Determining the effects of paternal obesity on sperm chromatin at histone H3 lysine 4 tri-methylation in relation to the placental transcriptome and cellular composition
Figures
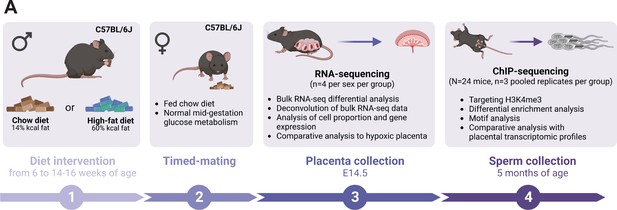
Experimental design showing the timeline and methods used to study the consequences of an obesity-induced altered sperm epigenome on the placenta.
(A) Six-week-old C57BL/6J sires were fed either a control or high-fat diet (CON or HFD, respectively) for 8–10 weeks. Males were then time-mated with CON-fed C57BL/6J females to generate pregnancies. Pregnant females were sacrificed at embryonic day (E)14.5 and placentas were collected to perform RNA-sequencing (RNA-seq, n=4 per sex per dietary group). Sires were sacrificed at 5 months of age and sperm from cauda epididymides was collected for chromatin immunoprecipitation sequencing (ChIP-seq, n=3 replicates per dietary group) targeting histone H3 lysine 4 tri-methylation (H3K4me3). Figure created with BioRender.com.
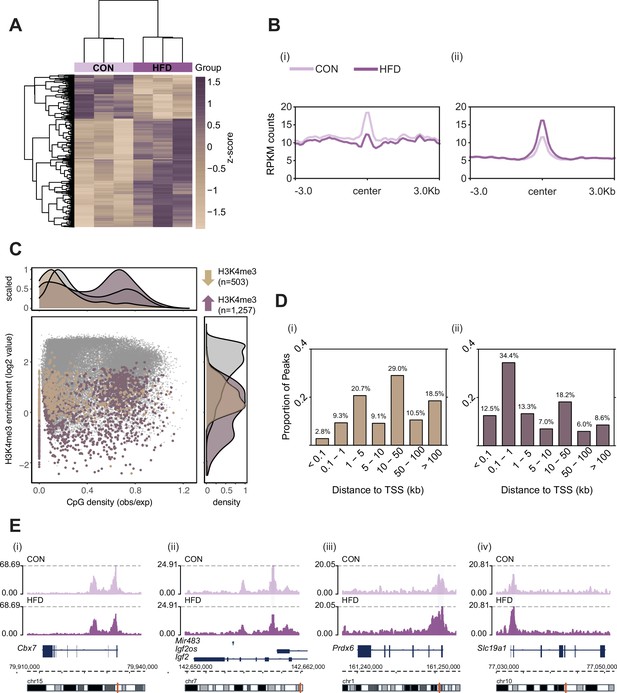
Histone H3 lysine 4 tri-methylation (H3K4me3) signal profile at obesity-sensitive regions in sperm.
(A) Heatmap of log2 normalized counts for obesity-sensitive regions in sperm (n=1760). Columns (samples) and rows (genomic regions) are arranged by hierarchical clustering with complete-linkage clustering based on Euclidean distance. Samples are labeled by dietary group (light and dark purple). (B) Profile plots showing RPKM H3K4me3 counts ±3 kilobase around the center of genomic regions with decreased (i) and increased (ii) H3K4me3 enrichment in high-fat diet (HFD)-sperm compared to control (CON)-sperm. (C) Scatter plot showing H3K4me3 enrichment (log2 counts) versus CpG density (observed/expected) for all H3K4me3-enriched regions in sperm (n=35,184, in gray), regions with HFD-induced decreased H3K4me3 enrichment (n=503, in beige), and regions with increased H3K4me3 enrichment (n=1257, in purple). The upper and right panels represent the data points density for CpG density and H3K4me3 enrichment, respectively. (D) Bar plots showing the proportion of peaks for each category of distance from the transcription start site (TSS) of the nearest gene in kilobase (kb), for obesity-sensitive regions with decreased (i) and increased (ii) H3K4me3 enrichment in HFD-sperm. (E) Genome browser snapshots showing genes with altered sperm H3K4me3 at promoter regions (CON light purple, HFD dark purple).
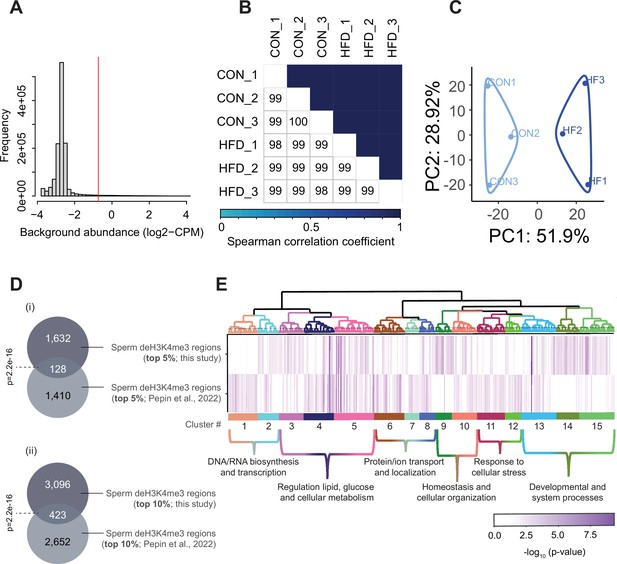
Sperm histone H3 lysine 4 tri-methylation (H3K4me3) ChIP-sequencing data quality and normalization.
(A) Histogram showing frequency distributions of read abundances of genome-wide 150 bp windows. The vertical red line indicates the cut-off where windows with low read counts were filtered out (abundance below log2(4) fold over 2000 bp bins). The remaining windows (considered enriched for H3K4me3) which were less than 100 bp apart were merged allowing a maximum width of 5000 bp (n=35,184 merged regions enriched for H3K4me3 in sperm). (B) Spearman correlation heatmap on counts at sperm H3K4me3-enriched genomic regions after TMM normalization and batch adjustment. Color gradients represent correlation coefficients for each pairwise comparison. (C) Principal component analysis (PCA) plot for counts in H3K4me3-enriched regions in sperm after normalization. The top 5% regions contributing to principal component 1 (PC1) were selected as those associated with sample separation according to dietary treatment. (D) Venn diagrams showing the overlap of the top 5% (i) or top 10% (ii) regions contributing to PC1 and associated with sample separation according to dietary group, from this study (dark gray) and our previous study (Pepin et al., 2022; light gray). Fisher’s exact test was used to assess significance of overlap across region sets, using the common universe (background) of the datasets being compared, and p<0.05 was considered significant. (E) Heatmap showing significant gene ontology (GO) terms clustered based on functional similarity, comparing enriched biological functions in obesity-sensitive regions located at promoters detected in this study (top row) and in our previous study (Pepin et al., 2022, bottom row). Columns represent enriched GO terms ordered by hierarchical clustering based on Wang’s semantic similarity distance and ward.D2 aggregation criterion. The color intensity represents the GO term enrichment significance (-log10 p-value). Interactive versions of these figures can be found in Supplementary file 2 and the complete lists of significantly enriched GO terms can be found in Supplementary file 1b.
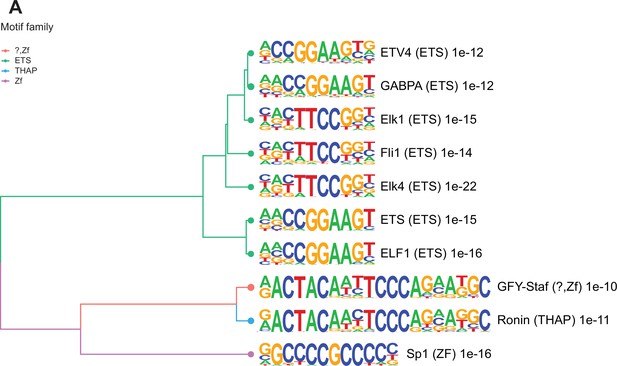
Enriched motifs at obesity-sensitive regions in sperm.
(A) Top 10 significantly enriched known motifs at obesity-sensitive regions with increased histone H3 lysine 4 tri-methylation (H3K4me3) enrichment in high-fat diet (HFD)-sperm. Motifs are clustered based on sequence similarity with hierarchical clustering. Branches of the dendrogram tree are color-coded by motif family. The name of the motif is indicated on the right, with the motif family in parenthesis, and the associated p-value for enrichment significance (binomial statistical test). The full list of enriched motifs can be found in Supplementary file 3.
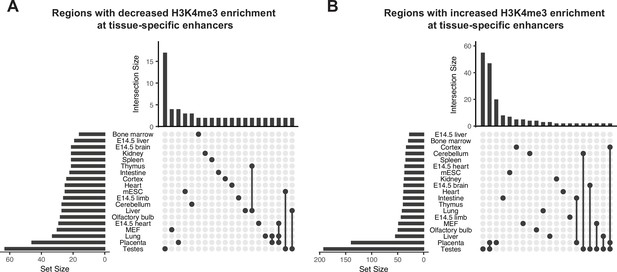
Obesity-sensitive regions in sperm are found at tissue-specific enhancers important for development.
(A–B) Upset plots showing annotations for tissue-specific enhancers overlapping with differentially expressed histone H3 lysine 4 tri-methylation (deH3K4me3) regions with decreased enrichment in high-fat diet (HFD) sperm (A) and increased enrichment in HFD-sperm (B). Horizontal bars on the left sides of each panel represent the number of regions overlapping with each genomic annotation (set size). Vertical bars on the top of each panel represent the number of regions belonging to intersecting annotations (intersection size). Intersection sets are represented by connecting nodes.
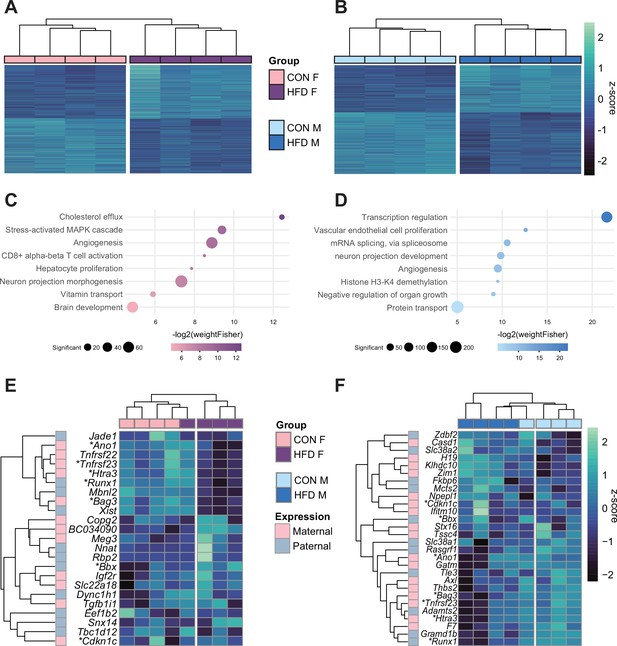
Paternal obesity alters the F1 placental transcriptome in a sex-specific manner.
(A–B) Heatmaps of normalized counts scaled by row (z-score) for transcripts that code for the detected differentially expressed genes (Lancaster p<0.05) in female (A, n=2035 genes) and male (B, n=2365 genes) placentas. Rows are orders by k-means clustering and columns are arranged by hierarchical clustering with complete-linkage based on Euclidean distances. (C–D) Gene ontology (GO) analysis for differentially expressed genes in female (C) and male (D) placentas. The bubble plot highlights eight significantly enriched GO terms, with their -log2(p-value) depicted on the y-axis and with the color gradient. The size of the bubbles represents the number of significant genes annotated to a specific enriched GO term. Supplementary file 1e and f includes the full lists of significant GO terms. (E–F) Heatmaps of normalized counts scaled by row (z-score) for detected differentially expressed imprinted genes (Lancaster p<0.05) in female (E, n=23 genes) and male (F, n=28 genes) placentas. Genes are labeled based on their allelic expression (paternally expressed genes in pale gray, maternally expressed genes in pale pink). Imprinted genes differently expressed in both male and female placentas (n=7) are marked with an asterisk (*). Rows are orders by k-means clustering and columns are arranged by hierarchical clustering with complete-linkage based on Euclidean distances.
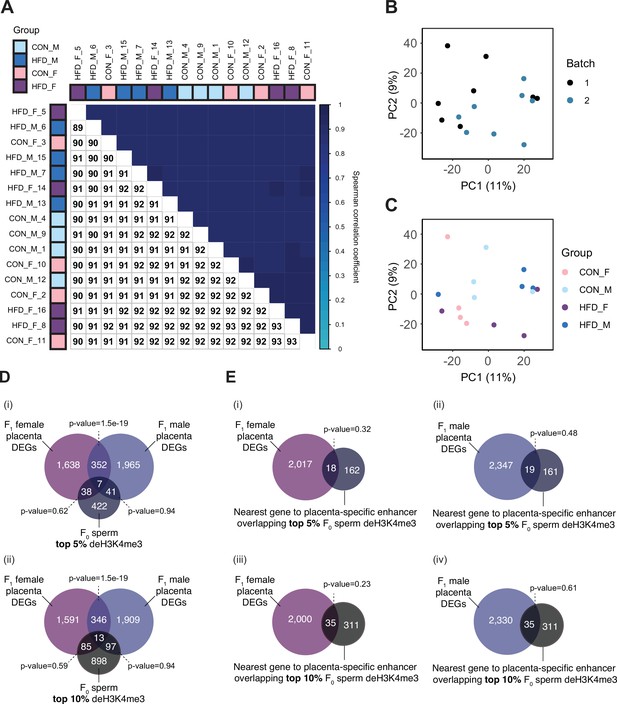
Placenta RNA-sequencing data quality assessment.
(A) Spearman correlation heatmap on variance stabilized transcripts. The color gradient represents the Spearman correlation coefficient for each sample pairwise comparison. (B–C) Principal component analysis (PCA) on variance stabilized transcripts with samples labeled by batch (B) and experimental group (C). (D) Venn diagrams showing the overlap of paternal obesity-induced de-regulated genes between female and male placentas with the top 5% (i) and the top 10% (ii) promoter regions most sensitive to obesity in sperm. Fisher’s exact test was used to assess significance of overlap across gene sets, using the common universe (background) of the datasets being compared, and p<0.05 was considered significant. (E) Venn diagrams showing the overlap of paternal obesity-induced de-regulated genes in female (i and iii) and male (ii and iv) placentas, with the nearest gene to placental-specific enhancer overlapping sperm differentially expressed histone H3 lysine 4 tri-methylation (deH3K4me3), using the top 5% (i–ii) or top 10% (iii–iv) sperm regions associated with dietary group. Fisher’s exact test was used to assess significance of overlap across gene sets, using the common universe (background) of the datasets being compared, and p<0.05 was considered significant.
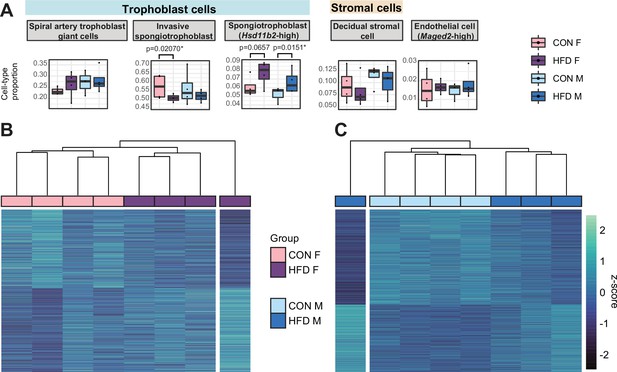
Paternal obesity-induced changes in placental cellular composition and differential expression.
(A) Boxplots showing sample-specific proportions for the top 5 cell types with highest proportions detected in the bulk RNA-sequencing (RNA-seq) data deconvolution analysis across experimental groups (n=4 per sex per group). Beta regression was used to assess differences in cell-type proportions associated with paternal obesity for each placental sex. p<0.05 was considered significant. (B–C) Heatmaps of normalized counts scaled by row (z-score) for transcripts that code for the detected differentially expressed genes (Lancaster p<0.05) in female (B, n=423 genes) and male (C, n=1487 genes) placentas, after adjusting for cell-type proportions. Rows are orders by k-means clustering and columns are arranged by hierarchical clustering with complete-linkage based on Euclidean distances.
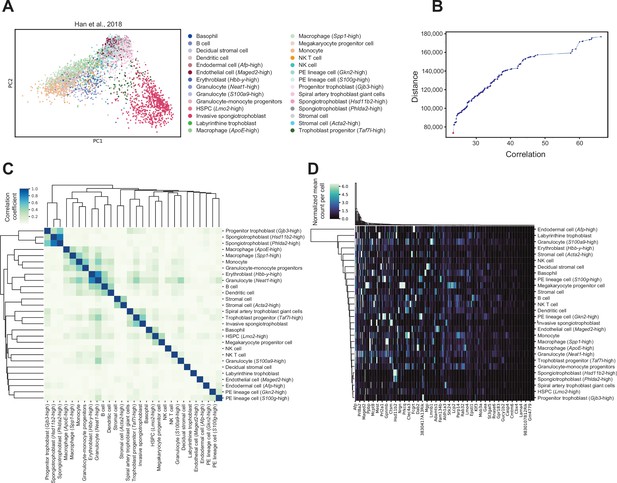
Cell-type-specific marker genes selection using reference mouse embryonic day (E)14.5 placenta single-cell RNA-sequencing dataset.
(A) Principal component analysis (PCA) plot of 4346 single cells from mouse E14.5 placenta, with the 28 different cell types previously identified within the placenta (Han et al., 2018). The number of cells annotated to each cell type can be found in Supplementary file 1g. (B) The 4000 most highly variable genes were used for feature selection using a multi-objective optimization approach with the AutoGeneS package (Aliee and Theis, 2021). The plot shows distance and correlation values for each Pareto-optimal solution. The red triangle indicates the Pareto-optimal solution used to select the 400 marker genes which maximizes distance and minimizes correlation values across cell types. (C) Heatmap showing Pearson correlation between each cell type based on expression values of the selected marker genes. The color gradient represents the Pearson correlation coefficients. Cell types are arranged by hierarchical clustering. (D) Expression signatures of marker genes distinguishing the different cell types detected. The heatmap shows the mean normalized counts per cell type (rows) for the 400 marker genes (columns) as identified by AutoGeneS (Aliee and Theis, 2021). Rows and columns are arranged by hierarchical clustering.
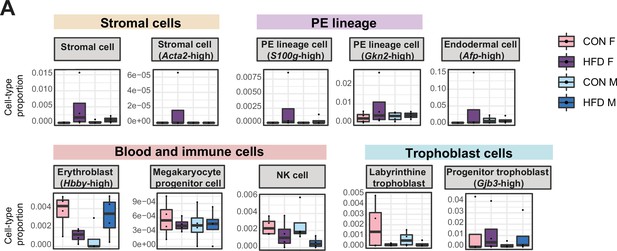
Estimated cell-type proportions across experimental groups for male and female embryonic day (E)14.5 bulk placenta tissues derived from control (CON)- and high-fat diet (HFD)-fed sires.
(A) Boxplots showing sample-specific proportions for the remaining cell types detected in the bulk RNA-sequencing (RNA-seq) data deconvolution analysis across experimental groups (n=4 per sex per group). Beta regression was used to assess differences in cell-type proportions associated with paternal obesity for each placental sex. p<0.05 was considered significant.
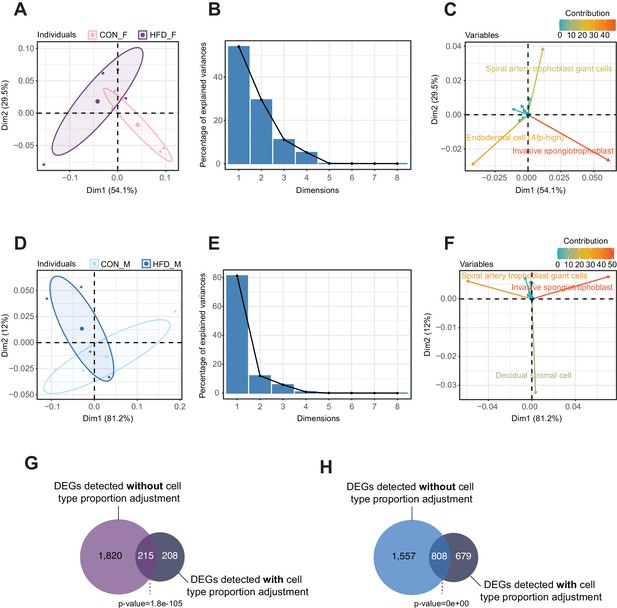
Principal component analysis (PCA) of estimated cell-type proportions.
(A–F) Principal component results for female (A–C) and male (D–F) placentas. (A and D) PCA plot of cell proportions. Confidence ellipses are drawn around mean points for each experimental group. (B and E) Scree plots showing percentage of variances explained by each principal component (dimension). (C and F) Variables factor map showing the top cell types contributing to sample variances. The color gradients on vectors represent the contribution values for each variable (cell type). (G–H) Venn diagrams showing the overlap between the differentially expressed genes in female (G) and male (H) placentas, before and after adjusting for cell-type proportions. Fisher’s exact test was used to assess significance of overlap across gene sets, using the common universe (background) of the datasets being compared, and p<0.05 was considered significant.

Hypoxia-induced growth restriction is associated with changes in placental cellular composition and differential expression.
(A) Boxplots showing sample-specific proportions for the top 10 cell types with highest proportions detected in the bulk RNA-sequencing data deconvolution analysis across experimental groups (n=5 per group). Beta regression was used to assess differences in cell-type proportions associated with hypoxia-induced intrauterine growth restriction. p<0.05 was considered significant. (B) Pyramid plot showing the median values of cell-type proportions commonly detected in both datasets assessed. The asterisks (*) denote significance (p<0.05) between control versus hypoxia groups or control (CON) M versus high-fat diet (HFD) groups, as calculated by beta regression. (C) Heatmap of normalized counts scaled by row (z-score) for transcripts that code for the detected differentially expressed genes (Lancaster p<0.05, n=1477 genes) in hypoxic placentas, after adjusting for cell-type proportions. Rows are orders by k-means clustering and columns are arranged by hierarchical clustering with complete-linkage based on Euclidean distances. (D) Venn diagram showing overlap between hypoxia-induced de-regulated genes in an intrauterine growth restriction model (Chu et al., 2019), and paternal obesity-induced de-regulated genes (this study) in male placentas. Fisher’s exact test was used to assess significance of overlap across gene sets, using the common universe (background) of the datasets being compared, and p<0.05 was considered significant.
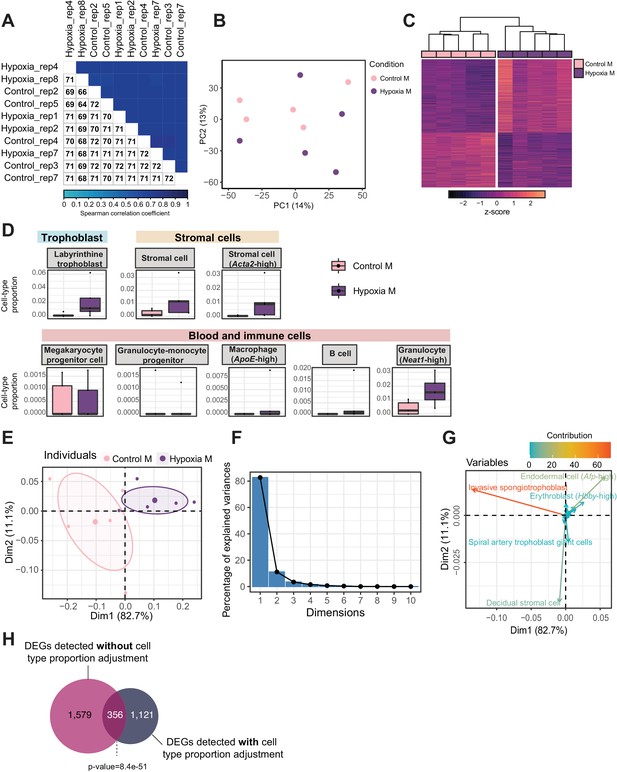
Quality assessment, processing, differential analysis, and deconvolution of RNA-sequencing data from mouse placenta in a hypoxia-induced intrauterine growth restriction mouse model.
(A) Spearman correlation heatmap on variance stabilized transcripts. The color gradient represents the Spearman correlation coefficient for each sample pairwise comparison. (B) Principal component analysis (PCA) on variance stabilized transcripts with samples labeled by experimental group. (C) Heatmap of normalized counts scaled by row (z-score) for transcripts that code for the detected differentially expressed genes (Lancaster p<0.05, n=1935 genes) placentas. Rows are orders by k-means clustering and columns are arranged by hierarchical clustering with complete-linkage based on Euclidean distances. (D) Boxplots showing sample-specific proportions for cell types detected in the bulk RNA-sequencing data deconvolution analysis across experimental groups (n=5 per group). (E–G) PCA of estimated cell-type proportions. (E) PCA plot of cell-type proportions. Confidence ellipses are drawn around mean points for each experimental group. (F) Scree plot showing percentage of variances explained by each principal component (dimension). (G) Variables factor map showing the top cell types contributing to sample variances. The color gradients on vectors represent the contribution values for each variable (cell type). (H) Venn diagram showing the overlap between the differentially expressed genes detected in hypoxic placentas, before and after adjusting for cell-type proportions. Fisher’s exact test was used to assess significance of overlap across gene sets, using the common universe (background) of the datasets being compared, and p<0.05 was considered significant.
Tables
Reagent type (species) or resource | Designation | Source or reference | Identifiers | Additional information |
---|---|---|---|---|
Biological sample (Mus musculus, male) | Spermatozoa | C57BL/6J, The Jackson Laboratory | Isolated from Mus musculus (C57BL/6J) | |
Biological sample (Mus musculus) | Placenta | C57BL/6J, The Jackson Laboratory | Isolated from Mus musculus (C57BL/6J) | |
Antibody | Tri-Methyl-Histone H3 (Lys4) (C42D8) (Rabbit monoclonal) | Cell Signaling Technology | Cat#:9751 | (5 µg) |
Commercial assay or kit | ChIP DNA Clean and Concentrator | Zymo Research | Cat#:D5201 | |
Commercial assay or kit | RNeasy Mini Kit | QIAGEN | Cat#:74104 | |
Chemical compound, drug | Dithiothreitol | Bio Shop | Cat#:3483-12-3 | |
Chemical compound, drug | Micrococcal nuclease (MNase) | Roche | Cat#:10107921001 | |
Chemical compound, drug | Complete Tablets EASYpack | Roche | Cat#:04693116001 | |
Chemical compound, drug | DynaBeads, Protein A | Thermo Fisher Scientific | Cat#:10002D | |
Chemical compound, drug | Bovine Serum Albumin (BSA) | Sigma-Aldrich | Cat#:BP1600-100 | |
Chemical compound, drug | RNase A | Sigma-Aldrich | Cat#:10109169001 | |
Chemical compound, drug | Proteinase K | Sigma-Aldrich | Cat#:P2308 | |
Software, algorithm | R (version 4.0.2) | R Core Team, 2018 | ||
Software, algorithm | Python (version 3.7.4) | Van Rossum and Drake, 2009 | ||
Software, algorithm | Trimmomatic (version 0.36) | Bolger et al., 2014 | ||
Software, algorithm | Bowtie2 (version 2.3.4) | Langmead and Salzberg, 2012 | ||
Software, algorithm | SAMtools (version 1.9) | Li et al., 2009 | ||
Software, algorithm | Deeptools (version 3.2.1) | Ramírez et al., 2016 | ||
Software, algorithm | Trim Galore (version 0.5.0) | Krueger, 2015 | ||
Software, algorithm | Hisat2 (version 2.1.0) | Kim et al., 2015 | ||
Software, algorithm | Stringtie (version 2.1.2) | Pertea et al., 2015 | ||
Software, algorithm | Seaborn (version 0.9.0) | Waskom, 2021 | ||
Software, algorithm | Betareg (version 3.1–4) | Ferrari and Cribari-Neto, 2004 | ||
Software, algorithm | Csaw (version 1.22.1) | Lun and Smyth, 2016 | ||
Software, algorithm | Sva (version 3.36.0) | Leek et al., 2012; Zhang et al., 2020 | ||
Software, algorithm | topGO (version 2.40.0) | Alexa et al., 2006 | ||
Software, algorithm | trackplot | Bolger et al., 2014 | ||
Software, algorithm | Rtracklayer (version 1.48.0) | Lawrence et al., 2009 | ||
Software, algorithm | HOMER (version 4.10.4) | Heinz et al., 2010 | ||
Software, algorithm | ViSEAGO (version 1.2.0) | Brionne et al., 2019 | ||
Software, algorithm | DESeq2 (version 1.28.1) | Love et al., 2014 | ||
Software, algorithm | Aggregation (version 1.0.1) | Yi et al., 2018 | ||
Software, algorithm | Corrplot (version 0.88) | Taiyun and Simko, 2021 | ||
Software, algorithm | Pheatmap (version 1.0.12) | Kolde, 2019 | ||
Software, algorithm | Numpy (version 1.17.2) | Harris et al., 2020 | ||
Software, algorithm | Pandas (version 0.25.2) | McKinney, 2010 | ||
Software, algorithm | Pickle (version 4.0) | Van Rossum, 2020 | ||
Software, algorithm | Scanpy (version 1.8.2) | Wolf et al., 2018 | ||
Software, algorithm | Scipy (version 1.7.3) | Virtanen et al., 2020 | ||
Software, algorithm | Autogenes (version 1.0.4) | Aliee and Theis, 2021 |
Additional files
-
Supplementary file 1
Data quality statistics and gene ontology analysis.
(a) ChIP-sequencing sample information and read statistics. (b) Significant gene ontology terms enriched in high-fat diet (HFD)-sperm differentially expressed histone H3 lysine 4 tri-methylation (deH3K4me3) regions at promoters detected in our previous study and this study, related to Figure 2—figure supplement 1E. (c) Significant gene ontology terms enriched in HFD-sperm at regions showing a decrease in H3K4me3 at promoters, related to Figure 2—figure supplement 1E. (d) Significant gene ontology terms enriched in HFD-sperm at regions showing an increase in H3K4me3 at promoters, related to Figure 2—figure supplement 1E. (e) Significant gene ontology terms enriched in differentially expressed genes in female placentas derived from HFD-sires, related to Figure 4C. (f) Significant gene ontology terms enriched in differentially expressed genes in male placentas derived from HFD-sires, related to Figure 4D. (g) Reference single-cell RNA-sequencing data information (from Han et al., 2018) – number of cells per cell type, related to Figure 5—figure supplement 1.
- https://cdn.elifesciences.org/articles/83288/elife-83288-supp1-v2.xlsx
-
Supplementary file 2
Interactive heatmap for significant gene ontology terms enriched in high-fat diet (HFD)-sperm deH3K4me3 regions at promoters detected in our previous study (Pepin et al., 2022) and this study, related to Figure 2—figure supplement 1E.
- https://cdn.elifesciences.org/articles/83288/elife-83288-supp2-v2.png
-
Supplementary file 3
Motif analysis, showing significantly enriched known motifs in regions gaining H3K4me3 in high-fat diet (HFD)-sperm, related to Figure 3.
- https://cdn.elifesciences.org/articles/83288/elife-83288-supp3-v2.zip
-
MDAR checklist
- https://cdn.elifesciences.org/articles/83288/elife-83288-mdarchecklist1-v2.docx