Uncovering natural variation in root system architecture and growth dynamics using a robotics-assisted phenomics platform
Figures
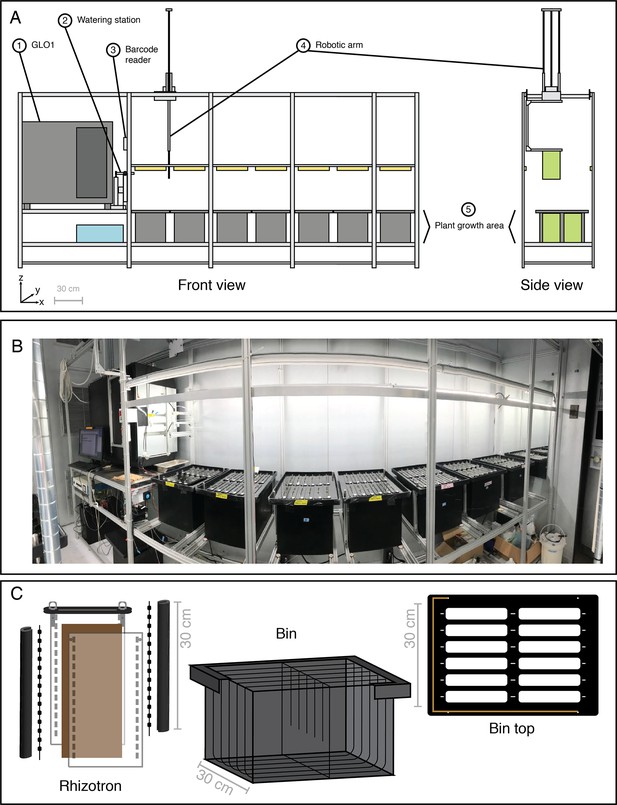
Growth and Luminescence Observatory for Roots (GLO-Roots) automation: GLO-Bot.
(A) Schematic of the GLO-Bot Cartesian gantry system includes: (1) The Growth and Luminescence Observatory 1 (GLO1) imaging system published in Rellán-Álvarez et al., 2015, which houses two cameras and a rotating stage for root imaging, (2) a station for general watering or treatment with diluted luciferin solution prior to imaging, (3) a barcode scanner to identify the rhizotron and load a specific watering and imaging protocol, (4) a robotic arm, which moves in the x-, y-, and z-directions and has a hook at the end to pick up rhizotrons, and (5) an area for plant growth, which can be seen in the photograph of GLO-Bot (B). (C) Automation updates required modification of the GLO-Roots growth vessel design to include a black acrylic plate and hooks for rhizotron handling as well as dividers within the growth boxes and guides along the bin top, which allow the rhizotron to hang and shield the roots from light. Copper tape along the edge of the bin top enables positioning. Gray-scale bars denote 30 cm.
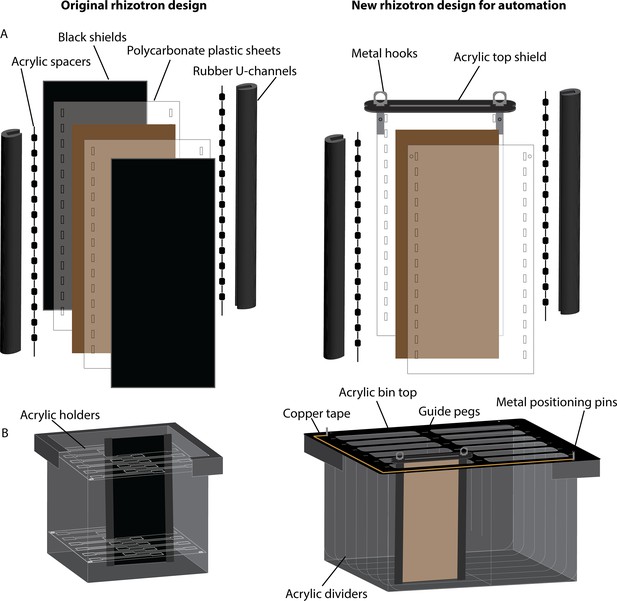
Detailed schematic of rhizotron design for automation.
(A) Rhizotron design changes between the original rhizotron design published in Rellán-Álvarez et al., 2015 (left) and new rhizotron for automation (right). The new design uses an acrylic top shield secured in place by metal hooks instead of the black shields to protect roots from light. (B) This light protection is possible since rhizotrons hang in the bin rather than stand in the bin. Guide pegs adhered to the bin top help the robotic arm position pick-up and drop off the rhizotrons while acrylic dividers within the bin provide additional light protection for neighboring rhizotrons if one rhizotron is removed from the bin. Copper tape and metal positioning pins are used by the robotic arm to detect the position of the bin and calculate the exact position of each rhizotron.
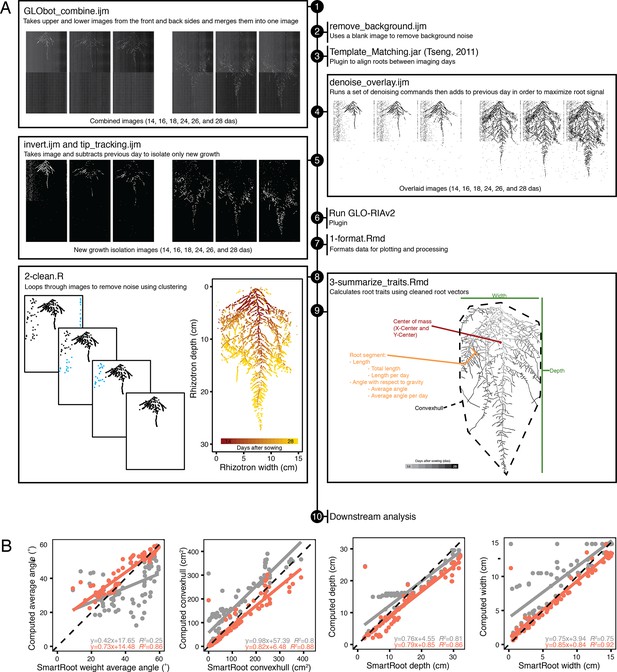
Workflow of image analysis pipeline that enables robust trait measurement.
(A) Sample workflow for processing time-series images from multiple days after sowing (das) starts by (1) combining raw images to merge front, back, upper, and lower images, (2) a blank image is then subtracted to remove inherent noise, (3) images are registered using the ImageJ plugin Template Matching and Slice Alignment to account for x- and y-movement caused by slight position changes during rhizotron insertion into the imager, (4) registered images are then de-noised and overlaid, which helps overcome luciferase signal loss in older root tissues; however, this step also compounds any background noise. (5) Image subtraction between each day removes this noise and isolates new growth. Processed images are run through GLO-RIAv2 (6) and the output is formatted, such that in silico vectorized roots can be reconstructed (7). Additional noise is cleaned up (8) using an iterative distance-clustering method. Traits are then extracted from cleaned roots (9) and the outputs can be used for downstream analysis (10). (B) Comparison of 85 images measured with previous root analysis methods (GLO-RIAv1, gray) and the new analysis methods (GLO-RIAv2, coral) vs. manually traced ground truth measurements from SmartRoot (x-axis) reveals that the new analysis method increases accuracy, as demonstrated by the r2 values, higher slopes, and lower y-intercepts.
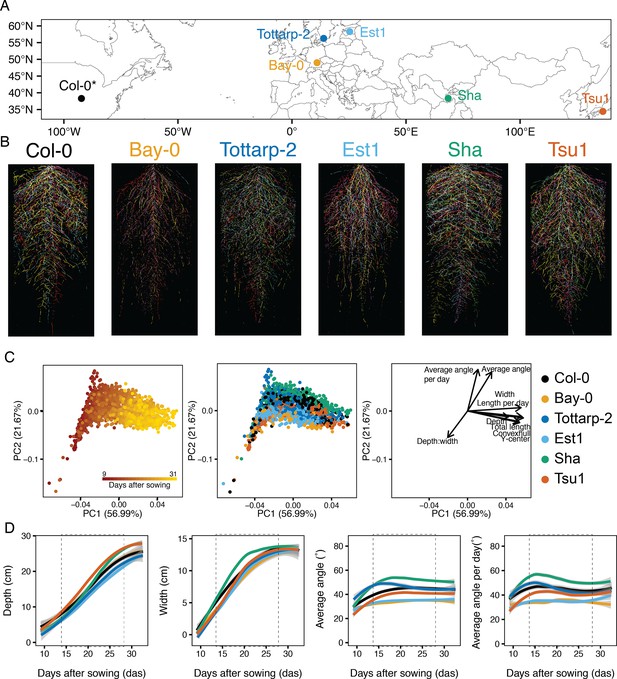
Root system architecture (RSA) of six Arabidopsis accessions over time.
(A) Six accessions from diverse locations were imaged continuously from 9 to 31 days after sowing (DAS). *Col-0 location reflects where accession was originated, not collected. (B) Each accession had a unique RSA pattern (five root systems per accession at 21 DAS each shown in a different color overlaid on top of each other). (C) Principal component analysis (PCA) using raw trait values for eight time points and nine traits shows time (red is 9 DAS and yellow is 31 DAS), as the largest source of variation (PC1) and angle and depth-to-width ratios further distinguishing the root systems (PC2). When colored by accession, this analysis shows separation between the accessions. Loading plot illustrates the impact of each trait on the overall variation. (D) Four root system raw traits for six accessions growing over time from 9 to 31 DAS. Trends were calculated using LOESS smoothing (n=12–15 plants at each time point). 95% confidence interval shown by gray shading. Non-smoothed raw data and additional traits are plotted in Figure 3—figure supplement 2. Two vertical dashed lines highlight the interval (14–28 DAS) chosen for future experiments. Depth and width measurements are constrained by the rhizotron size. Colors correspond to accessions in panel B.
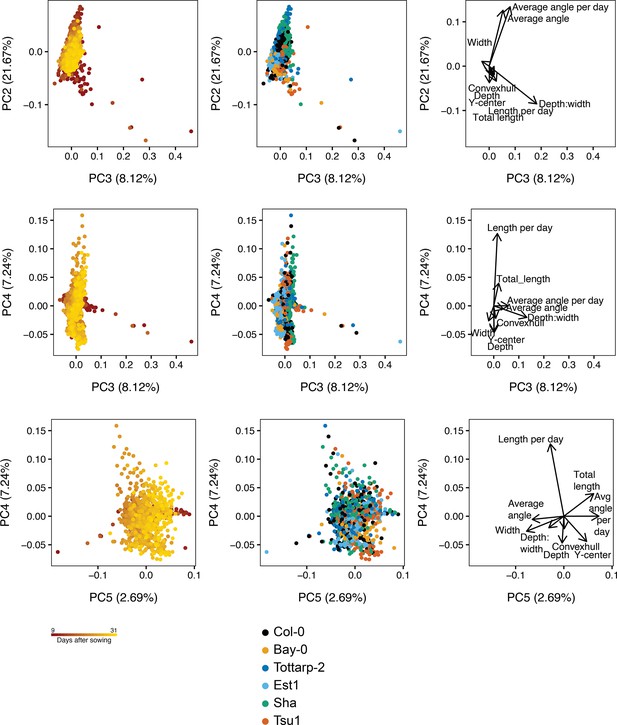
Principal component analysis (PCA) plots of nine root system architecture (RSA) traits for the six accessions.PCA using raw trait values for nine traits. Left plots show PCA colored by time (red is 9 DAS and yellow is 31 DAS), center shows PCA colored by accessions, and the right shows the loading plot to demonstrate the traits influencing each PC.
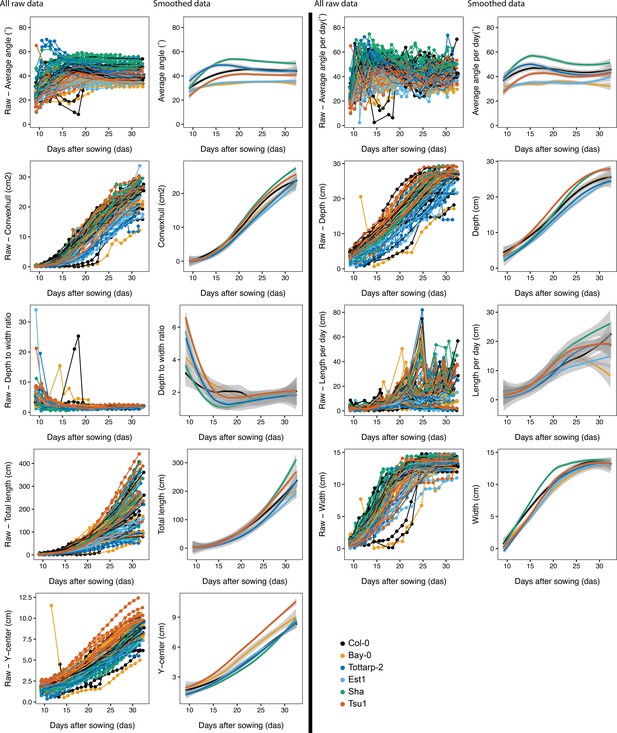
Root system architecture (RSA) traits over time for the six accessions.
All nine root system raw traits for the six Arabidopsis accessions growing over time from 9 to 31 DAS. All raw data plotted on the left, trends of the raw data, plotted on the right, were plotted using LOESS smoothing (n = 12-15 plants at each time point). 95% confidence interval shown by gray shading.
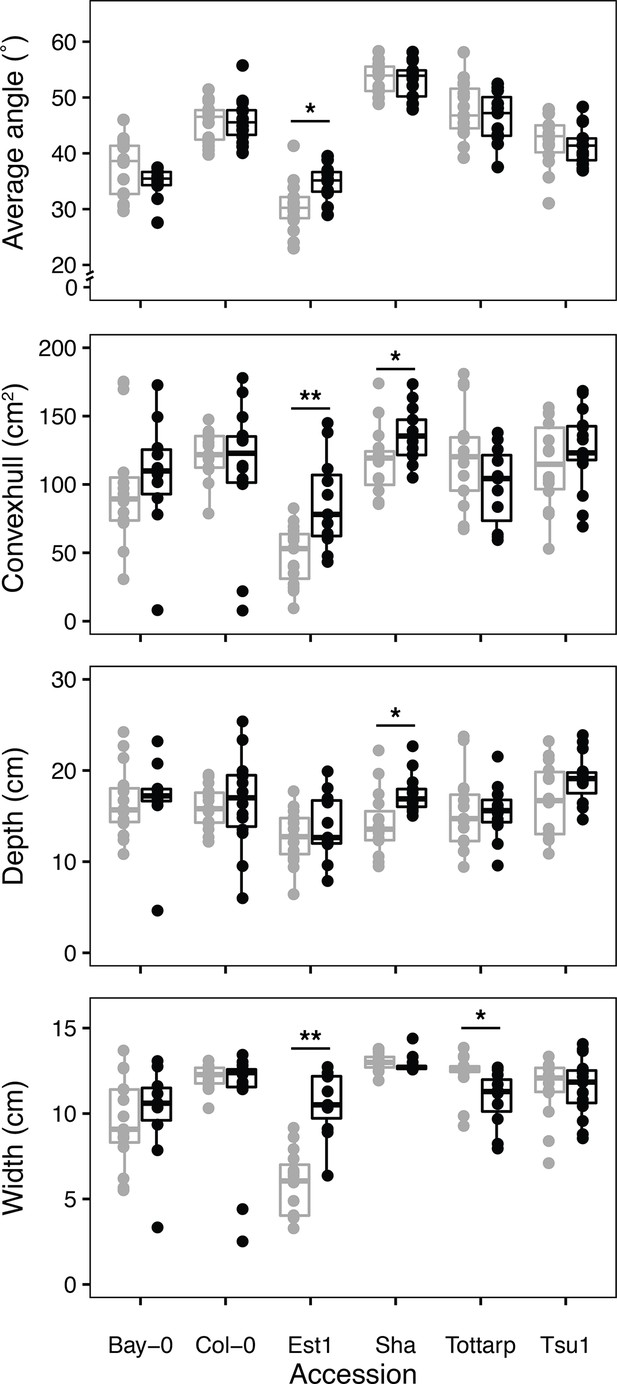
Continuous vs. single time point imaging.
Comparison between four raw traits measured on plants imaged and handled only once at 21 DAS (gray) and plants that were imaged and handled every day up until 21 DAS (black). Comparisons are shown as boxplots (n = 12-15 plants). Box boundaries represent the 25% to 75% percentile range and median value is indicated by the black line. Points beyond whiskers extend beyond 1.5 times the interquartile range. Asterisks indicate significance: *p < 0.05, **p < 0.01.
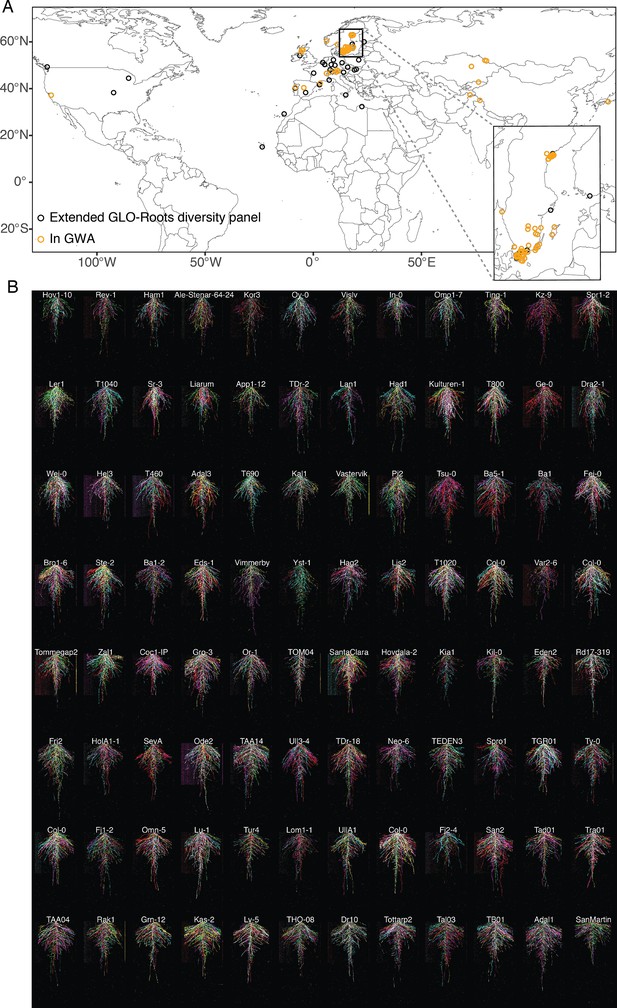
Root system architecture (RSA) of the Growth and Luminescence Observatory for Roots (GLO-Roots) diversity panel.
(A) Locations of all accessions used in this study. Inset highlights the concentration of accessions from the Swedish population. (B) RSAs of the GLO-Roots diversity panel used in the genome-wide association studies (GWAS) at 20 days after sowing (DAS). Six root systems overlaid on top of each other with root system color indicating each replicate. Root systems are arranged in order of median average angle of the root system from deepest in the upper left to shallowest in the bottom right.
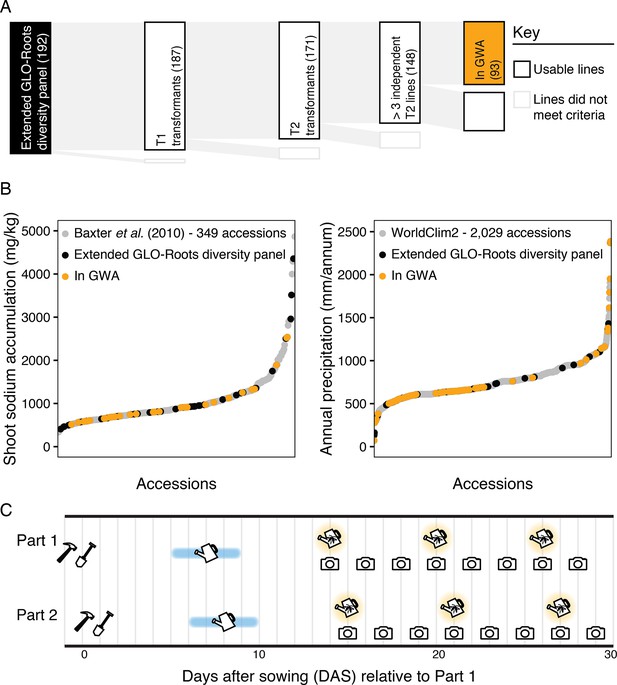
Overview of the Growth and Luminescence Observatory for Roots (GLO-Roots) diversity panel.
(A) Tracking of the transformation of 192 Arabidopsis accessions and final selection for the genome-wide association (GWA) population. (B) Both the extended GLO-Roots diversity panel and the GLO-Roots diversity panel used in the genome-wide association studies (GWAS) capture the published distribution of variation found among larger populations. (C) Schematic of GLO-Bot growth for the GLO-Roots diversity panel including the building (hammer), sowing (shovel), watering (blue watering can), luciferin watering (yellow firefly watering can), and imaging (camera), timeline for one replicate.
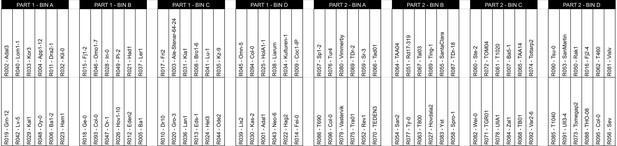
Growth and Luminescence Observatory for Roots (GLO-Roots) diversity panel rhizotron positioning.
At the start of each replicate rhizotrons were placed in growth boxes (bins) at specific locations. The 96 rhizotrons were imaged in two parts on two consecutive days.
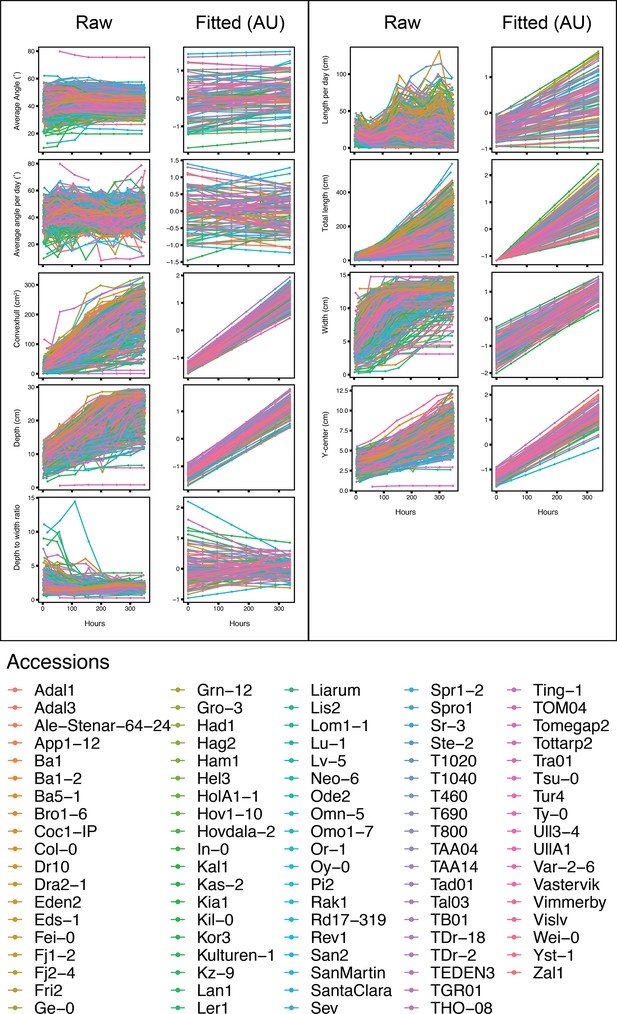
Raw vs. fitted values.
Raw (left) and fitted values (right) for all nine root traits. Colored by accession. Time represented in hours after the start of imaging: Hours 0, 48, 144, 192, 240, 288, and 336 are equivalent to 14, 16, 18, 20, 22, 24, and 28 days after sowing (DAS), respectively.
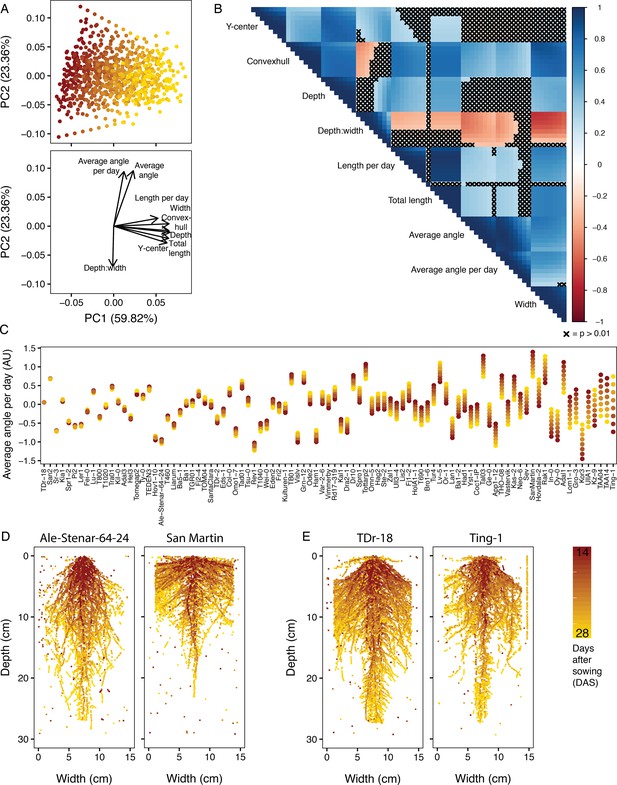
Trait relationships among the Growth and Luminescence Observatory for Roots (GLO-Roots) diversity panel.
(A) Principal component analysis (PCA) of nine fitted traits (i.e. fitted values) for the 93 accessions of the GLO-Roots diversity panel reveals traits influenced by time as the predominant source of variation within the dataset (PC1), while angle measurements and the depth-to-width ratio further distinguish accessions (PC2). (B) A correlogram of the fitted trait values at each time point, increasing left to right and top to bottom, further shows the negative correlations between the depth-to-width ratio and the angle traits and displays the relationships between all nine traits at each time point. Correlations where p>0.01 have been crossed out with an ‘X’. While some traits remain stable over time, other traits change over time. The extent to which a trait changes varies between accessions. (C) In some accessions, the fitted angle of new growth (average angle per day) remains constant each day, while in others the angle of new growth increases or decreases over time. The accessions are ordered based on variability of average angle per day with similar angles to the left and strikingly different angles on the right. (D) Ale-Stenar-64–24 and San Martin demonstrate contrasting root architectures, with opposite depth-to-width ratios and opposite average angles. (E) TDr-18 and Ting-1, on the other hand, are examples of accessions with constant and variable (respectively) average angle per day measurements.
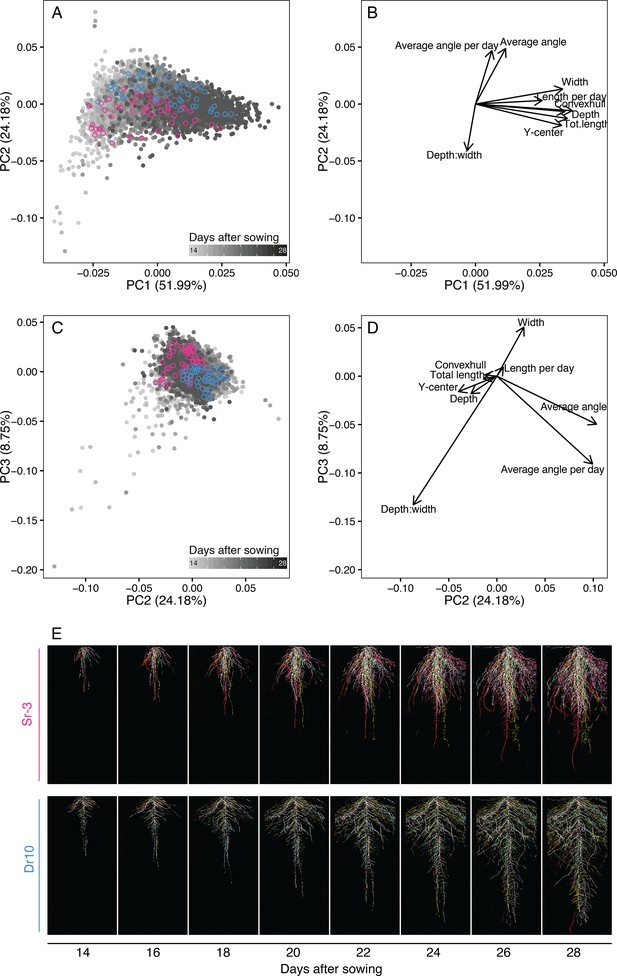
Principal component analysis (PCA) for nine root traits describing root system architecture (RSA).
(A–B) PCA of raw RSA traits for the 93 accessions in the Growth and Luminescence Observatory for Roots (GLO-Roots) diversity panel shows time and traits correlated with time as the primary axis of variation (PC1). (A–D) Angle and depth-to-width ratio are negatively correlated along PC2 and distinguish accessions with steep vs. shallow angles. (A, C) Two accessions, one with steep angles (pink) and the other with shallow angles (blue), are highlighted. (E) Composite images of these two accessions growing over time. Six root systems overlaid on top of each other with root system color indicating replicate.
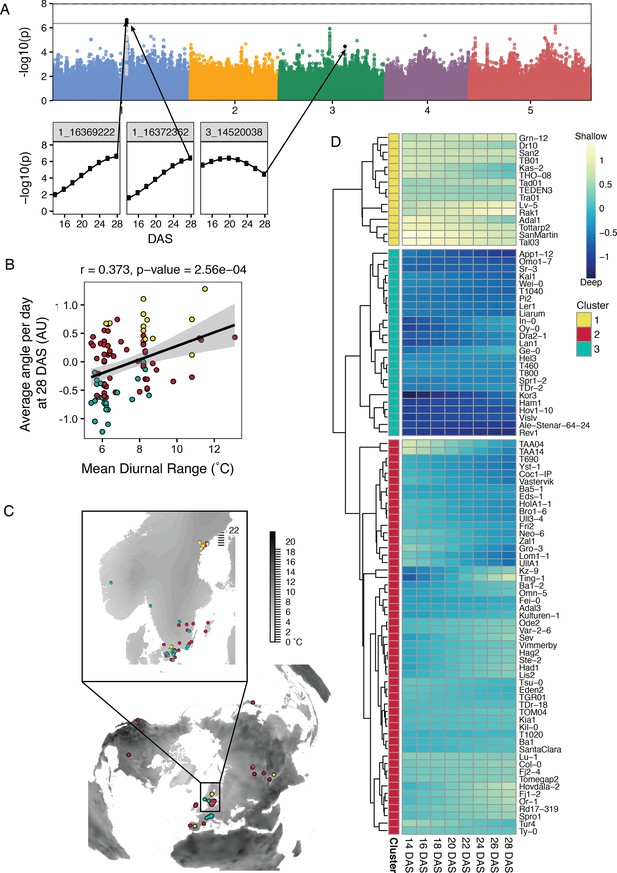
Genome-wide association studies (GWAS) and climate correlations of root traits of the Growth and Luminescence Observatory for Roots (GLO-Roots) diversity panel.
(A) Manhattan plot for average angle per day at 28 days after sowing (DAS). Black points indicate SNP positions that pass the Bonferroni threshold (solid line) at least once throughout the time series, as shown in the inset plots. Gray points are those in linkage disequilibrium (LD) with the significant SNPs. (B) Correlation between the average angle per day and the mean diurnal temperature range indicates the potential importance of root angle for surviving highly variable climates. (C) A world map showing mean diurnal range (black represents larger fluctuations) with the distribution of accessions (points). Points in (B) and (C) colored by cluster identity based on changes in average angle per day over time and computed by between-group average linkage hierarchical clustering with distance = 1.75, as shown in the heatmap (D). Heatmap depicts average angle per day for each day with blue indicating steeper angles and yellow indicating shallower angles. Changes in average angle per day divided the accessions into three clusters: the yellow cluster with consistently shallow root growth, the blue cluster with consistently deep root growth, and the red cluster with intermediate or changing root angles.
-
Figure 6—source data 1
Manhattan plots and SNP development of all nine root system architecture (RSA) traits.
(A) Manhattan plots of genome-wide association (GWA) run on each of the nine RSA traits at each time point with corresponding Q-Q plots (B). SNPs highlighted in black pass the threshold, gray indicates SNPs in linkage disequilibrium (LD). Genes in this region are reported in Supplementary file 4. (C) SNP development of the 29 unique SNPs that pass the Bonferroni threshold at least once throughout the time series.
- https://cdn.elifesciences.org/articles/76968/elife-76968-fig6-data1-v2.pdf
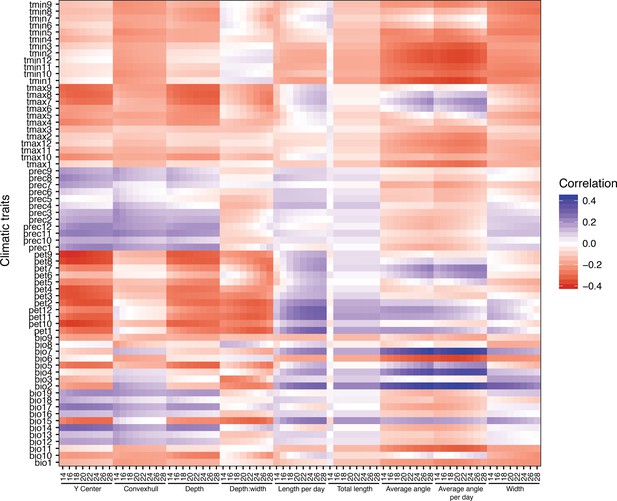
Correlations between average climatic variables and root traits.
Pearson correlations between average climatic traits and fitted root traits. We used minimum and maximum temperature for each month (1–12), total precipitation per month, and their composite Bio1–19 were averages in the period 1960–1990 (Fick and Hijmans, 2017). Potential evapotranspiration (pet) was calculated from the same data as described in Exposito-Alonso et al., 2019.
Videos
GLO-Bot running.
Root system architecture (RSA) growth of six Arabidopsis accessions over time.
Root system architecture (RSA) growth of Growth and Luminescence Observatory for Roots (GLO-Roots) diversity panel over time.
Additional files
-
Supplementary file 1
Analyzed root traits.
- https://cdn.elifesciences.org/articles/76968/elife-76968-supp1-v2.xlsx
-
Supplementary file 2
Accessions used for genome-wide association studies (GWAS).
- https://cdn.elifesciences.org/articles/76968/elife-76968-supp2-v2.xlsx
-
Supplementary file 3
Replicates with anomalies.
- https://cdn.elifesciences.org/articles/76968/elife-76968-supp3-v2.xlsx
-
Supplementary file 4
SNP list of 29 significant SNPs.
- https://cdn.elifesciences.org/articles/76968/elife-76968-supp4-v2.xlsx
-
Supplementary file 5
Broad-sense heritability of traits.
- https://cdn.elifesciences.org/articles/76968/elife-76968-supp5-v2.xlsx
-
Supplementary file 6
Removed images of the Growth and Luminescence Observatory for Roots (GLO-Roots) diversity panel.
- https://cdn.elifesciences.org/articles/76968/elife-76968-supp6-v2.xlsx
-
Transparent reporting form
- https://cdn.elifesciences.org/articles/76968/elife-76968-transrepform1-v2.docx