Prediction of type 2 diabetes mellitus onset using logistic regression-based scorecards
Figures
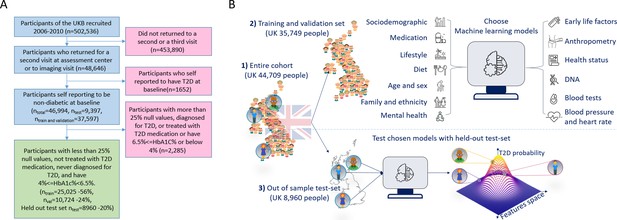
A flowchart of the cohort selection process and an illustrative figure of the model’s extraction.
(A) A flowchart of the selection process of participants in this study. We selected participants who came for a repeated second or third visit from the 502,536 participants of the UK Biobank (UKB). Next, we excluded 1652 participants who self-reported having type 2 diabetes (T2D). We then split the data into 80% for the training and validation sets and 20% for the holdout test set. We excluded an additional 2285 participants due to (1) having 25% or more missing values from the full feature list, (2) having HbA1c levels above or equal to 6.5%, or (3) being treated with metformin or insulin, (4) found to be diagnosed with T2D before the first UKB visit. The final training, validation, and test sets included 25,025 participants (56% of the cohort), 10,724 participants (24%), and 8960 participants (20%), respectively. (B) The process flow during the training and testing of the models. We first split the data and kept a holdout test set. We then explored several models using the training and validation datasets. We then compared the selected models using the holdout test set and reported the results. We calibrated the output of the models to predict the probability of a participant developing T2D.
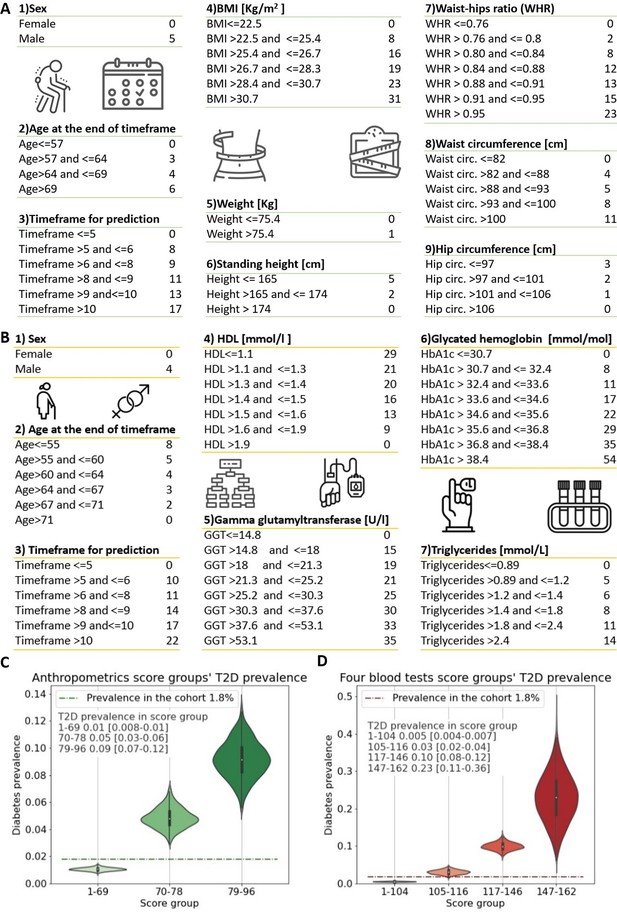
Anthropometrics and blood tests scorecards.
(A) Anthropometrics-based scorecard. Summing the scores of the various features provides a final score that we quantified into one of three risk groups (figure 2C). (B) “Four blood test” scorecard. Adding the scores of the various features provides a final score that we quantified into one of four risk groups (Figure 2D). (C) Anthropometrics scorecards risk groups - first group score range [1-69] 1% [0.8-1%] 95%CI of developing T2D which is below the cohorts 1.8% prevalence of T2D (green dashed line); Second group, score range 70-78 predicts a 5% [3-6%] 95%CI of developing T2D; Third group 79-96 9% [7-12%] 95%CI of developing T2D. (D) four blood tests scorecards risk groups - first group score range [1-104] <0.5% [0.04-0.7%] 95%CI of developing T2D which is below the cohorts 1.8% prevalence of T2D (red dashed line); Second group, score range 105-116 predicts a 3% [2-4%] 95%CI of developing T2D.; Third group range 117-146 with 10% [8-12%] 95%CI of developing T2D. Fourth group range 147-162 predicts 23% [10-37%] 95%CI of developing T2D, which is X13 fold prevalence enrichment compared to the cohort’s T2D prevalence.
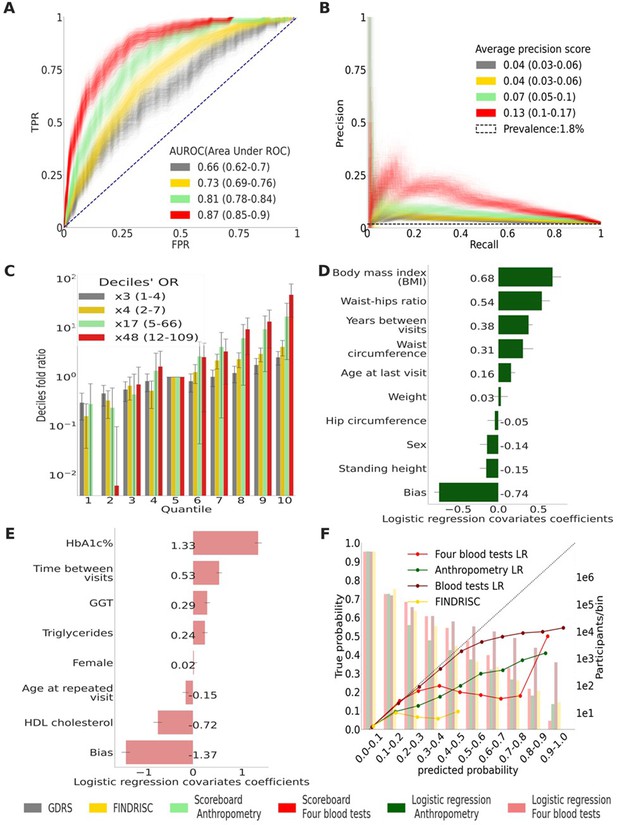
Main results calculated using 1000 bootstraps of the cohort population.
Each point in the graphs represents a bootstrap iteration result. The color legend is at the bottom of the figure. (A) Receiver operating characteristic (ROC) curves comparing the models developed in this research: a Gradient Boosting Decision Trees (GBDT) model of all features; logistic regression models of four blood tests; an anthropometry-based model compared to the well-established German Diabetes Risk Score (GDRS) and Finnish Diabetes Risk Score (FINDRISC). (B) Precision–recall (P-R) curves, showing the precision versus the recall for each model, with the prevalence of the population marked with the dashed line. (C) Deciles’ odds ratio graph, the prevalence ratio in each decile to the prevalence in the fifth decile. (D) A feature importance graph of the logistic regression anthropometry model for a model with normalized features values. The bars indicate the feature importance values’ standard deviation (SD). The top predictive features of this model are the body mass index (BMI) and waist-to-hip ratio (WHR). (E) Feature importance graph of logistic regression blood tests model with SD bars. While higher levels of HbA1c% positively contribute to type 2 diabetes (T2D) prediction, and high-density lipoprotein (HDL) cholesterol levels are negatively correlated with the predicted probability of T2D, the information provided by age and sex relevant for predicting T2D onset is screened by other features. (F) A calibration plot of the anthropometry, four blood tests, full blood test, and the FINDRISC models. Calibration of the models’ predictions allows reporting the probability of developing T2D (see ‘Methods’).
-
Figure 3—source data 1
Detailed results for the top to bottom quantiles OR calculation.
- https://cdn.elifesciences.org/articles/71862/elife-71862-fig3-data1-v3.csv
-
Figure 3—source data 2
Detailed coefficients for the non-laboratory logistic regression model.
- https://cdn.elifesciences.org/articles/71862/elife-71862-fig3-data2-v3.csv
-
Figure 3—source data 3
Detailed coefficients for the laboratory logistic regression model.
- https://cdn.elifesciences.org/articles/71862/elife-71862-fig3-data3-v3.csv
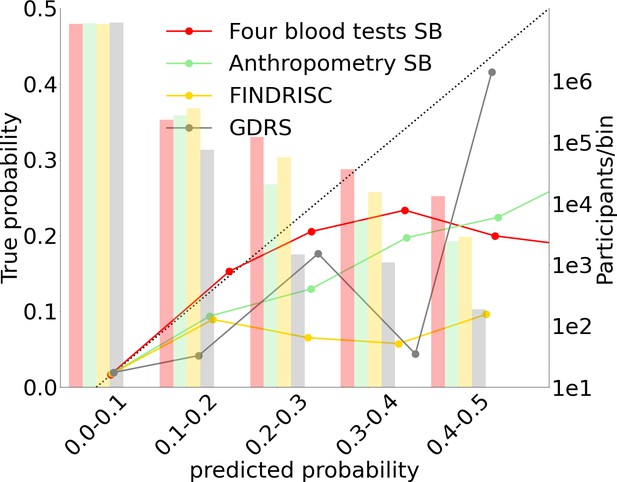
Models calibration plots.
Anthropometric, four blood tests, Finnish Diabetes Risk Score (FINDRISC), and German Diabetes Risk Score (GDRS) scorecards calibration graphs.
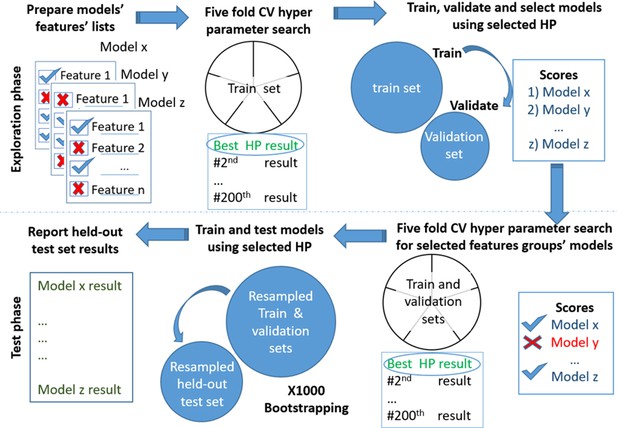
Models testing and training process.
Models’ development. Scheme of the models' exploration and evaluation process. For the models’ selection process, we used a fivefold cross-validation with 200 iterations of the random hyperparameters process for each group of features. We then selected the top-scored hyperparameters for each feature’s group. We trained a new model based on the training set and measured the area under the receiver operating curve (auROC) using the validation set. Out of the validated models, we chose the models that had a minimal number of features and provided high performance. The reported results are of the heldout test set.
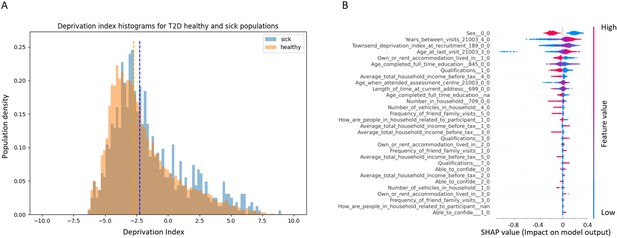
Socioeconomic impact on prediction of risk of developing type 2 diabetes (T2D).
(A) Deprivation index differences between T2D sick and healthy populations in our data: a density histogram showing the differences in deprivation index of participants who were diagnosed with T2D in one of their returning visits to the assessment center and for healthy participants. Executing a Mann–Whitney test on this data yields a p-value lower than 2.37 * 10–137, indicating a correlation between lower socio-demographic state with higher T2D prevalence. (B) Shapley Additive Explanations (SHAP) analysis of the socio-demographic features for a Gradient Boosting Decision Trees (GBDT) predictor of T2D: Each dot represents a participant’s value for each feature along the Y-axis. The colors indicate the values of the features: red indicates higher feature values, blue indicates lower feature values. The X-axis is the SHAP value, where higher SHAP values indicate a stronger positive impact on the positive prediction of the GBDT predictor, that is, higher risk for T2D onset. The analysis indicates that higher values of deprivation index and lower household income push the probability of T2D onset to higher values. The full meaning of the codes is provided at the UK Biobank data showcase.
Tables
Cohort statistical data.
Characteristics of this study’s cohort population and the UK Biobank (UKB) population. A ‘±’ sign denotes the standard deviation. While type 2 diabetes (T2D) prevalence in the UKB participants is 4.8%, it is 1.79% in our cohort as we screened the cohort at baseline for HbA1c% levels <6.5%. The age range of the participants at the first visit was 40–69; thus, our models are not suitable for people who develop T2D at younger ages. The models predict the risk of developing T2D between the first visit to the UKB assessment center and the last visit. We refer to this feature as ‘the time between visits’.
UKB population | Train, validation, and test sets | Test set | Train set | Validation set | |
---|---|---|---|---|---|
Number of participants | 502,536 | 44,709 | 8,960 | 25,025 | 10,724 |
Age at first visit (years) | 56.5 ± 8.1 | 55.6 ± 7.6 | 55.5 ± 7.5 | 55.6 ± 7.6 | 55.6 ± 7.6 |
Age at last visit (years) | - | 62.9 ± 7.5 | 62.9 ± 7.4 | 62.9 ± 7.5 | 62.9 ± 7.5 |
The time between visits (years) | - | 7.3 ± 2.3 | 7.3 ± 2.3 | 7.3 ± 2.3 | 7.3 ± 2.3 |
Males in the population (%) | 45.5 | 47.8 | 47.9 | 47.9 | 47.5 |
Diabetic at first visit (%) | 4.8 | 0 | 0 | 0 | 0 |
Diabetic at last visit (%) | - | 1.79 | 1.76 | 1.75 | 1.91 |
Hba1c at first visit (%) | 5.5 ± 0.6 | 5.3 ± 0.3 | 5.3 ± 0.3 | 5.3 ± 0.3 | 5.3 ± 0.3 |
Hba1c at last return (%) | - | 5.4 ± 0.4 | 5.4 ± 0.3 | 5.4 ± 0.4 | 5.4 ± 0.4 |
Weight at first visit (kg) | 78.1 ± 15.9 | 76.6 ± 14.7 | 76.4 ± 14.6 | 76.7 ± 14.7 | 76.8 ± 14.9 |
Weight at last visit (kg) | - | 76.2 ± 15.2 | 76.0 ± 14.9 | 76.2 ± 15.2 | 76.5 ± 15.3 |
Body mass index at first visit (kg/m2) | 27.4 ± 4.8 | 26.6 ± 4.2 | 26.5 ± 4.1 | 26.6 ± 4.2 | 26.7 ± 4.3 |
Body mass index at last visit (kg/m2) | - | 26.6 ± 4.4 | 26.5 ± 4.3 | 26.6 ± 4.4 | 26.7 ± 4.5 |
Hips circumference at first visit (cm) | 103.4 ± 9.2 | 102.1 ± 8.2 | 101.9 ± 8.0 | 102.1 ± 8.2 | 102.3 ± 8.3 |
Hips circumference at last visit (cm) | - | 101.6 ± 8.8 | 101.4 ± 8.7 | 101.6 ± 8.8 | 101.8 ± 9.0 |
Waist circumference at first visit (cm) | 90.3 ± 13.5 | 87.9 ± 12.5 | 87.7 ± 12.4 | 87.9 ± 12.4 | 88.2 ± 12.7 |
Waist circumference at last visit (cm) | - | 88.7 ± 12.7 | 88.5 ± 12.5 | 88.7 ± 12.7 | 89.0 ± 12.9 |
Height at first visit (cm) | 168.4 ± 9.3 | 169.5 ± 9.2 | 169.5 ± 9.1 | 169.5 ± 9.2 | 169.4 ± 9.1 |
Height at last visit (cm) | - | 169.0 ± 9.2 | 169.0 ± 9.2 | 169.0 ± 9.3 | 168.9 ± 9.2 |
External validation cohort (‘Clalit’) statistical data.
Males (%) | HbA1c (%) | GGT | Reticulocyte count | HDL | Triglycerides | Age | Weight | Height | BMI | |
---|---|---|---|---|---|---|---|---|---|---|
Number of samples | 17,132 | 17,132 | 83 | 17,132 | 17,132 | 17,132 | 17,051 | 17,051 | 17,051 | |
Mean value | 45 | 5.56 | 32.31 | 56.33 | 49.77 | 141.33 | 56.40 | 79.00 | 1.66 | 28.72 |
Standard deviation | 0.41 | 49.29 | 36.97 | 13.33 | 82.09 | 8.06 | 49.90 | 0.09 | 19.58 | |
0.25 | 5.30 | 17.00 | 38.35 | 40.00 | 90.00 | 50.14 | 67.00 | 1.59 | 24.80 | |
0.50 | 5.60 | 23.00 | 58.00 | 48.00 | 123.00 | 57.02 | 77.00 | 1.65 | 27.68 | |
0.75 | 5.90 | 33.00 | 78.60 | 57.00 | 170.00 | 62.83 | 87.82 | 1.72 | 31.25 |
-
HbA1c, hemoglobin A1c; GGT, gamma-glutamyl transferase; HDL, high-density lipoprotein; BMI, body mass index.
Comparing models' main results.
The values in parentheses indicate a 95% confidence interval (CI). The deciles’ odds ratio (OR) measures the ratio between type 2 diabetes (T2D) prevalence in the top risk score decile bin and the prevalence in the fifth decile bin (see ‘Methods’).
Measure type | Model type | APS | auROC | Decile’s prevalence OR |
---|---|---|---|---|
GDRS | Score card cox regression for 5 years | 0.04 (0.03–0.06) | 0.66 (0.62–0.70) | 2.5 (1.46–4.45) |
FINDRISC | Score card logistic regression | 0.04 (0.03–0.06) | 0.73 (0.69–0.76) | 4.13 (2.29–7.37) |
Anthropometry | Score card cox regression for 5 years | 0.04 (0.03–0.07) | 0.79 (0.75–0.83) | 8.8 (3.6–36) |
Anthropometry | Score card cox regression for 10 years | 0.06 (0.04–0.09) | 0.79 (0.76–0.82) | 10 (4.6–32.9) |
Anthropometry | Score card logistic regression | 0.07 (0.05–0.10) | 0.81 (0.77–0.84) | 17.2 (5–66) |
Anthropometry | Logistic regression | 0.09 (0.06–0.13) | 0.81 (0.78–0.84) | 16.9 (4.8–66) |
Anthropometry | Cox regression | 0.10 (0.07–0.13) | 0.82 (0.79–0.85) | 10.7 (5–24) |
Four blood tests | Score card cox regression for 10 years | 0.13 (0.10–0.16) | 0.87 (0.85–0.90) | 22.4 (9.8–54) |
Four blood tests | LR score card | 0.13 (0.10–0.17) | 0.87 (0.85–0.90) | 48 (11.9–109) |
Four blood tests | Score card cox regression for 5 years | 0.09 (0.06–0.12) | 0.89 (0.86–0.92) | 53.2 (18.9–84.2) |
Four blood tests | Cox regression | 0.25 (0.18–0.32) | 0.88 (0.85–0.90) | 43 (13.6–109) |
Four blood tests | Logistic regression | 0.24 (0.17–0.31) | 0.88 (0.85–0.91) | 32.5 (10.89–110) |
Blood tests | Logistic regression | 0.26 (0.19–0.33) | 0.91 (0.89–0.93) | 75.4 (17.7–133) |
All features | Boosting decision trees | 0.27 (0.20–0.34) | 0.91 (0.89–0.93) | 72.6 (15.1–135) |
-
APS, average precision score; auROC, area under the receiver operating curve; GDRS, German Diabetes Risk Score; FINDRISC, Finnish Diabetes Risk Score; DT, Decision Trees.
Comparing model results applied to an HbA1c% stratified population.
The values in parentheses indicate 95% confidence interval (CI). Results of the models applied to a stratified population. The mixed population-based model column provides the results of the scorecard models presented in Figure 2 applied to normoglycemic and prediabetes stratified population.
Population | Mixed population-based model: tested on a stratified population | Models built using a stratified training set | |||
---|---|---|---|---|---|
auROC | APS | auROC | APS | ||
Prediabetic (N = 1006, prevalence = 9.4%) | GDRS | 0.64 (0.57–0.70) | 0.17 (0.12–0.23) | - | |
FINDRISC | 0.66 (0.61–0.72) | 0.20 (0.14–0.27) | - | ||
Anthropometry | 0.73 (0.68–0.77) | 0.20 (0.15–0.26) | 0.73 (0.68–0.78) | 0.21 (0.16–0.27) | |
Four blood tests | 0.73 (0.68–0.77) | 0.20 (0.15–0.26) | 0.72 (0.67–0.77) | 0.21 (0.15–0.26) | |
Normoglycemic (N = 7948, prevalence = 0.8%) | GDRS | 0.67 (0.61–0.74) | 0.02 (0.01–0.03) | - | |
FINDRISC | 0.74 (0.69–0.79) | 0.04 (0.02–0.07) | - | ||
Anthropometry | 0.81 (0.76–0.86) | 0.04 (0.02–0.07) | 0.81 (0.76–0.85) | 0.03 (0.02–0.06) | |
Four blood tests | 0.81 (0.76–0.85) | 0.03 (0.02–0.05) | 0.82 (0.77–0.86) | 0.05 (0.03–0.09) |
-
auROC, area under the receiver operating curve; FINDRISC, Finnish Diabetes Risk Score; GDRS, German Diabetes Risk Score; APS, average precision score.
Four blood tests scorecard results from the external validation cohort (‘Clalit’).
Label | Cohort size | Prevalence (%) | APS | auROC |
---|---|---|---|---|
Full population (HbA1c% < 6.5%) | 17,132 | 4.1 | 0.11 (0.10–0.11) | 0.75 (0.74–0.75) |
Normoglycemic population (HbA1c% < 5.7%) | 10,064 | 2 | 0.04 (0.04–0.05) | 0.69 (0.66–0.69) |
Prediabetes population (5.7% = <HbA1c% < 6.5%) | 7059 | 7.1 | 0.12 (0.12–0.13) | 0.68 (0.67–0.69) |
-
APS, average precision score; auROC, area under the receiver operating curve.
Predicting using feature domain groups.
Results of Gradient Boosting Decision Trees (GBDT) models for various feature domains.
Label | APS | auROC |
---|---|---|
All features without genetic sequencing | 0.28 (0.20–0.36) | 0.92 (0.89–0.94) |
All features | 0.27 (0.20–0.34) | 0.91 (0.89–0.93) |
All blood tests | 0.28 (0.21–0.36) | 0.90 (0.88–0.93) |
Four blood tests | 0.20 (0.14–0.27) | 0.88 (0.85–0.90) |
Blood tests without HbA1c% | 0.13 (0.09–0.18) | 0.84 (0.81–0.87) |
HbA1c% | 0.17 (0.12–0.23) | 0.84 (0.80–0.87) |
Blood tests without HbA1c% nor glucose | 0.10 (0.07–0.13) | 0.82 (0.79–0.86) |
Anthropometry | 0.07 (0.05–0.11) | 0.79 (0.75–0.82) |
Lifestyle and physical activity | 0.05 (0.04–0.07) | 0.73 (0.69–0.77) |
Blood pressure and heart rate | 0.05 (0.03–0.07) | 0.69 (0.64–0.73) |
Nondiabetes-related medication | 0.04 (0.03–0.06) | 0.67 (0.62–0.73) |
Mental health | 0.04 (0.03–0.06) | 0.67 (0.62–0.71) |
Family and ethnicity | 0.04 (0.03–0.05) | 0.66 (0.60–0.71) |
Diet | 0.04 (0.03–0.06) | 0.66 (0.60–0.71) |
Socio-demographics | 0.03 (0.02–0.05) | 0.65 (0.60–0.70) |
Early-life factors | 0.03 (0.02–0.05) | 0.64 (0.59–0.69) |
Age and sex | 0.03 (0.02–0.04) | 0.61 (0.56–0.67) |
Only genetics | 0.03 (0.02–0.04) | 0.57 (0.51–0.63) |
-
APS, average precision score; auROC, area under the receiver operating curve.
Summary of Incremental feature’s model.
Comparison table of average precision score (APS) and area under the receiver operating curve (auROC) for the Gradient Boosting Decision Trees (GBDT) models, where each model includes the preceding model’s features plus an additional feature domain. The largest increase in prediction accuracy was the result of adding the HbA1C% feature, which is also a biomarker for type 2 diabetes (T2D) diagnosis. Adding the DNA sequencing data did not significantly contribute to the prediction power of the model.
Label | APS | auROC |
---|---|---|
Age and sex | 0.03 (0.02–0.04) | 0.61 (0.56–0.67) |
HbA1c% | 0.17 (0.12–0.23) | 0.84 (0.80–0.87) |
Four blood tests | 0.20 (0.14–0.27) | 0.88 (0.85–0.90) |
All blood tests | 0.28 (0.21–0.36) | 0.90 (0.88–0.93) |
Adding anthropometrics | 0.23 (0.17–0.30) | 0.90 (0.87–0.92) |
Adding physical health DT | 0.28 (0.21–0.36) | 0.91 (0.89–0.93) |
Adding lifestyle DT | 0.24 (0.18–0.32) | 0.91 (0.88–0.93) |
Adding blood pressure and heart rate | 0.25 (0.19–0.33) | 0.91 (0.88–0.93) |
Adding non-T2D-related medical diagnosis | 0.24 (0.18–0.32) | 0.91 (0.88–0.93) |
Adding mental health | 0.28 (0.20–0.36) | 0.91 (0.89–0.93) |
Adding medication | 0.28 (0.20–0.35) | 0.91 (0.89–0.93) |
Adding diet | 0.24 (0.18–0.31) | 0.91 (0.89–0.93) |
Adding family-related information | 0.28 (0.21–0.35) | 0.91 (0.89–0.94) |
Adding early-life factors | 0.24 (0.17–0.31) | 0.91 (0.89–0.93) |
Adding socio-demographic | 0.27 (0.20–0.36) | 0.92 (0.89–0.94) |
Adding genetics | 0.27 (0.20–0.34) | 0.91 (0.89–0.93) |
Comparing models main results.
Label | Model type | APS | auROC | Deciles prevalence odds ratio |
---|---|---|---|---|
GDRS SA | Scoreboard | 0.04 (0.03-0.06) | 0.66 (0.62-0.70) | 11 (3.8-38) |
FINDRISC LR | Scoreboard | 0.04 (0.03-0.06) | 0.73 (0.69-0.76) | 33(9.6-67) |
Anthropometry | Scoreboard | 0.07(0.05-0.10) | 0.81(0.77-0.84) | 54(18-79) |
Anthropometry | Logistic regression | 0.09(0.06-0.13) | 0.82(0.78-0.84) | 54(18-80) |
Anthropometry | Cox regression | 0.10(0.07-0.13) | 0.82(0.79-0.85) | 69(27-89) |
Four blood tests | Scoreboard | 0.13(0.10-0.17) | 0.87(0.85-0.90) | 96(79-115) |
Four blood tests | Cox regression | 0.25(0.18-0.32) | 0.88(0.85-0.90) | 101(84-121) |
Four blood tests | Logistic regression | 0.24(0.17-0.31) | 0.88(0.85-0.91) | 104(84-125) |
Blood tests | Logistic regression | 0.26(0.19-0.33) | 0.91(0.89-0.93) | 116(95-138) |
All features DT | Boosting decision trees | 0.27(0.20-0.34) | 0.91(0.89-0.93) | 117(98-139) |
Label | Model type | APS | auROC | Decile’s prevalence odds ratio |
---|---|---|---|---|
Anthropometry | SA Scoreboard 5yrs | 0.04 (0.03-0.07) | 0.79 (0.75-0.83) | 8.8 (3.6-36) |
Anthropometry | SA Scoreboard 10yrs | 0.06 (0.04-0.09) | 0.79 (0.76-0.82) | 10 (4.6-32.9) |
Anthropometry | Scoreboard | 0.07 (0.05-0.10) | 0.81 (0.77-0.84) | 17.2 (5-66) |
Anthropometry | Logistic regression | 0.09 (0.06-0.13) | 0.81 (0.78-0.84) | 16.9 (4.8-66) |
Anthropometry | Cox regression | 0.10 (0.07-0.13) | 0.82 (0.79-0.85) | 10.7 (5-24) |
Four blood tests | SA Scoreboard 10yrs | 0.13 (0.10-0.16) | 0.87 (0.85-0.90) | 22.4 (9.8-54) |
Four blood tests | Scoreboard | 0.13 (0.10-0.17) | 0.87 (0.85-0.90) | 48 (11.9-109) |
Four blood tests | SA Scoreboard 5yrs | 0.09 (0.06-0.12) | 0.89 (0.86-0.92) | 53.2 (18.9-84.2) |
Four blood tests | Logistic regression | 0.24 (0.17-0.31) | 0.88 (0.85-0.91) | 32.5 (10.89-110) |
Four blood tests | Cox regression | 0.25 (0.18-0.32) | 0.88 (0.85-0.90) | 43 (13.6-109) |