Deciphering the combinatorial landscape of immunity
Figures
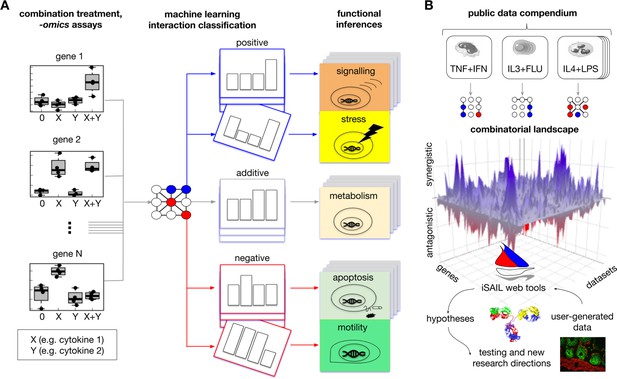
Comprehensive approach to map interaction effects within and among -omics datasets from combination treatment experiments.
(A) We developed iSAIL (immune Synergistic/Antagonistic Interaction Learner), a machine learning framework to decipher the effect of combination treatments. iSAIL accommodates -omics datasets from the four prototypical conditions of combination treatments: 0 (control), stimulus X, stimulus Y, and the combination X+Y (left subpanel). The dataset is analyzed by a classifier previously trained to map each gene into a complete taxonomy of theoretically possible interaction profiles (middle subpanel, see also Figure 1—figure supplement 1). The slanting of a single profile rendition in the middle subpanel is to indicate that the slanted pattern is one of an entire deck of synergistic or antagonistic patterns classified by iSAIL. The taxonomy helps infer the functional role of different types of positive and negative interactions (right subpanel). (B) By applying iSAIL to combination treatments from diverse immune cell types (right subpanel), we built a combinatorial landscape of immunity comprising ~30,000 interactions. Global analysis of the landscape and of user-generated data drive new hypotheses on the role of interactions in immune cells.
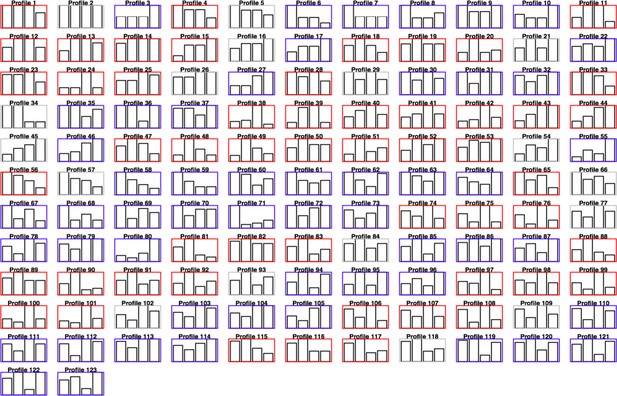
A comprehensive taxonomy of response profiles to combination treatments.
Each card corresponds to a possible response profile of a standard combination treatment experiment involving the conditions 0 (control), X, Y, and the combination X+Y. The color code keeps track of the sign of interaction: red for positive interactions, blue for negative interactions, gray for additive. The taxonomy includes all the qualitative responses defined in our previous work (Cappuccio et al., 2015), as well as additional profiles that capture the semi-quantitative effect of X, Y in case these two signals have opposite effects (one gene is upregulated by X and downregulated by Y or vice versa). In this case, we introduce new profiles by comparing the magnitude of the opposing effect.
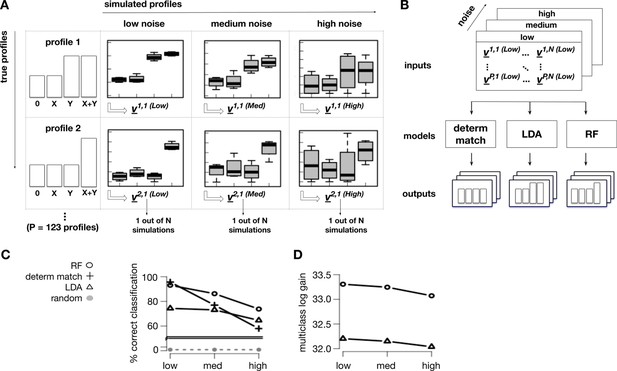
Machine learning classification of treatment interactions.
(A) We generated a training set of simulated interaction profiles. Each profile was simulated multiple times with realistic group means for the conditions 0, X, Y, X+Y. Variability around the group means was assumed to be normally distributed. To build a robust classifier, we simulated three noise levels: low, medium, high. From each simulated profile i, instance j, and noise level n, we extracted a vector vi,j (n)of statistical features including the p-values for all possible pairwise contrasts from the groups 0, X, Y, X+Y. (B) The vectors vi,j (n), labeled with their originating profiles, provided a training set. The training set was used to develop a machine learning classifier that takes as input a vector of statistical features, and predicts as output the most probable profile. We compared three classification algorithms: Deterministic Match, Linear Discriminant Analysis (LDA), and Random Forests (RF). (C) To compare their performance, we measured the classification accuracy -defined as the fraction of correct predictions- on independent test sets. RF provided the most consistent distribution of accuracy over the different profiles in the three noise regimes. (D) To further compare LDA and RF, we computed the multiclass log gain, a metric that accounts for the overall distribution of class probabilities returned by these classifiers. Again, RF showed the best performance and was selected as the most robust model.
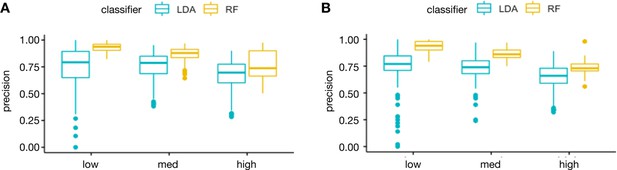
Distribution of precision and recall of LDA and RF across the taxonomy of response profiles.
To evaluate the performance of LDA and RF, we measured the distribution of precision (A) and recall (B) over all the 123 elements in the taxonomy of response profiles to combination treatments (Figure 1—figure supplement 1). These metrics were evaluated on independent test sets simulating three levels of noise: low, medium, and high. The results show that LDA fails to detect certain response profiles even in the low noise range. Overall, RF provides higher precision and recall, and a more consistent performance over the different classes.
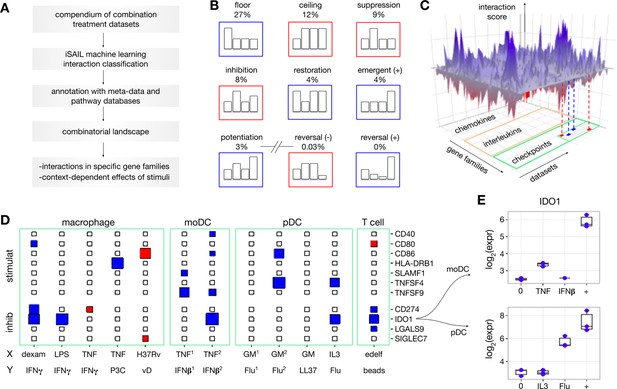
Building a combinatorial landscape of immunity.
(A) We developed a strategy to map and investigate combinatorial effects from multiple combination treatment experiments. Using public repositories, we selected 32 -omics datasets of combination treatment experiments from diverse immune cells and combinations of stimuli. For each dataset, we applied iSAIL and classified a total of ~30,000 interaction effects. (B) The first seven cards represent the most frequent interactions across datasets. The last two cards represent interactions that occur with vanishingly low frequency. (C) To integrate interactions from different datasets, we created a 3D structure with axes representing datasets, genes, and scores quantifying the intensity and robustness of the effects. The resulting landscape, supplemented with metadata and prior knowledge, makes it possible to comprehensively investigate the effect of combination treatments on immune cells. (D) The plane shows a 2D projection of the landscape focusing on immune checkpoints in selected datasets: (stimulat = stimulatory; inhibit = inhibitory). The size and color of the rectangles keep track respectively of the interaction score and sign (blues: positive interactions, red: negative interactions). The immune checkpoint IDO1 is synergistically induced in multiple datasets. Non-standard abbreviations: P3C = Pam3 CSK; vD = vitamin D; GM = GM CSF; edelf = edelfosine; Flu = influenza virus. (E) By looking at the specific nature of these synergies, we found two cases of potentiation in human moDC (top) and pDC (bottom).
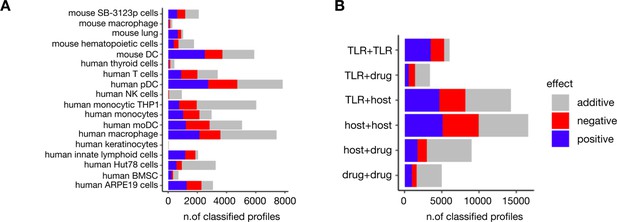
Quantification of interaction by cell type and type of combination.
Quantification of the ~30,000 interactions included in combinatorial landscape aggregated by immune cell type (A) and by type of combination (B). Non-standard abbreviations: host = host derived factors (e.g. cytokines).
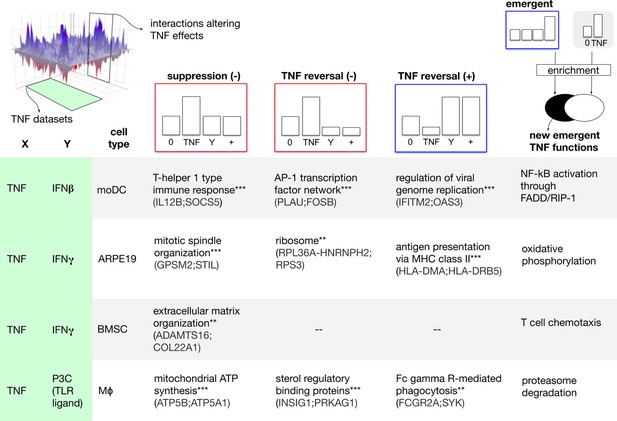
Combinatorial interactions determine a context-dependent TNF biology.
To explore how cofactors might alter the TNF biology, we sliced the combinatorial landscape (top-left) along two axes. From the dataset axis, we extracted combination treatments involving TNF and concomitant factors including IFNγ and IFNβ in four human cellular models (left-margin). From the interaction axis, we extracted interaction profiles that encode a qualitative change of the effect of TNF mono-treatment. We started by considering three types of qualitative changes: suppression, antagonistic reversal, and synergistic reversal (top-margin). For each dataset and profile, we processed the corresponding gene list with enrichment analysis to gain insight at functional level. The matrix elements correspond to selected significantly enriched functions (hypergeometric test; * adjusted p<0.05, ** adjusted p<0.01, *** adjusted p<0.001). Example hits from each function are shown in parentheses. In the case of emergent effects (last column), we looked for the presence of new functions, not regulated by TNF alone (top right, see also Materials and methods). The results suggest that cofactors could drive the emergence of new TNF functions.
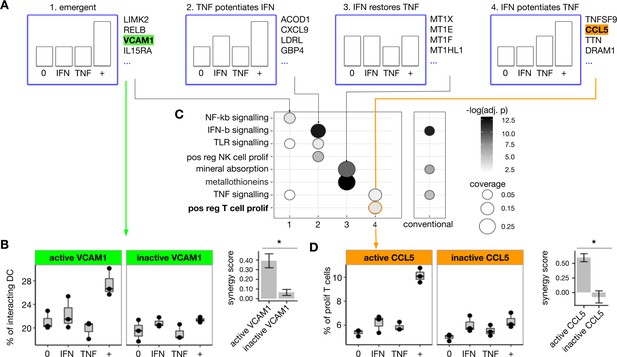
Prediction and validation of IFNβ and TNF synergistic effects in monocyte-derived dendritic cells .
(A) We applied iSAIL to analyze the synergistic effects induced by IFNβ (3000 pg/mL) and TNF (4500 pg/mL) on monocyte-derived dendritic cells after 1 hr of exposure. We focused on the four profile groups with the largest number of synergies. In the group of emergent synergies, we found VCAM1, a gene involved in the regulation of cell adhesion. (B) We tested the hypothesis that the emergent induction of VCAM1 in the DC would mediate an increase in DC-T cell interaction. Assayed by imaging flow cytometry, moDCs exposed to IFNβ+TNF showed an emergent increase in DC-T cell adhesion. VCAM-1 neutralization abolished this synergistic effect. The right subpanel shows the mean synergy score of the three replicates, plus or minus the standard error of the mean. VCAM-1 neutralization significantly reduced the synergy score (t-test, p=0.03). (C) To explore whether TNF+IFNβ synergy profiles represented coherent gene programs, we determined the functional enrichment for each of the four patterns, shown in the columns. (C, left panel). We compared these iSAIL-based functional analyses to results obtained with a conventional analysis of unclassified synergy genes. (C, right panel). iSAIL-based enrichment provided a richer functional annotation. In particular, it suggested that synergy genes in the ‘IFNβ potentiates TNF’ pattern rightmost panel in (A) may mediate T cell proliferation. (D) This hypothesis was tested using allogeneic cross donor stimulation. The synergy group ‘IFNβ potentiates TNF’ contained two hits potentially responsible for T cell proliferation: TNFSF9 and CCL5. Using CCL-5 neutralizing antibodies, we confirmed that IFNβ and TNF act in synergy to promote T cell proliferation, and that this proliferation depends on CCL-5. The right subpanel shows the mean synergy score of the three replicates, plus or minus the standard error of the mean. CCL-5 neutralization significantly reduced the synergy score (t-test, p=0.008).
Tables
Datasets used to construct the combinatorial landscape of immunity.
Accession | Species | Cell type | Signal X | Signal Y | Time point |
---|---|---|---|---|---|
GSE5054 | Human | Thyroid cells | IFNγ | IL1β | 1d |
GSE36331 | Human | ARPE-19 cells | IFNγ | TNF | 2d |
GSE43409 | Human | Innate lymphoid cells | cocktail (IL-1/IL-7/IL-23) | aNKp44 | 3.5 hr |
GSE53712 | Human | Monocytic THP-1 | LPS | SB203580 | 4h |
GSE53712 | Human | Monocytic THP-1 | LPS | SB203580 | 1d |
GSE59179 | Human | Hut78 cells | Enzastaurin | AR-A014418 | 3d |
GSE63038 | Human | NK cells | FcR activation | IL-12 | 12 hr |
GSE79077 | Human | MDMs | Dexamethasone | IFNγ | 20 hr |
GSE57915 | Human | pDC | IL3 | Flu | 6h |
GSE57915 | Human | pDC | GM-CSF | Flu | 6h |
GSE57915 | Human | pDC | GM-CSF | Flu | 1d |
GSE57915 | Human | pDC | GM-CSF | LL37 | 1d |
GSE57915 | Human | Monocytes | NOD2 | TLRs | 6h |
GSE57915 | Human | Monocytes | NOD2 | TLRs | 1d |
GSE57915 | Human | Monocytes | IFNγ | TLRs | 6h |
GSE46903 | Human | Macrophage | IFNγ | TNF | 3d |
GSE46903 | Human | Macrophage | TNF | P3C | 3d |
GSE36323 | Human | Monocytic THP-1 | D3 | TsA | 2.5 hr |
GSE52819 | Human | Macrophage | Vitamin D | H37Rv | 24 hr |
GSE44392 | Human | CD4+ T cell | edelfosine | beads | 30 hr |
GSE24767 | Human | Keratinocyte | IL-17 | TNF | 1d |
GSE77814 | Human | BMSC | IFNγ | TNF (1.5 ng/ml) | 2d |
GSE77814 | Human | BMSC | IFNγ | TNF (15 ng/ml) | 2d |
GSE134209 | Human | moDC | TNF | IFNβ | 1h |
GSE134209 | Human | moDC | TNF | IFNβ | 2.5 hr |
GSE20302 | Mouse | DC | Lact acidophilus | Bifid bifidum | 10 hr |
GSE28994 | Mouse | Lung | Pam2CSK4 | ODN2395 | 4h |
GSE32986 | Mouse | DC | Curdlan (1 mg/ml) | GM-CSF | 4h |
GSE32986 | Mouse | DC | Curdlan (100 mg/ml) | GM-CSF | 4h |
GSE35291 | Mouse | HSPCs | Valproic acid | lithium | 7d |
GSE53986 | Mouse | macrophage | IFNγ | LPS | 1d |
GSE62249 | Mouse | SB-3123p cells | Cocktail (TNF/IFNγ) | Vemurafenib | 4d |
-
Non-standard abbreviations: MDM = Monocyte Derived Macrophages; P3C = Pam3 CSK; D3 = nuclear hormone 1,25(OH)2D3; TsA = trichostatin A; BMSC = Bone Marrow Stromal Cells; HSPCs = hematopoietic stem/progenitor cells; Lact = Lactobacillus; Bifid = Bifidobacterium.
Additional files
-
Source data 1
VCAM-1 neutralizing experiment.
- https://cdn.elifesciences.org/articles/62148/elife-62148-data1-v2.csv.zip
-
Source data 2
CCL-5 neutralizing experiment.
- https://cdn.elifesciences.org/articles/62148/elife-62148-data2-v2.csv.zip
-
Transparent reporting form
- https://cdn.elifesciences.org/articles/62148/elife-62148-transrepform-v2.docx