Unsupervised changes in core object recognition behavior are predicted by neural plasticity in inferior temporal cortex
Figures
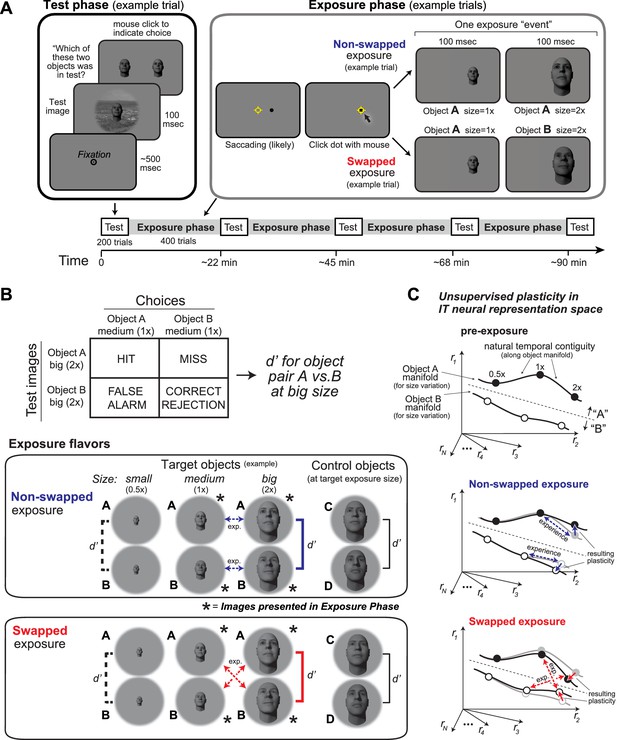
Experimental design and conceptual hypothesis.
(A) Illustration of human behavioral experimental design and an example trial from the Test phase and from the Exposure phase. (B) Top: example confusion matrix for a two-way alternative forced choice (2AFC) size-specific sub-task run during each Test phase to monitor object-specific, size-specific changes in discrimination performance (see Materials and methods). Bottom: the two unsupervised exposure flavors deployed in this study (see Materials and methods). Only one of these was deployed during each Exposure phase (see Figure 2). Exposed images of example-exposed objects (here, faces) are labeled with asterisks, and the arrows indicate the exposure events (each is a sequential pair of images). Note that other object and sizes are tested during the Test phases, but not exposed during the Exposure phase (see d’ brackets vs. asterisks). Each bracket with a d’ symbol indicates a preplanned discrimination sub-task that was embedded in the Test phase and contributed to the results (Figure 2). In particular, performance for target objects at non-exposed size (d’ labeled with dashed lines), target objects at exposed size (d’ labeled with bold solid lines), and control objects (d’ labeled with black line) was calculated based on test phase choices. (C) Expected qualitative changes in the inferior temporal (IT) neural population representations of the two objects that results from each flavor of exposure (based on Li and DiCarlo, 2010). In each panel, the six dots show three standard sizes of two objects along the size variation manifold of each object. Assuming simple readout of IT to support object discrimination (e.g., linear discriminant, see Majaj et al., 2015), non-swapped exposure tends to build size-tolerant behavior by straightening out the underlying IT object manifolds, while swapped exposure tends to disrupt (‘break’) size-tolerant behavior by bending the IT object manifolds toward each other at the swapped size. This study asks if that idea is quantitatively consistent across neural and behavioral data with biological data-constrained models.
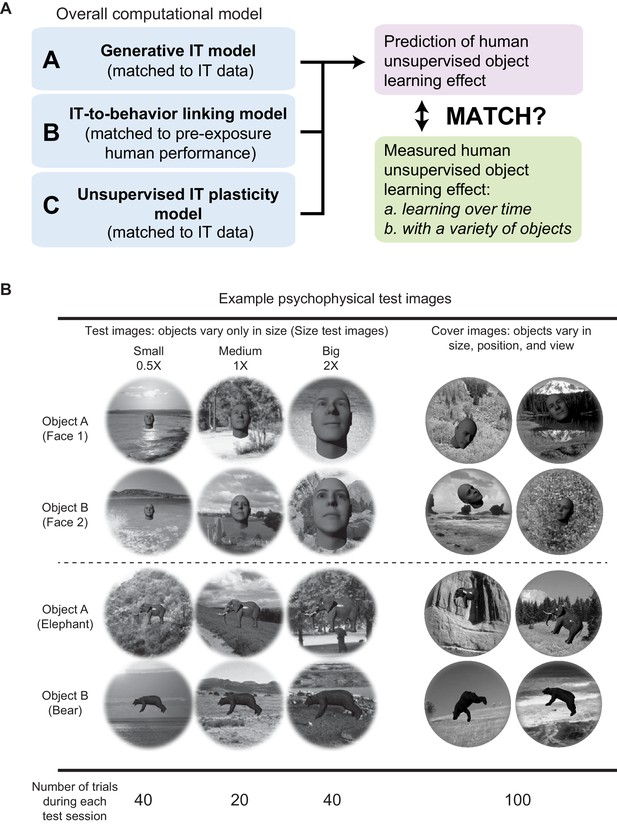
Outline and example test images.
(A) Outline diagram. (B) Example test images and number of different types of test images per test phase (200 trials total).
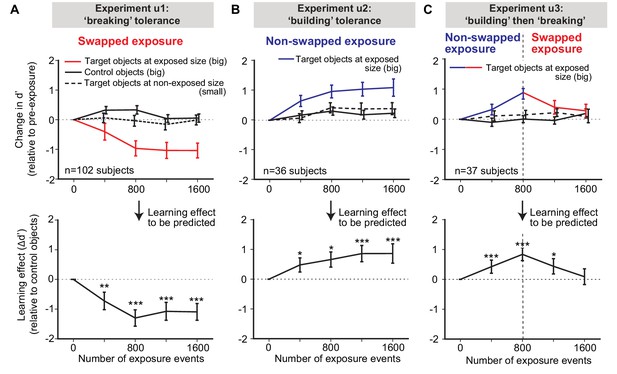
Measured human unsupervised learning effects as a function of amount of unsupervised exposure.
Each ‘exposure event’ is the presentation of two, temporally adjacent images (see Figure 1A, right). We conducted three longitudinal unsupervised exposure experiments (referred to as u1, u2, and u3). (A) Swapped exposure experiment intended to ‘break’ size tolerance (n = 102 subjects; u1). Upper panels are the changes in d’ relative to initial d’ for targeted objects (faces) at exposed size (big) (red line), control objects (other faces) at the same size (big) (black line), and targeted faces at non-exposed size (small) (dashed black line) as a function of number of exposure events prior to testing. Lower panel is the to-be-predicted learning effect determined by subtracting change of d’ for control objects from the change of d’ for target objects (i.e., red line minus black line). (B) Same as (A), but for non-swapped exposure experiment (n = 36 subjects; u2). (C) Same as (A), except for non-swapped exposure followed by swapped exposure (n = 37 subjects; u3) to test the reversibility of the learning. In all panels, performance is based on population pooled d’ (see Materials and methods). Error bars indicate bootstrapped standard error of the mean population pooled d’ (bootstrapping is performed by sampling with replacement across all trials). p-value is directly estimated from the bootstrapped distributions of performance change by comparing to no change condition. * indicates p-value<0.05; ** indicates p-value<0.01; *** indicates p-value<0.001.
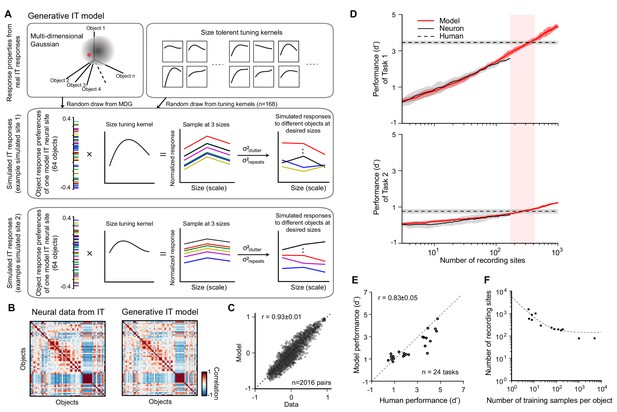
Generative ITmodel and validation of the IT-to-core object recognition (COR)-behavior-linking model.
(A) Generative IT model based on real IT population responses. Top left box: schematic illustration of the neuronal representation space of IT population with a multi-dimensional Gaussian (MDG) model. Each point of the Gaussian cloud is one IT neural site. Middle box: an example of simulated IT neural site. The distribution of object preference for all 64 objects is created by a sample randomly drawn from the MDG (highlighted as a red dot; each color indicates a different object). Then, a size-tuning kernel is randomly drawn from a pool of size-tuning curves (upper right box; kernels fit to real IT data) and multiplied by the object response distribution (outer product), resulting in a fully size-tolerant (i.e., separable) neural response matrix (64 objects × 3 sizes). To simulate the final mean response to individual images with different backgrounds, we added a ‘clutter’ term to each element of the response matrix (σ2clutter; see Materials and methods). To simulate the trial-by-trial ‘noise’ in the response trials, we added a repetition variance (σ2repeats; see Materials and methods). Bottom box: another example of simulated IT site. (B) Response distance matrices for neuronal responses from real IT neuronal activity (n = 168 sites) and one simulated IT population (n = 168 model sites) generated from the model. Each matrix element is the distance of the population response between pairs of objects as measured by Pearson correlation (64 objects, 2016 pairs). (C) Similarity of the model IT response distance matrix to the actual IT response distance matrix. Each dot represents the unique values of the two matrices (n = 2016 object pairs), calculated for the real IT population sample and the model IT population sample (r = 0.93 ± 0.01). (D) Determination of the two hyperparameters of the IT-to-behavior-linking model. Each panel shows performance (d’) as a function of number of recording sites (training images fixed at m = 20) for model (red) and real IT responses (black) for two object discrimination tasks (task 1 is easy, human pre-exposure d’ is ~3.5; task 2 is hard, human pre-exposure d’ is ~0.8; indicated by dashed lines). In both tasks, the number of IT neural sites for the IT-to-behavior decoder to match human performance is very similar (n ~ 260 sites), and this was also true for all 24 tasks (see E), demonstrating that a single set of hyperparameters (m = 20, n = 260) could explain human pre-exposed performance over all 24 tasks (as previously reported by Majaj et al., 2015). (E) Consistency between human performance and model IT-based performance of 24 different tasks for a given pair of parameters (number of training samples m = 20 and number of recording sites n = 260). The consistency between model prediction and human performance is 0.83 ± 0.05 (Pearson correlation ± SEM). (F) Manifold of the two hyperparameters (number of recording sites and number of training images) where each such pairs (each dot on the plot) yields IT-based performance that matches initial (i.e., pre-exposure) human performance (i.e., each pair yields a high consistency match between IT model readout and human behavior, as in E). The dashed line is an exponential fit to those dots at any of the three sizes as the outer product of the object and size-tuning curves (A, bottom). However, since most measured size-tuning curves are not perfectly separable across objects (DiCarlo et al., 2012; Rust and Dicarlo, 2010) and because the tested conditions included arbitrary background for each condition, we introduced independent clutter variance caused by backgrounds on top of this for each size of an object (A) by randomly drawing from the distribution of variance across different image exemplars for each object. We then introduced trial-wise variance for each image based on the distribution of trial-wise variance of the recorded IT neural population (Figure 3—figure supplement 1E). In sum, this model can generate a new, statistically typical pattern of IT response over a population of any desired number of simulated IT neural sites to different image exemplars within the representation space of 64 base objects at a range of sizes (here targeting ‘small,’ ‘medium,’ and ‘big’ sizes to be consistent with human behavioral tasks; see Materials and methods for details). The simulated IT population responses were all constrained by recorded IT population statistics (Figure 3—figure supplement 1). These statistics define the initial simulated IT population response patterns, and thus they ultimately influence the predicted unsupervised neural plasticity effects and the predicted behavioral consequences of those neural effects.
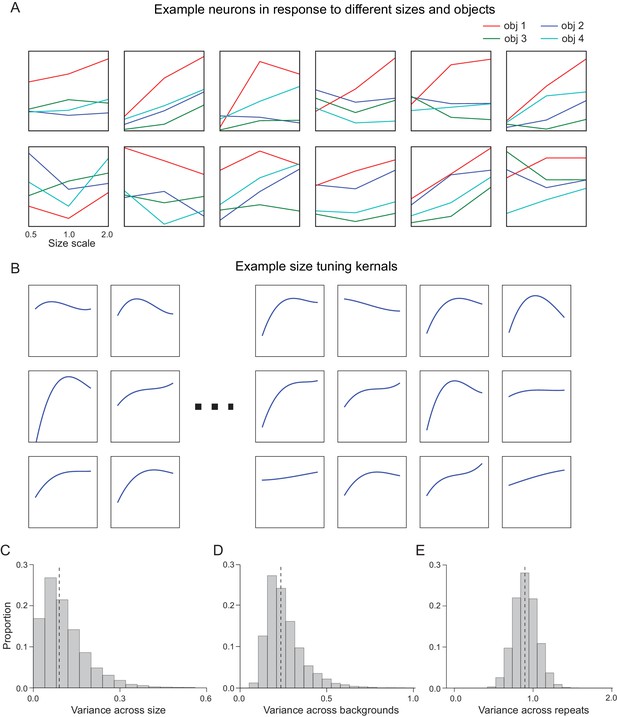
Supplemental information for generative IT model.
(A) Example recorded IT neurons in response to different sizes and objects (normalized response by z-score). (B) Example size-tuning kernels. (C) Distribution of variance across size for all IT neurons and all objects (n = 168 sites * 64 objects). (D) Distribution of variance across backgrounds (n = 168 sites * 64 objects). (E) Distribution of variance across repeats. Dashed line indicates median of distribution (n = 168 sites * 64 objects * 10 exemplars).
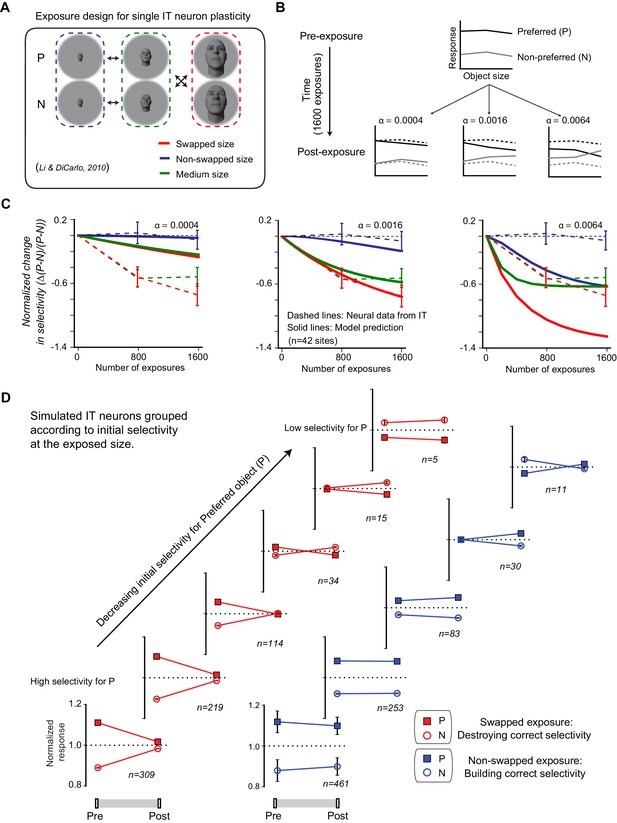
Temporal continuity-based inferior temporal (IT) neuronal plasticity rule.
(A) Illustration of exposure design for IT neuronal plasticity (adapted directly from Li and DiCarlo, 2010) measured with single electrodes. P refers to preferred object of the IT unit, and N refers to non-preferred object of that unit. (B) We developed an IT plasticity rule that modifies the model neuronal response to each image in individual neural sites according to the difference in neuronal response between lagging and leading images for each exposure event (see Materials and methods). The figure shows the model-predicted plasticity effects for a standard, size-tolerance IT neural site and 1600 exposure events (using the same exposure design as Li and DiCarlo, 2010; i.e., 400 exposure events delivered [interleaved]) for each of the four black arrows in panel (A) for three different plasticity rates. Dashed lines indicate model selectivity pattern before learning for comparison. (C) Normalized change over time for modeled IT selectivity for three different plasticity rates. Dashed lines are the mean neuronal plasticity results from the same neural sites in Li and DiCarlo, 2010 (mean change in P vs. N responses, where the mean is taken over all p > N selective units that were sampled and then tested; see Li and DiCarlo, 2010). Solid lines are the mean predicted neuronal plasticity for the mean IT model ‘neural sites’ (where these sites were sampled and tested in a manner analogous to Li and DiCarlo, 2010; see Materials and methods). Blue line indicates the change in P vs. N selectivity at the non-swapped size, green indicates change in selectivity at the medium size, and red indicates change in selectivity at the swapped size. Error bars indicate standard error of the mean. (D) Mean swapped object (red) and non-swapped object (blue) plasticity that results for different model IT neuronal sub-groups – each selected according to their initial pattern of P vs. N selectivity (analogous to the same neural sub-group selection done by Li and DiCarlo, 2010; c.f. their Figure 6).
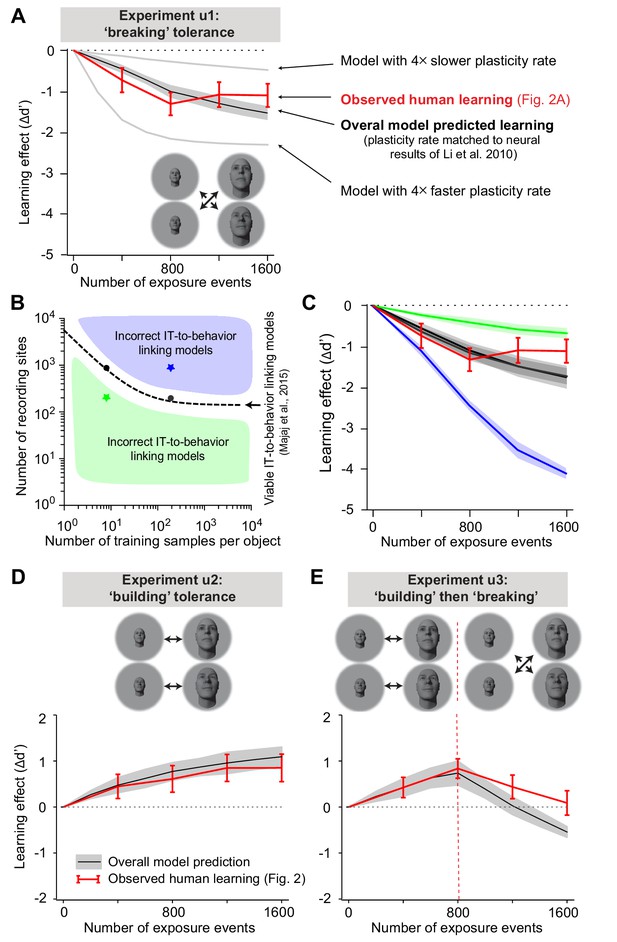
Overall model-predicted learning effects vs. actual learning effects.
(A) Overall model-predicted learning effect (solid black line) for experiment u1 (swapped exposure) with the IT-to-behavior-linking model matched to initial human performance (hyperparameters: number of training images m = 20, number of model neural sites n = 260; see Figure 3) and the IT plasticity rate matched to prior IT plasticity data (0.0016; see Figure 4). Red line indicates measured human learning effect (reproduced from Figure 2A, lower). Gray lines indicate model predictions for four times smaller plasticity rate and four times larger plasticity rate. Error bars are standard error over 100 runs of the overall model; see text. (B) Decoder hyperparameter space: number of training samples and number of neural features (recording sites). The dashed line indicates pairs of hyperparameters that give rise to IT-to-behavior performances that closely approximate human initial (pre-exposure) human object recognition performance over all tasks. (C) Predicted unsupervised learning effects with different choices of hyperparameters (in all cases, the IT plasticity rate was 0.0016 – i.e., matched to the prior IT plasticity data; see Figure 4). The two black lines (nearly identical, and thus appear as one line) are the overall model-predicted learning that results from hyperparameters indicated by the black dots (i.e., two possible correct settings of the decoder portion of the overall model, as previously established by Majaj et al., 2015). Green and blue lines are the overall model predictions that result from hyperparameters that do not match human initial performance (i.e., non-viable IT-to-behavior-linking models). (D) Predicted learning effect (black line) and measured human learning effect (red) for building size-tolerance exposure. (E) Model-predicted learning effect (black line) and measured human learning effect (red) for building and then breaking size-tolerance exposure. In both (D) and (E), the overall model used the same parameters as in (A) (i.e., IT plasticity rate of 0.0016, number of training samples m = 20, and number of model neural sites n = 260).
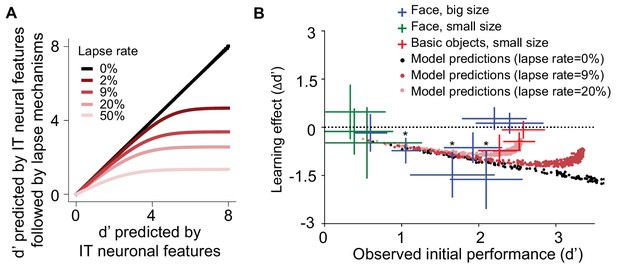
Learning effect as a function of initial task difficulty.
(A) Illustration of the saturation of measured d’ that results from the assumption that the subject guesses on a fraction of trials (lapse rate), regardless of the quality of the sensory evidence provided by the visually evoked inferior temporal (IT) neural population response (x-axis). (B) Measured human learning effect for different tasks (colored crosses) as a function of initial (pre-exposure) task difficulty (d’) with comparison to model predictions with or without lapse rate (dots). Each cross or dot is a specific discrimination task. For crosses, different colors indicate different types of tasks and exposures: green indicates small-size face discrimination learning effect induced with medium-small swapped exposure (n = 100 subjects); blue indicates big-size face discrimination learning effect induced with medium-big swapped exposure (n = 161 subjects); red indicates small-size basic-level discrimination learning effect induced with medium-small swapped exposure (n = 70 subjects). Performance is based on population pooled d’. Error bars indicate bootstrapped standard error of the mean population pooled d’ (bootstrapping is performed by sampling with replacement across all trials). p-value is directly estimated from the bootstrapped distributions of performance change by comparing to no change condition. * indicates p-value<0.05.
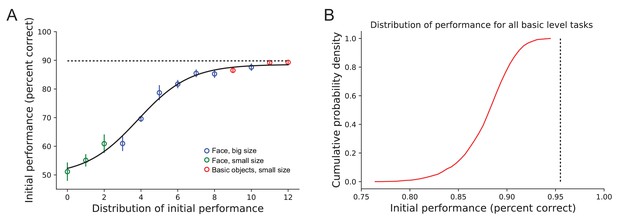
Distribution of initial task performance.
(A) Population performance of all tasks in Figure 6, rank ordered by an estimation of the sensory evidence available on each task (x-axis). Tasks are ranked by their performance calculated from half of the trials (an estimate of sensory evidence strength), and the mean population performance of the other half of the trials is plotted. A sigmoid least-squares fit is shown. This figure suggests a saturation of performance around 90%, which corresponds to a lapse rate of 20% (indicated by dashed line). Error bars indicate standard deviation variance of random splits across trials (n = 100 splits). (B) Probability density distribution of initial performance accuracy of all basic-level tasks in Figure 6. Dashed line indicates the 95.5% performance corresponding to 9% lapse rate.