biases that vary with geography, time, age, sex, and socioeconomic characteristics (Tolonen et al., 2014; Hayes et al., 2011; Ezzati et al., 2006). We also excluded data on population subgroups whose anthropometric status may differ systematically from the general population, including
studies that had included or excluded people based on their health status or cardiovascular risk;
studies whose participants were only ethnic minorities;
specific educational, occupational, or socioeconomic subgroups, with the exception noted below; and
those recruited through health facilities, with the exception noted below.
We included school-based data in countries and age–sex groups with enrolment of 70% or higher. We also included data whose sampling frame was health insurance schemes in countries where at least 80% of the population were insured. Finally, we included data collected through general practice and primary care systems in high-income and Central European countries with universal insurance because contact with the primary care systems tends to be as good as or better than the response rates for population-based surveys. The list of data sources with participants aged 20–79 years and their characteristics is provided in Supplementary file 4, with additional information in Source data 1.
Duplicate data were identified by comparing studies from the same country and year, and then discarded. All NCD-RisC members are also periodically asked to review the list of sources from their country, to verify that the included data meet the inclusion criteria and are not duplicates, and to suggest additional sources. The NCD-RisC database is continuously updated through all the above routes. For each data source, we recorded the study population, sampling approach, years of measurement, and measurement methods. Only population-representative data were included, and these were assessed in terms of whether they covered the whole country, multiple sub-national regions, or one or a small number of communities, and whether rural, urban, or both participants were included. All submitted data were checked independently by at least two persons. Questions and clarifications were discussed with NCD-RisC members and resolved before data were incorporated in the database.
We calculated mean BMI and the associated standard errors by sex and age. All analyses incorporated sample weights and complex survey design, when applicable, in calculating summary statistics, with computer code provided to NCD-RisC members who requested assistance.
Additionally, summary statistics for nationally representative data from sources that were identified but not accessed via the above routes were extracted from published reports. Data were also extracted for nine STEPS surveys that were not publicly available, one Countrywide Integrated Non-communicable Diseases Intervention survey, and five sites of the WHO Multinational MONItoring of trends and determinants in CArdiovascular disease (MONICA) project that were not deposited in the MONICA Data Centre. We also included data from a previous global data pooling study (Finucane et al., 2011) when they had not been accessed as described above.
Here, to estimate the association of underweight, obesity, and severe obesity prevalence with mean BMI as described below, we used data collected from 1985 to 2019 with measured height and weight among men and women aged 20–79 years, in 10-year age groups. Data that did not cover the complete 10-year age groups, for example, 25–29 or 60–64 years, were excluded. We included data from study–age–sex strata with a prevalence between 0 and 1 to allow probit transformation and with at least 25 participants in each stratum. These data were summarised into 11,652 study–age–sex-specific pairs of mean and prevalence of adults with underweight, obesity, or severe obesity.
Statistical methods
Request a detailed protocolAnonymised data from studies in the NCD-RisC database were reanalysed according to a common protocol. We calculated mean BMI and prevalence of underweight, obesity, and severe obesity by sex and age group in each study in the NCD-RisC database from 1985 to 2019. We used data through 2019 so that the prevalence–mean association is informed by as much data as possible. All calculations took into account complex survey design where relevant. We excluded study–age–sex groups with less than 25 participants because their means and prevalence have larger uncertainty.
We then estimated the relationship between probit-transformed prevalence of underweight, obesity, and severe obesity and mean BMI in a regression model, separately for each of these prevalences. The correlation coefficient between mean BMI and median BMI was ≥0.98 in different age–sex groups, indicating a strong correlation between the two. In our statistical model, described below, the prevalence of underweight, obesity, or severe obesity depends on population mean BMI as well as on age group, region, and year.
All analyses were done separately for men and women. We chose a probit-transformed prevalence because it changes in an approximately linear manner as the mean changes, thus providing a better fit to the data. The regressions also included age group in 10-year bands, region and the year when the data were collected. The regions, used in previous analyses of cardiometabolic risk factors (NCD Risk Factor Collaboration (NCD-RisC), 2017a; NCD Risk Factor Collaboration (NCD-RisC), 2019; NCD Risk Factor Collaboration (NCD-RisC), 2020; NCD Risk Factor Collaboration (NCD-RisC), 2016a; NCD Risk Factor Collaboration (NCD-RisC), 2018; NCD Risk Factor Collaboration (NCD-RisC), 2017b; NCD Risk Factor Collaboration (NCD-RisC), 2016b; NCD Risk Factor Collaboration (NCD-RisC), 2016c), were Central and Eastern Europe; Central Asia, the Middle East, and North Africa; East and Southeast Asia; high-income Asia Pacific region; high-income western region; Latin America and the Caribbean; Oceania; South Asia; and sub-Saharan Africa. Countries in each region are listed in Supplementary file 3. The model also included interactions between mean BMI and age group, mean BMI and region, age group and region, age group and year, and year and region. These terms allowed the prevalence–mean association to vary by age group, region, and over time. The models were fitted in statistical software R (version 4.0.2) (Source code 1). The coefficients of the regression models are presented in Supplementary files 1 and 2.
We used the fitted regressions to quantify how much of the change over time in the prevalence of underweight, obesity, or severe obesity in each region and age group can be explained by the corresponding change in mean BMI. To do so, we first used the region- and age–sex-specific mean BMI in 1985 and 2016 in the fitted association and then estimated the total change in prevalence of underweight, obesity, or severe obesity by region. The mean BMI values were from a recent comprehensive analysis of worldwide trends in mean BMI (NCD Risk Factor Collaboration (NCD-RisC), 2017a) and are listed in Supplementary file 5. We then calculated the contribution of change in mean BMI to the change in prevalence of underweight or obesity by allowing mean BMI for each age group and region to change over time, while keeping year fixed at 1985. Results were calculated by 10-year age group and then aggregated into two age bands, 20–49 and 50–79 years, by taking weighted average of age-specific results using weights from the WHO standard population (Ahmad et al., 2001).
Data availability
Names and characteristics of data sources included in this pooling analysis are listed in Supplementary file 4. Of these data, some are from public sources, for which we have provided the data in Source data 1. Others are from individual researchers and/or from government and international agencies; these should be requested from the data holders on a study to study basis using the information in Source data 1.
References
-
U.S. nutrition assistance, 2018 - Modifying SNAP to promote population healthNew England Journal of Medicine 376:1205–1207.https://doi.org/10.1056/NEJMp1613222
-
Trends in national and state-level obesity in the USA after correction for self-report Bias: analysis of health surveysJournal of the Royal Society of Medicine 99:250–257.https://doi.org/10.1177/014107680609900517
-
Changes in the distribution of body mass index of adults and children in the US populationInternational Journal of Obesity 24:807–818.https://doi.org/10.1038/sj.ijo.0801232
-
Population-level trends in the distribution of body mass index in England, 1992-2013Journal of Epidemiology and Community Health 70:832–835.https://doi.org/10.1136/jech-2015-206468
-
Reducing child malnutrition: how far does income growth take Us?The World Bank Economic Review 17:107–131.https://doi.org/10.1093/wber/lhg012
-
Age-specific changes in BMI and BMI distribution among Australian adults using cross-sectional surveys from 1980 to 2008International Journal of Obesity 39:1209–1216.https://doi.org/10.1038/ijo.2015.50
-
Modelling obesity trends in Australia: unravelling the past and predicting the futureInternational Journal of Obesity 41:178–185.https://doi.org/10.1038/ijo.2016.165
-
Changes in the distribution of body mass index of white US men, 1890-2000Annals of Human Biology 31:174–181.https://doi.org/10.1080/03014460410001663434
-
Population-level trends in the distribution of body mass index in Canada, 2000-2014Canadian Journal of Public Health 109:539–548.https://doi.org/10.17269/s41997-018-0060-7
-
Is obesity replacing or adding to undernutrition? Evidence from different social classes in BrazilPublic Health Nutrition 5:105–112.https://doi.org/10.1079/PHN2001281
-
Contributions of mean and shape of blood pressure distribution to worldwide trends and variations in raised blood pressure: a pooled analysis of 1018 population-based measurement studies with 88.6 million participantsInternational Journal of Epidemiology 47:872–883.https://doi.org/10.1093/ije/dyy016
-
The changing shape of the body mass index distribution curve in the population: implications for public health policy to reduce the prevalence of adult obesityPreventing Chronic Disease 3:A74.
-
Health impacts of macroeconomic crises and policies: determinants of variation in childhood malnutrition trends in CameroonInternational Journal of Epidemiology 35:648–656.https://doi.org/10.1093/ije/dyl016
-
Recent dynamics suggest selected countries catching up to US obesityThe American Journal of Clinical Nutrition 91:284S–288.https://doi.org/10.3945/ajcn.2009.28473C
-
New dynamics in global obesity facing low- and middle-income countriesObesity Reviews 14 Suppl 2:11–20.https://doi.org/10.1111/obr.12102
-
Genetic and environmental effects on body mass index from infancy to the onset of adulthood: an individual-based pooled analysis of 45 twin cohorts participating in the COllaborative project of development of anthropometrical measures in twins (CODATwins) studyThe American Journal of Clinical Nutrition 104:371–379.https://doi.org/10.3945/ajcn.116.130252
-
Differences in genetic and environmental variation in adult BMI by sex, age, time period, and region: an individual-based pooled analysis of 40 twin cohortsThe American Journal of Clinical Nutrition 106:457–466.https://doi.org/10.3945/ajcn.117.153643
-
Patterns, distribution, and determinants of under- and overnutrition: a population-based study of women in IndiaThe American Journal of Clinical Nutrition 84:633–640.https://doi.org/10.1093/ajcn/84.3.633
-
Change in the distribution of body mass index in Brazil: analysing the interindividual inequality between 1974 and 2013Journal of Epidemiology and Community Health 73:544–548.https://doi.org/10.1136/jech-2018-211664
-
Trends in the distribution of body mass index among Chinese adults, aged 20-45 years (1989-2000)International Journal of Obesity 31:272–278.https://doi.org/10.1038/sj.ijo.0803416
Article and author information
Author details
Funding
Wellcome Trust
- Majid Ezzati
Medical Research Council
- Maria LC Iurilli
The funders had no role in study design, data collection and interpretation, or the decision to submit the work for publication.
Acknowledgements
We thank WHO country and regional offices and World Heart Federation for support in data identification and access. The NCD-RisC database was funded by the Wellcome Trust. Maria LC Iurilli was supported by a Medical Research Council studentship. Sylvain Sebert received funding by the European Commission with grant agreements 633595 and 874739, respectively, for the DynaHEALTH and LongITools projects. The following contributors have deceased: Konrad Jamrozik, Altan Onat, Robespierre Ribeiro, Michael Sjöström, Agustinus Soemantri, Jutta Stieber, and Dimitrios Trichopoulos. The list of authors shows their last affiliation.
Copyright
© 2021, NCD Risk Factor Collaboration (NCD-RisC)
This article is distributed under the terms of the Creative Commons Attribution License, which permits unrestricted use and redistribution provided that the original author and source are credited.
Metrics
-
- 9,628
- views
-
- 1,374
- downloads
-
- 58
- citations
Views, downloads and citations are aggregated across all versions of this paper published by eLife.
Download links
Downloads (link to download the article as PDF)
Open citations (links to open the citations from this article in various online reference manager services)
Cite this article (links to download the citations from this article in formats compatible with various reference manager tools)
Further reading
-
- Epidemiology and Global Health
- Microbiology and Infectious Disease
Several areas of the world suffer a notably high incidence of Shiga toxin-producing Escherichia coli. To assess the impact of persistent cross-species transmission systems on the epidemiology of E. coli O157:H7 in Alberta, Canada, we sequenced and assembled E. coli O157:H7 isolates originating from collocated cattle and human populations, 2007–2015. We constructed a timed phylogeny using BEAST2 using a structured coalescent model. We then extended the tree with human isolates through 2019 to assess the long-term disease impact of locally persistent lineages. During 2007–2015, we estimated that 88.5% of human lineages arose from cattle lineages. We identified 11 persistent lineages local to Alberta, which were associated with 38.0% (95% CI 29.3%, 47.3%) of human isolates. During the later period, six locally persistent lineages continued to be associated with human illness, including 74.7% (95% CI 68.3%, 80.3%) of reported cases in 2018 and 2019. Our study identified multiple locally evolving lineages transmitted between cattle and humans persistently associated with E. coli O157:H7 illnesses for up to 13 y. Locally persistent lineages may be a principal cause of the high incidence of E. coli O157:H7 in locations such as Alberta and provide opportunities for focused control efforts.
-
- Epidemiology and Global Health
Given the rapid cross-country spread of SARS-CoV-2 and the resulting difficulty in tracking lineage spread, we investigated the potential of combining mobile service data and fine-granular metadata (such as postal codes and genomic data) to advance integrated genomic surveillance of the pandemic in the federal state of Thuringia, Germany. We sequenced over 6500 SARS-CoV-2 Alpha genomes (B.1.1.7) across 7 months within Thuringia while collecting patients’ isolation dates and postal codes. Our dataset is complemented by over 66,000 publicly available German Alpha genomes and mobile service data for Thuringia. We identified the existence and spread of nine persistent mutation variants within the Alpha lineage, seven of which formed separate phylogenetic clusters with different spreading patterns in Thuringia. The remaining two are subclusters. Mobile service data can indicate these clusters’ spread and highlight a potential sampling bias, especially of low-prevalence variants. Thereby, mobile service data can be used either retrospectively to assess surveillance coverage and efficiency from already collected data or to actively guide part of a surveillance sampling process to districts where these variants are expected to emerge. The latter concept was successfully implemented as a proof-of-concept for a mobility-guided sampling strategy in response to the surveillance of Omicron sublineage BQ.1.1. The combination of mobile service data and SARS-CoV-2 surveillance by genome sequencing is a valuable tool for more targeted and responsive surveillance.