A dynamic and expandable digital 3D-atlas maker for monitoring the temporal changes in tissue growth during hindbrain morphogenesis
Figures
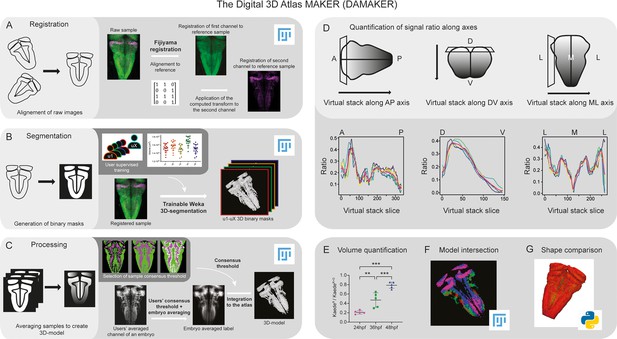
Image analysis pipeline (DAMAKER) and digital 3D-atlas construction.
Schematic depiction of sample processing through the digital 3D-atlas making pipeline. (A) For image registration, acquired dual channel images from embryos are registered using the Fijiyama registration plugin in FIJI, the first channel (HuC:GFP signal in green) is aligned to a previously selected HuC signal which serves as reference orientation. The resulting transform file is then used to align the signal of interest (e.g. gad1b:DsRed displayed in magenta). (B) For image segmentation, Tg[HuC:GFP] embryos are used for supervised training of the Trainable Weka 3D-Segmentation algorithm. Images are provided to users, and each user inputs brush strokes on areas with signal and areas considered as background across the stack, in order to obtain a classifier (e.g. a set of filters and thresholds to be used by the algorithm to isolate the signal). Users’ generated classifiers are compared by quantification of a dozen of HuC-signals from various embryos. User classifiers resulting in more than 10% variability among users (ANOVA test) are considered outliers (e.g. u4, user 4) and are not used for sample segmentation. For each embryo, as many 3D-binary masks as user-generated classifiers are created. (C) For processing, 3D-binary masks of a single embryo are averaged to obtain a users’ average segmentation; this allows to assess the interuser segmentation variability and limits the human bias introduced by the supervised training. Signal similarity threshold between users can then be selected (here, consensus among all users was isolated). Users’ average embryo 3D-models of a given condition (n=5) are then averaged together to obtain the embryo’s averaged label. To select sample consensus threshold, we compare embryos’ averaged labels at different thresholds with their negative imprint onto the neuronal differentiation domain. Signal consensus among at least three embryos seems to recapitulate best the original signal, with marginal overlap between the signal and its imprint. Sample consensus threshold is then applied to the embryos’ averaged label to generate the final 3D-model, ready for the integration into the atlas. Several functions can be performed with DAMAKER such as (D) quantification of the signal ratio along body axes by virtually reslicing embryos’ averaged models along the desired axis, (E) quantification of users’ averaged label volumes and display them as a ratio of signal over the neuronal differentiation domain, (F) intersection of the 3D-model integrated in the atlas to assess overlapping or segregation of signals, and (G) analysis of the tissue shape.
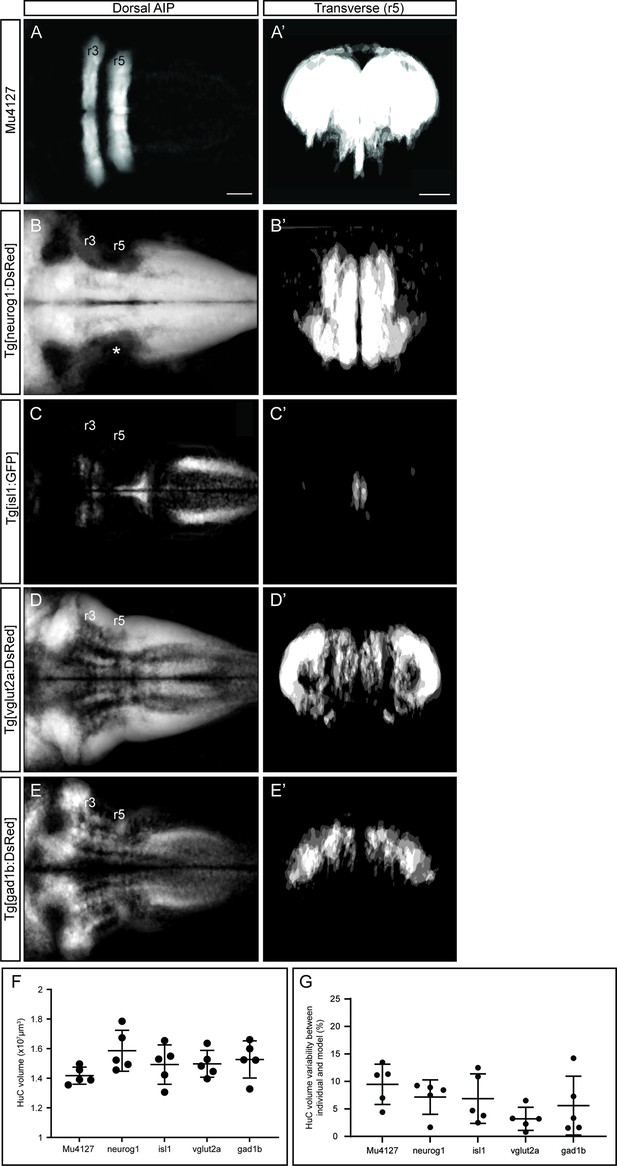
Analyses of interindividual signal variability.
(A–E) Dorsal average intensity projections (AIP) with anterior to the left, and (A’–E’) transverse views through r5 overlaying different 72hpf embryos of the indicated transgenic lines (n=5 embryos each). Interindividual variability is displayed as a gray scale. Comparison of average signal was done prior to threshold among individuals. (F) Dot-plot displaying the quantification at 72hpf of the volume of the HuC signal (x107, μm3) in the different transgenic combinations. Tg[Mu4127;HuC:GFP] as Mu4127; Tg[neurog1:DsRed;HuC:GFP] as neurog1; Tg[isl1:GFP;HuC:KaedeRed] as isl1; Tg[vglut2a:DsRed:HuC:GFP] as vlgut2a; and Tg[gad1b:DsRed:HuC:GFP] as gad1b. Each dot corresponds to a different embryo (n=5 embryos each). Note that the HuC volume is comparable among different transgenic lines. Kruskal-Wallis ANOVA test followed by a Dunn’s multiple comparison test; p>0.2. (G) Differences between individual segmented embryos and the resulting HuC volume 3D-models. Results are plotted as the percentage of volume difference from the model. Note that this difference never exceeded 10%. Scale bar, 50μm.
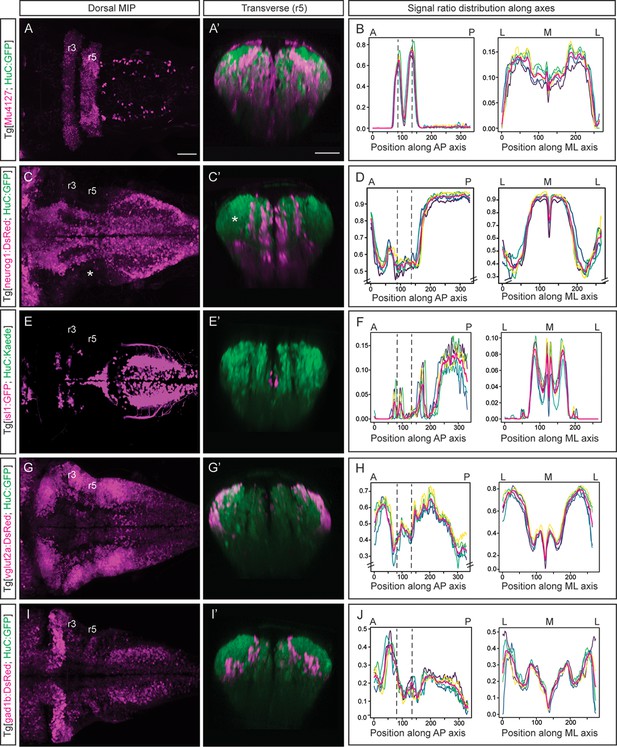
Incorporating territory landmarks allows precise spatial characterization of signals.
(A, C, E, G, I) Dorsal maximal intensity projections (MIP) with anterior to the left, and (A’, C’, E’, G’, I’) transverse views through r5 of registered embryos, in the Tg[HuC:GFP] background (72hpf). (A, C, E, G, I) display only the magenta signals such as r3 and r5 (Mu4127), neurog1, isl1, vglut2a and gad1b, whereas (A’, C’, E’, G’, I’) display these signals and the HuC (green). Scale bar, 50μm. (B, D, F, H, J) Signal ratio distribution along the anteroposterior (AP) and mediolateral (ML) axes of different individual embryos (n=5; colored lines), with the average value displayed as magenta line. Position along the AP and ML body axes is indicated on the top of the graph. Black dashed lines parallel to the Y-axis correspond to r3 and r5 positions assessed by Mu4127 signal. Note in (D, H) the offset in the Y-axis for easier readability of the signal variations. Scale bar, 50μm.
3D-models of rhombomeres 3 and 5, and neurog1-derivatives.
(A) Mu4127 3D-model displayed as 360° rotation. Note the volume that the domain occupies corresponds to r3 and r5. (B) neurog1 3D-model displayed as 360° rotation, showing the neurog1-derivatives.
3D-models of different neuronal populations.
(A–C) Motoneurons (isl1), glutamatergic neurons (vglut2a), and GABAergic neurons (gad1b) 3D-models, displayed as 360° rotation. (D) Overlay of the three 3D-models color-coded according to the specific neuronal population as in (A-C), displayed as 360° rotation.
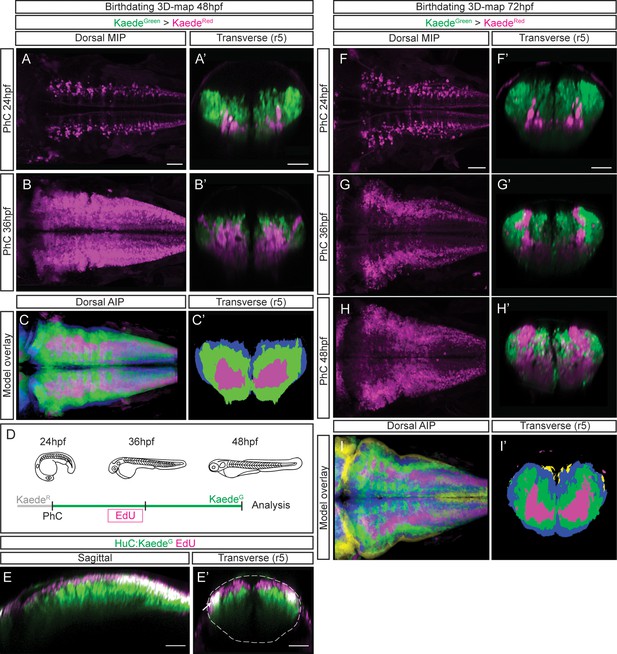
Differentiated neurons spatially organize according to their birthdate.
(A–B) Dorsal maximal intensity projections (MIP) showing only the HuC:KaedeRed, and (A’–B’) transverse views through r5 displaying both HuC:KaedeGreen and HuC:KaedeRed at 48hpf. HuC:KaedeGreen was photoconverted at the indicated times (PhC). Note that HuC:KaedeRed is displayed in neurons born before the time of photoconversion, and HuC:KaedeGreen in neurons born after. (C–C’) Neuronal birthdate 3D-map at 48hpf resulting from the intersection of photoconversion 3D-models. Neurons produced before 24hpf are depicted in magenta, those produced between 24hpf and 36hpf are in green, and the blue regions display neurons produced between 36hpf and 48hpf (n=5 embryos each). (D) Scheme depicting the photoconversion experiment followed by EdU-treatment. HuC:KaedeGreen was photoconverted at 24hpf, embryos were let to develop, pulsed with EdU for 6hr at 30hpf, and imaged at 48hpf. (E–E’) Tg[HuC:KaedeGreen] embryo displaying EdU (magenta) and HuC (green) in sagittal and transverse views, respectively. Note that EdU-cells are within the most dorsal part of the hindbrain, specifically in the progenitor domain and in the dorsal HuC domain (E’, see white arrow). The contour of the neural tube in (E’) is indicated with a white dashed line. (F–H) Dorsal maximal intensity projections (MIP) showing only the HuC:KaedeRed, and (F’–H’) transverse views through r5 displaying both HuC:KaedeGreen and HuC:KaedeRed at 72hpf. HuC:KaedeGreen was photoconverted at the indicated times (PhC). (I–I’) Neuronal birthdate 3D-map at 72hpf resulting from the intersection of photoconversion 3D-models. Neurons produced before 24hpf are depicted in magenta, those between 24 and 36hpf in green, the ones between 36 and 48hpf in blue, and neurons produced between the last photoconversion (48hpf) and the time of acquisition (72hpf) in yellow (n=5 embryos each). Dorsal and sagittal views display anterior to the left. Scale bar, 50μm.
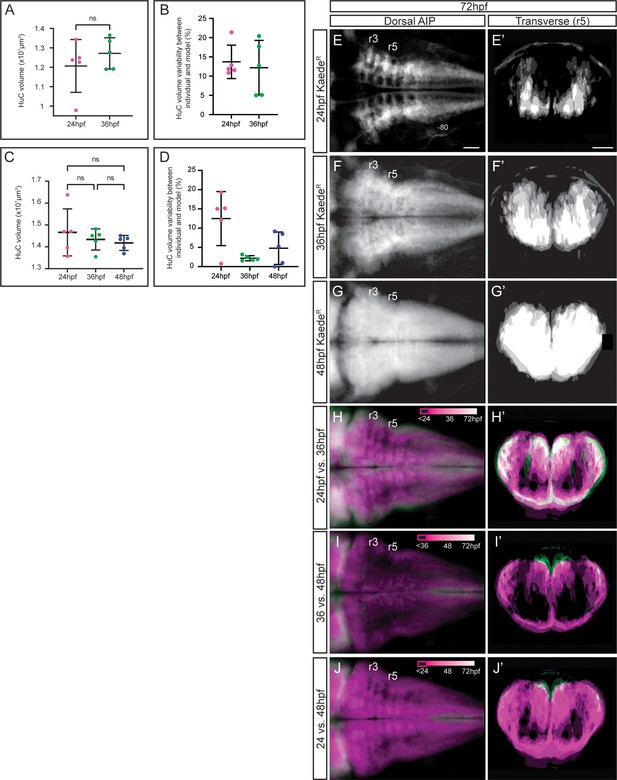
Interindividual variability analyses of the birthdating 3D-maps.
(A, C) Total HuC volume at 48hpf and 72hpf, respectively, of Tg[HuC:Kaede] embryos in which KaedeGreen was photoconverted at the indicated stages (each dot corresponds to an embryo; n=5 embryos each). Note that there are no significant differences in the HuC volumes between embryos. (A) Welch’s two-tailed t-test, and (C) ordinary one-way ANOVA followed by Tukey’s multiple comparison test; p>0.2. (B, D) Difference between individual segmented embryos at 48hpf and 72hpf and their resulting HuC volume 3D-models. Results are plotted as the percentage of volume difference from the 3D-model. Note that they do not exceed 15% in any of the cases. (E–G) Dorsal average intensity projections (AIP) with anterior to the left and (E’–G’) transverse views through r5, resulting from the overlay of different users’ averaged Tg[HuC:Kaede] embryos at 72hpf, in which KaedeGreen was photoconverted at the indicated stages (n=5 embryos each). Interindividual variability is displayed as a gray scale. (H–J, H’–J’) 3D-models of Tg[HuC:Kaede] embryos acquired at 72hpf showed as dorsal AIP or transverse views, in which HuC:KaedeGreen was photoconverted at 24 and 36hpf (H–H’), at 36 and 48hpf (I–I’) or at 24 and 48hpf (J–J’), and overlaid on each other. Neurons produced before the earliest of the two photoconversions are depicted in black, those produced in between the two times of photoconversion are in magenta, and the neurons produced from the last time of photoconversion and the time of acquisition in white. Scale bar, 50μm.
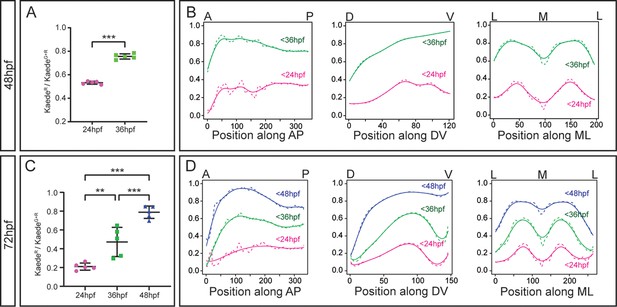
Quantification of the neuronal growth volume according to cell birthdate.
(A, C) Quantification of the relative contribution of different birthdated neurons to the neuronal differentiation domain at 48hpf and 72hpf, respectively. The ratio of early-born neurons over the total neuronal differentiation domain was plotted for each individual embryo (n=5 embryos each). (A) Welch’s two-tailed t-test, and (C) ordinary one-way ANOVA followed by Tukey’s multiple comparison test; p<0.002 (**) p<0.001 (***). (B, D) Relative contribution of late-born and early-born neurons along the anteroposterior (AP), dorsoventral (DV) and mediolateral (ML) axes. X-axis displays the number and position of virtual slices along the given body axis. The ratios of early-born neurons over the total neuronal differentiation domain were plotted as mean (dashed line) and non-linear regression (solid line). Color-coded lines correspond to the neurons born at the indicated embryonic stages (magenta, green and blue, born before 24hpf, 36hpf, and 48hpf, respectively). Position along the axes is indicated on the top.
The temporal registration 3D-map at 48hpf.
3D-model of the neuronal differentiation domains contributed by neurons born between 24 and 36hpf (A, green), and between 36 and 48hpf (B, blue), displayed as 360° rotation. These 3D-models were obtained by subtracting the model of the HuC domain photoconverted at 24hpf to the model of the HuC domain photoconverted at 36hpf (A), and the model of the domain photoconverted at 36hpf to the model of the neuronal differentiation domain at 48hpf (B).
The temporal registration 3D-map at 72hpf.
3D-model of the neuronal differentiation domains contributed by neurons born between 24 and 36hpf (A, green), and between 36 and 48hpf (B, blue), between 48 and 72hpf (C, yellow), displayed as 360° rotation. These 3D-models were obtained by subtracting the model of the domain photoconverted at 24hpf to the model of the domain photoconverted at 36hpf (A), the 36hpf one to the model of the domain photoconverted at 48hpf (B), and the 48hpf to the model of at the model of the neuronal differentiation domain at 72hpf (C).
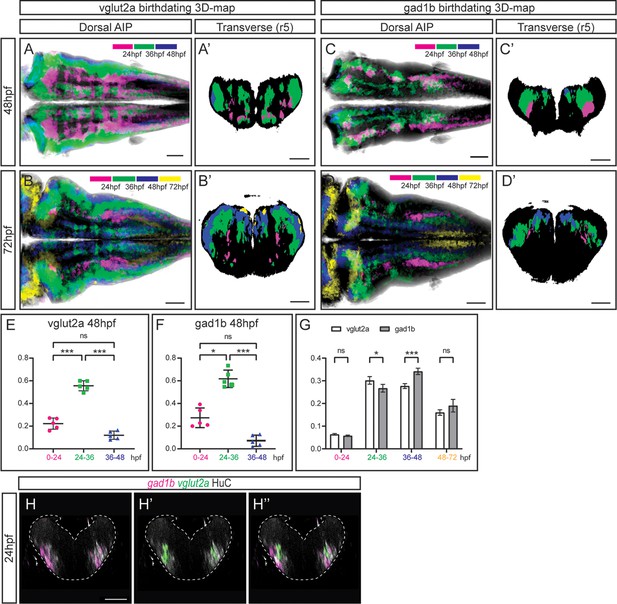
The temporal registration 3D-atlas can be used as a proxy to reveal the birthdate of the desired neuronal differentiated neurons.
(A–D) Dorsal average intensity projections (AIP) with anterior to the left, and (A–D’) transverse views through r5, of the glutamatergic (A–B) and GABAergic (C–D) 3D-models intersected with the birthdating 3D-map at 48hpf (A, C) and at 72hpf (B, D). vglut2a or gad1b neurons produced before 24hpf, between 24 and 36hpf, between 36 and 48hpf and between 48 and 72hpf are color-coded as indicated. The differentiated domain is depicted in black. (E–F) Dot-plots showing the relative contribution to the corresponding vglut2a/gad1b-neuronal differentiation domains at 48hpf of neurons born at the indicated time intervals. (G) Interleaved bar-plot showing the relative contribution of glutamatergic and GABAergic neurons generated at different time intervals to the vglut2a/gad1b-neuronal differentiation domains at 72hpf, respectively. (E–F) RM one-way ANOVA with Tukey’s multiple comparison test, and (G) two-way ANOVA with Šídák’s multiple comparisons test; p<0.033 (*) p<0.002 (**) p<0.001 (***). (H–H’’) Transverse views of a 24hpf Tg[HuC:GFP] embryo hybridized with vglut2a (green) and gad1b (magenta), and immunostained with HuC (gray). Images are displayed as the overlay of gad1b and HuC (H), vglut2a and HuC (H’), and the merge of the three (H’’). Note that already at 24hpf, there are vglut2a and gad1b cells within the HuC domain. The neural tube contour is depicted with a white dashed line. Scale bar, 50μm.
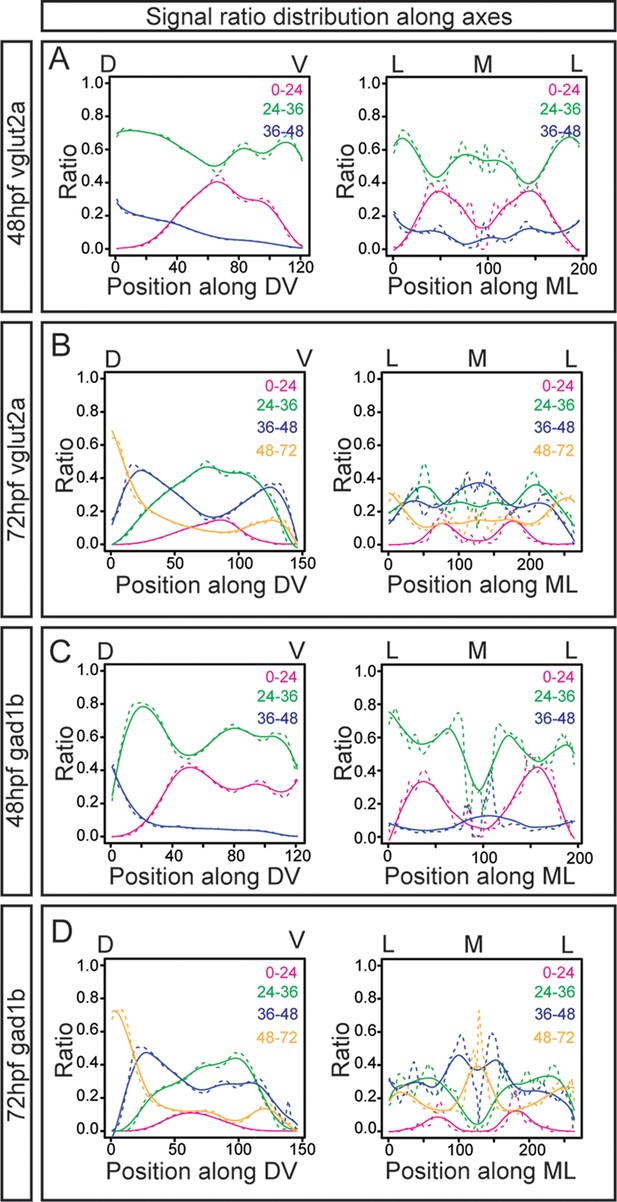
Quantification of the signal ratio distribution of glutamatergic and GABAergic neurons along the axes.
(A–D) Signal ratio distribution along the dorsoventral (DV) and mediolateral (ML) axes at 48hpf (A, C) and 72hpf (B, D) of glutamatergic (A–B) and GABAergic (C–D) neurons differentiated at distinct time intervals (magenta, before 24hpf; green, between 24 and 36hpf; blue, between 36 and 48hpf; yellow, between 48nd 72hpf), with the average values displayed as dashed lines and non-linear regression as solid lines. Position along the axes is indicated on the top.
The neuronal birthdate 3D-atlas as a proxy to reveal the order of neuronal differentiation.
Intersection of glutamatergic (A–B) and GABAergic (C–D) neuronal 3D-models and the birthdating 3D-maps at 48hpf (A, C) and 72hpf (B, D). Neurons born before 24hpf are in magenta, between 24 and 36hpf in green, between 36 and 48hpf in blue, and between 48 and 72hpf in yellow. Intersectional maps are displayed as 360° rotation.
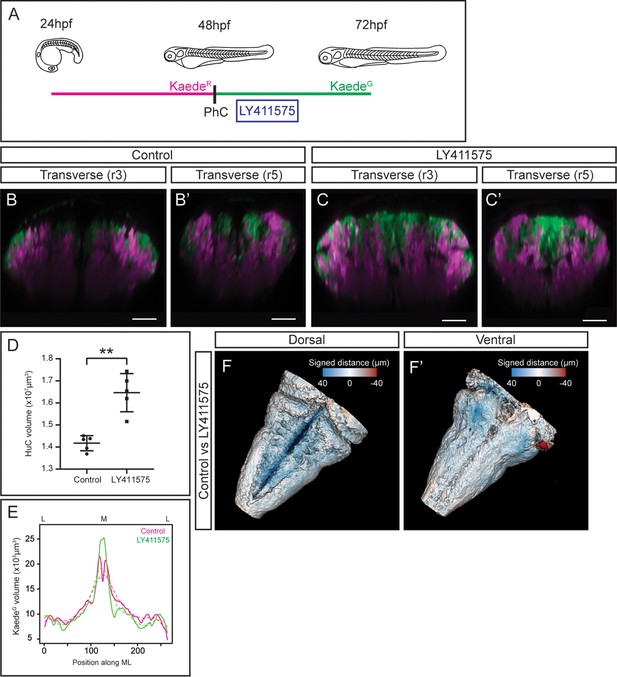
Tissue shape analysis to reveal the impact of neurogenesis defects.
(A) Scheme with the outline of the experiment. HuC:KaedeGreen was photoconverted at 48hpf, embryos were incubated with the gamma-secretase inhibitor LY411575 during 4h, and imaged at 72hpf. As control, HuC:Kaede photoconverted non-treated embryos were used. (B–C, B’–C’) Transverse views through r3 and r5 of control (B–B’) and LY411575-treated (C–C’) embryos. Note the increase of the HuC:KaedeGreen domain upon conditionally inhibiting Notch-signaling, and the consequently remodeling of the HuC:KaedeRed domain. (D) Dot-plot displaying the neuronal differentiation volume in control and LY411575-treated embryos (n=5 embryos each). Welch’s two-tailed t-test; P<0.002 (**). Note the increase upon Notch-inhibition. (E) Quantification of the HuC:KaedeGreen volume in control and treated embryos along the ML axis. Note the accumulation of neurons in the medial differentiation domain of treated embryos. The average value is displayed as solid line and the non-linear regression as dashed line (n=5 embryos each). (F–F’) Shape comparison analysis of the neuronal differentiation domain between control and LY411575-treated embryos. Overlay of total 3D-neuronal volumes of control and treated embryos in dorsal (F) and ventral (F’) views. Color-coded legend indicates the signed distance between the two conditions. Note that the dorsal part is bluer compared with the ventral part, due to the accumulation of more neurons in this domain (n=5 embryos each). Scale bar, 50μm.
Tissue shape comparison after increasing neurogenesis.
Model of the total neuronal differentiation domain of control embryos displayed as 360° rotation. Color-coded legend indicates the signed distance between the control and treated models. Note that the dorsal part is bluer compared with the ventral part, due to the accumulation of more neurons in this domain (n=5 embryos each).
Tables
Reagent type (species) or resource | Designation | Source or reference | Identifiers | Additional information |
---|---|---|---|---|
Genetic reagent (Danio rerio) | Tg[HuC:GFP] | PMID:11071755 | Tg[HuC:GFP] | |
Genetic reagent (Danio rerio) | Tg[HuC:KAEDE] | PMID:25264359 | Tg[HuC:KAEDE] | |
Genetic reagent (Danio rerio) | Tg[gad1b:DsRed] | PMID:23946442 | Tg[gad1b:lox-DsRed-lox-EGFP] | |
Genetic reagent (Danio rerio) | Tg[vglut2a:DsRed] | PMID:23946442 | Tg[vglut2a:lox-DsRed-lox-EGFP] | |
Genetic reagent (Danio rerio) | Mü4127 | PMID:19628697 | ||
Genetic reagent (Danio rerio) | Tg[neurog1:DsRed] | PMID:23468645 | ||
Genetic reagent (Danio rerio) | Tg[Isl1:GFP] | PMID:10627598 | ||
Sequence-based reagent (primers) | gad1b Fw | PMID:32443726 | ||
Sequence-based reagent (primers) | gad1b Rev | PMID:32443726 | ||
Sequence-based reagent | vglut2b probe | PMID:15515025 | ||
Antibody | mouse anti-HuC | ThermoFisher | A-21271 | (1:400) |
Antibody | F(ab')2-Goat anti-Mouse IgG (H+L) Cross-Adsorbed Secondary Antibody, Alexa Fluor 633 | Invitrogen | A21053 | (1:500) |
Chemical compounds, drug | LY411575 | Sigma-Aldrich | SML0506-5MG | γ-secretase inhibitor |
Chemical compounds, drug | Click-iT EdU Imaging Kit | ThermoFisher Scientific | C10086 | |
Software, algorithm | FIJI | PMID:22743772 | ||
Software, algorithm | Fijiyama registration FIJI plugin | PMID:32997734 | ||
Software, algorithm | Trainable Weka Segmentation FIJI plugin | PMID:28369169 | TWS | |
Software, algorithm | VEDO | doi:10.5281/zenodo.4287635 |
Additional files
-
MDAR checklist
- https://cdn.elifesciences.org/articles/78300/elife-78300-mdarchecklist1-v2.docx
-
Source data 1
z-stacks resulting from the pipeline, and subsequent intersections can be found in the 3D-model collection.
- https://cdn.elifesciences.org/articles/78300/elife-78300-data1-v2.zip