Evaluation of in silico predictors on short nucleotide variants in HBA1, HBA2, and HBB associated with haemoglobinopathies
Figures
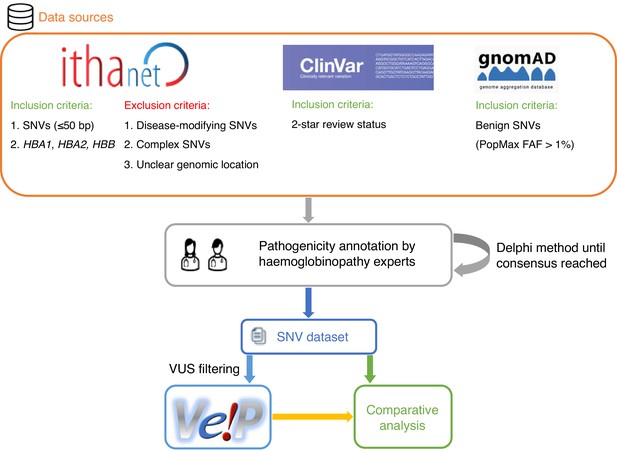
A schematic flowchart of the methodology followed for this comparative analysis.
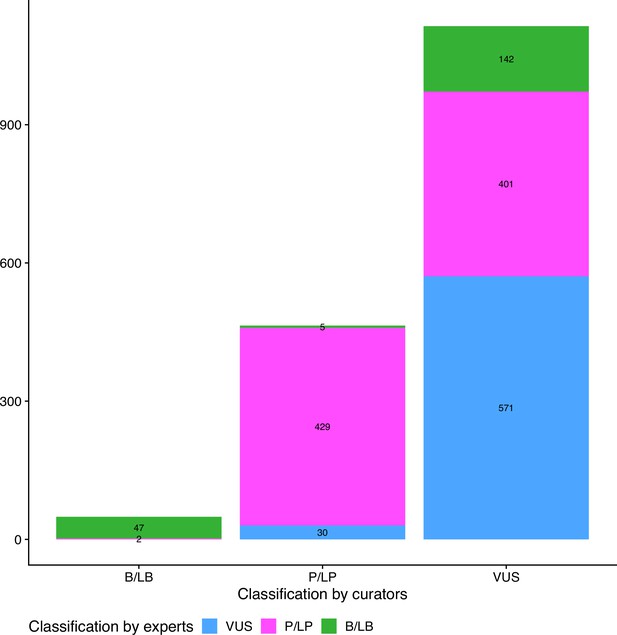
Comparison of initial and final pathogenicity classification of variants in the dataset.
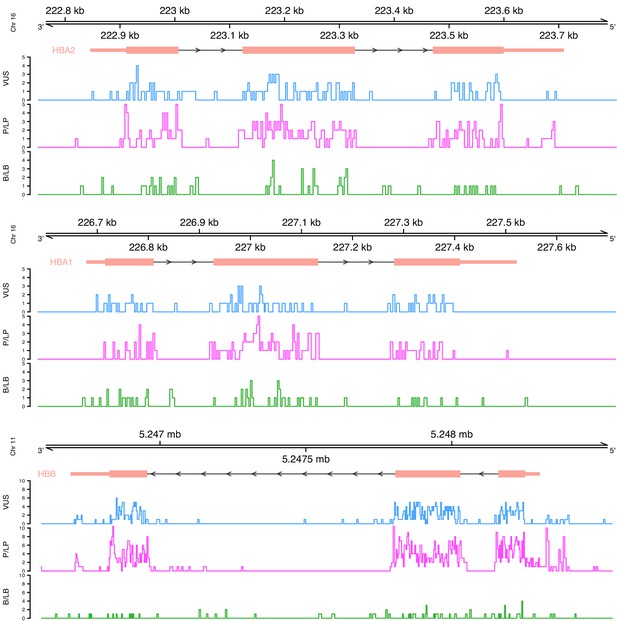
Distribution of variants on each globin gene based on their actual pathogenicity.
A bin size of 3 bp (inframe) and 5 bp in exonic and intronic regions, respectively, is used for the illustration.
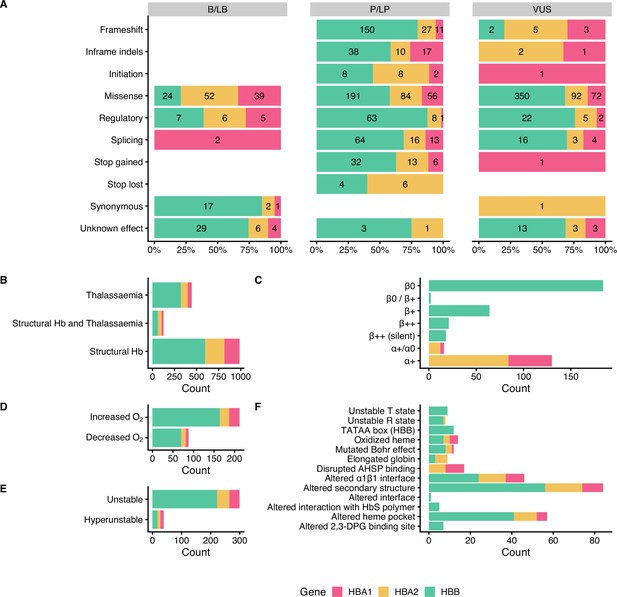
Descriptive plots of the short nucleotide variant (SNV) dataset.
(A) Variant effect on gene/protein function with respect to the annotated pathogenicity status. (B) Haemoglobinopathy group, (C) thalassaemia phenotype, (D) O2 affinity, (E) Hb stability, and (F) molecular mechanisms.
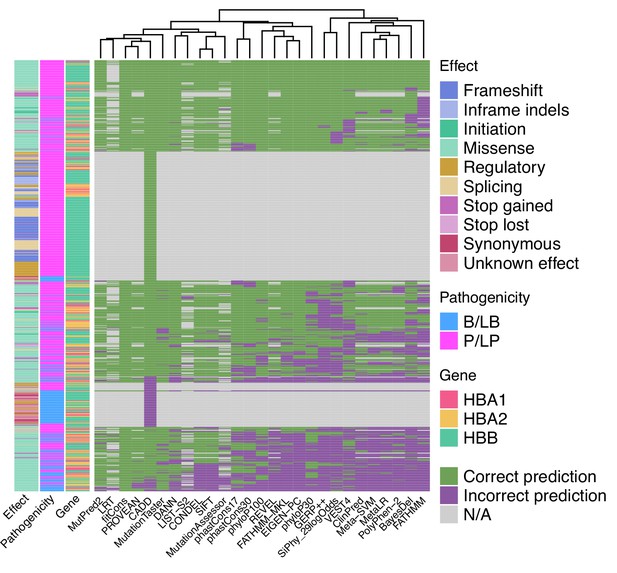
Heatmap illustrating the concordance and clustering of in silico tools with respect to the variant type and globin gene using the threshold that optimises the Matthews correlation coefficient (MCC), as shown in Table 1.
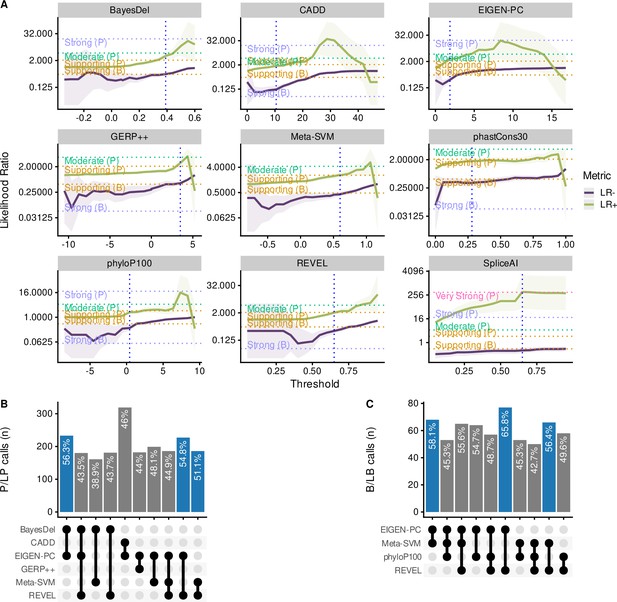
Comparison of the top performing in silico tools.
(A) Likelihood ratios of the top performing in silico tools with variable threshold. Vertical dashed lines indicate the optimal threshold based on the highest Matthews correlation coefficient (MCC). (B) Concordant pathogenic/likely pathogenic (P/LP) calls by any given combination of in silico tools (among top performing tools) for pathogenic variants. (C) Concordant benign/likely benign (B/LB) calls by any given combination of in silico tools (among top performing tools) for benign variants. For Panels B and C, the concordance rate (i.e., variant assertion for all tools in the combination matches the expert annotation) is provided as text annotation on the bar chart. Only the first top 10 tool combinations based on concordance rate are shown, with the top three shown in blue.
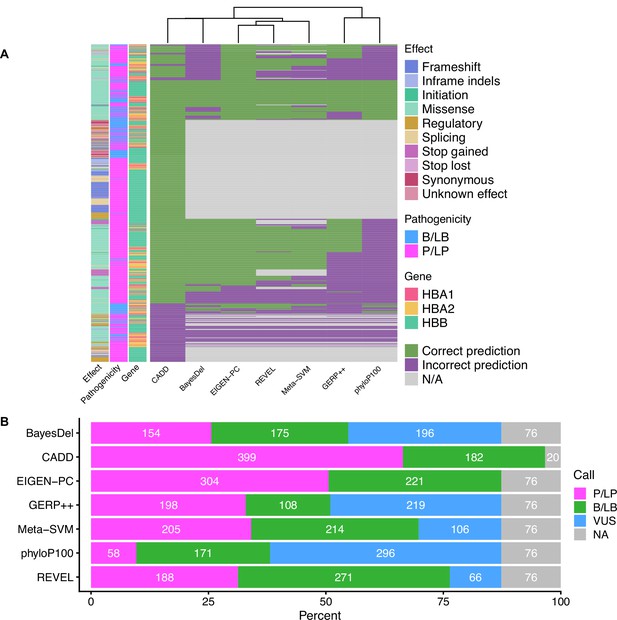
Concordance and VUS prediction of the top performing in silico tools.
(A) Heatmap illustrating the concordance and clustering of the top performing in silico tools with respect to the variant type and globin gene, using the separate non-overlapping thresholds for pathogenic and benign prediction, as shown in Table 2. (B) Prediction of variants of uncertain significance (VUS) using thresholds for the full dataset (at supporting strength).
Tables
Results and performance comparison of in silico predictors with the optimal threshold based on MCC.
#PV: number of predicted variants; Ac: accuracy; Se: sensitivity; Sp: specificity; MCC: Matthews correlation coefficient; LR+: positive likelihood ratio; LR-: negative likelihood ratio; 95% CI: 95% confidence interval.
Tool | Decision threshold | #PV | TP | FN | FP | TN | Ac | Se | Sp | MCC | LR+ | LR +95% CI | LR- | LR- 95% CI |
---|---|---|---|---|---|---|---|---|---|---|---|---|---|---|
BayesDel_addAF | ≥0.39 | 531 | 250 | 164 | 22 | 95 | 0.65 | 0.6 | 0.81 | 0.34 | 3.21 | [2.19, 4.72] | 0.49 | [0.42, 0.57] |
CADD | >10.44 | 886 | 655 | 39 | 101 | 91 | 0.84 | 0.94 | 0.47 | 0.49 | 1.79 | [1.57, 2.05] | 0.12 | [0.08, 0.17] |
ClinPred | >0.95 | 481 | 265 | 99 | 43 | 74 | 0.7 | 0.73 | 0.63 | 0.32 | 1.98 | [1.55, 2.53] | 0.43 | [0.35, 0.53] |
Condel | >0.3 | 481 | 331 | 33 | 76 | 41 | 0.77 | 0.91 | 0.35 | 0.31 | 1.4 | [1.22, 1.61] | 0.26 | [0.17, 0.39] |
DANN | >0.96 | 531 | 372 | 42 | 71 | 46 | 0.79 | 0.9 | 0.39 | 0.33 | 1.48 | [1.28, 1.72] | 0.26 | [0.18, 0.37] |
Eigen-PC | >1.87 | 531 | 329 | 85 | 35 | 82 | 0.77 | 0.79 | 0.7 | 0.44 | 2.66 | [2, 3.52] | 0.29 | [0.23, 0.37] |
FATHMM | ≤–3.39 | 481 | 150 | 214 | 23 | 94 | 0.51 | 0.41 | 0.8 | 0.19 | 2.1 | [1.42, 3.08] | 0.73 | [0.65, 0.83] |
fathmm-MKL | >0.7 | 531 | 328 | 86 | 39 | 78 | 0.76 | 0.79 | 0.67 | 0.41 | 2.38 | [1.83, 3.09] | 0.31 | [0.25, 0.39] |
GERP++ | >3.49 | 531 | 248 | 166 | 26 | 91 | 0.64 | 0.6 | 0.78 | 0.31 | 2.7 | [1.9, 3.82] | 0.52 | [0.44, 0.6] |
integrated_fitCons | >0.05 | 531 | 414 | 1 | 117 | 1 | 0.78 | 1 | 0.01 | 0.04 | 1.01 | [0.99, 1.02] | 0.28 | [0.02, 4.51] |
LIST-S2 | ≥0.75 | 344 | 246 | 28 | 39 | 31 | 0.81 | 0.9 | 0.44 | 0.36 | 1.61 | [1.3, 1.99] | 0.23 | [0.15, 0.36] |
LRT | <0.3 | 270 | 169 | 7 | 84 | 10 | 0.66 | 0.96 | 0.11 | 0.13 | 1.07 | [1, 1.16] | 0.37 | [0.15, 0.95] |
MetaLR_score | >0.8 | 481 | 251 | 113 | 42 | 75 | 0.68 | 0.69 | 0.64 | 0.29 | 1.92 | [1.49, 2.47] | 0.48 | [0.39, 0.59] |
MetaSVM_score | >0.6 | 481 | 260 | 104 | 39 | 78 | 0.7 | 0.71 | 0.67 | 0.34 | 2.14 | [1.65, 2.79] | 0.43 | [0.35, 0.53] |
MutationAssessor | >2.53 | 359 | 249 | 36 | 41 | 33 | 0.79 | 0.87 | 0.45 | 0.33 | 1.58 | [1.28, 1.94] | 0.28 | [0.19, 0.42] |
MutationTaster | >0.95 | 531 | 386 | 28 | 102 | 15 | 0.76 | 0.93 | 0.13 | 0.09 | 1.07 | [0.99, 1.15] | 0.53 | [0.29, 0.95] |
MutPred | >0.5 | 467 | 343 | 12 | 96 | 16 | 0.77 | 0.97 | 0.14 | 0.2 | 1.13 | [1.04, 1.22] | 0.24 | [0.12, 0.49] |
phastCons17way | >0.17 | 531 | 357 | 57 | 57 | 60 | 0.79 | 0.86 | 0.51 | 0.38 | 1.77 | [1.46, 2.14] | 0.27 | [0.2, 0.36] |
phastCons30way | >0.28 | 531 | 329 | 85 | 51 | 66 | 0.74 | 0.79 | 0.56 | 0.33 | 1.82 | [1.48, 2.25] | 0.36 | [0.28, 0.47] |
phyloP100way | >0.42 | 531 | 349 | 65 | 56 | 61 | 0.77 | 0.84 | 0.52 | 0.35 | 1.76 | [1.45, 2.14] | 0.3 | [0.23, 0.4] |
phyloP30way | >0.51 | 531 | 307 | 107 | 63 | 54 | 0.68 | 0.74 | 0.46 | 0.18 | 1.38 | [1.15, 1.64] | 0.56 | [0.43, 0.72] |
PolyPhen-2 | >0.65 | 481 | 243 | 121 | 37 | 80 | 0.67 | 0.67 | 0.68 | 0.31 | 2.11 | [1.6, 2.78] | 0.49 | [0.4, 0.59] |
PROVEAN | ≤–1.03 | 481 | 358 | 6 | 106 | 11 | 0.77 | 0.98 | 0.09 | 0.18 | 1.09 | [1.02, 1.15] | 0.18 | [0.07, 0.46] |
REVEL | >0.65 | 481 | 294 | 70 | 46 | 71 | 0.76 | 0.81 | 0.61 | 0.39 | 2.05 | [1.63, 2.59] | 0.32 | [0.25, 0.41] |
SIFT | <0.1 | 481 | 325 | 39 | 74 | 43 | 0.77 | 0.89 | 0.37 | 0.3 | 1.41 | [1.22, 1.63] | 0.29 | [0.2, 0.43] |
SiPhy_29way | >10.62 | 531 | 233 | 181 | 33 | 84 | 0.6 | 0.56 | 0.72 | 0.23 | 2 | [1.48, 2.7] | 0.61 | [0.52, 0.71] |
VEST4 | >0.7 | 531 | 273 | 141 | 33 | 84 | 0.67 | 0.66 | 0.72 | 0.32 | 2.34 | [1.74, 3.15] | 0.47 | [0.4, 0.57] |
Splicing prediction | ||||||||||||||
ada | >0.5 | 56 | 47 | 3 | 1 | 5 | 0.93 | 0.94 | 0.83 | 0.68 | 5.64 | [0.94, 33.8] | 0.07 | [0.02, 0.23] |
MaxEntScan | Diff >2 and Per >5 | 54 | 50 | 2 | 1 | 2 | 0.95 | 0.96 | 0.67 | 0.55 | 2.88 | [0.58, 14.31] | 0.06 | [0.01, 0.28] |
rf | >0.6 | 56 | 47 | 3 | 1 | 5 | 0.93 | 0.94 | 0.83 | 0.68 | 5.64 | [0.94, 33.8] | 0.07 | [0.02, 0.23] |
SpliceAI | >0.65 | 663 | 35 | 23 | 1 | 604 | 0.96 | 0.6 | 1 | 0.75 | 365.09 | [50.94, 2616.41] | 0.4 | [0.29, 0.55] |
In silico tools with pairs of non-overlapping thresholds that reach at least supporting evidence strength for both pathogenic and benign prediction.
LR: likelihood ratio; CI: confidence interval; PV: predicted variants.
Pathogenic prediction | Benign Prediction | |||||||||||
---|---|---|---|---|---|---|---|---|---|---|---|---|
Tool | Pathogenic threshold | Sensitivity | LR+ | LR+ 95% CI | Strength (pathogenic) | Benign threshold | Specificity | LR- | LR- 95% CI | Strength (benign) | Correctly PV | % of correctly PV |
All SNVs | ||||||||||||
BayesDel_addAF | ≥0.39 | 0.6 | 3.21 | [2.19, 4.72] | Supporting | <0.23 | 0.44 | 0.35 | [0.26, 0.47] | Supporting | 302 | 56.87 |
CADD | >25 | 0.39 | 8.27 | [4.34, 15.75] | Moderate | ≤21.75 | 0.78 | 0.42 | [0.37, 0.48] | Supporting | 418 | 47.18 |
CADD | >16.3 | 0.82 | 2.59 | [2.1, 3.2] | Supporting | ≤16.3 | 0.68 | 0.26 | [0.21, 0.31] | Supporting | 703 | 79.35 |
Eigen-PC | >1.9 | 0.79 | 3 | [2.21, 4.07] | Supporting | ≤1.9 | 0.74 | 0.28 | [0.22, 0.35] | Supporting | 415 | 78.15 |
GERP++ | >4.22 | 0.44 | 4.33 | [2.51, 7.49] | Supporting | ≤0.15 | 0.35 | 0.32 | [0.22, 0.46] | Supporting | 225 | 42.37 |
MetaSVM | >0.81 | 0.55 | 3.25 | [2.16, 4.89] | Supporting | ≤0.46 | 0.6 | 0.38 | [0.3, 0.48] | Supporting | 272 | 56.55 |
phyloP100way | >7.32 | 0.15 | 17.8 | [2.5, 127] | Supporting | ≤0.8 | 0.57 | 0.36 | [0.28, 0.46] | Supporting | 130 | 24.48 |
REVEL | >0.77 | 0.63 | 3.05 | [2.12, 4.4] | Supporting | ≤0.7 | 0.69 | 0.38 | [0.31, 0.47] | Supporting | 309 | 64.24 |
SpliceAI | >0.3 | 0.67 | 58.12 | [27.23, 124.03] | Strong | ≤0.3 | 0.99 | 0.33 | [0.23, 0.48] | Supporting | 637 | 96.08 |
Missense only | ||||||||||||
BayesDel_addAF | ≥0.41 | 0.54 | 3.35 | [2.2, 5.12] | Supporting | <0.22 | 0.44 | 0.32 | [0.23, 0.45] | Supporting | 241 | 51.72 |
CADD | >23.25 | 0.6 | 3.19 | [2.17, 4.69] | Supporting | ≤20.9 | 0.62 | 0.36 | [0.28, 0.46] | Supporting | 283 | 60.6 |
Eigen-PC | >1.9 | 0.78 | 2.93 | [2.16, 3.98] | Supporting | ≤1.9 | 0.74 | 0.3 | [0.24, 0.38] | Supporting | 357 | 76.61 |
GERP++ | >4.22 | 0.44 | 4.27 | [2.47, 7.4] | Supporting | ≤–0.87 | 0.29 | 0.31 | [0.2, 0.47] | Supporting | 187 | 40.13 |
MetaSVM | >0.8 | 0.58 | 3.08 | [2.09, 4.53] | Supporting | ≤0.39 | 0.56 | 0.37 | [0.29, 0.48] | Supporting | 267 | 57.3 |
phastCons30way | >0.94 | 0.52 | 3.19 | [2.09, 4.88] | Supporting | ≤0.41 | 0.61 | 0.36 | [0.28, 0.46] | Supporting | 252 | 54.08 |
phyloP100way | >7.32 | 0.16 | 19.11 | [2.68, 136.47] | Supporting | ≤0.56 | 0.53 | 0.35 | [0.27, 0.46] | Supporting | 119 | 25.54 |
REVEL | >0.77 | 0.62 | 3.02 | [2.09, 4.35] | Supporting | ≤0.7 | 0.69 | 0.39 | [0.32, 0.48] | Supporting | 297 | 63.73 |
Non-missense only | ||||||||||||
CADD | >11.5 | 0.93 | 8.62 | [3.42, 21.77] | Supporting | ≤11.5 | 0.89 | 0.08 | [0.05, 0.11] | Supporting | 350 | 92.84 |
SNVs in HBB | ||||||||||||
BayesDel_addAF | ≥0.31 | 0.8 | 6.43 | [2.23, 18.58] | Supporting | <0.31 | 0.88 | 0.22 | [0.17, 0.3] | Supporting | 210 | 81.08 |
CADD | >25.25 | 0.42 | 31.64 | [4.5, 222.38] | Moderate | ≤22.65 | 0.92 | 0.42 | [0.37, 0.48] | Supporting | 264 | 48.71 |
CADD | >10.8 | 0.94 | 3.26 | [2.29, 4.64] | Supporting | ≤10.8 | 0.71 | 0.08 | [0.05, 0.12] | Supporting | 494 | 91.14 |
SNVs in HBA1 | ||||||||||||
CADD | >22.95 | 0.59 | 4.94 | [2.29, 10.68] | Supporting | ≤17 | 0.66 | 0.3 | [0.19, 0.48] | Supporting | 84 | 61.76 |
Additional files
-
Supplementary file 1
The list of ClinGen Hemoglobinopathy variant curation expert panel (VCEP) members.
- https://cdn.elifesciences.org/articles/79713/elife-79713-supp1-v2.xlsx
-
Supplementary file 2
Table with the dataset used in this study and the resulting scores obtained by the in silico predictors, divided into different sheets and subsets: all short nucleotide variants (SNVs), missense only, non-missense only, HBB, HBA1, and HBA2.
- https://cdn.elifesciences.org/articles/79713/elife-79713-supp2-v2.xlsx
-
Supplementary file 3
Refined thresholds for the nine selected in silico predictors, divided into different subsets: all short nucleotide variants (SNVs), missense only, non-missense only, HBB, HBA1, and HBA2.
Only decision thresholds passing the likelihood ratio (LR) criteria for supporting evidence are shown.
- https://cdn.elifesciences.org/articles/79713/elife-79713-supp3-v2.xlsx
-
MDAR checklist
- https://cdn.elifesciences.org/articles/79713/elife-79713-mdarchecklist1-v2.docx