Quantifying the relationship between SARS-CoV-2 viral load and infectiousness
Figures
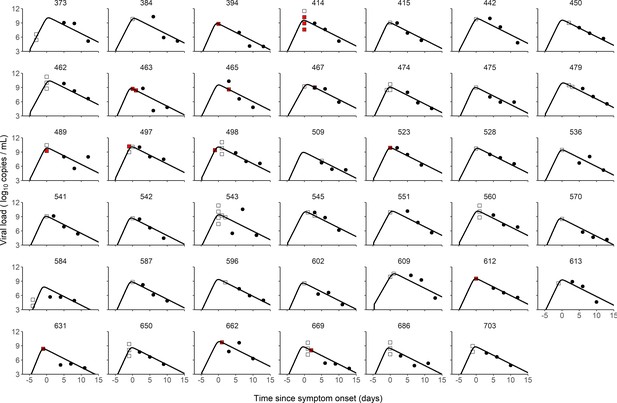
Individual fits of viral dynamics in index cases and occurrence of high-risk contacts.
Black dots represent the measured viral load. Squares indicate documented high-risk contacts, with empty squares representing contacts without transmission, and red squares representing contacts with a subsequent infection. Results obtained in the 41 index cases having three viral load measurements.
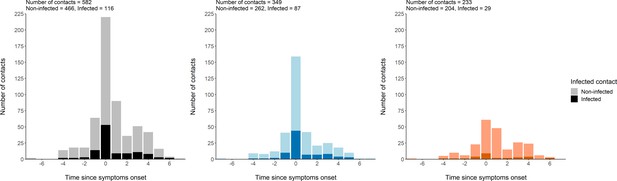
Distribution of contacts.
Distribution of the times at which a high-risk contact occurred between an index and a contact, with reference to the time of symptom onset of the index case. This indicates that most contacts reported in the study occurred at the time of symptom onset of the index, and that many contacts occurred during the pre-symptomatic period of the index. All contacts (Left). Household contacts (Middle). Non-household contacts (Right).
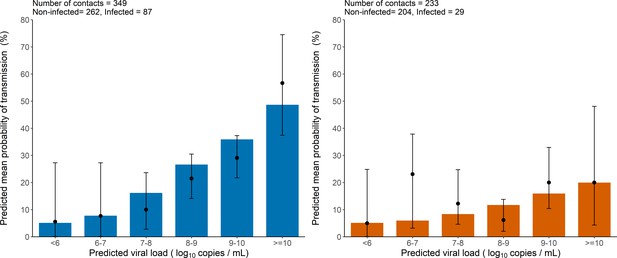
Model-based predictions of the effect of viral load on the risk of transmission and comparison to observed data.
Bars represent the mean predicted probability of transmission obtained from 1000 simulations of the model M2 and stratified by viral load level at the time of contact. Black dots are the proportion of transmission events observed in the data stratified by the predicted viral load of the index cases at the time of contact (along with their 95% confidence intervals). Household contacts (Left). Non-household contacts (Right).
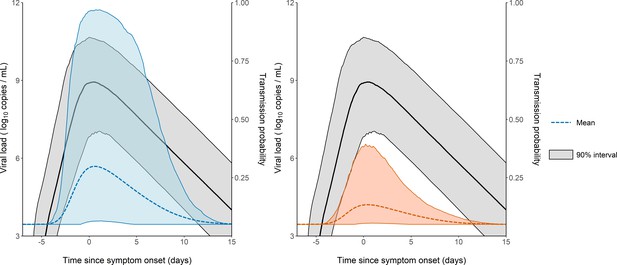
Model-based predictions of the dynamics of viral load and infectiousness over time.
Prediction interval of the viral load (black) and the probability of transmission over time after a high-risk contact obtained from 1000 simulations of the model. The shaded area represents the 90% inter quantile range. Household contacts (Left). Non-household contacts (Right).
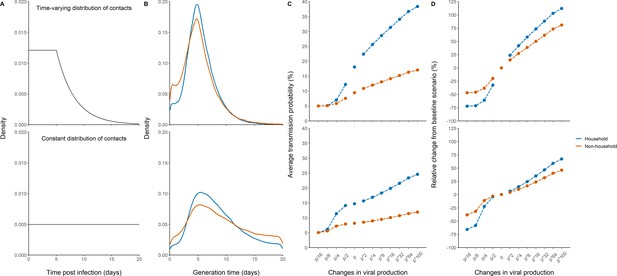
Effects of variants of concern and vaccination on transmission for different distributions of contacts.
(A) We considered a rate of contacts that could either decline after 5 days (top) or remain constant for the whole considered period (bottom). (B) Distribution of the generation interval using model M2 under each scenario. (C) Impact of changes in viral production on the average probability of transmission. (D) Impact of changes in viral production on the relative change from the baseline scenario in model M2.
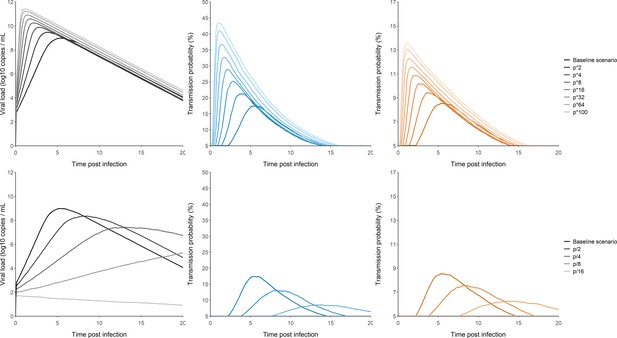
Effect of changes in the viral production on the viral load and transmission probabilities trajectories.
Effect of variants of concern (Top). Effect of vaccination rollout (Bottom). We only displayed the median trajectories over the simulated individuals. Viral load (Black). Transmission probability for household contacts (Blue). Transmission probability for non-household contacts (Orange).
Tables
Parameters estimates of the three candidate models.
, within-host basic reproductive number; , loss rate of infected cells; , rate of viral production; represents the effect of household contacts on the transmission probability; represents the effect of non-household contacts on the transmission probability. M1 assumes that transmission probability does not depend on the viral load. M2 and M3 use different parametric functions to relate the transmission probability to viral load at the time of contact. The distribution of the incubation period was fixed to values from the literature (see Materials and methods).
Parameter estimates (RSE %) | |||||||
---|---|---|---|---|---|---|---|
No effect of viral load (M1) | Logit-Linear (M2) | Log-Linear model (M3) | |||||
Fixed effect | Random effect SD | Fixed effect | Random effect SD | Fixed effect | Random effect SD | ||
Incubation period () | 5 | 0.125 | 5 | 0.125 | 5 | 0.125 | |
12.20 (14) | 0.32 (34) | 13.60 (15) | 0.38 (21) | 13.40 (22) | 0.423 (35) | ||
0.83 (1) | 0.019 (47) | 0.84 (4) | 0.037 (77) | 0.832 (100) | 0.023 (74) | ||
() | 1.97 × 105 (41) | 2.38 (9) | 2.8 × 105 (50) | 2.35 (8) | 2.40 × 105 (47) | 2.3 (9) | |
1.28 (38) | 0.82 (55) | 0.49 (20) | 0.85 (32) | 0.47 (6) | 0.545 (23) | ||
0.57 (62) | 0.21 (44) | 0.25 (17) | |||||
2502 | 2497 | 2500 |
Additional files
-
Source data 1
Viral load of index cases and list of their high-risk contacts.
- https://cdn.elifesciences.org/articles/69302/elife-69302-data1-v1.xlsx
-
Supplementary file 1
Parameter estimates of models with a fixed incubation period ranging from 4 to 7 days.
- https://cdn.elifesciences.org/articles/69302/elife-69302-supp1-v1.docx
-
Supplementary file 2
Parameters estimates of models with different threshold values below which the transmission is set to 5%.
- https://cdn.elifesciences.org/articles/69302/elife-69302-supp2-v1.docx
-
Supplementary file 3
Parameter estimates of the Model M2 (Left) and the same model without variability in transmission (Right).
- https://cdn.elifesciences.org/articles/69302/elife-69302-supp3-v1.docx
-
Supplementary file 4
Proportion of contacts wearing masks in each category (Left).
Secondary attack rate calculated for each category (Right).
- https://cdn.elifesciences.org/articles/69302/elife-69302-supp4-v1.docx
-
Transparent reporting form
- https://cdn.elifesciences.org/articles/69302/elife-69302-transrepform-v1.docx