Integrated transcriptomic and neuroimaging brain model decodes biological mechanisms in aging and Alzheimer’s disease
Figures
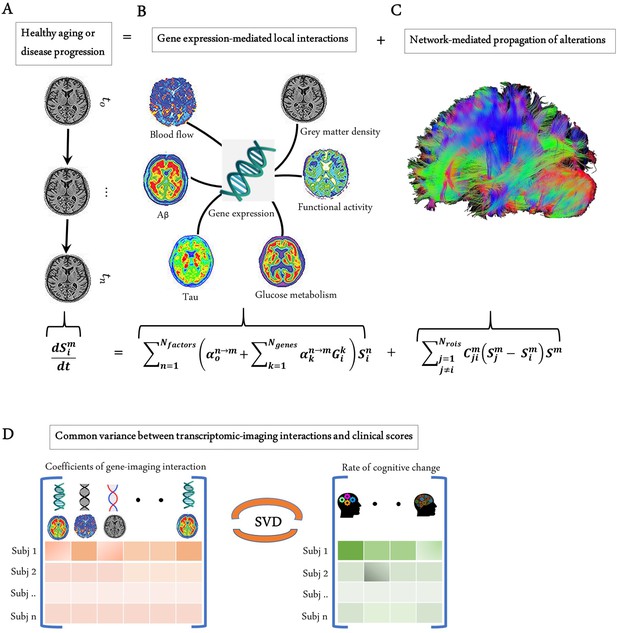
Modelling the gene-imaging interactions driving healthy aging and AD progression.
(A) The longitudinal alteration of macroscopic biological factors in healthy and diseased brain due to gene-imaging interactions and the propagation of the ensuing alterations across brain network. (B) Regional multifactorial interactions between six macroscopic biological factors/imaging modalities are modulated by local gene expression. (C) Causal multifactorial propagation network capturing the interregional spread of biological factor alterations through physical connections. (D) By applying a multivariate analysis through singular value decomposition (SVD), the maximum cross-correlation between age-related changes in cognitive/clinical evaluation and the magnitude of genetic modulation of imaging modalities are determined in a cohort of stable healthy subjects (for healthy aging), mild cognitive impairment (MCI) converters, and Alzheimer’s disease (AD) subjects (for AD progression). The key causal genes driving healthy aging and AD progression are identified through their absolute contributions to the explained common variance between the gene-imaging interactions and cognitive scores.
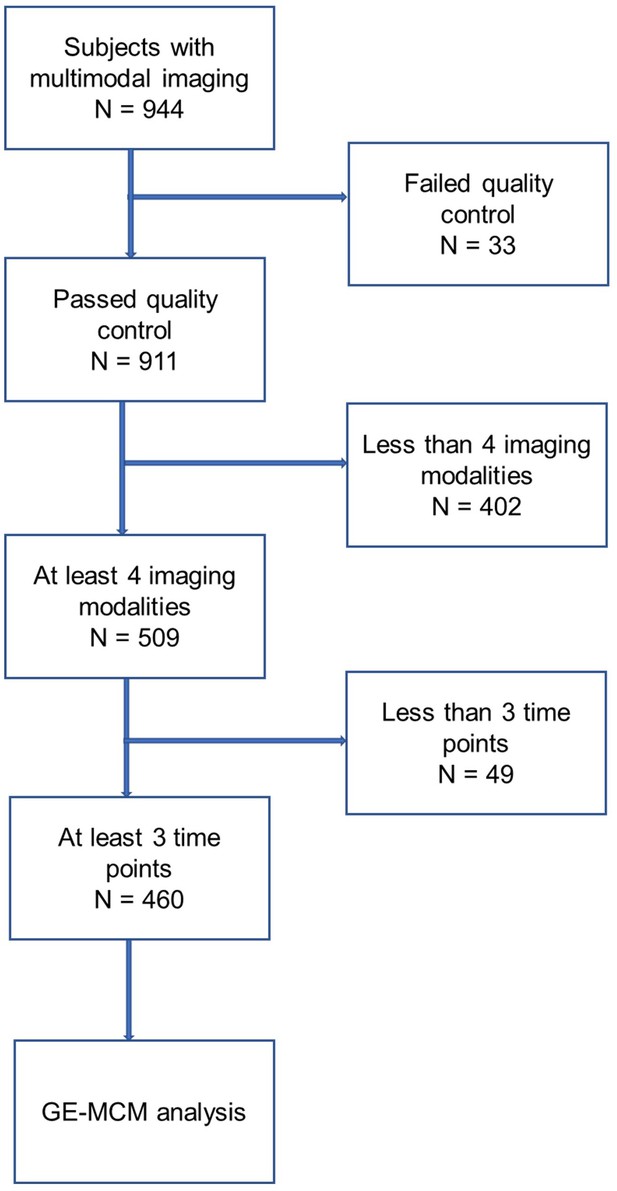
Subject selection.
A multivariate outlier identification was performed based on 944 subjects. From the 911 subjects that survived outlier detection, 509 subjects having at least four imaging modalities were chosen. Then, 460 subjects with at least three time points in any of the imaging modalities were selected. Next, for each of the 460 subjects, missing imaging modalities at each time point having actual individual data were automatically imputed using the trimmed scores regression with internal principal component analysis (PCA).
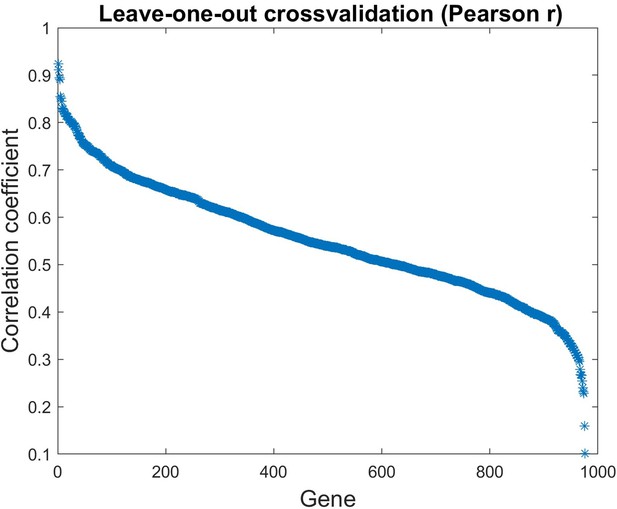
Correlation of predicted mRNA expression with actual mRNA expression across 976 genes.
Using the actual mRNA values of 3702 samples from Allen Human Brain Atlas (AHBA), Gaussian kernel regression was used to reproduce the mRNA intensities through a leave-one-out cross-validation, and the Pearson correlation coefficient between the actual and reproduced values was calculated for each gene.
-
Figure 1—figure supplement 2—source data 1
Source data for Figure 1—figure supplement 2.
- https://cdn.elifesciences.org/articles/62589/elife-62589-fig1-figsupp2-data1-v1.xlsx
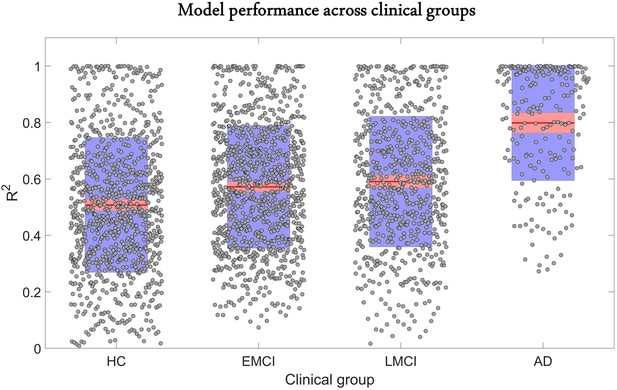
Reconstruction of individual multifactorial alteration patterns across all subjects in the AD continuum.
Plots are shown for the obtained across all six biological factors in the healthy control (HC) (n=151), early mild cognitive impairment (EMCI) (n=161), late mild cognitive impairment (LMCI) (n=113), and Alzheimer’s disease (AD) (n=35) cohorts. Points are laid over a 2.58 standard error of the mean (SEM) (99% confidence interval) in red and at 1 SD in blue. Notice that model performance improves with disease progression. We attribute this effect to the typical larger variation in longitudinal biological factor alterations with disease evolution, which provides the optimization algorithm with further biological information and results in a more accurate data fitting and parameter identification.
-
Figure 2—source data 1
Source data for Figure 2.
- https://cdn.elifesciences.org/articles/62589/elife-62589-fig2-data1-v1.xlsx
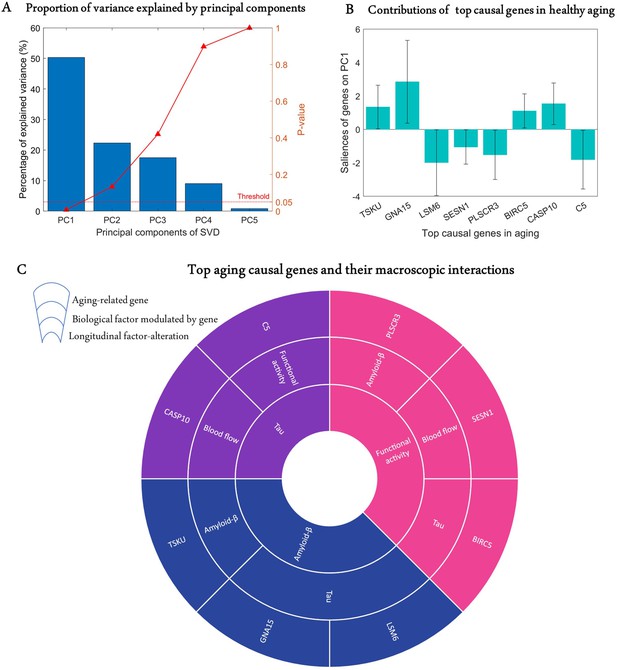
Identification of top genetic modulators of cognitive change in healthy aging.
(A) Common variance (and associated p-values) captured by the top five principal components (PCs) of the singular value decomposition (SVD) in explaining the rate of change of cognitive scores due to healthy aging. Only the first PC is significant (p<0.05). (B) Genetic contributions (and 99% CI) on the first PC, depicted only for the eight highly stable aging-related genes, the bootstrap ratios of which are above 2.58. (C) Top genetic determinants of multifactorial alterations in healthy aging. The innermost ring shows the longitudinal biological factor altered with aging, the middle ring displays the interacting biological factors driving the longitudinal alteration, and the outermost ring represents the causal genes modulating the interactions among biological factors (e.g., SESN1 directly modulates blood flow to drive age-related alteration in neuronal activity).
-
Figure 3—source data 1
Source data for Figure 3.
- https://cdn.elifesciences.org/articles/62589/elife-62589-fig3-data1-v1.xlsx
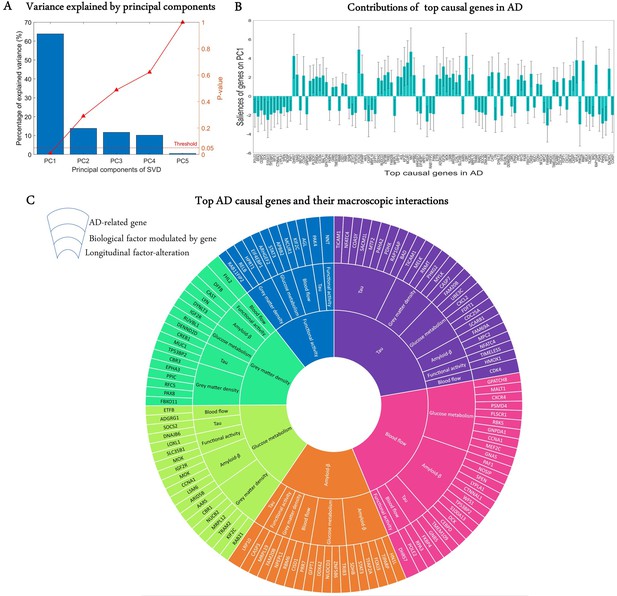
Uncovering the top genetic determinants of AD progression.
(A) The common variance captured by the principal components (PCs) of the singular value decomposition (SVD) in explaining how clinical evaluations change with Alzheimer’s disease (AD) evolution. P-values after 10,000 permutations are also shown. (B) Contributions of top AD causal genes (with 99% CI) to the first PC. Top causal genes are identified by selecting those genes whose bootstrap ratios of saliences are above 2.58. (C) Multifactorial interactions between the identified genes and imaging modalities. The innermost ring shows the longitudinal biological factor changes with AD, the middle ring displays the interacting biological factors driving the longitudinal alteration, and the outermost ring represents the causal genes modulating the interactions among biological factors. A gene directly influences how a biological factor interacts with other factors to cause a factorial alteration along the disease’s course.
-
Figure 4—source data 1
Source data for Figure 4.
- https://cdn.elifesciences.org/articles/62589/elife-62589-fig4-data1-v1.xlsx
Additional files
-
Source code 1
Codes for statistical analyses and plotting.
- https://cdn.elifesciences.org/articles/62589/elife-62589-code1-v1.zip
-
Supplementary file 1
Main demographic characteristics of the included ADNI subjects.
- https://cdn.elifesciences.org/articles/62589/elife-62589-supp1-v1.docx
-
Supplementary file 2
List of 976 genes used in this study.
- https://cdn.elifesciences.org/articles/62589/elife-62589-supp2-v1.xlsx
-
Supplementary file 3
Brain regions used in this study.
- https://cdn.elifesciences.org/articles/62589/elife-62589-supp3-v1.docx
-
Supplementary file 4
Distribution of stable gene-imaging interaction parameters in healthy aging and AD progression (99% CI).
- https://cdn.elifesciences.org/articles/62589/elife-62589-supp4-v1.xlsx
-
Supplementary file 5
Identified molecular pathways underlying AD progression.
- https://cdn.elifesciences.org/articles/62589/elife-62589-supp5-v1.docx
-
Transparent reporting form
- https://cdn.elifesciences.org/articles/62589/elife-62589-transrepform-v1.pdf