Adapting non-invasive human recordings along multiple task-axes shows unfolding of spontaneous and over-trained choice
Figures
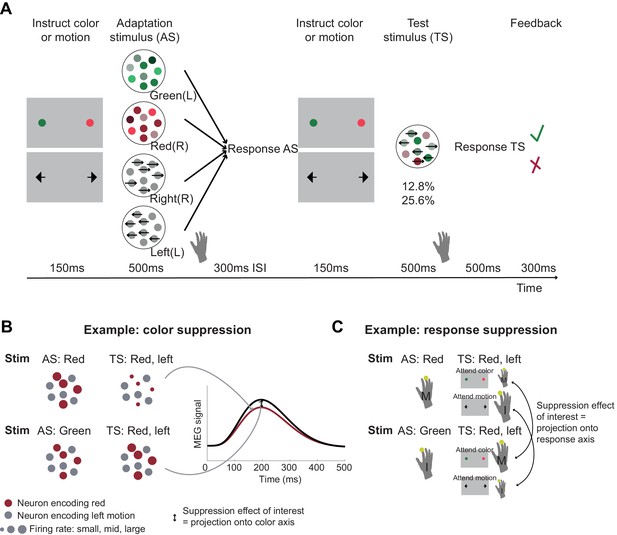
Experimental task involving manipulation of specific choice features.
(A) Human participants performed a perceptual choice task adapted from the macaque version in Mante et al., 2013 while magnetoencephalography (MEG) data were recorded. Each trial involved two random-dot-motion stimuli – an adaptation stimulus (AS) and a test stimulus (TS). A flanker cue (coloured dots or arrows) instructed which choice dimension, colour or motion, to attend to for making a choice. Responses were given using the right-hand index or middle finger for green/left and red/right stimuli, respectively. By presenting two choices with varying features in rapid succession, we selectively suppressed the subset of neurons sensitive to repeated features. To maximize suppression effects, AS colour and motion was strong compared to the TS (70% compared to 25.6 or 12.8% motion coherence or colour dominance). Feedback at the end of each trial related to performance on the TS. In total, there were 64 conditions: 4 AS x 2 contexts x 2 directions x 2 colours x 2 coherence levels. The rationale for the selective suppression of choice features is illustrated for two examples in (B) and (C). (B) The top and bottom rows show two combinations of AS and TS that were compared to extract colour suppression. At the time of the TS (the focus of all analyses), the stimulus is identical, containing predominantly red colour and left-ward motion. If preceded by a red AS (top row), any red-coding neurons will show a reduced signal at the time of the TS (red dots), but any other neurons will show the same response (grey dots). Thus, the overall MEG signal will be reduced compared to a situation where the preceding AS stimulus does not share any features with the TS (green; bottom row). This suppression effect, i.e., the difference in the MEG signal for two identical TS as a function of their preceding AS (arrow) can be captured in a time-resolved manner, thus showing not only whether but also when colour is being processed. This experimental repetition suppression manipulation can be conceptualized as a projection (or ‘squashing’) of the MEG signal onto the axis that captures variation in colour processing. (C) The sequence of stimuli shown in (B) can also be used to probe response suppression. When participants are attending to colour at the time of the TS, a middle finger response will be repeated in the top example but not in the bottom. If they are attending to motion, an index finger response will be repeated in the bottom but not in the top example. The respective differences (arrows) thus provide a time-resolved measure of response suppression, analogous to projections of neurons onto a response axis.
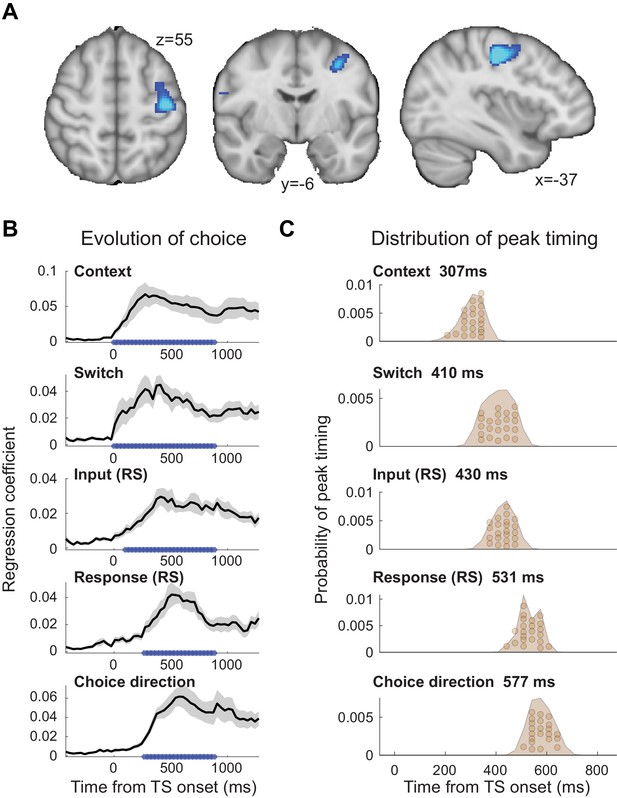
The evolution of choice in human premotor cortex.
(A) Dorsal premotor cortex (PMd) was the a priori region of interest for this study. Indeed, using source localization and a contrast between trials containing any input or response adaptation, compared to no adaptation, revealed a cluster involving M1 and left PMd (group contrast, whole-brain cluster-level FWE-corrected at p<0.05 after initial thresholding at p<0.001; PMd peak coordinate: x=-37, y=-6, z = 55). Importantly, this contrast was agnostic and orthogonal to any differences between input and response adaptation, relevant and irrelevant input adaptation, or pre- vs. post-training effects (including any timing differences, as beamforming was performed in the time window [−250, 750] ms). (B) The time-resolved nature of magnetoencephalography (MEG), combined with a selective suppression of different choice features, allowed us to track the evolution of the choice on a millisecond timescale. Linear regression coefficients from a regression performed on data from left premotor cortex (PMd) demonstrate an early representation of context (motion or colour) and switch (attended dimension same or different from TS) from ~8 ms (sliding window centred on 8 ms contains 150 ms data from [−67,83] ms). The representation of inputs, as indexed using repetition suppression (RS), emerged from around 108 ms (whether or not the input feature, colour, or motion was repeated). Finally, the motor response, again indexed using RS (same finger used to respond to adaptation stimulus [AS] and test stimulus [TS] or not), and TS choice direction (left or right, unrelated to RS) were processed from 275 ms. *p<0.001; error bars denote SEM across participants; black line denotes group average. (C) The distribution of individual peak times across the 22 participants directly reflects this evolution of the choice process. In particular, it shows significant differences in the processing of input and response, consistent with premotor cortex transforming sensory inputs into a motor response.
-
Figure 2—source data 1
Contains 'pre' and 'post' [Time x Regressors x Subjects] for Figure 2B.
- https://cdn.elifesciences.org/articles/60988/elife-60988-fig2-data1-v2.mat
-
Figure 2—source data 2
Contains 'dat' [Regressors x Subjects] for Figure 2B.
- https://cdn.elifesciences.org/articles/60988/elife-60988-fig2-data2-v2.mat
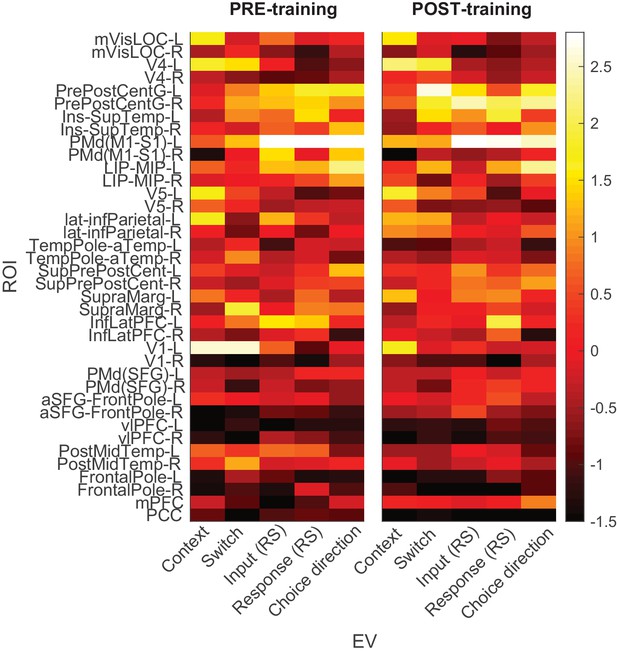
Premotor cortex processes choice inputs and outputs.
Dorsal premotor cortex (PMd) was the a priori region of interest for this study. As shown in Figure 2A, beamforming for source localization identified a cluster involving left PMd from a contrast probing any input or response adaptation across both pre- and post-training sessions. Here we show an additional parcellation into 38 regions using beamforming, which confirmed that the linear regression coefficients for both input and response suppression were strongest in the parcel containing premotor cortex (PMd(M1-S1)-L). Colours denote the maximum linear regression coefficient in the range [−500,1150] ms. Unlike in Figure 6, this analysis used the same regressors used to examine PMd responses in Figures 2–4, with input and response regressors capturing repetition suppression effects.
-
Figure 2—figure supplement 1—source data 1
Contains 'pre' and 'post' [ROIs x Regressors].
- https://cdn.elifesciences.org/articles/60988/elife-60988-fig2-figsupp1-data1-v2.mat
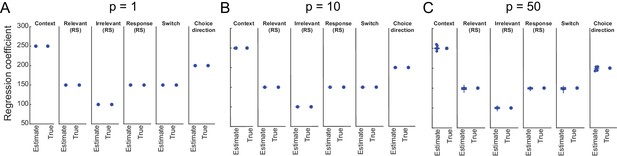
Simulations show sufficient parameter recovery given the experimental design.
To test whether we could recover linear regression coefficients using the same design matrix as used in the experiment, we first simulated magnetoencephalography (MEG) signals using y = X∗b + ε∗p, where y is a vector of size T × 1, X is the design matrix of a specific subject in the experiment of size T × M, b is the vector of true linear regression coefficients of size M × 1, T is the number of trials, M is the number of regressors, ε is Gaussian noise drawn from N(0,1), and p is the noise multiplication factor. We set b, the true linear regression coefficients to [250, 150, 100, 150, 150, 200]. These are analogous to the six regressors in the main analyses (Context, Relevant adaptation [RelvAdpt], Irrelevant adaptation [IrrelAdpt], Response adaptation [RespAdpt], Switch, and Choice). We varied the noise multiplication factor between [1, 5, 50]. We used a regularized regression to mimic the regression procedure used for the real data. We varied the regularization term (λ) between [0.00001, 0.00005, 0.0001, 0.0005] and used the optimal one in each case. We repeated this analysis for all subjects and averaged the estimated linear regression coefficients across subjects. We found that for all noise levels (A for p=1 [λ = 0.00001]; B for p=10 [λ = 0.0001]; C for p=50 [λ = 0.0005]), we could accurately recover the true values. The horizontal line indicates the median. The bottom and top edges of the box indicate the 25th and 75th percentiles, respectively. The circles denote outliers and the whiskers extend to the most extreme data points not considered as outliers.
-
Figure 2—figure supplement 2—source data 1
Contains 'a_est', 'b_est', 'c_est' [Subjects x Regressors], and 'betas_true' [Regressors x 1].
- https://cdn.elifesciences.org/articles/60988/elife-60988-fig2-figsupp2-data1-v2.mat
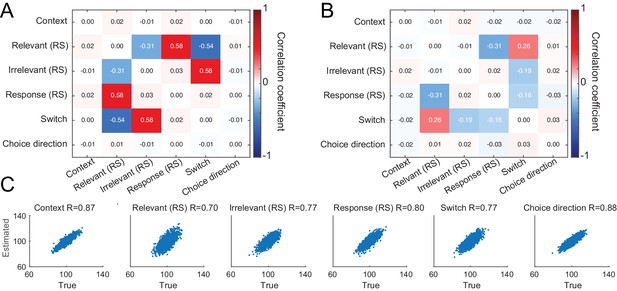
Simulations show sufficient independence between estimated linear regression coefficients.
In addition to showing sufficient parameter recovery (Figure 2—figure supplement 2), we probed the correlation among estimated linear regression coefficients. Even if the true coefficients were not correlated, estimated coefficients may show artificial dependencies due to correlations present in the design matrix. To investigate this possibility, we generated synthetic data using y = X∗b + ε∗p, as before. This time, we set all values in b to the same value of 100. Critically, we added Gaussian noise drawn from N(0,5) to each linear regression coefficient separately. We repeated this procedure 100 times for each subject and calculated the correlation between estimated linear regression coefficients for the five regressors within a given subject. We set p and λ to 50 and 0.0005, respectively, as in the previous simulation. We averaged obtained correlation matrices across all subjects. We found that the correlation across estimated linear regression coefficients (B) was lower than expected, given correlations present in design matrix (A). Again, we confirmed that linear regression coefficients were recoverable for all regressors (C; scatter plots showing results from all subjects; R corresponds to Pearson’s R between estimated and true linear regression coefficients).
-
Figure 2—figure supplement 3—source data 1
Contains 'a', 'b' [Regressors x Regressors], 'c_betas_true' and 'c_betas_pred' [Repetition x Regressors x Subjects].
- https://cdn.elifesciences.org/articles/60988/elife-60988-fig2-figsupp3-data1-v2.mat
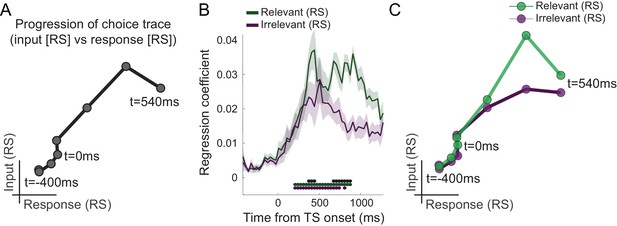
Choice trace: progression along task-axes shows filtering out of irrelevant inputs in PMd.
(A) By plotting the processing of input on one and response on the other axis (both as in Figure 2B), we can derive a choice trace that shows the signal progression along the two task-axes, thus mimicking state space trajectories obtained from non-human primate (NHP) recordings (Mante et al., 2013). (B) Separation of input suppression into relevant and irrelevant inputs shows slightly diminished processing of irrelevant inputs in dorsal premotor cortex (PMd) (p<0.001; black * shows the difference between relevant and irrelevant inputs, significant between 375–442 and 675–875 ms; green and purple * indicates significance separately for relevant and irrelevant inputs). (C) This can also be seen in the choice trace split for relevant and irrelevant inputs. We observed partial but incomplete filtering of irrelevant inputs in PMd.
-
Figure 3—source data 1
Contains 'pre.relirrel' and 'post.relirrel' [Time x Regressors x Subjects].
- https://cdn.elifesciences.org/articles/60988/elife-60988-fig3-data1-v2.mat
-
Figure 3—source data 2
Contains structs of 'pre' and 'post'.
These structs have four regressors [Time x 1].
- https://cdn.elifesciences.org/articles/60988/elife-60988-fig3-data2-v2.mat
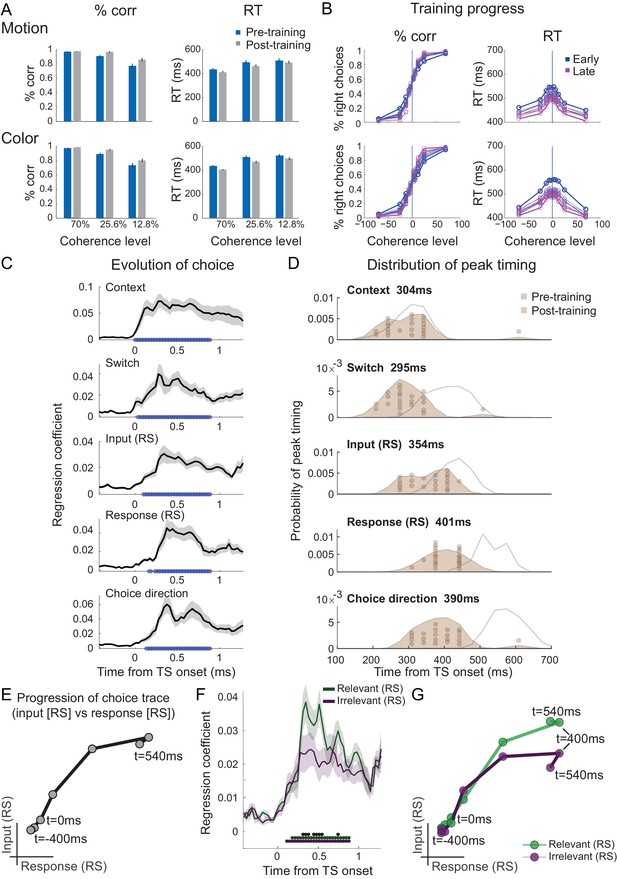
Filtering out of irrelevant inputs remains after over-training on >20,000 trials.
(A) Over a period of 4 weeks, participants performed >1000 trials of training on the random-dot-motion task for 5 or 6 days a week before returning for two further post-training magnetoencephalography (MEG) sessions. Comparison of pre- and post-training task performance showed significant reaction time (RT) speeding and performance improvements (% correct choices) in both colour and motion contexts. (B) Performance improvements are also clear in psychometric curves plotted across training sessions (blue = early, purple = late; see also Figure 5A and Figure 5—figure supplement 1A). (C) The evolution of a choice can be tracked in PMd post-training (as shown in Figure 2B for the pre-training sessions). (D) However, peak timings of the majority of regressors occur earlier in the post-compared to the pre-training data, suggesting a faster and possibly more efficient coding of the choice process. (E) The choice trace extracted from the repetition suppression regressors for input and response in PMd shows a transformation from a processing of inputs to a processing of outputs, similar to Figure 3A, for the data acquired pre-training. (F) The difference between the processing of relevant versus irrelevant inputs is preserved even after extensive training, thus suggesting some filtering out of irrelevant inputs. (G) This can be visualized in two divergent choice traces, separately showing relevant and irrelevant input adaptation.
-
Figure 4—source data 1
Contains 'dat' [Regressors x Subjects].
- https://cdn.elifesciences.org/articles/60988/elife-60988-fig4-data1-v2.mat
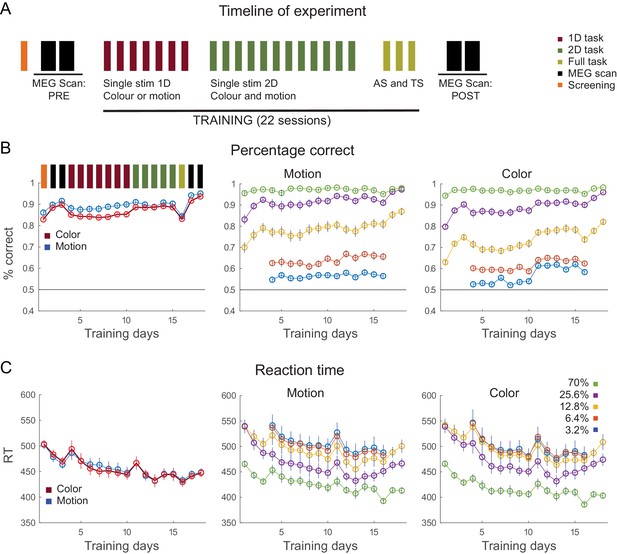
Timeline, performance progression during training sessions, and behavioural effects of relevant and irrelevant inputs and AS.
(A) The timeline of the experiment is illustrated. The manuscript focusses on the neural data acquired from the two PRE-training and the two POST-training magnetoencephalography (MEG) scans (black). The training performed in between was performed in three stages, starting with random-dot-motion (RDM) stimuli containing only one dimension (colour or motion: 1D), progressing onto RDM stimuli containing both dimensions (colour and motion: 2D), followed by three sessions that contained 2D stimuli pairing the adaptation stimulus (AS) and the test stimulus (TS). Not all participants completed all 7 1D + 12 2D + 3 (AS/TS) = 22 training sessions. Everyone performed the screening, the four MEG sessions, and all seven 1D sessions. Of the 12 2D sessions, participants completed between 5–12 (mean 10.7) and of the final three full task sessions, they completed between 1–3 (mean 2.5) before coming back for the two post-training MEG sessions. In total, everyone completed >20,000 trials before the final MEG sessions and, on average, 26,594 trials (minimum 20,288; maximum 28,688). (B) Choice performance (% correct) is shown for the sessions completed by all participants, split by motion and colour (left), by coherence level for motion (middle) and for colour (right). (C) The same progression as in (C) is shown for reaction times (RTs).
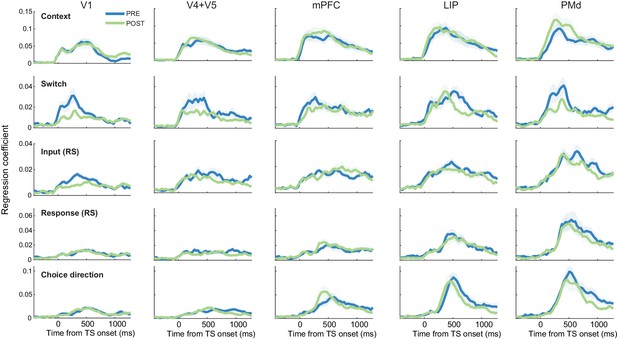
Transition from choice to response processing is specific to PMd.
All key analyses were repeated for four other regions: primary visual cortex (V1), higher-order visual cortices (V4 +V5), medial prefrontal cortex (mPFC), and lateral intraparietal area (LIP). We found that dorsal premotor cortex (PMd) (right most column) showed the strongest evidence for a decision computation transitioning from input to response processing. We used the same parcellation as in Figure 2—figure supplement 1 to extract source data (see 'Materials and methods': 'Region of interest') for all regions, including PMd. Error bars denote SEM across participants; blue lines and green lines indicate pre- and post-training, respectively.
-
Figure 4—figure supplement 2—source data 1
Contains 'pre' and 'post' [Time x Regressors x Subjects] for LIP.
- https://cdn.elifesciences.org/articles/60988/elife-60988-fig4-figsupp2-data1-v2.mat
-
Figure 4—figure supplement 2—source data 2
Contains 'pre' and 'post' [Time x Regressors x Subjects] for PMd.
- https://cdn.elifesciences.org/articles/60988/elife-60988-fig4-figsupp2-data2-v2.mat
-
Figure 4—figure supplement 2—source data 3
Contains 'pre' and 'post' [Time x Regressors x Subjects] for V1.
- https://cdn.elifesciences.org/articles/60988/elife-60988-fig4-figsupp2-data3-v2.mat
-
Figure 4—figure supplement 2—source data 4
Contains 'pre' and 'post' [Time x Regressors x Subjects] for V4.
- https://cdn.elifesciences.org/articles/60988/elife-60988-fig4-figsupp2-data4-v2.mat
-
Figure 4—figure supplement 2—source data 5
Contains 'pre' and 'post' [Time x Regressors x Subjects] for mPFC.
- https://cdn.elifesciences.org/articles/60988/elife-60988-fig4-figsupp2-data5-v2.mat
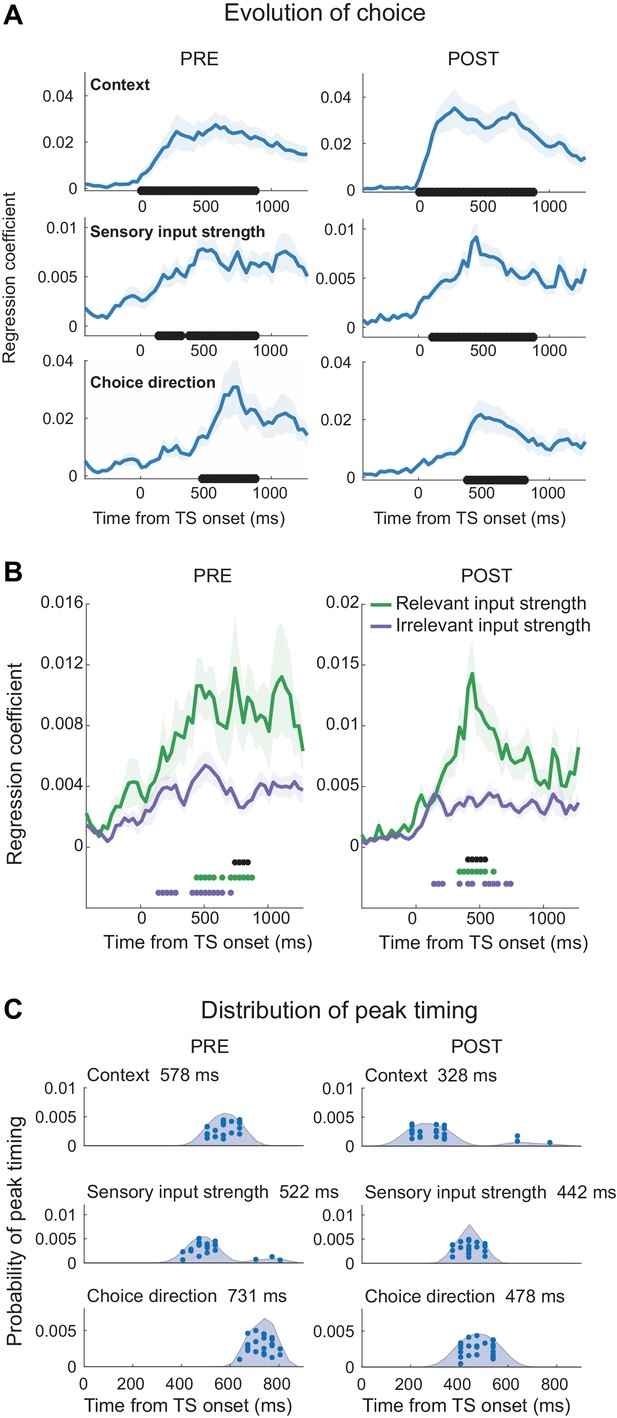
Task feature processing independent of repetition suppression in PMd.
The same statistical analysis procedures were used as in Figures 2–4 but this time the generalized linear model (GLM) only contains regressors that are independent of any repetition suppression; all regressors relate to the stimulus properties of the test stimulus (TS) alone and are used to explain the univariate signal measured across trials in dorsal premotor cortex (PMd). Even though the task was not optimized for this analysis, this analysis confirmed our key effects, albeit with less specificity than the main repetition suppression analysis (e.g., here we cannot rule out the influence of confounding factors such as attention or difficulty). (A) All task variables are processed in PMd. (B) Relevant input strength is processed more strongly than irrelevant input strength, both pre- and post-training. (C) Timings indicate a transition from input strength to response direction processing.
-
Figure 4—figure supplement 3—source data 1
Contains 'pre' and 'post' [Time x Regressors x Subjects].
- https://cdn.elifesciences.org/articles/60988/elife-60988-fig4-figsupp3-data1-v2.mat
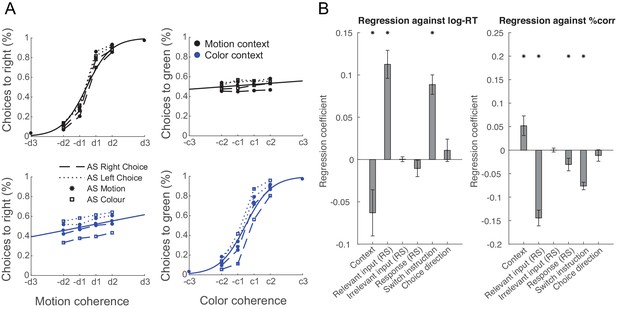
Manipulating information content through repetition suppression changes behaviour.
(A) Psychometric curves show a strong influence of the relevant feature (colour in a colour trial and motion in a motion trial) and a weak influence of the irrelevant feature (colour in a motion trial and motion in a colour trial), consistent with data in non-human primates (Mante et al., 2013). In addition, suppressing motion inputs affects choices based on motion and suppressing colour inputs affects choices based on colour, but not vice versa. (B) Relevant, but not irrelevant, input adaptation slowed log-reaction times (RTs), and both relevant input adaptation and response adaptation reduced choice accuracies (% correct). This is consistent with a potential neuronal fatigue mechanism whereby repeated exposure to a feature reduces the received input, which would be expected to affect behaviour.
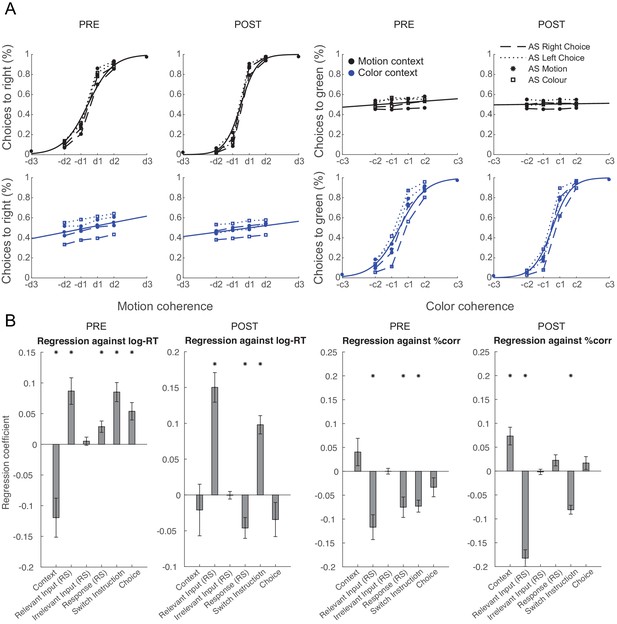
Pre- vs. post-training effects of repetition suppression on behavioural performance.
(A) Psychometric curves are shown using the same conventions as in Figure 5A but separately for pre- and post-training. (B) Regression analyses performed on log-reaction times (RTs) and choice accuracies, as shown in Figure 5B, shown separately for pre- and post-training. While effects of relevant sensory input adaptation became more pronounced with training, response adaptation effects diminished.
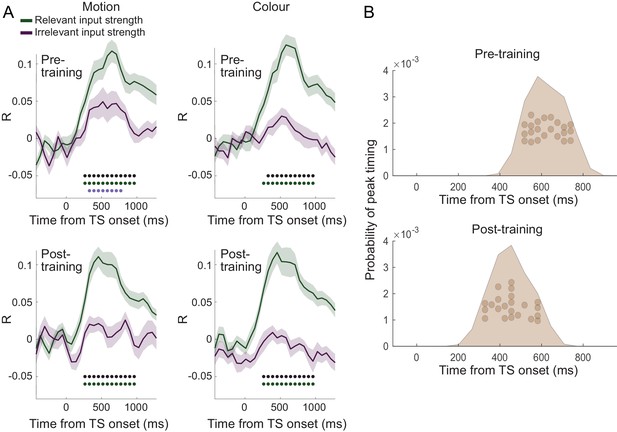
Filtering of irrelevant inputs is also present in whole-brain decoding.
(A) Decoding performed on the source reconstructed signal in 38 parcels covering the whole brain showed a significant difference in decoding accuracy between relevant and irrelevant inputs, suggesting an attenuation of information about irrelevant choice features. Thus, a filtering out of irrelevant information is apparent even when considering data from the whole brain, and it is not affected by extensive training on the task (compare top vs. bottom rows). As in Figure 3B, black * denotes significance at p<0.001 for the difference between relevant and irrelevant input coding; green and purple * denotes significance for relevant and irrelevant input decodings, respectively. (B) Consistent with the encoding approach focused on PMd, peak decoding times are faster following the training when considering data from the whole brain.
-
Figure 6—source data 1
Contains structs of 'pre' and 'post'.
These structs have two structs 'relv' and 'irrel', having two matrices of 'motion' and 'colour' [Subjects x Time].
- https://cdn.elifesciences.org/articles/60988/elife-60988-fig6-data1-v2.mat
-
Figure 6—source data 2
Contains 'pre' and 'post' [1 x Subjects].
- https://cdn.elifesciences.org/articles/60988/elife-60988-fig6-data2-v2.mat
Additional files
-
Supplementary file 1
Task conditions and the corresponding regressors.
The list of task conditions and corresponding regressors of the experiment are shown. The four bold lines are illustrated as examples in Figure 1B.
- https://cdn.elifesciences.org/articles/60988/elife-60988-supp1-v2.docx
-
Transparent reporting form
- https://cdn.elifesciences.org/articles/60988/elife-60988-transrepform-v2.pdf