Variation in the modality of a yeast signaling pathway is mediated by a single regulator
Abstract
Bimodal gene expression by genetically identical cells is a pervasive feature of signaling networks and has been suggested to allow organisms to hedge their ‘bets’ in uncertain conditions. In the galactose-utilization (GAL) pathway of Saccharomyces cerevisiae, gene induction is unimodal or bimodal depending on natural genetic variation and pre-induction conditions. Here, we find that this variation in modality arises from regulation of two features of the pathway response: the fraction of cells that show induction and their level of expression. GAL3, the galactose sensor, controls the fraction of induced cells, and titrating its expression is sufficient to control modality; moreover, all the observed differences in modality between different pre-induction conditions and among natural isolates can be explained by changes in GAL3’s regulation and activity. The ability to switch modality by tuning the activity of a single protein may allow rapid adaptation of bet hedging to maximize fitness in complex environments.
Introduction
Non-genetic heterogeneity is a pervasive feature of gene expression and cellular signaling (Kærn et al., 2005; Balázsi et al., 2011; Raj and van Oudenaarden, 2008). Bimodal responses, where cells in an isogenic population adopt one of two distinct states, are particularly important for microbes coping with fluctuating environments (Grimbergen et al., 2015; Veening et al., 2008) and cells of multicellular organisms differentiating into discrete types (Xiong and Ferrell, 2003; MacArthur et al., 2009).
The galactose-utilization (GAL) pathway in Saccharomyces cerevisiae is a well-characterized bimodal response and a classic model of microbial decision-making (Johnston, 1987; Bhat, 2008). Bimodality of GAL gene expression has been attributed to bistability arising from positive feedback through the Gal1p kinase and the Gal3p transducer (Venturelli et al., 2012; Acar et al., 2005). Perturbations of many of the components of the GAL pathway such as the Gal2p permease, the Gal4p activator, and the Gal80p repressor have been found to affect quantitative features of the GAL response (Acar et al., 2005; Hawkins and Smolke, 2006; Acar et al., 2010; Ramsey et al., 2006) and in principle could modify the feedback in the system and thus affect whether the response is bimodal or unimodal. However, only changes in Gal1p and Gal3p Venturelli et al., 2012; Acar et al., 2005 have been shown to affect modality.
Our existing insight into modality in the GAL system comes almost entirely from measuring one pathway phenotype, the induced fraction, under one environmental perturbation, galactose titration (Venturelli et al., 2012; Acar et al., 2010; Venturelli et al., 2015; Peng et al., 2015; Lee et al., 2017). The few studies that have deviated from this experimental approach have resulted in observations that raise new questions. For example, the GAL response was found to be unimodal or bimodal depending on the carbon source prior to encountering galactose (Biggar and Crabtree, 2001); the molecular basis of this behavior is unknown. In our own previous work that focused on differences in GAL genes induction in mixtures of glucose and galactose between natural isolates (Lee et al., 2017; Wang et al., 2015), we noted that some strains showed a bimodal response while others had a unimodal response (Lee et al., 2017). These differences provide an opportunity to dissect the genetic basis underlying the differences in modality.
In this work, we confirm and expand the observation that the pattern of GAL pathway induction can be either unimodal or bimodal depending on genetic background and pre-induction conditions. A phenomenological model of GAL induction led us to a conceptual framework for variation in modality that identified the relative thresholds of induced fraction and expression level regulation as the two critical factors. Using this simple framework, we can explain the variation in modality we observed and predict how new perturbations would affect modality. Finally, we show that both natural variation and pre-induction conditions achieve changes in modality by tuning the expression and activity of a single signaling protein in the GAL pathway. These results reveal a simple evolutionary mechanism by which organisms can shape their responses to the environment, and suggest that modality is a highly adaptable feature of a signaling response.
Results
Genetic and environmental factors affect the modality of the GAL response
To study what causes the GAL response to be unimodal in some strain backgrounds and bimodal in other strain backgrounds, we measured the expression of a GAL1 promoter driving YFP (GAL1pr-YFP) in 30 geographically and ecologically diverse yeast strains (Wang et al., 2015; Liti et al., 2009; Cromie et al., 2013) grown in different combinations of glucose and galactose (Figure 1A). We titrated glucose concentration in a constant concentration of galactose and observed that some displayed bimodal population distributions (Figure 1B, ‘DBVPG1106’) while others displayed unimodal population distributions with a glucose-dependent GAL1 expression level (Figure 1B, ‘BC187’, Figure 1—figure supplement 1; Ricci-Tam et al., 2021). Both unimodal and bimodal population distributions were stable even when cells were kept in the same environment for 24 hr (Figure 1—figure supplement 2), suggesting that the difference in modality is a steady-state phenomenon. In addition, we studied what causes the modality of the GAL response to change based on growth history. Biggar and Crabtree, 2001, previously showed that populations of the laboratory strain S288C had a unimodal GAL induction when grown with raffinose as a carbon source prior to encountering mixtures of glucose and galactose, but a bimodal response when mannose was used as the initial carbon source (Figure 1C). In contrast to our results when titrating galactose in the presence of glucose, all 30 of our natural isolates showed bimodal responses when we titrated the galactose concentration in the absence of glucose (Figure 1D, Figure 1—figure supplement 3). These observations suggest that glucose plays a critical role as a second input to the bistable GAL pathway that can cause a qualitative change in the modality of GAL pathway induction.
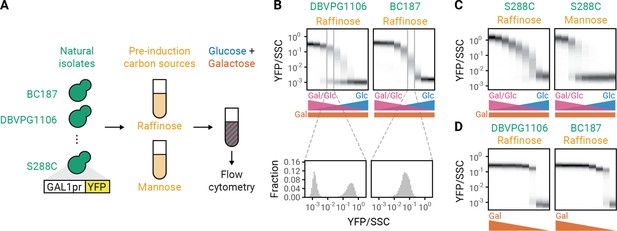
Genetic and environmental factors change galactose-utilization (GAL) modality.
(A) Experimental workflow. Natural isolates of yeast tagged with a fluorescent reporter of GAL1 (GAL1pr-YFP) expression were first grown in synthetic (S) medium with a pre-induction carbon source for 16 hr, then switched to S medium with mixtures of glucose and galactose. After 8 hr, GAL1 expression was analyzed by flow cytometry. (B–C) GAL induction of two natural isolates (DBVPG1106 and BC187) or a lab strain (S288C) in mixtures of glucose and galactose after pre-induction growth in raffinose or mannose. Glucose concentration was titrated in twofold steps from 0.0039% to 1% while galactose concentration was kept constant at 0.25%. Top: Induction profiles of two natural isolates. Each plot is composed of nine histograms with color intensities corresponding to the density of cells with a given YFP abundance. Galactose concentration was titrated in twofold steps from 1% to 0.0039%. Bottom: Blow out of two histograms of YFP level normalized by side scatter (SSC) at a single concentration of glucose and galactose (see Figure 1—figure supplement 4 for histograms of all conditions). Since the GAL pathway responds to the ratio of galactose and glucose (Escalante-Chong et al., 2015), increasing the glucose concentration while keeping the galactose concentration constant simultaneously decreases GAL activation and increases glucose repression. All measurements are representative examples of at least two independent repeats. Repeat measurements are plotted in Figure 1—figure supplement 5. (D) GAL induction of two natural isolates in different galactose concentrations after pre-induction growth in raffinose.
Differences in induction and expression level regulation can explain the variation of modality
In the absence of galactose, Gal80p binds the transcription factor Gal4p and keeps it in an inactive state (Lue et al., 1987; Wu et al., 1996). In the presence of galactose, Gal3p binds Gal80p, releasing Gal4p from the Gal80p-Gal4p complex, and allowing Gal4p to drive the transcription of a number of GAL genes. The initiation of transcription of the galactose sensors Gal1p and Gal3p by Gal4p creates a positive feedback loop, and this is believed to be the mechanism underlying the bistability of the system (Venturelli et al., 2012).
While glucose can completely inhibit the GAL response, its role in determining the modality of the response has been poorly explored. Glucose has two inhibitory effects on the GAL pathway. (1) It indirectly decreases the intracellular concentration of galactose through competition for binding to transporters (Escalante-Chong et al., 2015). This in turn decreases the amount of active Gal3p and thereby affects the fraction of cells that induce the GAL pathway (induced fraction) (Ricci-Tam et al., 2021). (2) It directly increases the activity of the transcriptional repressor Mig1p which regulates GAL4 expression and thereby decreases the expression of GAL genes (induction level) (Ricci-Tam et al., 2021; Figure 2A). Because the system is bimodal in pure galactose, glucose cannot drive unimodality solely through the transporter-dependent indirect effect, leading us to suspect that the Mig1p-dependent mechanism is responsible.
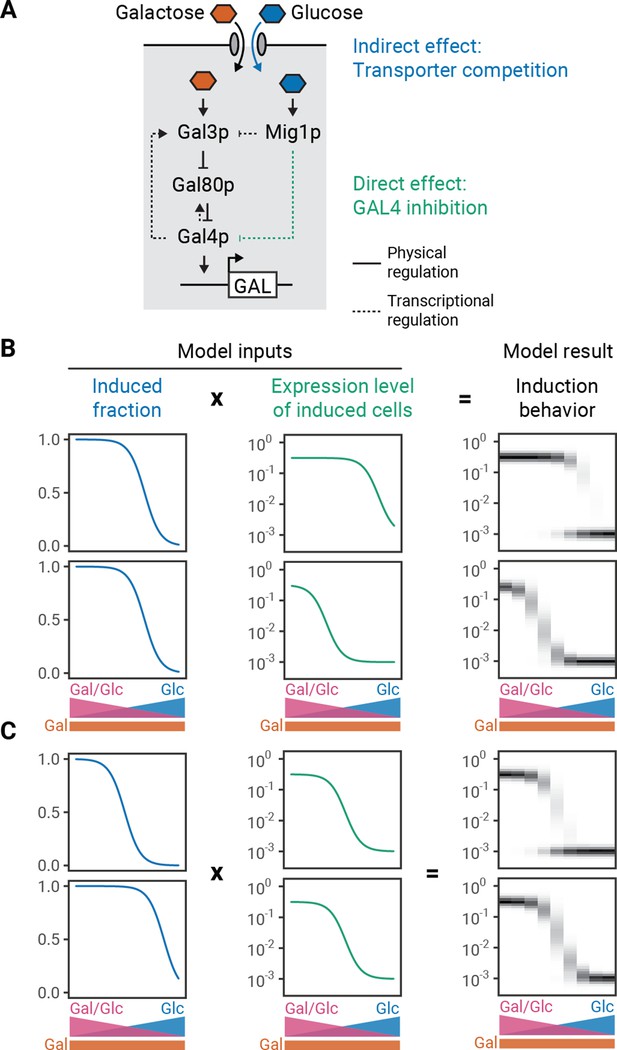
Phenomenological modeling of galactose-utilization (GAL) induction.
(A) Schematic description of the GAL pathway with indirect or direct inhibition by glucose. (B–C) Modeling results for varying expression level regulation with constant induced fraction regulation (B) or varying induced fraction regulation with constant expression level regulation (C). The induced fraction and mean induced level curves were chosen to be Hill functions based on empirical data (e.g. Figure 1—figure supplement 5). To create a population distribution, normal distributions were defined around the mean (log) induced level and a constant uninduced expression level, with standard deviations determined by fitting to observed distributions (Figure 2—figure supplement 1). The overall expression distribution is the induced-fraction-weighted sum of induced and uninduced distributions. For the uninduced subpopulation, the relative expression level was set to 10−3. Model results are represented by histograms at nine different glucose and galactose combinations. The intensity of the color on the plot corresponds to the density of cells with a given induction value. This analysis can be extended to a continuous range of induced fraction and induced level behaviors (Figure 2—figure supplement 2).
We built a phenomenological model of the GAL pathway to determine whether independently tuning the indirect and direct effects of glucose are sufficient to change modality. Based on our measurements of the pathway response (Ricci-Tam et al., 2021), we mathematically described the indirect and direct effects of glucose as Hill functions that decrease with increasing glucose concentration, with the final induction profiles being a simple product of these composite functions. To simulate a range of population induction profiles, we generated population distributions for the induced fraction and induced level from a normal distribution whose standard deviation is derived from GAL gene expression measurements (Figure 2—figure supplement 1; Materials and methods). We then varied the glucose threshold for the induced level while keeping the glucose threshold for the induced fraction constant (Figure 2B) or the glucose threshold for the induced fraction while keeping the glucose threshold for the expression level constant (Figure 2C). Indeed, changing either can switch the population behavior between unimodal and bimodal. In both cases, the pathway is bimodal when the glucose inhibition threshold for the induction level is less than the glucose inhibition threshold for the induced fraction.
Differences in induction and expression level regulation predict the modality of natural isolates
To analyze whether natural genetic variation could change modality by altering the induced fraction or induction level, we analyzed these features in our 30 natural yeast isolates. First, we determined the induced and uninduced subpopulations by comparing GAL reporter distributions to the distribution of an uninduced sample (as in Peng et al., 2015, Figure 3—figure supplement 1). From these two subpopulations, we then calculated two summary metrics for each strain’s behavior: E10 (‘expression level threshold’), the glucose concentration where the GAL1 expression level of the induced subpopulation reaches 10% of its level in pure galactose (Figure 3A), and F90 (‘induced fraction threshold’), the glucose concentration where 90% of cells are in the induced subpopulation (Figure 3B). Our modeling suggests that determining the E10 and F90 should be sufficient to predict whether the induction behavior is bimodal or unimodal. To test this hypothesis, we used the measured E10 and F90 as inputs into phenomenological model and computed modality. Overall, for both bimodal and unimodal strains, the experiments and models are in good agreement (Figure 3C and D, Figure 3—figure supplements 2–3). One minor discrepancy is that metrics from unimodal strains often predict a narrow range of bimodality in our model. We believe there are two factors that might explain the difference between the experimental data and the model predictions. First, to measure the F90, there must be measurable gene induction. Therefore, the calculated F90 is a lower bound for the actual value of F90 in unimodal strains; in many cases, the calculated F90 will be higher than the actual F90. If the calculated F90 for simulations of unimodal strains is increased by even a factor of 2, the discrepancy between the behaviors disappears (Figure 3—figure supplement 4). Second, the slopes of the induced fraction curves could also be subject to variation and this could affect modality. Increasing the steepness of the induced fraction curve or induced level curves by increasing the Hill coefficients in our models can make simulations more unimodal (Figure 3—figure supplement 5). Indeed, some natural isolates appear to have steeper induction curves (Figure 3—figure supplement 6). Overall, however, our model correctly predicts and provides a useful conceptual lens for understanding bimodality of GAL pathway induction across natural isolates.
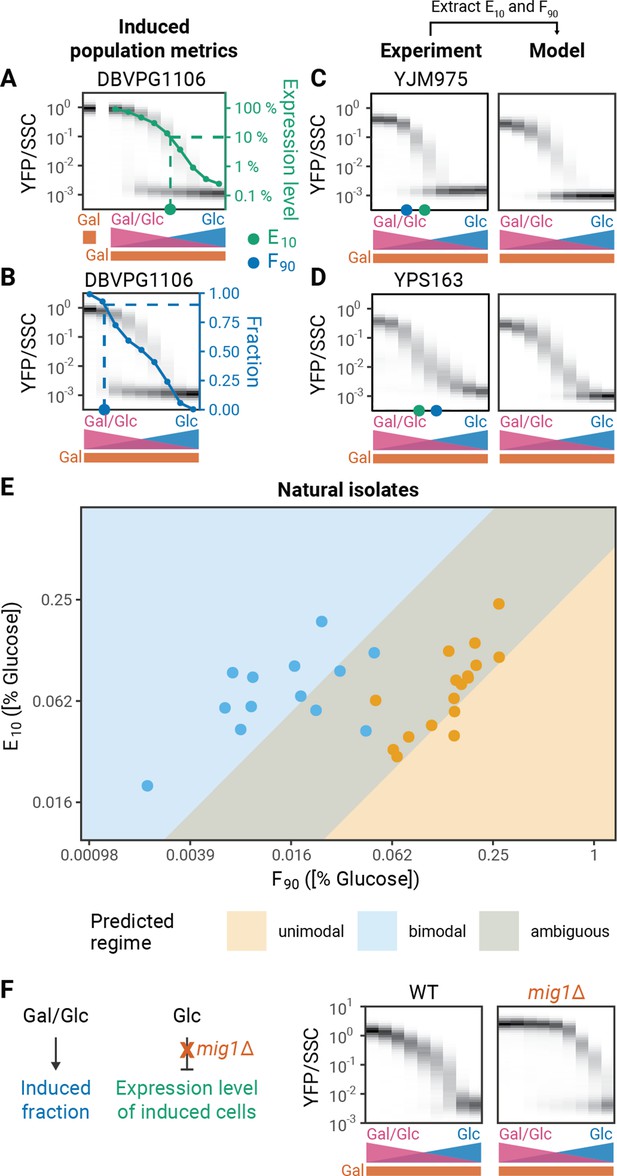
Experimental validation of model predictions.
(A) Expression level metric (E10). GAL induction is measured in 2% galactose to determine the maximal expression level. The E10 is the glucose concentration at which the expression level of the induced subpopulation reaches 10% of the expression level in 2% galactose in the absence of glucose. (B) Induced fraction metric (F90). The induced fraction for each strain is calculated as the fraction of cells with an expression level that is outside the range of an uninduced population grown in 2% glucose (Figure 3—figure supplement 1). The F90 is the glucose concentration where the induced fraction reaches 90%. (C–D) Modality prediction based on induction metrics. In the phenomenological model, the position of the induced fraction and induced level functions are determined by the F90 and the E10, respectively. (E) F90 and E10 values of a panel of 30 natural isolates. Values correspond to the mean of two to five replicates. Modality was determined by comparing the fit of the data to a single or double Gaussian model (See Materials and methods). Background colors correspond to predicted regimes for unimodal and bimodal strains. Simulations with diverse combinations of F90 and E10 values as well as different slopes for the induced fraction and induced level curves delineate regimes of unimodal and bimodal behaviors. The overlap represents an ambiguous regime where both unimodal and bimodal behaviors are possible. Standard deviations of the F90 and E10 measurements are plotted in Figure 3—figure supplement 7. (F) Effect of mig1Δ on GAL1 induction profiles.
We next scanned the parameters for our phenomenological model using a wide range of summary metrics and slopes to delimit a phase diagram of GAL induction modality. We then compared this phase diagram to the experimental data from natural isolates (Figure 3E). The modality of the natural isolates agrees well with their predicted modality in the phase diagram, supporting our hypothesis that the E10 and F90 measurements capture the important biological features that determine the modality of a strain.
Our phenomenological model gives us a potential molecular explanation for unimodality: Mig1p activity leads to unimodality by inhibiting GAL4 expression in the regime where Gal3p activation is still strongly dependent on glucose and galactose. Therefore, a strong prediction of the model is that removing Mig1p regulation should restore bimodality in unimodal strains. To test this prediction, we analyzed the induction profile of a mig1Δ strain. Deleting MIG1 removes the glucose dependent regulation of GAL4 expression level and thus the E10 of the deletion strain is increased compared to the wild-type strain (Figure 3F). As predicted, deleting MIG1 converts the strain from unimodal to bimodal. In addition, the observed F90 value for the bimodal mig1Δ strain is higher than that for the unimodal wild type. This supports our hypothesis that Mig1p-dependent repression conceals the actual F90 value of unimodal strains and that the observed F90 value of unimodal strains is a lower bound for the actual F90 value.
Differences in induction regulation explain the history dependence in modality
It has previously been reported that pre-induction growth conditions can affect the modality of GAL induction (Biggar and Crabtree, 2001). This offers another opportunity to test the predictions from our modeling framework. To see how metabolic history affects F90 and E10, we grew 13 natural isolate strains in mannose, raffinose, acetate, or glycerol prior to transferring them into mixtures of glucose and galactose (Figure 4—figure supplement 1). A range of different behaviors were observed, which could be broken into two categories of responses. The first category is strains that are unimodal in some pre-induction conditions but bimodal in others (Figure 4A and C). The second category is strains that do not change modality based on the tested pre-induction conditions; the strains are always bimodal or always unimodal in all pre-induction carbon sources tested here (Figure 4E). As predicted by the model, pre-induction conditions led to changes in F90 and/or E10 that should change modality; all our experimental results agree with the predictions from our phase diagram of GAL induction (Figure 4B,D and F). While changing either F90 or E10 in model is sufficient to change modality, we found that in response to changes in pre-induction carbon the change in F90 was considerably larger than the change in E10 (8.6 versus 1.8, respectively; Figure 4—figure supplement 2). Our phenomenological model predicts that the magnitude of the changes in F90 alone are sufficient to explain the observed changes of modality.
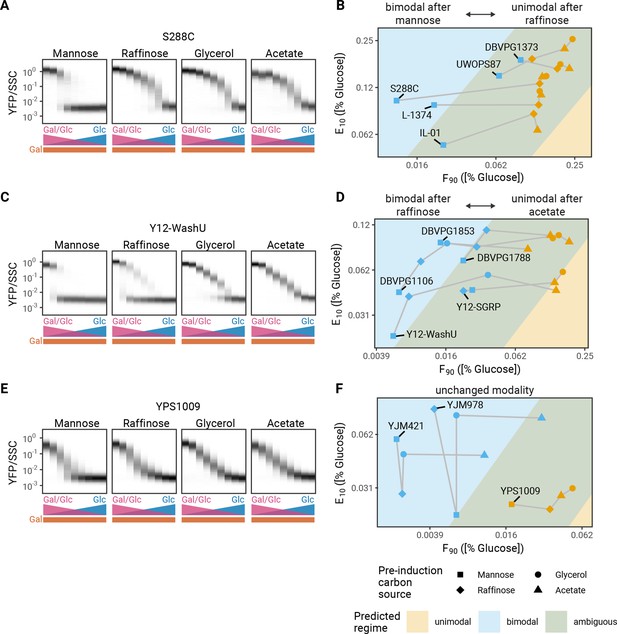
Metabolic history changes modality.
(A) History dependence of induction profiles. Induction profiles of S288C in mixtures of glucose and galactose after pre-induction growth in different carbon sources for 16 hr. (B) F90 and E10 values for isolates that are unimodal after growth in raffinose and bimodal after growth in mannose. (C) Induction profiles of Y12-WashU after growth in different carbon sources for 16 hr. (D) F90 and E10 values for isolates that are bimodal after growth in raffinose and unimodal after growth in either acetate or glycerol. (E) Induction profiles of YPS1009 after growth in different carbon sources for 16 hr. (F) F90 and E10 values for isolates that are always unimodal or bimodal. All measurements are representative examples of two independent repeats (compared in Figure 4—figure supplements 3–4).
Differences in GAL3 expression explain the history dependence in modality
To determine how pre-induction carbon source modulates F90, we measured the expression of GAL genes in pre-induction conditions using transcriptional reporters (Figure 5A). We found that GAL genes are down-regulated in carbon sources that lead to bimodal induction (mannose) and up-regulated in carbon sources that lead to unimodal induction (acetate, glycerol). Among all GAL genes, the expression levels of GAL3 and GAL4 show the strongest fold change between the carbon sources tested (Figure 5A).
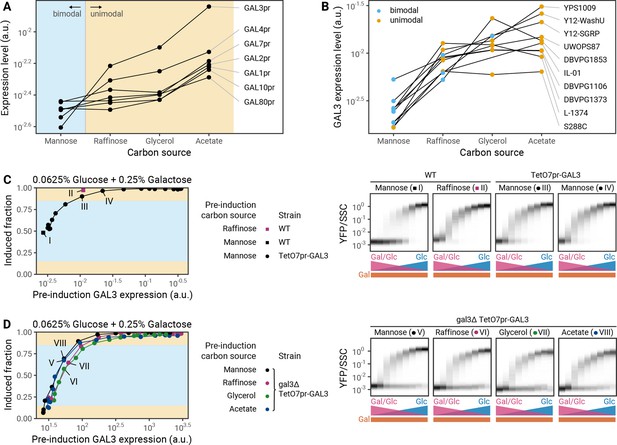
Pre-induction GAL3 levels determine the modality of induction profiles.
(A) Expression of galactose-utilization (GAL) genes in different pre-induction carbon sources in S288C as determined by fluorescence of GAL promoter-YFP protein transcriptional reporter strains. (B) Effect of pre-induction growth in different carbon sources on the expression of a GAL3S288C reporter in S288C and nine natural isolates. Colors correspond to the modality of the induction profile of these strains in the given pre-induction carbon source. (C) Left: Effect of GAL3 overexpression during pre-induction growth in mannose. Connected points correspond to a doxycycline titration series for a S288C TetO7pr-GAL3 strain. Right: Complete induction profiles of S288C in mannose (I) or raffinose (II) and S288C TetO7pr-GAL3 in mannose at two concentrations of doxycycline that lead to GAL3 expression levels that bracket the expression level of GAL3 from a raffinose pre-induction culture (III, IV). (D) Left: Effect of synthetic GAL3 expression in a Δgal3 during pre-induction growth in different carbon sources. Connected points correspond to a doxycycline titration series in different carbon sources for a S288C Δgal3 TetO7pr-GAL3-mScarlet strain. Right: Complete induction profiles of S288C Δgal3 TetO7pr-GAL3-mScarlet after pre-induction growth in different carbon sources with constant GAL3 expression.
We hypothesized that GAL3 was more likely than GAL4 to be the dominant factor due to its high dynamic range of expression (Figure 5A) and prior evidence that GAL3 can have a large effect on the GAL decision (Acar et al., 2010). We therefore analyzed the regulation of GAL3 by a range of pre-induction carbon sources in nine natural isolates using a transcriptional reporter for the GAL3S288C promoter. We found that in each strain the GAL3 expression level in a pre-induction conditions generally correlates with the modality observed later (Figure 5B).
To test if GAL3 expression prior to induction is the key determinant of modality, we used a tetracycline-inducible promoter to control the expression of GAL3 directly. We predicted that forcing a change in GAL3 expression while keeping the pre-induction carbon the same should change modality. Conversely, changing the pre-induction carbon without changing GAL3 expression should not change modality.
Indeed, we found that the pre-induction level of Gal3p, not the pre-induction carbon, is critical for setting modality. For the laboratory strain S288C, pre-induction growth in mannose leads to low GAL3 expression and a bimodal induction profile, while pre-induction growth in raffinose leads to higher GAL3 expression and a unimodal induction profile (Figures 4A and 5A). When we overexpressed GAL3 during pre-induction growth in mannose using tetracycline induction, we saw an increase in the induced fraction and a loss of bimodality (Figure 5C). Thus, the GAL3 concentration pre-induction is sufficient to set the modality in this strain background. Similarly, artificially setting the GAL3 level of mannose pre-induction cultures to that of a raffinose pre-induction culture converted the induction profiles to one similar to a raffinose pre-induction culture (Figure 5C,II and IV). In addition to showing unimodal induction after raffinose pre-induction, this strain also shows unimodal behavior after pre-induction in acetate or glycerol (Figure 4A). Next, we titrated GAL3 in a strain where the endogenous GAL3 gene was deleted to allow for constant GAL3 expression below the wild-type level. As predicted, when pre-induction GAL3 expression in these sugars was low, we saw bimodal induction almost identical to that seen with mannose pre-induction (Figure 5D). Pre-induction GAL3 concentrations also set the induced fraction with almost no dependence on the pre-induction carbon source (Figure 5D). We conclude that regulation of GAL3 expression in pre-induction conditions is the major driver of history dependence in the modality of GAL induction.
Natural variation in GAL3 alleles underlies the genetic changes in modality
The central role of GAL3 expression in setting the modality of induction suggests that natural variation in GAL3 alleles could be responsible for the observed differences in modality between isolates (Figure 1B). Previously, we showed that polymorphisms in the GAL3 gene explain most of the natural variation in the decision to induce the GAL pathway (i.e. the F90) (Lee et al., 2017), suggesting that allele swaps of the GAL3 ORF should alter the F90 of the strain, which in some cases would be enough to switch the modality of induction. To test this prediction, we determined the modality of a set of 30 allele swap strains comprised of 10 GAL3 alleles in three genetic backgrounds. The experimentally determined modality of all allele swap strains agrees with the expected modality (Figure 6A, Figure 6—figure supplement 1). For example, replacing the GAL3 allele of two unimodal strains, BC187 and S288C, with the GAL3 allele of any of the bimodal strains is sufficient to change the induction profiles from unimodal to bimodal (Figure 6B and C). In agreement with our model, the F90 in these strains decreases sufficiently to convert strains from unimodal to bimodal. Replacing the GAL3 gene of the bimodal strain YJM978 with the alleles of unimodal strains does not change the modality (Figure 6D). This is also in agreement with the model which predicts that the magnitude of the increase in F90 caused by allele swaps in the YJM978 strain background is insufficient to change modality (Figure 6D). Swapping the alleles of GAL1, the second galactose sensor, or GAL4, the transcription factor that activates GAL gene expression, does not affect modality (Figure 6—figure supplement 2). Similarly, swaps of GAL1 or GAL4 alleles in GAL3 allele swap strains have no additional effect on modality (Figure 6—figure supplement 3), suggesting that the role of GAL3 in setting modality can evolve independently of other GAL genes.
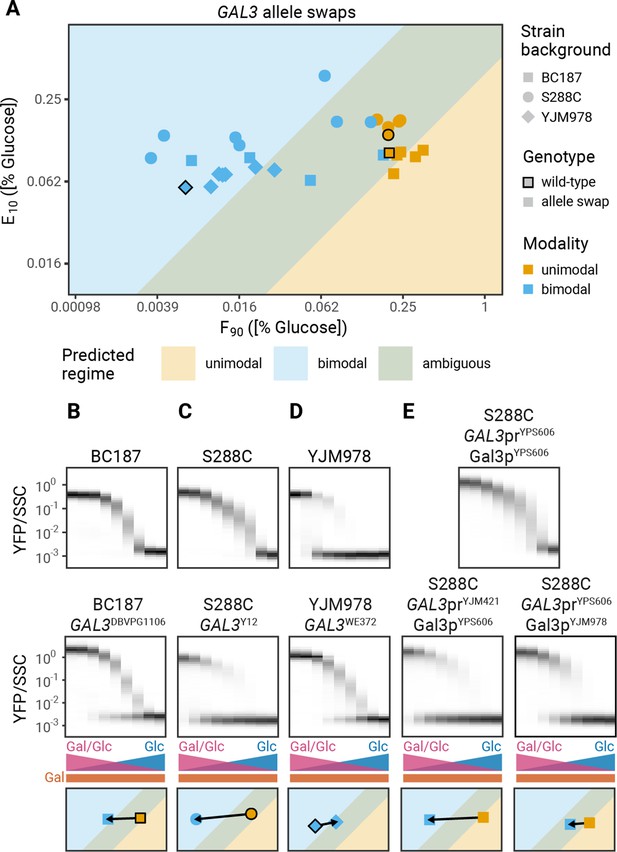
Allele swaps of GAL3 change modality.
(A) F90 and E10 values for a panel of allele swaps (10 GAL3 alleles in three different genetic backgrounds). Strains that never reach an induced fraction of 90% are not shown here. Black outlines denote the wild-type strains. All measurements are representative examples of two independent repeats (compared in Figure 6—figure supplements 4–5). (B–E) Effect of GAL3 allele swaps on modality. (Top) Induction profiles of (B–D) wild-type isolates or (E) S288C GAL3YPS606. (Middle) Induction profiles of (B–D) GAL3 allele swaps and (E) GAL3 promoter or GAL3 coding regions (CDS) swaps. (Bottom) Effect of the perturbation on F90 and E10. Arrows start at the values of the wild-type or S288C GAL3YPS606 strains and end at the values of the perturbed strain.
To further explore the variations in modality among the natural isolates, we analyzed the contribution of promoter and coding sequence variation. We found that SNPs (single nucleotide polymorphisms) in either promoter or coding regions (CDS) are sufficient to change modality (Figure 6E–F). For example, S288C with GAL3YPS606 is unimodal, but replacing the YPS606 promoter in this strain with the YJM421 promoter leads to bimodal induction (Figure 6E). Similarly, BC187 with GAL3NC-02 is unimodal, but replacing the NC-02 coding sequence with the YJM978 coding sequence leads to bimodal induction (Figure 6F). The mechanisms by which promoter and CDS changes are able to change the modality will be the subject of future work.
Discussion
In mixtures of glucose and galactose, the response of the GAL pathway in a population of yeast cells can be either unimodal or bimodal depending on their evolutionary history and their current environmental conditions. Here, we show that the modality of GAL induction in different strains depends on the relationship between the glucose effects on the induced fraction and the expression level. Glucose inhibits the expression of GAL genes, preventing the positive feedback that is crucial for bistability. In general, any input to a pathway that can conditionally create or eliminate feedback has the potential to modulate bistability. Since many signaling responses are controlled by multiple inputs, our findings imply that other unimodal responses could be bimodal in different conditions and vice versa.
Bimodality in the GAL response is considered a bet-hedging strategy where a fraction of the population prepares for glucose depletion by inducing the GAL pathway while other cells maximize their current growth and do not induce the pathway (Venturelli et al., 2015; Wang et al., 2015). This heterogeneity helps populations deal with uncertain, fluctuating environments. Bet-hedging is advantageous in the GAL system when the switching rate between glucose and galactose environments is high (Acar et al., 2008). Indeed, cells evolve bimodality in MAL gene expression when they are continuously switched between glucose and maltose (New et al., 2014). Because cells can sometimes be in environments with a high switching rate and other times in environments with a low switching rate, a strategy that allows the extent of bet-hedging to be tuned could be optimal. In this work, we show strains can tune the amount of bimodality both physiologically, based on their metabolic history, and genetically, presumably based on the environmental statistics that different natural isolates have faced in their evolutionary history. Further work will be needed to determine the evolutionary consequences of tunable bimodal responses such as the ones we characterize here.
Previous work on cell-to-cell heterogeneity has typically emphasized the complex genetic architecture of the pathways involved (Ansel et al., 2008; Fehrmann et al., 2013). In contrast, the physiological and genetic variation in modality in the GAL pathway can be explained by changes in the behavior of a single gene. Swapping the GAL3 alleles of natural isolates can turn a unimodal strain into a bimodal strain. We show that the environment tunes the expression level of GAL3, and this tuning is sufficient to change the modality of GAL induction (Figure 6 and Figure 6—figure supplement 1). Circuit designs such as these, where a single gene controls modality, may have been selected in evolution, since they allow cells to easily adapt their behavior on both physiological and evolutionary timescales.
The control of GAL pathway modality by mannose, raffinose, glycerol, and acetate suggests an additional layer of metabolic regulation that has been largely missed in previous analyses of this pathway. These findings show that factors other than canonical glucose catabolite repression can be important in determining the inducibility of GAL genes, consistent with our findings that many mutants outside the GAL pathway can have a significant effect on GAL response (Hua and Springer, 2018). The fact that pre-induction carbon sources mostly affect F90, just as GAL3 allele swaps do, suggests that the GAL3 positive feedback loop may be a nexus of regulation of GAL genes by multiple signals in the cell. In future studies, understanding the metabolic regulation of this well-studied system could give insight into the connections between metabolism and metabolic signaling in a variety of systems.
Materials and methods
Strains and media
Request a detailed protocolStrains were obtained as described in Lee et al., 2017; Wang et al., 2015. An initial set of 36 strains were assayed in a glucose gradient (1–0.0039%) with a constant background of 0.25% galactose. Strains DBVPG6765, CLIB324, L-1528, M22, W303, YIIC17-E5 were excluded from downstream analysis due to poor growth in our media conditions. Strain 378604X was also excluded due to a high basal expression phenotype that was an outlier in our collection. The genetic basis of this behavior is likely an interesting topic for follow-up studies. All experiments were performed in synthetic minimal medium (‘S’), which contains 1.7 g/L yeast nitrogen base (YNB) (BD, Franklin Lakes, NJ) and 5 g/L ammonium sulfate (EMD). In addition, D-glucose (EMD, Darmstadt, Germany), D-galactose (MilliporeSigma, St. Louis, MO), mannose (MilliporeSigma), glycerol (EMD), acetate (MilliporeSigma), and/or raffinose (MilliporeSigma) were added as a carbon source. Cultures were grown in a humidified incubator (Infors Multitron, Bottmingen, CH) at 30°C with rotary shaking 999 rpm (500 µL cultures in 1 mL 96-well plates).
Flow cytometry
Request a detailed protocolCells were struck onto YPD agar from −80°C glycerol stocks, grown to colonies, then inoculated from colony into YPD liquid and cultured for 16–24 hr. Then, cultures were inoculated in a dilution series (1:200 to 1:6400) in S + 2% pre-induction carbon source medium. The pre-induction cultures were incubated for 16–20 hr, and then their optical density (OD600) was measured on a plate reader (PerkinElmer Envision). The outgrowth culture with OD600 closest to 0.1 was selected for each strain, and then washed twice in S (with no carbon sources). To determine expression levels in pre-induction conditions, washed cells were then diluted in Tris-EDTA pH 8.0 (TE) in a shallow microtiter plate (CELLTREAT, Pepperell, MA). For sugar gradient experiments, washed cells were diluted 1:200 into the appropriate sugar in 96-well plates (500 µL cultures in each well) and incubated for 8 hr. Then, cells were harvested and fixed by washing twice in TE and resuspended in TE before transferring to microtiter plate for measurement. Flow cytometry was performed using a Stratedigm S1000EX with A700 automated plate handling system.
GAL3 titration in pre-induction conditions
Request a detailed protocolTo titrate GAL3 levels in the presence of the native GAL3 gene, the AGA1 gene was replaced with a MYO2pr-rtTA-TetO7pr-GAL3 construct in a hoΔ:GAL1pr-YFP strain. Cells were grown for 16 hr in S + 2% mannose as described above, but the medium was supplemented with doxycycline (MilliporeSigma) concentrations ranging from 38.9 to 0.0176 μg/mL in 1.5× dilutions steps. To measure the total GAL3 expression level after pre-induction growth, the AGA1 gene was replaced with a MYO2pr-rtTA-TetO7pr-YFP construct in a hoΔ:GAL3pr-YFP reporter strain. After pre-induction growth in the same dilution doxycycline concentrations, cells were harvested and YFP levels were determined using flow cytometry as described above.
To titrate GAL3 levels in the absence of the native GAL3 gene, the AGA1 gene was replaced with a MYO2pr-rtTA-TetO7pr-GAL3-mScarlet construct in a gal3Δ hoΔ:GAL1pr-YFP strain. Cells were grown for 16 hr in S + 2% pre-induction carbon source as described above, but the medium was supplemented with doxycycline concentrations ranging from 38.9 to 0.0176 μg/mL in 1.5× dilutions steps. To measure the total GAL3 expression level after pre-induction growth, cells were washed and mScarlet levels were determined by fluorescence microscopy using a Hamamatsu Orca-R2 camera (Hamamatsu, Japan) on a Ti Eclipse inverted Nikon microscope (Tokyo, Japan). Microscopy images were analyzed using U-net (Falk et al., 2019) and custom Python scripts.
Data analysis
Request a detailed protocolData analysis was performed using custom MATLAB scripts, including Flow-Cytometry-Toolkit (https://github.com/springerlab/Flow-Cytometry-Toolkit); Springer, 2016.
To determine the modality of GAL induction experiments, a Gaussian function was fitted to the population distribution for each of the nine sugar combinations. If the degree-of-freedom adjusted R2 of the fit was less than 0.99, two Gaussian functions were fitted to the data. Distributions were then determined to be bimodal if the distance between the means of the Gaussians was more than twice of the highest standard deviation of the Gaussian (as in Venturelli et al., 2012) and the fraction of the smaller Gaussian was higher than 0.15. The modality of induction profiles was mostly unaffected by changes in this threshold (Figure 3—figure supplement 7). GAL induction experiments or simulations that had a bimodal distribution in at least one combination of glucose and galactose in all replicates were called bimodal.
Phenomenological model
Request a detailed protocolInduction profiles were simulated from E10 and F90 metrics using functions that describe the induced fraction and the mean expression level of the induced subpopulations as a function of the glucose concentration. For the mean induced level, the following function was used:
where converts the glucose concentration where 10% of the maximal expression level is reached (i.e. the E10) to the glucose concentration where 50% of the maximal expression level is reached. The function was scaled from −3 to −0.5 to match the range of the experimental data. To obtain realistic versions for the n constant, this function was fitted to the induced level curves of natural isolates, the mean fitted n value was extracted for every natural isolate, and the mean of these values was used for simulations (induced level curve: 1.15, induced fraction curve: 1.69, see Figure 3—figure supplement 6).
For the induced fraction, the following function was used:
where converts the glucose concentration where 90% of the cells are induced (i.e. the F90) to the glucose concentration where 50% of the cells are induced. This function was fitted to the induced level curves of bimodal natural isolates, the mean fitted n value extracted for every natural isolate, and the mean of these values was used for simulations (Figure 3—figure supplement 6).
For nine concentrations of glucose and galactose, induced level and induced fraction values were extracted from these curves to generate simulated populations in these conditions. For a total population size of 20,000 cells, uninduced and induced subpopulations were generated according to the induced fraction value. The expression level values of cells were drawn from normal distributions with the mean expression level of the uninduced subpopulation at a constant level of 10−3 and the mean expression level of the induced subpopulation determined by the equation above. The standard deviations of the distributions were determined by fitting a quadratic equation to experimental standard deviations at different expression levels:
To delineate possible unimodal and bimodal regimes, GAL induction was simulated using all possible combinations of 10 different values for E10 and F90 (1, 0.5, 0.25, 0.125, 0.0625, 0.0313, 0.156, 0.0078, 0.0039, 0.0020, 0.0010). The Hill constants n for the induced fraction and the induced level functions in these simulations were varied between the lowest and highest experimentally fitted n values (induced level curve: 0.84 and 1.50, induced fraction curve: 0.75 and 2.95, see Figure 3—figure supplement 6). The E10 metric, the F90 metrics, and the modality of the induction profile were determined from these simulations as described above. In the F90-E10 space, unimodal and bimodal regimes were delineated by the bounding line with a slope of 1 that would capture all the unimodal or bimodal simulations respectively on one side of the line.
Data availability
All data is deposited in a Dryad repository (https://doi.org/10.5061/dryad.69p8cz8z8).
-
Dryad Digital RepositoryData from: Variation in the modality of a yeast signaling pathway is mediated by a single regulator.https://doi.org/10.5061/dryad.69p8cz8z8
References
-
Stochastic switching as a survival strategy in fluctuating environmentsNature Genetics 40:471–475.https://doi.org/10.1038/ng.110
-
BookGalactose Regulon of YeastBerlin, Heidelberg: Springer.https://doi.org/10.1007/978-3-540-74015-5
-
Cell signaling can direct either binary or graded transcriptional responsesThe EMBO Journal 20:3167–3176.https://doi.org/10.1093/emboj/20.12.3167
-
Genomic sequence diversity and population structure of Saccharomyces cerevisiae assessed by RAD-seqG3: Genes, Genomes, Genetics 3:2163–2171.https://doi.org/10.1534/g3.113.007492
-
Microbial bet-hedging: the power of being differentCurrent Opinion in Microbiology 25:67–72.https://doi.org/10.1016/j.mib.2015.04.008
-
The regulatory roles of the galactose permease and kinase in the induction response of the GAL network in Saccharomyces cerevisiaeJournal of Biological Chemistry 281:13485–13492.https://doi.org/10.1074/jbc.M512317200
-
A model fungal gene regulatory mechanism: the GAL genes of Saccharomyces cerevisiaeMicrobiological Reviews 51:458–476.https://doi.org/10.1128/mr.51.4.458-476.1987
-
Stochasticity in gene expression: from theories to phenotypesNature Reviews Genetics 6:451–464.https://doi.org/10.1038/nrg1615
-
Interaction of GAL4 and GAL80 gene regulatory proteins in vitroMolecular and Cellular Biology 7:3446–3451.https://doi.org/10.1128/MCB.7.10.3446
-
Systems biology of stem cell fate and cellular reprogrammingNature Reviews Molecular Cell Biology 10:672–681.https://doi.org/10.1038/nrm2766
-
Dual feedback loops in the GAL regulon suppress cellular heterogeneity in yeastNature Genetics 38:1082–1087.https://doi.org/10.1038/ng1869
-
Bistability, epigenetics, and bet-hedging in BacteriaAnnual Review of Microbiology 62:193–210.https://doi.org/10.1146/annurev.micro.62.081307.163002
Article and author information
Author details
Funding
National Science Foundation (MCB-1349248)
- Jue Wang
- Michael Springer
National Institutes of Health (GM120122)
- Julius Palme
- Michael Springer
National Science Foundation (DGE1144152)
- Jue Wang
The funders had no role in study design, data collection and interpretation, or the decision to submit the work for publication.
Copyright
© 2021, Palme et al.
This article is distributed under the terms of the Creative Commons Attribution License, which permits unrestricted use and redistribution provided that the original author and source are credited.
Metrics
-
- 779
- views
-
- 112
- downloads
-
- 7
- citations
Views, downloads and citations are aggregated across all versions of this paper published by eLife.
Download links
Downloads (link to download the article as PDF)
Open citations (links to open the citations from this article in various online reference manager services)
Cite this article (links to download the citations from this article in formats compatible with various reference manager tools)
Further reading
-
- Computational and Systems Biology
- Microbiology and Infectious Disease
The Staphylococcus aureus clonal complex 8 (CC8) is made up of several subtypes with varying levels of clinical burden; from community-associated methicillin-resistant S. aureus USA300 strains to hospital-associated (HA-MRSA) USA500 strains and ancestral methicillin-susceptible (MSSA) strains. This phenotypic distribution within a single clonal complex makes CC8 an ideal clade to study the emergence of mutations important for antibiotic resistance and community spread. Gene-level analysis comparing USA300 against MSSA and HA-MRSA strains have revealed key horizontally acquired genes important for its rapid spread in the community. However, efforts to define the contributions of point mutations and indels have been confounded by strong linkage disequilibrium resulting from clonal propagation. To break down this confounding effect, we combined genetic association testing with a model of the transcriptional regulatory network (TRN) to find candidate mutations that may have led to changes in gene regulation. First, we used a De Bruijn graph genome-wide association study to enrich mutations unique to the USA300 lineages within CC8. Next, we reconstructed the TRN by using independent component analysis on 670 RNA-sequencing samples from USA300 and non-USA300 CC8 strains which predicted several genes with strain-specific altered expression patterns. Examination of the regulatory region of one of the genes enriched by both approaches, isdH, revealed a 38-bp deletion containing a Fur-binding site and a conserved single-nucleotide polymorphism which likely led to the altered expression levels in USA300 strains. Taken together, our results demonstrate the utility of reconstructed TRNs to address the limits of genetic approaches when studying emerging pathogenic strains.
-
- Computational and Systems Biology
Plasmid construction is central to life science research, and sequence verification is arguably its costliest step. Long-read sequencing has emerged as a competitor to Sanger sequencing, with the principal benefit that whole plasmids can be sequenced in a single run. Nevertheless, the current cost of nanopore sequencing is still prohibitive for routine sequencing during plasmid construction. We develop a computational approach termed Simple Algorithm for Very Efficient Multiplexing of Oxford Nanopore Experiments for You (SAVEMONEY) that guides researchers to mix multiple plasmids and subsequently computationally de-mixes the resultant sequences. SAVEMONEY defines optimal mixtures in a pre-survey step, and following sequencing, executes a post-analysis workflow involving sequence classification, alignment, and consensus determination. By using Bayesian analysis with prior probability of expected plasmid construction error rate, high-confidence sequences can be obtained for each plasmid in the mixture. Plasmids differing by as little as two bases can be mixed as a single sample for nanopore sequencing, and routine multiplexing of even six plasmids per 180 reads can still maintain high accuracy of consensus sequencing. SAVEMONEY should further democratize whole-plasmid sequencing by nanopore and related technologies, driving down the effective cost of whole-plasmid sequencing to lower than that of a single Sanger sequencing run.