Science Forum: Sex differences and sex bias in human circadian and sleep physiology research
- Article
- Figures and data
- Abstract
- Introduction
- Sex differences in sleep and circadian physiology
- The sex data gap in sleep and circadian physiology
- Understanding the research landscape and identifying opportunities for change
- Recommendations: Guiding principles to close the sex data gap
- Methods
- Data availability
- References
- Decision letter
- Author response
- Article and author information
- Metrics
Abstract
Growing evidence shows that sex differences impact many facets of human biology. Here we review and discuss the impact of sex on human circadian and sleep physiology, and we uncover a data gap in the field investigating the non-visual effects of light in humans. A virtual workshop on the biomedical implications of sex differences in sleep and circadian physiology led to the following imperatives for future research: i) design research to be inclusive and accessible; ii) implement recruitment strategies that lead to a sex-balanced sample; iii) use data visualization to grasp the effect of sex; iv) implement statistical analyses that include sex as a factor and/or perform group analyses by sex, where possible; v) make participant-level data open and available to facilitate future meta-analytic efforts.
Introduction
Despite marked sex differences in many aspects of human physiology and behaviour, biomedical research continues to be disproportionately biased towards the male sex. For example, women made up only 25% of participants in landmark trials for congestive heart failure and 19.2% of participants for studies in antiretroviral treatment of HIV (Criado-Perez, 2019). Such a skewed evidence base leads to disparities in clinical and non-clinal research applications and it weakens the impact of science-based policies and translational outcomes.
This sex bias or ’sex data gap' – whereby data mainly come from male individuals – has recently received widespread attention (Criado-Perez, 2019), with policy advisers (Buitendijk and Maes, 2015), funders (Lee, 2018; Clayton and Collins, 2014) and publishers (Rippon et al., 2017; Docherty et al., 2019) pushing for better inclusivity in research regarding sex. Embracing these new practices should improve translational outcomes and scientific efficiency, but this will require a two-pronged tactic that both strengthens forces for change and weakens barriers in the field (Karp and Reavey, 2019). The problems that allow sex bias to emerge are multifaceted and closing the data gap will require solutions to be bespoke for each research community.
Here, we explore the sex data gap in the context of human circadian physiology and sleep research. The field focuses on the temporal organization of physiology and behaviour at a daily scale, including rest-activity cycles, diurnal changes in hormone levels and cognitive performance, as well as the non-visual effects of light. First, we describe primary findings on sex differences in circadian physiology and sleep. Next, we discuss the sex data gap in circadian and sleep research based on an analysis of over 150 papers on the non-visual effects of light, and finally we outline recommendations emerging from a virtual workshop on the biomedical implications of sex differences in sleep and circadian physiology (held in June 2020).
While we distinguish between gender identity (how individuals and groups perceive themselves e.g. men, women, non-binary) and sex (the biological attributes that distinguish organisms as female, male or intersex), we note that these terms are often used interchangeably and wrongly in the literature (Tannenbaum et al., 2019). Yet, in biology, sex describes differences in sexual characteristics that go beyond reproductive functions. Furthermore, we acknowledge that there is very little to no research about intersex individuals within circadian physiology and sleep research, constituting an important gap. Addressing this gap may contribute to better granularity and understanding of sex-differentiated biological mechanisms and responses. When reporting on results from the literature, we use the terms used by the researchers in these studies, as we are unable to know whether participants were asked about their sex or their gender.
Sex differences in sleep and circadian physiology
Human circadian and sleep physiology features well-established sex differences: for instance, circadian timing is phase-advanced (earlier) in female compared to male individuals, as seen in the core body temperature minimum and evening rise in melatonin (Boivin et al., 2016; Cain et al., 2010). Female individuals also have a shorter circadian period of the temperature and melatonin rhythms (Duffy et al., 2011), and larger amplitude of the melatonin rhythm (Cain et al., 2010). Furthermore, sex differences exist in chronotype, the circadian continuum of early (‘larks’) to late (‘owls’) diurnal preference (Chontong et al., 2016), such that more male individuals are late types than females (Fischer et al., 2017; Roenneberg et al., 2004; Fischer et al., 2016; Phillips et al., 2017). With regard to sleep, female individuals have an earlier timing of sleep, longer sleep duration and more slow-wave sleep (Roenneberg et al., 2004; Dijk et al., 1989).
More recently, sleep regularity – the day-to-day consistency in sleep timing and duration – has emerged as an important factor in health (Bei et al., 2016). Irregular sleep is associated with cardiovascular disease (Yoon et al., 2014), inflammation (Okun et al., 2011), metabolic disorders (Patel et al., 2014; Chontong et al., 2016; Spruyt et al., 2011), mental health conditions (Lemola et al., 2013; Vanderlind et al., 2014), and cognitive impairment (McBean and Montgomery-Downs, 2013). The data on sex differences are mixed with reports ranging from no sex differences (Kaufmann et al., 2016; Xu et al., 2018; Minors et al., 1998) to more irregular sleep in female (Mezick et al., 2009; Dillon et al., 2015; Lunsford-Avery et al., 2018) or in male individuals (Roane et al., 2015; Yetish et al., 2018). Chronotype may account for these inconsistencies as ‘owls’ tend to be more irregular sleepers (Duffy et al., 2011; Fischer et al., 2017). Indeed, in revisiting three published datasets (Fischer et al., 2016; Fischer et al., 2020; Keller et al., 2017), more male individuals were found to be irregular sleepers than females when both were a later chronotype.
Finally, while data remain sparse, the adverse health effects of sleep irregularity itself may also differ between the sexes. To the best of our knowledge, only one study examined this question (Roane et al., 2015), finding that variability in sleep duration was significantly associated with weight gain in male but not female students. Overall, despite the far-reaching health implications, sex differences in sleep and circadian physiology remain underresearched.
Impact of sex differences in sleep and circadian physiology in a non-clinical setting
Perhaps the most observable effect of sex differences in sleep and circadian physiology in a non-clinical setting is in shift work, a ubiquitous facet of modern society. Shift workers (approximately 50% of which are women) account for about a third of the workforce in North America and Europe, (Kervezee et al., 2018). Women have higher injury rates during night work than men, despite the injury rates between men and women being similar in day workers (Safe Work Australia, 2009). The physiological mechanisms underlying this difference remains unclear, partly due to a lack of research on the female circadian system. An exception is a recent study on sex differences in the effects of acute sleep deprivation on alertness (Smolensky et al., 2017). This work showed that women in the follicular phase of their menstrual cycle had more sleep loss-related alertness failure than men, whereas there were no differences between women in the luteal phase and men (Vidafar et al., 2018). This powerful influence of sex hormones and the menstrual cycle in female individuals highlight the pressing need to consider sex differences in biomedical research.
Impact of sex differences in sleep and circadian physiology in a clinical setting
Evidence is converging that sex differences in sleep and circadian phenotypes play a role in medical conditions and should therefore be considered in medical treatments and interventions. The emerging field of chronotherapeutics or chronotherapy (Shuboni-Mulligan et al., 2019; Smolensky et al., 2017; Dijk and Duffy, 2020) focuses on medical treatment approaches that incorporate a patient’s circadian phase, or at least the time of day, into the treatment regime. Here, we highlight a key therapeutic area, cancer treatment, in which sex-specific differences in underlying circadian mechanisms affect outcomes.
Sex and age profoundly impact chemotherapy efficacy and tolerability. Female patients are more susceptible than their male counterparts to the side effects of widely used anticancer drugs (Milano et al., 1992; Stein et al., 1995; Chansky et al., 2005; Cristina et al., 2018), and they can experience more frequent and severe toxicities from chemotherapy protocols due to sex differences in pharmacokinetics and pharmacodynamics (Gandhi et al., 2004). Across the 24 hour cycle, the molecular circadian clock rhythmically controls drug bioactivation, detoxification and transport while the circadian timing system as a whole regulates drug absorption, distribution, metabolism and excretion (Dallmann et al., 2016). In experimental models, this results in strong circadian changes in the tolerability and efficacy of over 50 anticancer medications, indicating that timing is a critical factor (Dallmann et al., 2016; Lévi et al., 2010).
For instance, a study examining colorectal cancer – the third cause of cancer deaths worldwide – showed that the intravenous delivery of the drug 5-FU leucovorin (5-FU-LV) at a constant rate resulted in circadian changes in drug concentration in plasma (Petit et al., 1988; Fustin et al., 2012). Most importantly, female patients had reduced 24 hour mean and circadian amplitude of the 5-FU body clearance compared to their male counterparts (Bressolle et al., 1999). Furthermore, peak delivery at 1pm or 4pm for oxaliplatin (another anticancer drug) and at 1am or 4am for 5-FU-LV proved to be least toxic by up to six-fold in male patients, whilst optimal timing was located six hours later in female patients (Lévi et al., 2007). Thus, optimal drug timing and optimal drug doses can differ according to sex (Spitschan et al., 2020). While the underlying mechanisms appear to involve sex differences in molecular clock function, their links with chrono-pharmacological determinants prompt further investigation.
The sex data gap in sleep and circadian physiology
The sex data gap exists both in in vivo (Curtis et al., 2018) and in vitro research (National Institute for Health and Care Excellence, 2020) and is apparent even in diseases that predominantly affect women (Patel et al., 2014). Critically, the sex gap is not just restricted to inclusion at the experimental design stage: researchers frequently ignore sex as a factor in the analysis, even when males and females are included in the study (Karp and Reavey, 2019).
Apart from vision, light plays a critical role in regulating physiology and behaviour via its influence on the circadian system. These effects are mediated by a multi-component photoreceptor system consisting of rods, cones and intrinsically photosensitive retinal ganglion cells in the eye that transmit information to the circadian clock via the retinohypothalamic tract.
To ascertain whether there is a sex data gap in sleep and circadian physiology research, we focused on the non-visual effects of light on human physiology and behaviour – including how it suppresses melatonin and shifts the circadian system. This topical research area has applicability in lighting standards, regulations and guidelines (CIE, 2019; International WELL Building Institute, 2020), and various efforts are underway to incorporate scientific data from this research area into building recommendations. This highlights a pressing need to understand sex bias in this field.
A preliminary literature search identified 545 papers, which were evaluated against a list of exclusion criteria (see Methods for full details), yielding a total of 180 articles. In this specific analysis, we focused on the reported sex of participants, although in many instances the terms sex and gender were used interchangeably. Each paper was then reviewed by a single reviewer to determine if participant sex and numbers were reported, and where possible, the proportion of female participants was calculated. Of the papers assessed, 14 (7.77%) did not give sufficient information on the sex breakdown of participants for this to be determined. In the remaining 166 articles, females comprised an average of 33.9% of the sample. Seven papers reported studying exclusively female participants, while 56 papers reported studying only males. Figure 1 shows the proportion of female study participants as a function of the publication year, calculated from the per-sex participant sample sizes. We conducted binomial tests to investigate the possibility of deviations from a balanced distribution of sexes, finding a large proportion of studies using only male volunteers. Interestingly, for later years, there were fewer female-only studies (but also fewer studies in total). While this may represent a shift towards more sex-inclusive recruitment, it also means that large parts of the cited literature are based on imbalanced participant samples.
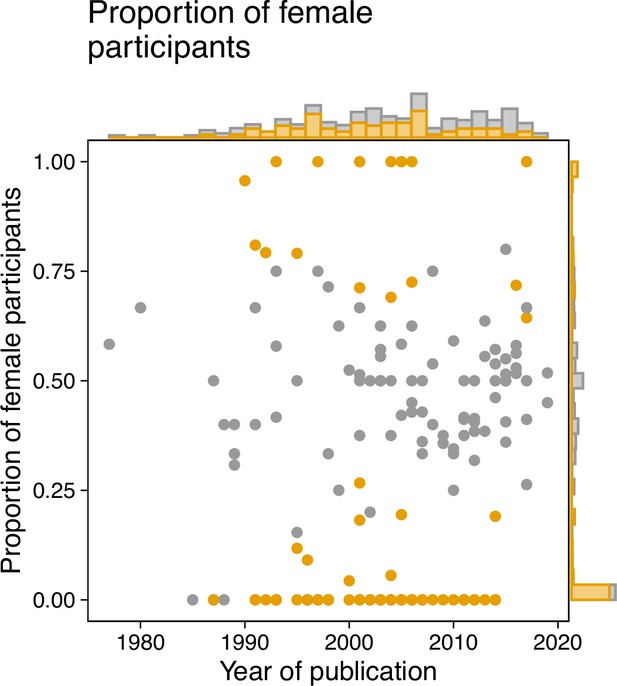
A review of the literature on the non-visual effects of light reveals a sex bias.
We analyzed a sample of the existing literature on the non-visual effects of light as a starting point for understanding the sex bias in the field. The sample included a total of 180 articles, and the breakdown of participant sex was then obtained in 166 articles. Binomial tests were conducted to evaluate the possibility that deviations from an even 50:50 sex distribution were attributable to chance alone. We implemented the Benjamini-Hochberg correction for multiple comparisons to control false-discovery rate (FDR). The proportion of female volunteers in each paper (represented by a dot) was plotted against the year of publication. Samples for which the proportion of female patients deviated significantly from 0.5 (P ≤ 0.05) were determined to be biased and colour-coded as orange. The marginal histograms show the numbers of papers irrespective of publication year (histogram on the right y axis), or irrespective of proportion (histogram on top x axis). Methods for paper selection are included in Methods.
-
Figure 1—source code 1
R code to produce Figure 1.
- https://cdn.elifesciences.org/articles/65419/elife-65419-fig1-code1-v2.zip
-
Figure 1—source data 1
Excel spreadsheet containing the data underlying Figure 1.
- https://cdn.elifesciences.org/articles/65419/elife-65419-fig1-data1-v2.xlsx
Next, we examined studies that exclusively involved male or female participants (N = 63). Of these, only eleven (17.5%) provided text to justify this sample choice. For studies with only male participants the justifications included female physiology being subject to confounding factors such as menstruation (N = 3), research into the other sex being unnecessary due to previously published observations (n = 3), the study involving a sex-specific condition (N = 2), not being able to recruit females with a specific genetic polymorphism (N = 1), the study being a case study (N = 1), and the study being conducted in a location (field station) with only male staff (N = 1). We found some evidence that the number of females increased over time, with publication year and proportion of female participants being correlated (r(164)=0.17, P = 0.02895). Interestingly, the total sample size correlates with the fraction of female participants in a given study (r(164)=0.3, P = 0.00008; Spearman’s correlation): larger studies seem to recruit more balanced samples.
In summary, we find a sex data gap in the literature on the non-visual effects of light, which needs to be considered in current efforts to translate research findings in the 'real world'.
Misconceptions underlying sex bias
One of the important aspects of an experimental design is to simplify a complex world to generate a testing space where cause and effect can be isolated. This approach is necessary to generate ‘doable problems’, allowing researchers to better understand the mechanisms that underlie a biologically intricate world (Gompers, 2018). In animal research, this simplification has led to studying one sex and strain in one batch, an approach supported by an interpretation of the ‘Reduce’ element of the 3R ethical framework. Historically, this has been conceived as a requirement for minimizing the number of animals in a single experiment, thus encouraging researchers to generate a narrow testing space before extrapolating and generalizing the results. Male animals were consistently selected due to the belief that the sex hormone cycle in females would lead to greater variability in the data, which would then require a larger number of female animals to achieve the same statistical power (Karp and Reavey, 2019). A recent meta-analysis looking at 9,932 traits found that the variability seen in female mice was not greater than for male mice – and in some cases was less – yet the legacy remains (Prendergast et al., 2014).
A related misconception is that studying both males and females requires the sample size to be doubled. Indeed, the analysis is not conducted independently for each sex; rather a regression analysis is used to explore the variation in the outcome variable of interest after accounting for effect of sex. Another benefit is that this approach also includes a statistical test for whether the treatment effect depends on sex. As regression analysis does not pool the data, the variance introduced by sex is accounted for, and the sensitivity for a treatment effect is minimally impacted by the inclusion of two sexes. The statistical test for the main treatment effect reveals the average treatment effect across the two sexes, and the interaction term shows how the treatment effect differs for the two sexes. The power for the main effect will be impacted when the treatment effect goes in the opposite direction for the sexes (crossed effect) but then the power to detect an interaction will increase. Biologically, crossed effects are rare, as shown in a large study assessing the prevalence of sexual dimorphism (Karp et al., 2017). In these situations, the treatment effect must be estimated for each sex individually. This potential situation may appear concerning to some, but it simply provides more evidence for the need to study both sexes to avoid misunderstanding biology.
Notably, the ongoing misconceptions about including female individuals in research have become part of the implicit scientific practice, and they are passed on to future generations of researchers. To curtail this, we point to the National Institutes of Health (NIH) guidelines which stipulate that male and female sexes should be included. Furthermore, rather than automatically powering to test for an interaction, we suggest that the average treatment effect represents both sexes, and a sex-disaggregated analysis would reveal possible large differences.
Sometimes, researchers propose studying one sex at the time, but it is important to collect data on both male and female individuals simultaneously to test how the treatment interacts with sex. If data is collected independently for the two sexes, it becomes impossible to determine whether differences in estimate emerge due to sample variation or because the effect depends on sex.
A common pushback is that other sources of variation, such as age, should be considered: why should sex be the variable that is prioritized? Conducting an experiment means simplifying a complex biological world that features many sources of variation into a testing space, before generalizing the findings to reach broader conclusions. In biomedical research, the target population will be, on average, 50% male and 50% female, and it is becoming clear that variations between male and female physiology extend beyond hormonal differences. Therefore, as a rule, sex should be the first variable to be included to significantly increase generalizability – except, as discussed in the NIH guidelines, for cases such as the study of sex-specific conditions or phenomena.
Understanding the research landscape and identifying opportunities for change
In a three-part virtual workshop held in June 2020, the authors of this paper explored practices, barriers, and challenges in designing and executing inclusive research in circadian physiology and sleep research. All materials from the workshop, including the recordings and the programme, are available under the CC-BY license (Fischer and Vidafar, 2020; Karp and Ahluwalia, 2020; White and Lévi, 2020).
The workshop series comprised three 90-minute sessions held a week apart and included invited talks as well as interactive sessions. The workshop was advertised through a range of channels, including Twitter, the UK Clock Club listserv, and the personal networks of the organisers and speakers. A total of 275 participants registered for the entire workshop. Across the three workshops, between 38 and 94 attendees participated in the interactive sessions, with approximately four out of five participants being researchers (82 out of 94 in Workshop 1, 47 out of 60 in Workshop 2 and 31 out of 38 in Workshop 3).
We used the web platform Mentimeter to implement polling amongst participants as well as open-ended questions. Prior to participating in the interactive sessions, attendees were informed that their responses would be used for write-up and published as a peer-reviewed article. Attendees were free to not participate in the interactive sessions. No personal data were collected as part of the interactive Mentimeter sessions. We combined yes/no, ranking and open-ended questions throughout the interactive sessions to vary the response modality. The results discussed below were selected from the results, which can be viewed in full on the Open Science Framework page. The number of responses to individual questions varied somewhat due to dropout during the interactive session as well as a time-limited response window; the total number of responses in the participatory parts are given on the bottom right-hand corner of the Materials document.
Workshop 1: Understanding differences
In the first workshop, we explored sex as a variable in research. In an interactive polling segment following this workshop, only 58% of respondents (out of 100) indicated previously analyzing data in a sex-disaggregated fashion. However, 88.1% (out of 101) agreed that sex could be a variable in their research, showing the large scope for sex-disaggregated analyses. Of note, sex was identified as just one of many characteristics contributing to individual differences in research results, alongside age chronotype, mental health status, genetics, body mass index and prior light exposure. When asked for the most pressing research questions involving individual differences, the answers ranged from developmental and lifespan factors to more fundamental research questions with no obvious individual difference angle. The video recording for Workshop 1 is available here, and the materials related to the participatory part are available here.
Workshop 2: Understanding impact
The second workshop focused on understanding the real-world impact of the participants’ research. In the interactive polling segment following this workshop, participants indicated that their research could mostly influence precision and personalized medicine, occupational timing and shift/rota planning, and guidelines for an indoor ‘circadian’ lighting.
When asked to identify the biggest barriers to addressing sex bias in research, research money or funding and time were the most mentioned factors, followed by guidelines and policies. This indicates a scope for funding agencies to specifically address researchers’ need for funding, as well as an opportunity for institutions, funders, professional bodies, learned societies and journals to develop clear guidance (see Box 1 for an example of a journal implementing a specific policy; and Figure 2). The video recording for Workshop 2 is available here, and the materials related to the participatory part are here.
Example journal policy to addressing sex bias.
Amrita Ahluwalia, Editor-in-Chief of British Journal of Pharmacology (BJP)
In 2018, the British Journal of Pharmacology identified the issue of sex bias in pharmacological research as a critical area for attention with respect to the work published in the journal. This came following an internal survey of our published work coupled with recognition of the activities and actions of the National Institutes of Health, in the US, raising the profile of this important issue (National Institutes of Health, 2020). We discovered that in addition to a prevailing reluctance to use female individuals in experimental research, both in vivo and in vitro, there was the unsurprising omission of detail regarding the sex of the source for experimental work involving primary cell culture (Docherty et al., 2019).
To address these issues, we introduced a number of initiatives, including: (1) publishing a themed issue in BJP containing a number of reviews and original articles focused on sex differences in pharmacology; (2) bringing together a collection of articles from all of the journals owned by the BPS in a virtual issue focused on sex; and, most importantly, (3) the elaboration and publication of guidelines for original research published in BJP. The aim of this guidance is to ensure that sex as an experimental variable is no longer ignored in articles published in BJP, but also to provide researchers with the tools to adapt their experimental design to accommodate for sex.
A key aspiration, of course, is that both male and female subjects are used as a default design in the experimental work detailed in all manuscripts submitted to the journal, but we do not mandate this at present. Our hope is that by insisting that these issues are considered within any submitted work, we raise their profile, organically leading to change. Of course, it is the responsibility of those who work with the journal to ensure that change does indeed occur. Indeed, there are many examples where such an advisory approach with other important issues related to transparency and reproducibility appear to have failed (Leung et al., 2018; Avey et al., 2016). Yet our experience in such approaches at BJP – for instance, with our guidelines on design and analysis (Curtis et al., 2018) – gives us strong hope that change will take place. We plan to conduct surveys of published material annually to assess this, and we will publish the outcome of these audits.
Workshop 3: Understanding change
The third workshop explored factors that would facilitate change in research. In the interactive polling segment, when asked to rank sources for guidance on sex-difference analysis, the participants first mentioned research institutes and universities, then societies and professional bodies and finally funders and publishers.
In further exploring the role of funders, the top three priorities for participants were: (1) provision of training and guidance to incorporate sex and gender analysis; (2) allocation of funding within regular grant mechanisms ringfenced for sex and gender analysis; and (3) simply more allocation of funds in regular research grants. Additionally, collaboratively developed guides, research toolkits, training programmes from societies and professional bodies were also indicated as facilitators of change.
When asked what researchers could personally do, three actionable items emerged: (1) inclusion of sex and gender analysis as a central step in research; (2) learning from peers and with examples; and (3) upskilling in the requisite statistical techniques. The video recording for Workshop three is available here, and the materials related to the participatory part are available here.
Recommendations: Guiding principles to close the sex data gap
Based on the workshop content and discussions, we propose the following guiding principles to address the sex data gap in biomedical research, and to build an evidence base which is better inclusive of sex and gender. The central tenet includes sex and gender analysis as an essential component of research design. The specific recommendations are:
Design research to be inclusive and accessible. In many cases, research is designed exclusively by researchers who may not necessarily have sufficient expertise on how to make their study inclusive and accessible. An important step is reaching clarity in recording and reporting participant sex and gender. As an example, one research team reporting the sex of participants may use participant-derived responses on a questionnaire or intake form, and another group may use the sex assigned at birth, based, for instance, on an ID card. While these could give congruent answers, they represent different types of information. Wider engagement with definitions of sex and gender and questions surrounding this topic within a research group or researcher community could lay the groundwork for making research more inclusive and accessible. As a formalised way to ensure inclusivity, we also suggest that research participants be integrated in the research planning process through Patient and Public Involvement (see Box 2) or similar mechanisms.
Implement recruitment strategies that lead to a sex-balanced sample. This includes wide advertisement of research studies, and tailoring recruitment strategies by engaging with patients, participants and the general public, for example through Patient and Public Involvement mechanisms (see Box 2). Given fixed resources, recruiting a sex-balanced sample does not simply mean doubling the sampling size, but merely recruiting a sample with 50% female and 50% male participants. A balanced design is recommended to ensure the resulting statistical analysis is robust and that the variance can be decomposed to the factors of interest without confounding these (Collins et al., 2009). While exceptions to this principle may arise from sex-specific research questions, as a general guiding principle there is little to argue against. Furthermore, this will allow sex to be included as a factor in the analysis without compromising sensitivity to a generalizable main effect.
Use data visualization to grasp the effect of sex. An informal visualization in the early stages of analyses can be used to ascertain sex difference trends, which can then be followed up with more rigorous statistical testing.
Implement statistical analyses that include sex as a factor and/or perform group analyses by sex, where possible. Sex can be included as a factor or a covariate in analyses, or an alternative strategy can be to perform a group analysis by sex. Both require a good understanding of effect sizes and statistical power. Researchers should seek to upskill in statistics to develop advanced analytic strategies.
Make participant-level data open and available to facilitate future meta-analytic efforts. This step requires data to be available, which many journals now mandate. The large, combined sample size afforded by the wide availability of data can enable a sex-related effect to be more readily detectable. We also suggest that researchers should include tables reporting the primary data and participant meta-data as supplementary information in articles. A recent analysis of open science practices in circadian rhythms and sleep research journals (Spitschan et al., 2020) has indicated an opportunity to mandate data sharing in journal policies. Journal policies requiring participant-level data sharing could facilitate future analyses incorporating sex.
Patient and Public Involvement as a vehicle to make research more inclusive.
Patient and Public Involvement (PPI) (National Institute for Health and Care Excellence, 2020; Arthritis Research UK, 2017; Bagley et al., 2016) is defined as research carried out ‘with’ or ‘by’ patients, those who have experience of a condition, and the broader public in general. PPI is a term that is largely used in the UK research landscape, but similar initiatives may exist in different countries. PPI differs markedly from engagement and participation; this refers to various types of interactions with people with a condition (such as providing information and knowledge in research) as well as surveying what people understand about a particular condition regardless of whether they experience it, or exploring what should be prioritized in basic or clinical research on that condition. Involvement, on the other hand, implies a more active collaboration between researchers, and the target group – and in some cases the general public – that helps shape the design of a research project. At different levels, all these interactions provide opportunities for dialogue and bring research to those directly impacted by conditions and the public. This, in turn, helps increase diversity – including, but not limited to, making research more inclusive with respect to sex and gender.
Engaging with the general public and with patients is now often asked by charities and research funding organizations but should be considered beyond being a box-ticking exercise. PPI will very likely impact the design of research projects by identifying what is vital to patients and society, and why. In turn, this will help to identify gaps in our understanding of the disease or condition in question thereby increasing research quality. This can help prioritize research areas and lead to research that is better aligned with patient and public interests. For example, the James Lind Alliance Priority Setting Partnerships is a non-profit initiative bringing patients, carers and clinicians together to identify and prioritise unresolved questions or evidence uncertainties they consider important. In this way, research funders become aware of what matters most to the people who use their research in their everyday lives. PPI will also help the target group to better understand research, and give an often unique opportunity for researchers – especially discovery scientists – to understand patients’ reality and perspective.
While none of these actions will suffice on their own, each will contribute to closing the sex data gap. Of course, the research ecosystem not only includes individual researchers but also institutions of varying sizes. We present multiple actions that can be adopted by institutions, funders, as well as professional bodies, learned societies, journals in Figure 2. These actions were developed from an interactive segment of Workshop 3.
Methods
To implement a breadth-first search for identifying relevant papers, we employed a pragmatic hybrid strategy, identifying relevant articles through three main sources, as listed in Table 1. We conducted a citation search of three key recent reviews (Brown, 2020; Souman et al., 2018; Lok et al., 2018) on the acute effects of light, producing a total of 88 papers of which 83 were included in the present analysis. We carried out a search for papers specifically discussing the melatonin-suppressive effects of light in SCOPUS (search carried out on 22 October 2019) through the search term “TITLE-ABS-KEY ((light AND melatonin AND suppress*)) AND (LIMIT-TO (DOCTYPE, "ar")) AND (LIMIT-TO (LANGUAGE, "English"))” (search carried out on 22 October 2019). Limiting the analysis to papers with a minimum of 30 citations, we identified 359 further papers (94 of which were included). Finally, relevant systematic reviews were identified in the Cochrane Library through the search terms "(light AND circadian OR sleep OR alertness)", generating 24 results with six relevant for the present analysis. A citation search was again conducted, generating a further 98 papers (of which three were included). Overall, a total of 545 papers were identified and analyzed, as shown in Table 1.
Articles included in the meta-analysis.
Database | Search strategy | Source paper | Articles considered | Articles included |
---|---|---|---|---|
– | – | Brown, 2020 | 19 | 18 |
– | – | Lok et al., 2018 | 20 | 20 |
– | – | Souman et al., 2018 | 49 | 45 |
SCOPUS | Citation count | - | 359 | 94 |
Cochrane | (light AND circadian OR sleep OR alertness)” | Pachito et al., 2018 | 5 | 0 |
Forbes et al., 2014 | 13 | 0 | ||
Montgomery and Dennis, 2002 | 0 | 0 | ||
Tuunainen et al., 2004 | 49 | 3 | ||
Slanger et al., 2016 | 21 | 0 | ||
Dennis and Dowswell, 2013 | 10 | 0 | ||
545 | 180 |
Inclusion and exclusion criteria
Papers were excluded where the following exclusion criteria applied, leaving a total of 180 papers for the present analysis:
Studies that do not assess the acute effect of light: including those looking at longitudinal exposures or habits rather than controlled light exposure within a specified time frame, e.g. cohort and case-control studies were excluded;
Studies in which the primary outcome measure did not relate to circadian physiology (e.g. the role of light exposure in treating affective disorders);
Studies assessing the effects of interventions other than light exposure, e.g. sleep deprivation or magnetic field exposure. In papers involving multiple studies, only those assessing the acute effects of light were included, with other studies excluded;
Studies for which the PDF of the paper could not be obtained, or could not be obtained in English;
Studies primarily focusing on non-human animals;
Review papers, opinion pieces or commentaries not including any primary data;
Studies not based on measurements taken from human participants, e.g. in vitro studies or mathematical models. Measurements of human materials such as blood or retinal cells were considered to be from human participants if the intervention (light exposure) was carried out before the material was isolated from participants, but they were excluded if measurements were taken after the materials were obtained;
Research involving participants under the age of 18;
Studies in which variables were not manipulated (i.e., naturalistic or observational studies);
Field studies, in which variables were manipulated outside of a laboratory setting.
Papers were not excluded based on participant disease status or outcome measure. No upper limit was set for participant age. In coding the articles, we did not make a distinction between sex and gender, as these are conflated in the literature.
Data availability
All data generated or analysed during this study are included in the manuscript and supporting files. Source data and source code files have been provided for Figure 1.
References
-
Circadian rhythm of 5-fluorouracil population pharmacokinetics in patients with metastatic colorectal cancerCancer Chemotherapy and Pharmacology 44:295–302.https://doi.org/10.1007/s002800050980
-
Melanopic illuminance defines the magnitude of human circadian light responses under a wide range of conditionsJournal of Pineal Research 69:e12655.https://doi.org/10.1111/jpi.12655
-
ConferenceGendered research and innovation: integrating sex and gender analysis into the research processLeague of European Research Universities.
-
Sex differences in phase angle of entrainment and melatonin amplitude in humansJournal of Biological Rhythms 25:288–296.https://doi.org/10.1177/0748730410374943
-
Higher sleep variability is associated with poorer glycaemic control in patients with type 1 diabetesJournal of Sleep Research 25:438–444.https://doi.org/10.1111/jsr.12393
-
Experimental design and analysis and their reporting II: updated and simplified guidance for authors and peer reviewersBritish Journal of Pharmacology 175:987–993.https://doi.org/10.1111/bph.14153
-
Dosing-time makes the poison: circadian regulation and pharmacotherapyTrends in Molecular Medicine 22:430–445.https://doi.org/10.1016/j.molmed.2016.03.004
-
Interventions (other than pharmacological, psychosocial or psychological) for treating antenatal depressionThe Cochrane Database of Systematic Reviews 2013:CD006795.https://doi.org/10.1002/14651858.CD006795.pub3
-
Novel approaches for assessing circadian rhythmicity in humans: a reviewJournal of Biological Rhythms 35:421–438.https://doi.org/10.1177/0748730420940483
-
Variability in self-reported normal sleep across the adult age spanThe Journals of Gerontology. Series B, Psychological Sciences and Social Sciences 70:46–56.https://doi.org/10.1093/geronb/gbu035
-
Sex: a change in our guidelines to authors to ensure that this is no longer an ignored experimental variableBritish Journal of Pharmacology 176:4081–4086.https://doi.org/10.1111/bph.14761
-
A novel method to visualise and quantify circadian misalignmentScientific Reports 6:38601.https://doi.org/10.1038/srep38601
-
WebsiteSex differences in circadian rhythms and sleep – Understanding differenceAccessed June 15, 2020.
-
Light therapy for improving cognition, activities of daily living, sleep, challenging behaviour, and psychiatric disturbances in dementiaThe Cochrane Database of Systematic Reviews 2014:CD003946.https://doi.org/10.1002/14651858.CD003946.pub4
-
Sex differences in pharmacokinetics and pharmacodynamicsAnnual Review of Pharmacology and Toxicology 44:499–523.https://doi.org/10.1146/annurev.pharmtox.44.101802.121453
-
Prevalence of sexual dimorphism in mammalian phenotypic traitsNature Communications 8:15475.https://doi.org/10.1038/ncomms15475
-
Sex bias in preclinical research and an exploration of how to change the status quoBritish Journal of Pharmacology 176:4107–4118.https://doi.org/10.1111/bph.14539
-
WebsiteSex differences in circadian rhythms and sleep – Understanding changeAccessed June 29, 2020.
-
Clinical significance of mobile health assessed sleep duration and variability in bipolar disorderJournal of Psychiatric Research 81:152–159.https://doi.org/10.1016/j.jpsychires.2016.07.008
-
Not later, but longer: sleep, chronotype and light exposure in adolescents with remitted depression compared to healthy controlsEuropean Child & Adolescent Psychiatry 26:1233–1244.https://doi.org/10.1007/s00787-017-0977-z
-
Impact of shift work on the circadian timing system and health in womenSleep Medicine Clinics 13:295–306.https://doi.org/10.1016/j.jsmc.2018.04.003
-
Implications of circadian clocks for the rhythmic delivery of cancer therapeuticsAdvanced Drug Delivery Reviews 59:1015–1035.https://doi.org/10.1016/j.addr.2006.11.001
-
Circadian timing in cancer treatmentsAnnual Review of Pharmacology and Toxicology 50:377–421.https://doi.org/10.1146/annurev.pharmtox.48.113006.094626
-
Light, alertness, and alerting effects of white light: a literature overviewJournal of Biological Rhythms 33:589–601.https://doi.org/10.1177/0748730418796443
-
Timing and variability of postpartum sleep in relation to daytime performancePhysiology & Behavior 122:134–139.https://doi.org/10.1016/j.physbeh.2013.09.003
-
Intra-individual variability in sleep duration and fragmentation: associations with stressPsychoneuroendocrinology 34:1346–1354.https://doi.org/10.1016/j.psyneuen.2009.04.005
-
Influence of sex and age on fluorouracil clearanceJournal of Clinical Oncology 10:1171–1175.https://doi.org/10.1200/JCO.1992.10.7.1171
-
Bright light therapy for sleep problems in adults aged 60+The Cochrane Database of Systematic Reviews 2002:CD003403.https://doi.org/10.1002/14651858.CD003403
-
Workplace lighting for improving alertness and mood in daytime workersThe Cochrane Database of Systematic Reviews 3:CD012243.https://doi.org/10.1002/14651858.CD012243.pub2
-
The association between sleep patterns and obesity in older adultsInternational Journal of Obesity 38:1159–1164.https://doi.org/10.1038/ijo.2014.13
-
Circadian rhythm-varying plasma concentration of 5-fluorouracil during a five-day continuous venous infusion at a constant rate in cancer patientsCancer Research 48:1676–1679.
-
Female mice liberated for inclusion in neuroscience and biomedical researchNeuroscience and Biobehavioral Reviews 40:1–5.https://doi.org/10.1016/j.neubiorev.2014.01.001
-
Journal of neuroscience research policy on addressing sex as a biological variable: comments, clarifications, and elaborationsJournal of Neuroscience Research 95:1357–1359.https://doi.org/10.1002/jnr.24045
-
What role does sleep play in weight gain in the first semester of university?Behavioral Sleep Medicine 13:491–505.https://doi.org/10.1080/15402002.2014.940109
-
A marker for the end of adolescenceCurrent Biology 14:R1038–R1039.https://doi.org/10.1016/j.cub.2004.11.039
-
Radiation chronotherapy-clinical impact of treatment time-of-day: a systematic reviewJournal of Neuro-Oncology 145:415–427.https://doi.org/10.1007/s11060-019-03332-7
-
Person-directed, non-pharmacological interventions for sleepiness at work and sleep disturbances caused by shift workThe Cochrane Database of Systematic Reviews 2016:CD010641.https://doi.org/10.1002/14651858.CD010641.pub2
-
Acute alerting effects of light: A systematic literature reviewBehavioural Brain Research 337:228–239.https://doi.org/10.1016/j.bbr.2017.09.016
-
Light therapy for non-seasonal depressionThe Cochrane Database of Systematic Reviews 2004:CD004050.https://doi.org/10.1002/14651858.CD004050.pub2
-
WebsiteSex differences in circadian rhythms and sleep – Understanding impactAccessed June 22, 2020.
-
Habitual sleep duration and sleep duration variation are independently associated with body mass indexInternational Journal of Obesity 42:794–800.https://doi.org/10.1038/ijo.2017.223
-
Sleep variability and nighttime activity among Tsimane forager-horticulturalistsAmerican Journal of Physical Anthropology 166:590–600.https://doi.org/10.1002/ajpa.23454
Decision letter
-
Elsa LoisselReviewing Editor; eLife, United Kingdom
-
Peter RodgersSenior Editor; eLife, United Kingdom
In the interests of transparency, eLife publishes the most substantive revision requests and the accompanying author responses.
Decision letter after peer review:
Thank you for submitting your article "Sex differences and sex bias in circadian rhythms and sleep research" to eLife for consideration as a Feature Article. Your article has been reviewed by three peer reviewers, and the evaluation has been overseen by a Reviewing Editor and Peter Rodgers as the Senior Editor.
The reviewers and editors have discussed the reviews and we have drafted this decision letter to help you prepare a revised submission.
Please also note that eLife does not permit figures in supplementary materials: instead we allow primary figures to have figure supplements (tables, however, can be supplementary materials).
After revision, please note that the eLife Features Editor may also contact you separately about some editorial issues that you will need to address.
Summary:
This article highlights the sex-bias present in chronobiology and sleep medicine research, and it provides recommendations and guidance for sex- and gender-inclusive research practices and policies. To do so, it relies on insight from a workshop conducted to evaluate sex differences in circadian and sleep research. Overall, the authors have provided robust examples from a growing body of evidence which suggests that biological sex influences the human circadian clock and that these sex differences may play a key role in health and disease. However, a number of points need to be addressed to make the article suitable for publication.
Essential revisions:
1. Please shorten the introduction, and reorganize the first two sections "Sex differences are ubiquitous in circadian rhythms and sleep" and "Sex differences in circadian rhythms can impact therapeutic interventions" into one cohesive introduction section which lays the ground for the literature analysis and the workshop discussion. In doing so, please make sure to streamline the text to ensure the reader feels it was written cohesively rather than by different people.
2. Lines 61-198 have a large overlap of topic matter with lines 200-266: please try to reduce this repetition. (For instance, the sub-section "What is the sex data gap?" repeats the conclusions from the previous section).
3. Line 73: Please discuss this point further, as "blockers" are not the only people who may resist change – they are probably a tiny minority of people. Far more people resisting change are probably people who don't know enough about how or why.
4. Line 217 onward: Please discuss why there appears to have been a thorough review of studies involving light impact on human circadian rhythms, but not of other types of studies that are only mentioned briefly (e.g., circadian period or phase)
5. Line 227 onwards: Please clarify whether there was a relationship between the proportion of female participants and the sample size of the study
6. Line 268 onwards: Please provide further information about the workshop participants and how they contributed to the outcomes of the paper.
– For instance, please clarify the methods used to obtain participant reflections/responses (i.e. Did you provide participants with a certain set of questions or surveys to obtain the information described in Workshops 1 and 2? Alternatively, did this information come from discussions which were transcribed and coded?)
– Please indicate whether the participants of the workshop gave consent for their responses/comments to be used in this format? If not, please discuss whether there is a need for a retrospective-IRB approval.
– Please report how many individuals attended each workshop
– For each workshop section, please give all answers, not a selection of them. You can group into categories or ones with similar wording. The number of each question is not needed since there was limited time to respond and participants may not have been able to include all possibilities (so the number of each response does not necessarily reflect the importance of said response).
7. Line 282: Please further discuss the statement that a common misconception in research is that the sample size must be doubled to include both sexes. The authors note that this is a flawed assumption because treatment effects are reported from the entire group. While this is certainly true, the authors should also acknowledge that the issue may be more complex than described, especially because sex differences exist. In the first half of this manuscript, the authors describe important differences that have been identified between sexes on a variety of outcomes (shiftwork tolerance, sleep differences, phase differences, etc.). Doesn't this suggest that pooling of males and females a bad idea, particularly for time-varying differences? For example, on lines 188-192, the authors describe the results of several important studies examining oxaliplatin and irinotecan in male and female patients. The authors note that the optimal timing for females and males were at different times for these treatments. If these reports would have pooled the data for males and females wouldn't that have attenuated the results or obscured the researchers' ability to observe a time-of-day effect at all? In this case, it seems pretty important that the analyses were stratified by sex. If a researcher wishes to examine outcomes by sex, as seems pretty important given the thesis of this manuscript, then one should be powered to conduct separate analyses (Diester CM, Banks ML, Neigh GN, Negus SS. Experimental design and analysis for consideration of sex as a biological variable. Neuropsychopharmacology. 2019;44(13):2159-2162. doi:10.1038/s41386-019-0458-9).
8. Line 343 onwards (Recommendations #2):
– Please discuss further whether 1:1 female:male is the only valid ratio. Would other ratios be ok in some circumstances? When is this ratio more important than other variables (e.g. age)?
– Please acknowledge that recruiting a sample that consists of 50% females and 50% males may increase variability (in some circumstances) compared to studies with 100% of one sex, and therefore change power calculations and/or require larger sample size.
9. Recommendations: Please consider discussing whether one option for closing the gap would be to fund/conduct studies that only include females.
10. Line 315: Please provide a copy of the workshop program booklet or guide as supplemental information, so that "the way [you] constructed these workshops and facilitated discussion around sex could be used as a template for exploring other aspects of difference in future as research progresses". The reviewer was unable to find this information based on the references provided [75-77].
11. Line 433 onwards (Methods): Please report whether one or more authors conducted the bibliometric analyses. If multiple authors contributed, please indicate whether there were any inter-rater reliability tests done to assess accurate coding.
12. Please provide the source data for the bibliometric analysis, which should contain citation data and sex-based coding as presented in Figure 1.
https://doi.org/10.7554/eLife.65419.sa1Author response
Essential revisions:
1. Please shorten the introduction and reorganize the first two sections "Sex differences are ubiquitous in circadian rhythms and sleep" and "Sex differences in circadian rhythms can impact therapeutic interventions" into one cohesive introduction section which lays the ground for the literature analysis and the workshop discussion. In doing so, please make sure to streamline the text to ensure the reader feels it was written cohesively rather than by different people.
We have now shortened the introduction and combined the two sections, thereby streamlining the flow and harmonising style.
2. Lines 61-198 have a large overlap of topic matter with lines 200-266: please try to reduce this repetition. (For instance, the sub-section "What is the sex data gap?" repeats the conclusions from the previous section).
We have now revised this section and removed repetitions.
3. Line 73: Please discuss this point further, as "blockers" are not the only people who may resist change – they are probably a tiny minority of people. Far more people resisting change are probably people who don't know enough about how or why.
The term blockers was used in reference 7 (Karp et al., 2017) in a change management reflection on the resistance to studying both sexes. So the term blocker doesn’t refer to people but encompasses a myriad of practical, cultural and other issues. We have rewritten the paragraph to improve clarity:
“Sex bias or the 'sex data gap' has recently gained widespread attention (Criado-Perez, 2019), with policy advisers (Buitendijk and Maes, 2015), funders (Lee, 2018; Clayton and Collins, 2014) and publishers (Rippon et al., 2017; Docherty et al., 2019) pushing for the inclusion of both sexes in research. […] This two-pronged approach will unfreeze the status quo and allow new approaches to emerge (Karp and Reavey, 2019).”
4. Line 217 onward: Please discuss why there appears to have been a thorough review of studies involving light impact on human circadian rhythms, but not of other types of studies that are only mentioned briefly (e.g., circadian period or phase)
We use the area of non-visual effects of light as a very tangible example in this article. The area is very topical. We have now clarified this in the text:
“Because there are various efforts underway to incorporate scientific data from this research area into guidelines and recommendations for the provision of lighting in the built environment, there is a pressing need to understand sex bias in this area, which we have prioritised in the analysis reported here.”
5. Line 227 onwards: Please clarify whether there was a relationship between the proportion of female participants and the sample size of the study
We have included this additional analysis. We have added the following sentence:
“Interestingly, we also find that the total sample size correlates with the fraction of female participants in a given study (r(164) = 0.23, p = 0.00275).”
6. Line 268 onwards: Please provide further information about the workshop participants and how they contributed to the outcomes of the paper.
– For instance, please clarify the methods used to obtain participant reflections/responses (i.e. Did you provide participants with a certain set of questions or surveys to obtain the information described in Workshops 1 and 2? Alternatively, did this information come from discussions which were transcribed and coded?)
– Please indicate whether the participants of the workshop gave consent for their responses/comments to be used in this format? If not, please discuss whether there is a need for a retrospective-IRB approval.
– Please report how many individuals attended each workshop
– For each workshop section, please give all answers, not a selection of them. You can group into categories or ones with similar wording. The number of each question is not needed since there was limited time to respond and participants may not have been able to include all possibilities (so the number of each response does not necessarily reflect the importance of said response).
We have expanded upon the description of the workshops in both the workshop sections and have added a methods section describing these aspects. All workshop materials have been made available on the Open Science Framework (https://doi.org/10.17605/OSF.IO/WU2QX). We have limited the discussion of findings to key results from the interactive sessions.
We have added information regarding participant consent in the methods section:
“Prior to participating in the interactive sessions, attendees were informed that their responses will be used for write-up and published as a peer-reviewed article. […] No personal data were collected as part of the interactive Mentimeter sessions.”
We have added information about attendance numbers in the main section:
“A total of 275 individuals registered for the workshop. Across the three workshops, between 38 and 94 attendees participated in the interactive sessions, with approximately 4/5 of all participants being researchers (82/94 in Workshop 1, 47/60 in Workshop 2, 31/38 in Workshop 3). […] Responses to individual questions varied somewhat due to drop-out during the interactive session as well as a time-limited response window; the total number of responses in the participatory parts are given on the bottom right-hand corner of the materials document.”
We have added information on the question methodology as well:
“Throughout the interactive sessions, we combined yes/no, ranking and open-ended questions to vary the response modality. The results discussed below were selected from the results, which can be viewed in full on the Open Science Framework page above.”
7. Line 282: Please further discuss the statement that a common misconception in research is that the sample size must be doubled to include both sexes. The authors note that this is a flawed assumption because treatment effects are reported from the entire group. While this is certainly true, the authors should also acknowledge that the issue may be more complex than described, especially because sex differences exist. In the first half of this manuscript, the authors describe important differences that have been identified between sexes on a variety of outcomes (shiftwork tolerance, sleep differences, phase differences, etc.). Doesn't this suggest that pooling of males and females a bad idea, particularly for time-varying differences? For example, on lines 188-192, the authors describe the results of several important studies examining oxaliplatin and irinotecan in male and female patients. The authors note that the optimal timing for females and males were at different times for these treatments. If these reports would have pooled the data for males and females wouldn't that have attenuated the results or obscured the researchers' ability to observe a time-of-day effect at all? In this case, it seems pretty important that the analyses were stratified by sex. If a researcher wishes to examine outcomes by sex, as seems pretty important given the thesis of this manuscript, then one should be powered to conduct separate analyses (Diester CM, Banks ML, Neigh GN, Negus SS. Experimental design and analysis for consideration of sex as a biological variable. Neuropsychopharmacology. 2019;44(13):2159-2162. doi:10.1038/s41386-019-0458-9).
There are ways in which sex can impact an outcome variable of interest. Firstly, the variables baseline levels can depend on sex. Secondly, the treatment effect can depend on sex. When studying both sexes, a regression analysis will account for the sex differences in baseline levels and this has minimal impact on power. Regression analysis is not pooling the data which is completely inappropriate and can deliver misleading results. Recommending half the animals are male and half are female for a treatment group, is based on the premise that you want to estimate an average effect and then you can see a large interaction if it occurs. If we are interested in these differences, we then need the power to study the interaction which is a different goal.
In typical time course studies, where the time effect is also of interest, a regression analysis would ask at each time point what is the effect of sex, what is the effect of the treatment and does the effect depend on sex.
The section on page 8 has been reworded to give greater clarity and to highlight that pooling is not recommended.
8. Line 343 onwards (Recommendations #2):
– Please discuss further whether 1:1 female:male is the only valid ratio.
Statistically it is good practice to have balanced designs [ref: https://www.ncbi.nlm.nih.gov/pmc/articles/PMC2796056/]. ‘In a balanced design the main effects and interactions are orthogonal so that each one is estimated and tested as if it were the only one under consideration, with very little loss of efficiency due to the presence of other factors’. Basically, having a balanced design makes analysis more robust and ensures that factors are independent and the variance can be decomposed in to the individual contributions without confounding. Consequently, this is our recommendation as the default basic design. The following sentence has been added to page 11 “A balanced design is recommended to ensure the resulting statistical analysis is robust and that the variance can be decomposed to the factors of interest without confounding.”
Would other ratios be ok in some circumstances? When is this ratio more important than other variables (e.g. age?)
We have added a paragraph to page 9:
“A common push back is that there are other sources of variation (e.g. age) that should be considered and ask why should sex be the variable that is prioritized. Whenever we conduct an experiment we are simplifying a complex biological world with lots of sources of variation into a testing space and we generalize the findings allowing us to make conclusions. When considering biomedical research, on average the target population will be 50% male and 50% female and we are now understanding that the differences between male and female are significant and stretch beyond hormonal differences. Therefore, as a general rule, including both sexes should be the first variable to be included to significant increase generalizability. However, there may be exceptions, such as the study of sex specific conditions or phenomena, as written into the NIH guidelines.”
– Please acknowledge that recruiting a sample that consists of 50% females and 50% males may increase variability (in some circumstances) compared to studies with 100% of one sex, and therefore change power calculations and/or require larger sample size.
The data is not pooled and hence the sex differences are accounted for. With a regression analysis, when the treatment effect is the same or in the same direction then there is no loss in power to estimating the average effect. We gain then that the conclusions space is larger. When the treatment effect is crossed, then we do lose power on estimating the average effect but we gain in ability to test for an interaction. However, in this situation if you had studied one sex you would have missed a major differences in the understanding of the outcome. We have reworded the section on page 8 to improve the clarity of this discussion.
9. Recommendations: Please consider discussing whether one option for closing the gap would be to fund/conduct studies that only include females.
We do not think that the sex data gap can be closed if data are collected in a sex-selective fashion. If generalizable data are to be collected, then biasing the sample in the opposite direction is not helpful. If the suggestion is to fund data collection including only females post-hoc, then it is not clear what justifies data collection of the first sample with only males. The only way to resolve this is to collect data on males and females from the get-go.
10. Line 315: Please provide a copy of the workshop program booklet or guide as supplemental information, so that "the way [you] constructed these workshops and facilitated discussion around sex could be used as a template for exploring other aspects of difference in future as research progresses". The reviewer was unable to find this information based on the references provided [75-77].
We have now included a copy of the workshop program in the supplemental information on the Open Science Framework page. We have also expanded our description and links in the text:
“In a three-part virtual workshop (June 2020). we explored practices, barriers, and challenges in designing and executing inclusive research in circadian physiology and sleep research. […] Across the three workshops, between 38 and 94 attendees participated in the interactive sessions, with approximately 4/5 of all participants being researchers (82/94 in Workshop 1, 47/60 in Workshop 2, 31/38 in Workshop 3).”
In the workshop descriptions, to make it easier for the reader, we now explicitly write:
“The video recording for Workshop 1 is available at https://osf.io/4mk9g/. The materials related to the participatory part are available at https://osf.io/y3ha2/.”
“The video recording for Workshop 2 is available at https://osf.io/ep6uh/. The materials related to the participatory part are available at https://osf.io/d32qr/.”
“The video recording for Workshop 3 is available at https://osf.io/rtaqu/. The materials related to the participatory part are available at https://osf.io/ntjbv/.”
11. Line 433 onwards (Methods): Please report whether one or more authors conducted the bibliometric analyses. If multiple authors contributed, please indicate whether there were any inter-rater reliability tests done to assess accurate coding.
We have clarified that only one reviewer reviewed the articles.
12. Please provide the source data for the bibliometric analysis, which should contain citation data and sex-based coding as presented in Figure 1.
Source data are included in the supplementary material.
https://doi.org/10.7554/eLife.65419.sa2Article and author information
Author details
Funding
Wellcome Trust (204686/Z/16/A)
- Manuel Spitschan
Deutsche Forschungsgemeinschaft (FI 2275/3-1)
- Dorothee Fischer
The funders had no role in study design, data collection and interpretation, or the decision to submit the work for publication.
Publication history
- Received:
- Accepted:
- Accepted Manuscript published:
- Version of Record published:
Copyright
© 2022, Spitschan et al.
This article is distributed under the terms of the Creative Commons Attribution License, which permits unrestricted use and redistribution provided that the original author and source are credited.
Metrics
-
- 1,675
- views
-
- 227
- downloads
-
- 24
- citations
Views, downloads and citations are aggregated across all versions of this paper published by eLife.