Disruption in structural–functional network repertoire and time-resolved subcortical fronto-temporoparietal connectivity in disorders of consciousness
Figures
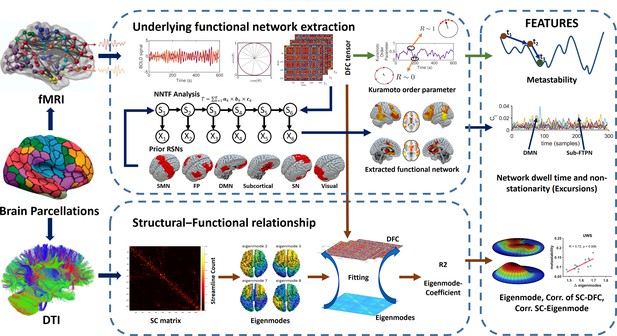
Overview of the analysis pipeline.
We used the same Shen parcellation for diffusion-weighted MRI (DWI) and functional magnetic resonance imaging (fMRI) data. Time-resolved functional connectivity was estimated using a metric for phase connectivity. A proxy measure for metastability was derived from the phase information. Time-resolved networks were subsequently extracted from the concatenated data from all subjects using non-negative tensor factorization (NNTF). Dwell times and nonstationarity (excursions from the median) were retrieved for each spatial pattern of functional connectivity (six resting-state networks and one ‘residual’ network). At the same time, time-resolved connectivity was predicted on a sample-by-sample basis based on a linear combination of eigenmodes (hidden patterns in the anatomical network). Measures were used for classification and feature ranking.
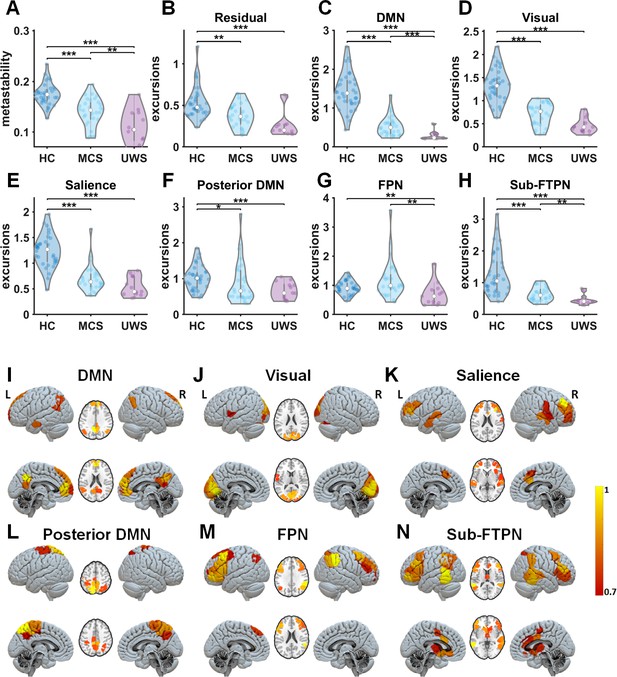
Metastability and time-resolved functional networks in disorders of consciousness (DoC).
(A) Metastability for all groups: healthy controls (HC), minimally conscious state (MCS), and unresponsive wakefulness state (UWS) patients. (B–H) Distributions of nonstationarity (excursions from the median) for the residuals and time-resolved networks. (I–N) Spatial patterns of time-resolved output networks. Abbreviations: DMN, default mode network; FPN, frontoparietal network; Sub-FTPN, subcortical fronto-temporoparietal network. *, ** and *** denote p < 0.05, p < 0.01 and p < 0.001, respectively (Mann–Whitney U tests). The colourbar indicates the strength of that specific area to the overall spatial pattern. Note, the Sub-FTPN appears as modified network from the initially assigned subcortical network. This Sub-FTPN consists of the following brain regions: bilateral thalamus, caudate, right putamen, bilateral anterior and middle cingulate, inferior and middle frontal areas, supplementary motor cortex, middle and inferior temporal gyrus, right superior temporal, bilateral inferior parietal, and supramarginal gyrus.
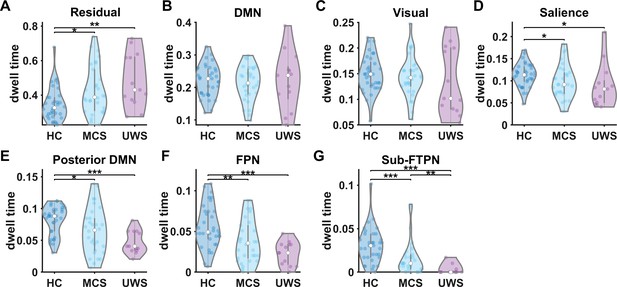
Dwell time of time-resolved functional networks in disorders of consciousness (DoC).
(A–G) Dwell time for all the networks for the three groups (healthy control [HC], minimally conscious state [MCS], and unresponsive wakefulness syndrome [UWS]). Dwell times for all states add up to one. Abbreviations: DMN, default mode network; FPN, frontoparietal network. *, ** and *** denote p < 0.05, p < 0.01 and p < 0.001, respectively.
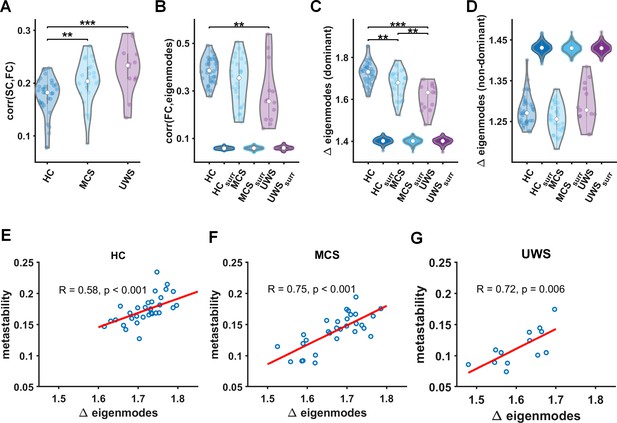
Relationship between time-resolved connectivity and eigenmodes.
(A) The prediction of static functional connectivity based on structural connectivity for all three groups (healthy control [HC], minimally conscious state [MCS], and unresponsive wakefulness syndrome [UWS]) in terms of the Pearson correlation coefficient. (B) The prediction of time-resolved functional connectivity based on eigenmodes. These distributions of eigenmode predictions are accompanied with predictions based on surrogate data. We further illustrate the level of fluctuations in eigenmode expression for all three groups (HC, MCS, and UWS) for dominant (reflecting network integration, C) and non-dominant eigenmodes (reflecting increasing network segregation, D) accompanied with results for surrogate data, ** and *** denote p < 0.01 and p < 0.001, respectively (Mann–Whitney U tests). (E–G) Metastability is strongly correlated to modulations in eigenmode expression within every group.
Additional files
-
Supplementary file 1
(A) Details about the patient population. (B) Brain regions involved in the extracted networks by non-negative tensor factorisation.
- https://cdn.elifesciences.org/articles/77462/elife-77462-supp1-v2.docx
-
MDAR checklist
- https://cdn.elifesciences.org/articles/77462/elife-77462-mdarchecklist1-v2.docx