Early immune markers of clinical, virological, and immunological outcomes in patients with COVID-19: a multi-omics study
Figures
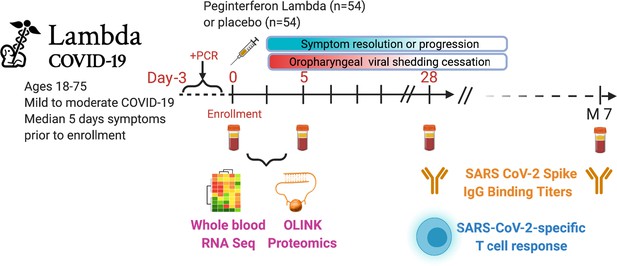
Study schema.
Outpatients (n=108) with PCR-confirmed severe acute respiratory syndrome-related coronavirus 2 (SARS-CoV-2) infection and swab obtained within 72 hr of randomization were enrolled in a phase 2 clinical trial of subcutaneous Peginterferon lambda vs. placebo. In-person follow-up visits were conducted at days 1, 3, 5, 7, 10, 14, 21, 28, and month 7 post-enrollment, with assessment of symptoms and vitals and collection of oropharyngeal swabs for SARS-CoV-2 testing. Blood obtained at days 0 and 5 were evaluated by whole blood transcriptomics (RNA sequencing), plasma proteomics (Olink), and SARS-CoV-2-specific antibodies. Clinical outcomes assessed included duration of symptoms and duration of virological shedding. Immunological outcomes assessed including SARS-CoV-2-specific T cell responses at day 28, and antibody responses at day 28 and month 7. Created with biorender.com.
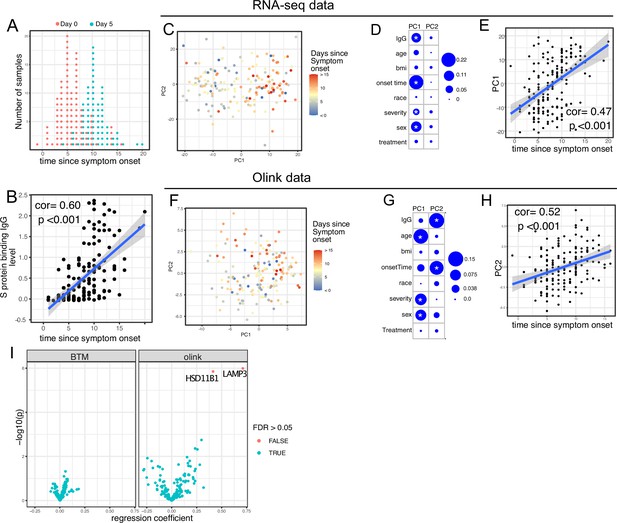
Transcriptomics and proteomics profiles correlate with the time to symptom onset in COVID-19 patients.
(A) The distribution of RNA-seq sample collection time in respect to symptom onset. The colors of the dots represent the sample collection time from the enrollment. Asymptomatic cases are not shown. (B) Scatter plot showing the positive correlation between severe acute respiratory syndrome-related coronavirus 2 (SARS-CoV-2) spike (S) protein-binding IgG antibody level and the time since symptom onset. Pearson correlation is reported. (C) Principal component analysis (PCA) plot of the RNA-seq samples. The colors of the dots represent the sample collection time from the enrollment. (D) The percent of the variances of PC1 and PC2 explained by different clinical variables. Stars indicate false discovery rate (FDR) <0.05. (E) Scatter plot showing the positive correlation between PC1 of the RNA-seq data and the time since symptom onset. Pearson correlation is reported. (F) PCA plot of the Olink proteomics data. The colors of the dots represent the sample collection time from the enrollment. (G) The percent of the variances of PC1 and PC2 explained by different clinical variables. Stars indicate FDR <0.05. (H) Scatter plot showing the positive correlation between PC2 of the Olink data and the time since symptom onset. Pearson correlation is reported. (I) Volcano plot showing the effect (measured as regression coefficient) and p value of Peginterferon lambda treatment on blood transcription modules and plasma proteins at day 5 post-treatment.
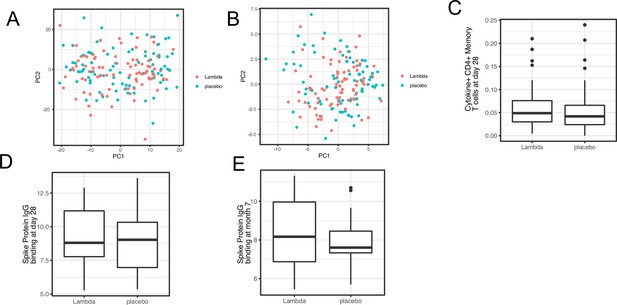
Immune profiles comparing Peginterferon Lambda vs. Placebo.
Comparing transcriptomics (A), proteomics (B), T cell responses at day 28 after enrollment (C), and antibody responses (at day 28 [D] and month 7 [E] after enrollment) between the two treatment arms.
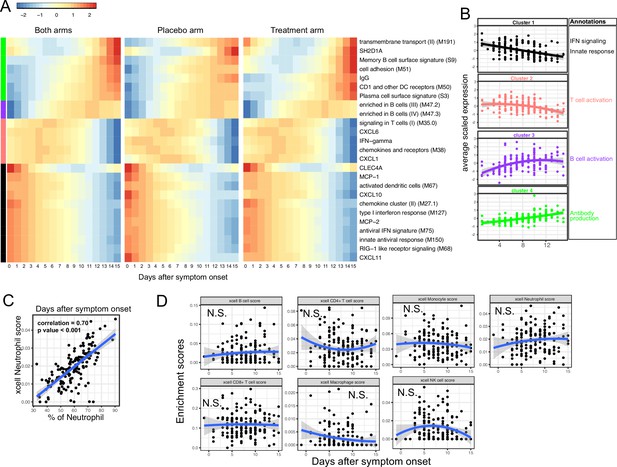
Trajectory analysis reveals sequential activation of immune modules in COVID-19 patients.
(A) The fitted expression level of immune modules and plasma proteins at 0–15 days after symptom onset. The values are calculated by fitting quadratic regressions and are scaled to a mean of 0 and an SD of 1. The left, middle, and right panels show the fitted trajectory using data from both arms, placebo arm only, and Peginteferon lambda arm only. Modules and proteins with false discovery rate (FDR) <0.05 (based on data from both arms) are shown. The color bar on the left side shows the clustering membership of the modules and plasma proteins. (B) The average trajectory of the clusters. We scaled the expression level of each module and plasma proteins to a mean of 0 and an SD of 1. We then calculated the average-scaled expression of all members in the clusters. Each dot represents the mean expression in each blood sample. The lines represent the fitted quadratic regression. The gray areas represent the 95% CI. (C) We estimated the Spearman correlation between the neutrophil enrichment score using the xCell. The plot shows the correlation between the xCell score and the counted neutrophil percentage in whole blood. (D) The relationship between xCell enrichment score and days after symptom onset.
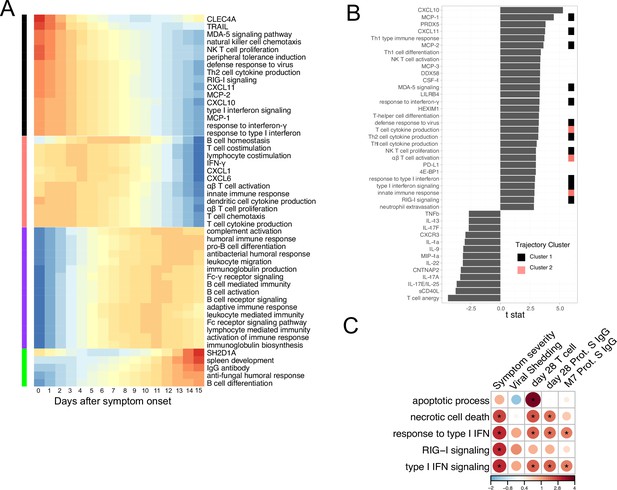
Gene Ontology analysis of immune profiles in COVID-19 outpatients.
(A) Characterizing the trajectory of plasma proteins and Gene Ontology pathways in COVID-19 patients. (B) The association between immune measures (plasma proteins and Gene Ontology pathways) and disease progression. We fitted regression models to test the relationship between the immune measurements and disease progression while controlling for the time after symptom onset. The t values for the disease progression term are reported. All measurements shown are significantly associated with disease progression (false discovery rate [FDR] <0.05) (C) Association between COVID-19 patient outcomes and the Gene Ontology pathways that are related to interferon signaling and cell death. Stars indicate FDR <0.05.
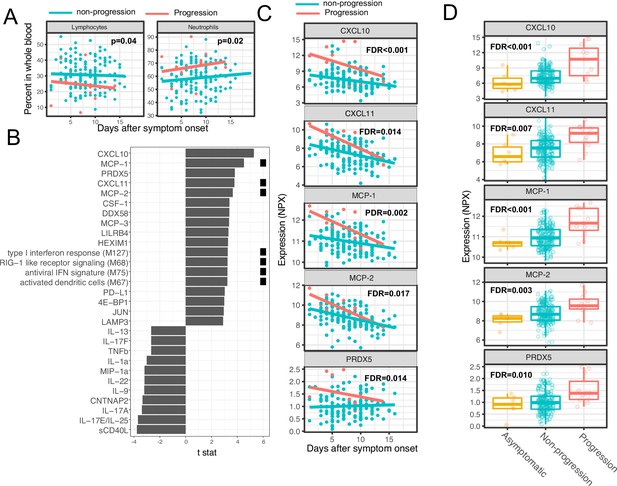
Variations in early immune responses are associated with disease severity in COVID-19 patients.
(A) Scatter plot comparing the percentage of lymphocytes and neutrophils in whole blood between moderate and severe cases. The lines represent the fitted linear relationship between the percentages and the time after symptom onset. We fitted regression models to test the relationship between the immune measurements and disease progression while controlling for the time after symptom onset and treatment. The p values for the disease progression are reported. (B) We fitted regression models to test the relationship between the immune measurements and symptom severity while controlling for the time after symptom onset. The bar plot shows the t score of the regression coefficient for disease progression. The colored squares represent the clusters each immune measurement belongs to. The clusters are defined in Figure 3A. False discovery rate (FDR) represents the p value of the regression coefficient of the disease progression term after multiple testing adjustment. (C) Scatter plot comparing the plasma protein levels between moderate and severe cases. The lines represent the fitted linear relationship between the percentages and the time after symptom onset. The top five significant proteins are shown. Data from asymptomatic cases are omitted, as their symptom onset time was unknown. FDR are the same as in B. (D) Box plots comparing the plasma protein levels between asymptomatic, non-progressed, and progressed cases. FDR represent the p value from one-way ANOVA after multiple testing adjustment.
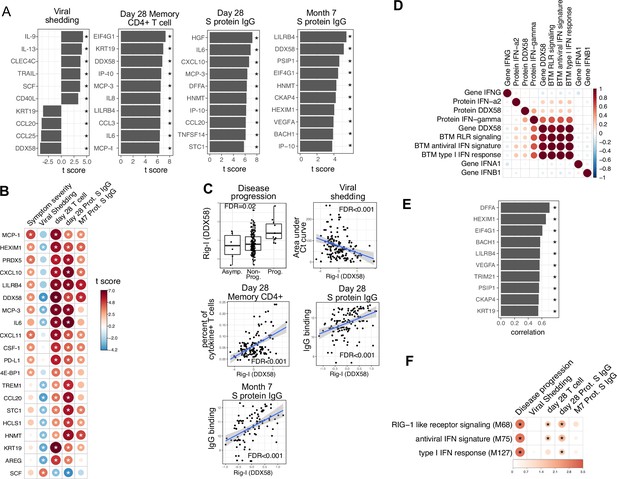
Plasma RIG-I is a biomarker for disease progression, viral shedding, T cell activity, and spike (S)-binding IgG levels.
(A) The association between plasma proteins and viral shedding, memory T cell activity, and anti-S-binding IgG levels. Memory CD4+ T cell activities are measured by the percent of cytokine positive T cells (TNF-α+ or TNFγ+ or IL21+) after S protein stimulation. T cells are collected from patients 28 days after enrollment. S protein-binding IgG levels are measured 28 days or 7 months after enrollment. We fitted regression models to test the relationship between the immune measurements and viral shedding, memory T cell activity, and S protein-binding IgG level while controlling for the time after symptom onset and treatment. The bar plot shows the t score of the regression coefficient for viral shedding, memory T cell activity, and anti-S-binding IgG levels. (B) Association between plasma proteins and multiple outcomes. The heatmaps include immune measurements that are significantly associated (indicated by stars) with at least three outcomes. (C) Correlation between plasma RIG-I (DDX58) and disease progression, viral shedding, memory T cell activity, and S protein-binding IgG levels. (D) Correlation between plasma RIG-I protein and selected level of plasma proteins, genes, and blood transcription modules (BTMs). (E) The top 10 plasma proteins correlated with plasma RIG-I protein. (F) The association between RIG-I and IFN related BTMs and the outcomes of COVID-19 patients. Stars represent false discovery rate (FDR) <0.05.
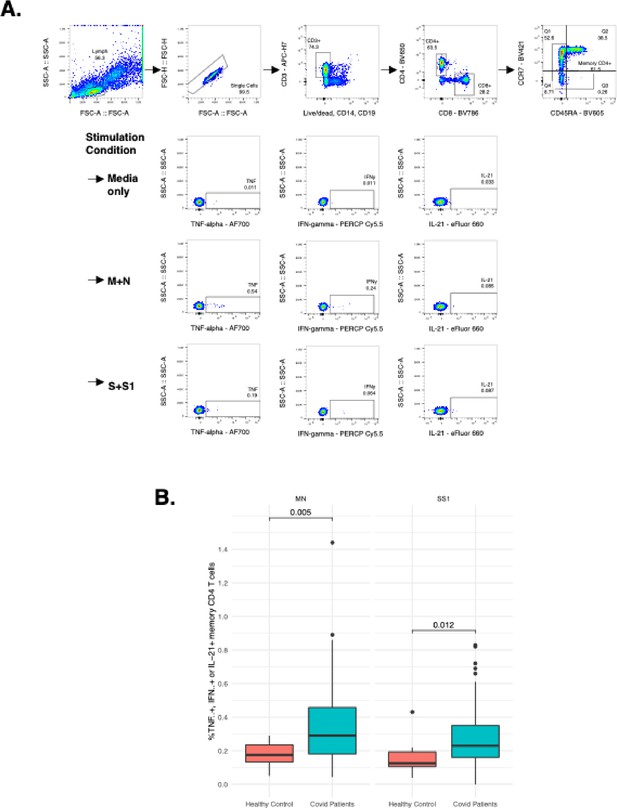
Measurement of severe acute respiratory syndrome-related coronavirus 2 (SARS-CoV-2) specific T cell responses by intracellular cytokine staining.
(A) Gating strategy to identify SARS-CoV-2-specific memory CD4+ T cells responsive to membrane (M), nucleocapsid (N), or spike (S and S1) immunodominant peptides by detection of intracellular cytokines TNF-alpha, IFN-gamma, or IL-21. (B) Comparison of SARS-CoV-2-specific T cell responses producing either TNF-alpha, IFN-gamma, or IL-21 following MN or SS1 stimulation compared to uninfected, healthy age-matched controls.
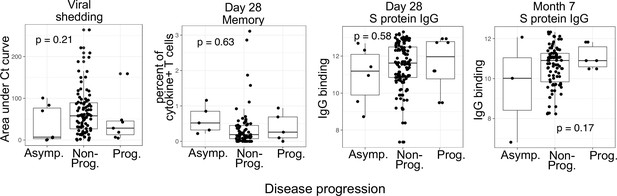
Boxplots visualizing the relationship between disease progression and other COVID-19 outcomes (viral shedding, memory T cell activity, and anti-spike-binding IgG levels).
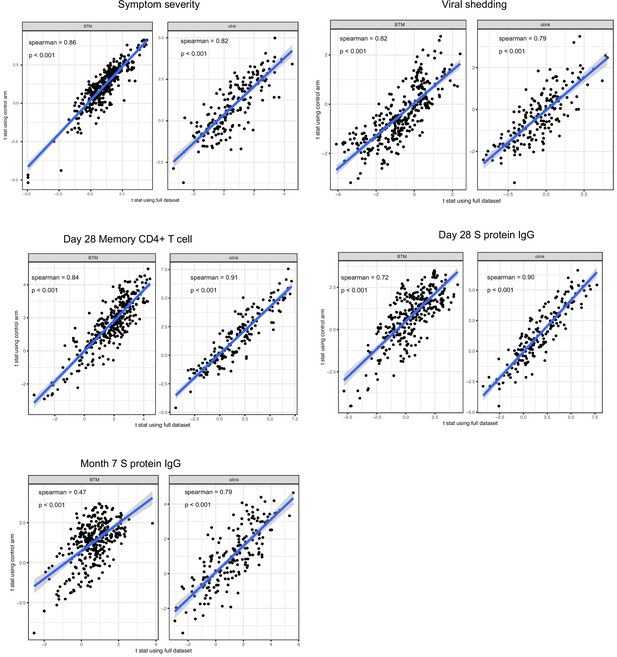
We estimated the association between immune measures (blood transcription module [BTM] and Olink proteins measures) and COVID-19 outcomes using data from both treatment arms and from only placebo arm of the lambda trial.
The scatter plots compare the two sets of the results.
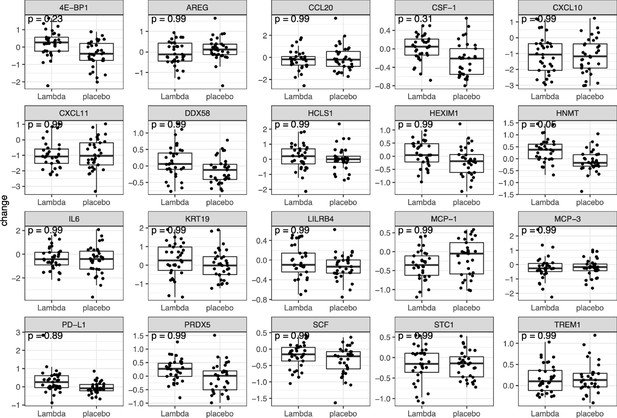
We performed t tests to compare the change of the 20 most relevant (as listed in Figure 5B) plasma proteins between day 0 and day 5 of the study.
p Value are adjusted for multiple hypothesis testing using the false discovery rate (FDR) method.
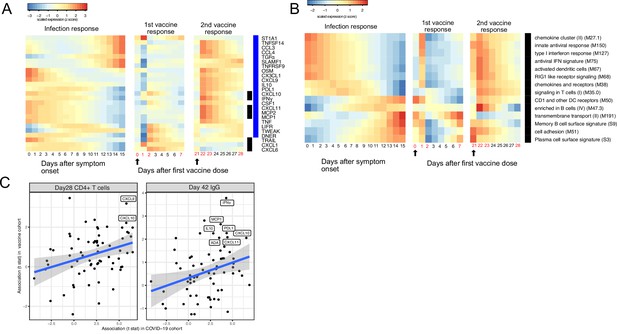
Comparing the immune response induced by severe acute respiratory syndrome-related coronavirus 2 (SARS-CoV-2) infection and COVID-19 vaccine (BNT162b2).
(A–B) The heat map shows the expression level of plasma proteins (A) and blood transcription modules (BTMs) (B) at 0–15 days after symptom onset in COVID-19 patients (left), 0–7 days after the first dose of vaccination, and 21–28 days after the second dose of vaccination in healthy individuals (right). The values from the SARS-CoV-2 dataset are calculated by fitting quadratic regressions and are scaled to a mean of 0 and an SD of 1. The values from the vaccination dataset are computed by fitting using linear interpolation between the measured time points (days in red color) and are scaled to a mean of 0 and an SD of 1. The black bar on the right side shows the protein markers that are significantly associated with time in COVID-19 patients (false discovery rate [FDR] <0.05). The blue bar on the right side shows the protein markers that are significantly associated with time after vaccination (FDR <0.05). The black arrows indicate the time of the first and the second doses of vaccination. (C) Comparing the biomarkers of immune outcomes in COVID-19 dataset and the BNT162b2 dataset. We fitted regression models to test the association between the highest level of protein markers after first vaccination (day 0 and day 21) and the T cell (left) and antibody responses (right). The t statistics from the regression model is compared with the t statistics from the COVID-19 dataset.
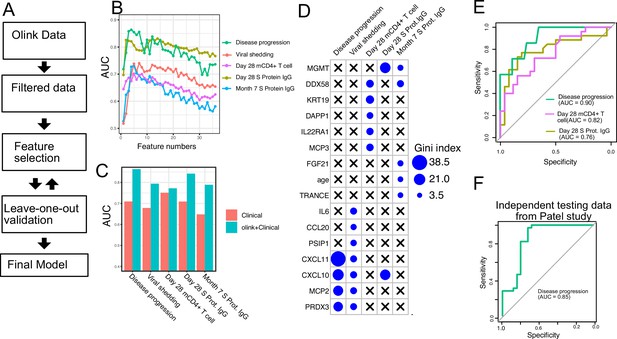
Plasma protein markers predict disease progression, T cell response, and spike (S) protein-binding IgG level in COVID-19 patients.
(A) Machine-learning procedure for predicting COVID-19 patient outcomes using Olink proteomics data collected at day 0. (B) Random forest models were built to predict symptom severity, S protein-binding IgG level at 28 days and 7 months after enrollment, and cytokine+ memory CD4+ T cells 28 days after enrollment. The plot shows the leave-one-out cross-validation performance (measured by AUC of the ROC) achieved by random forest models with different numbers of features. (C) The leave-one-out cross-validation performance of the best-performing models and the models using demographical data (age and sex) only. (D) Feature importance of the final random forest models for predicting symptom severity, S protein-binding IgG level at 28 days and 7 months after enrollment, and cytokine+ memory CD4+ T cells at 28 days after enrollment. (E) We generated an independent dataset using samples obtained from 64 COVID-19 patients enrolled in the placebo arm of a clinical trial of favipiravir. The performance of the machine-learning models was tested using the new dataset. (F) We used the final model to predict severe cases in an independent dataset and measured the performance of the model measured by the ROC curve.
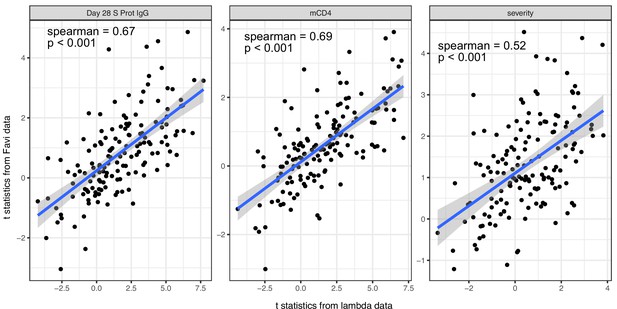
We estimated the association between immune measures (blood transcription module [BTM] and Olink proteins measures) and COVID-19 outcomes using data from both lambda trial and an independent dataset from Favipiravir trial.
The scatter plots compare the two sets of the results.
Additional files
-
Supplementary file 1
Characteristics of study participants (lambda study).
- https://cdn.elifesciences.org/articles/77943/elife-77943-supp1-v2.docx
-
Supplementary file 2
Statistical analysis of the trajectory analysis of early stage immune measures in COVID-19 patients.
- https://cdn.elifesciences.org/articles/77943/elife-77943-supp2-v2.csv
-
Supplementary file 3
Association between early stage immune measures in COVID-19 patients and their clinical outcomes.
- https://cdn.elifesciences.org/articles/77943/elife-77943-supp3-v2.csv
-
Supplementary file 4
Intracellular cytokine staining (ICS) antibody panel.
- https://cdn.elifesciences.org/articles/77943/elife-77943-supp4-v2.docx
-
Supplementary file 5
Characteristics of validation study participants (placebo arm and Favipiravir trial).
- https://cdn.elifesciences.org/articles/77943/elife-77943-supp5-v2.docx
-
MDAR checklist
- https://cdn.elifesciences.org/articles/77943/elife-77943-mdarchecklist1-v2.docx