Cerebral chemoarchitecture shares organizational traits with brain structure and function
Figures
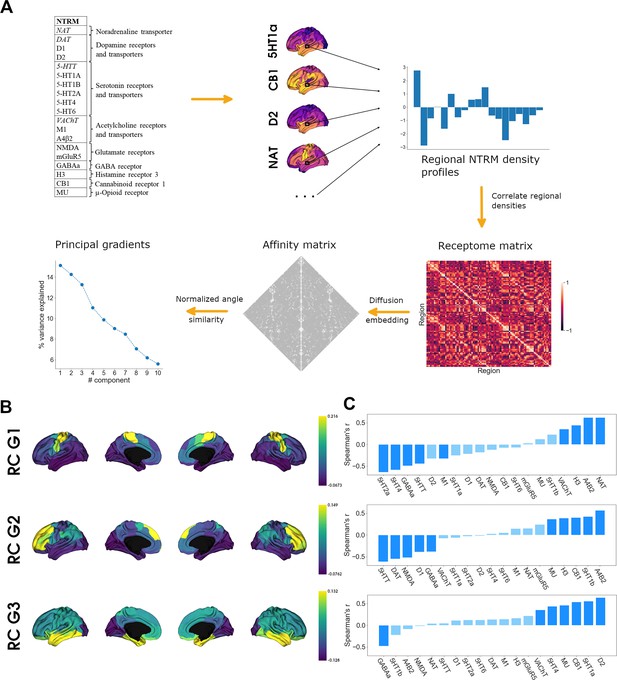
Organization of the cortical receptome.
(A) Analytic workflow of receptome generation and gradient decomposition. Node-level neurotransmitter receptor and transporter molecule (NTRM) fingerprints are derived from PET images of 19 different NTRM (in the top left, italic font denotes transporters). The fingerprints are then Spearman rank correlated to capture node-level similarity in chemoarchitectural composition, generating the receptome matrix. Next, to determine similarity between all rows of the receptome matrix, we used a normalized angle similarity kernel to generate an affinity matrix. Finally, we employ diffusion embedding, a nonlinear dimensionality reduction technique, to derive gradients of receptomic organization. (B) Receptome (RC) gradients projected on the cortical surface. Top: first receptome gradient (RC G1); middle: second receptome gradient (RC G2); bottom: third receptome gradient (RC G3). (C) Spearman rank correlations of cortical receptome gradients with individual NTRM densities. Top: first receptome gradient; middle: second receptome gradient; bottom: third receptome gradient. Saturated blue coloring corresponds to statistically significant correlations at p < 0.05.
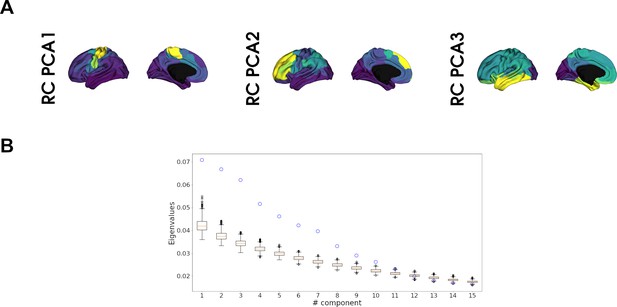
Cortical receptome gradients.
(A) The first three chemoarchitectural similarity axes generated by principal component analysis (PCA). Spearman rank correlations to the respective gradients exceeds r > 0.99, indicating a high similarity between axes derived using linear and nonlinear dimensionality reduction methods. PCA-derived chemoarchitectural similarity axes replicate findings by Hansen et al., 2022. (B) Significance of receptome gradients compared to gradients derived from randomized neurotransmitter receptor and transporter molecule (NTRM) density profiles. The blue circles indicate the eigenvalues of the true components, the boxplots display the eigenvalues of components resulting from performing gradient decomposition of n = 1000 randomized receptomes. Up to the 11th component, the true components display significantly higher eigenvalues (p<0.05).
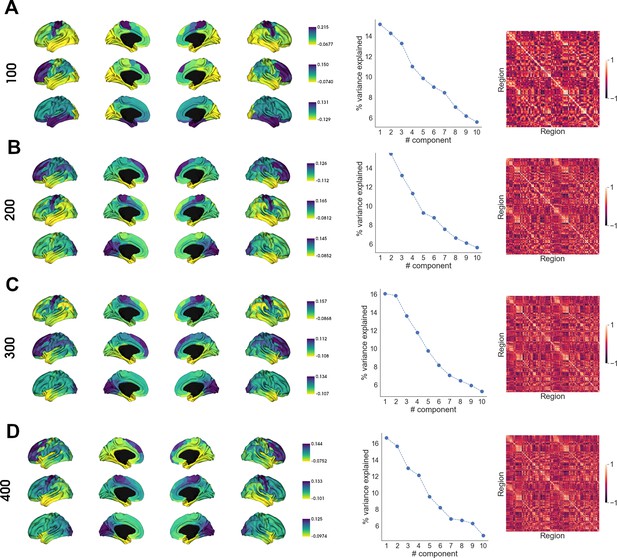
Robustness of receptome gradients.
Robustness of receptome gradient decomposition across different parcellation granularities. Left: RC G1, RC G2 and RC G3 (top-to-bottom) projected on the cortical surface. Middle: variance explained by gradient decomposition. Right: receptome matrix. (A) 100 parcels, (B) 200 parcels, (C) 300 parcels, and (D) 400 parcels.
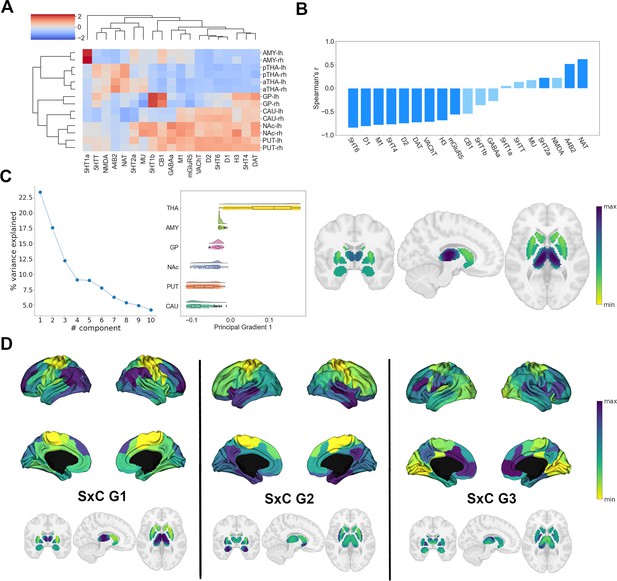
Organization of subcortical chemoarchitecture.
(A) Hierarchical agglomerative clustering of neurotransmitter receptor and transporter molecule (NTRM) densities in subcortical structures. aTHA: anterior thalamus; pTHA: posterior thalamus. (B) Spearman rank correlations of the first subcortical receptome gradient with individual NTRM densities. Saturated blue coloring corresponds to statistically significant correlations at p < 0.05. (C) Gradient decomposition of the subcortical receptome. Left: percentage of variance explained by components following gradient decomposition. Middle: value distribution of the first subcortical receptome gradient across subcortical structures. CAU: caudate nucleus; PUT: putamen; NAc: accumbens nucleus; GP: pallidal globe; AMY: amygdala; THA: thalamus. Right: subcortical projection of the first subcortical receptome gradient. (D) Gradients of the subcortico-cortical receptome projected to the cortical surface and to subcortical nuclei.
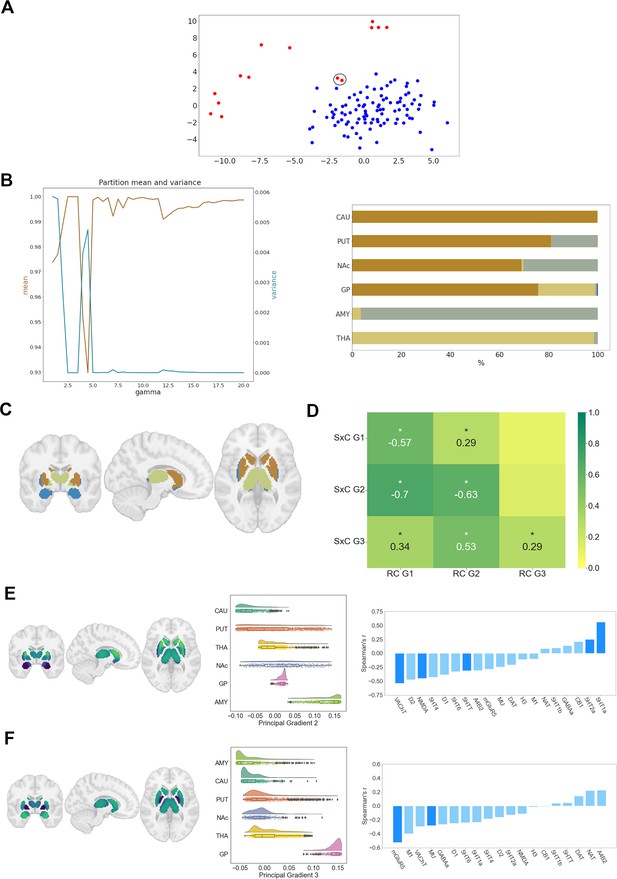
Subcortical receptome.
(A) MDS projection of cortico-subcortical similarity of chemoarchitectural composition. Subcortical nuclei are displayed in red, the cortex displayed in blue. The amygdala is encircled in black. (B) Leiden clustering of the subcortical receptome. Left: mean and variance of z-rand scores across Leiden algorithm partition resolutions. Note that the clustering results are very stable across gammas. Right: distribution of a stable partition at gamma = 2.5 across subcortical structures. (C) Projection of a stable partition at gamma = 2.5 to subcortical structures. (D) Spearman rank correlation of subcortico-cortical to cortical receptome gradients. (E) Receptome gradient decomposition of the subcortical receptome. Left: subcortical projection of the second gradient of the subcortical receptome. Middle: distribution of sRC G2 values across subcortical structures. Right: Spearman rank correlations of sRC G2 values with individual neurotransmitter receptor and transporter molecule (NTRM) densities. Saturated blue coloring corresponds to statistically significant correlations at p < 0.05. (F) Receptome gradient decomposition of the subcortical receptome. Left: subcortical projection of the third gradient of the subcortical receptome. Middle: distribution of sRC G3 values across subcortical structures. Right: Spearman rank correlations of sRC G3 values with individual NTRM densities. Saturated blue coloring corresponds to statistically significant correlations at p < 0.05.

Robustness of agglomerative hierarchical clustering – subcortex.
Replication of agglomerative hierarchical clustering of average neurotransmitter receptor and transporter molecule (NTRM) densities in subcortical structures, using Euclidean distance and different linkage methods.
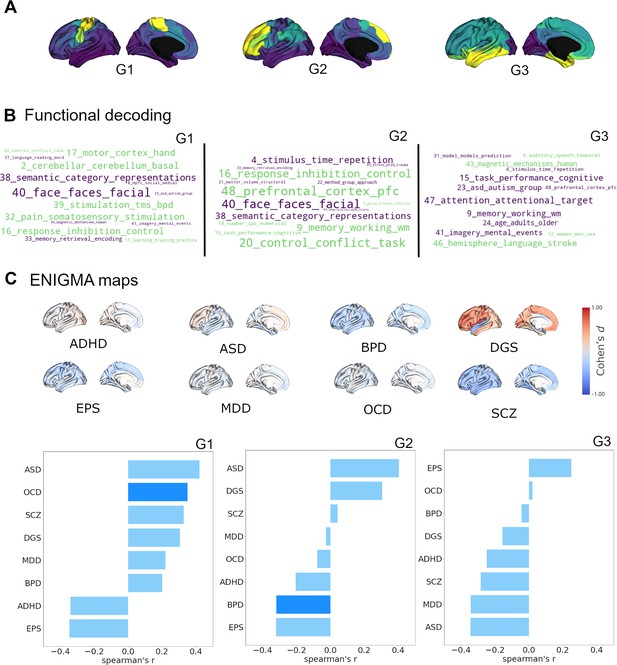
Cortical receptome gradients in term-based functional activation and disorder.
(A) Cortical receptome gradients projected to the cortical surface. (B) Functional decoding of cortical receptome gradients. Wordclouds display positive and negative correlations of receptome gradients and topic-based functional activation patterns. Word sizes encode absolute correlation strength, word colors are matched to the respective gradient poles. Only statistically significant correlations (p<0.05) are displayed. Left: RC G1; middle: RC G2; right: RC G3. (C) Disease decoding of cortical receptome gradients. Surface plots: effect size (Cohen’s d) of cortical thickness alterations in central nervous system disorders in patients vs. controls. Bar plots: Spearman rank correlations of receptome gradients and cortical thickness alterations. Saturated blue coloring corresponds to statistically significant correlations at p < 0.05. Left: RC G1; middle: RC G2; right: RC G3.
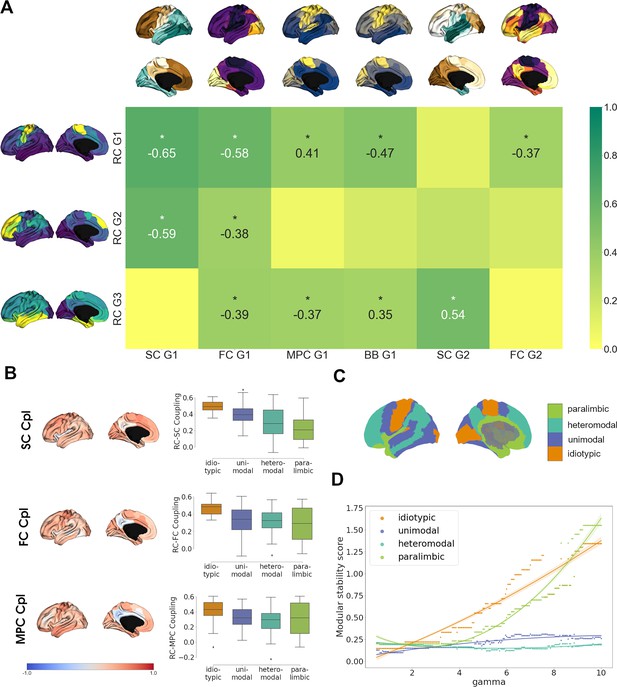
Multimodal contextualization of the cortical receptome.
(A) Correlation strengths of cortical receptome gradients to functional connectivity (FC), structural connectivity (SC), microstructural profile covariance (MPC), and BigBrain gradients. Coloring is scaled to absolute values. Surface-projected gradients are displayed next to their respective rows and columns. Asterisks indicate statistically significant correlations at p < 0.05. (B) Coupling of the cortical receptome to SC, FC, and MPC. Left: surface projection of coupling strengths. Right: coupling strengths across cytoarchitectural classes. (C) Surface projection of Mesulam cytoarchitectural classes. (D) Modular stability of receptome clustering in Mesulam cytoarchitectural classes, reflecting the heterogeneity of receptomic profile.
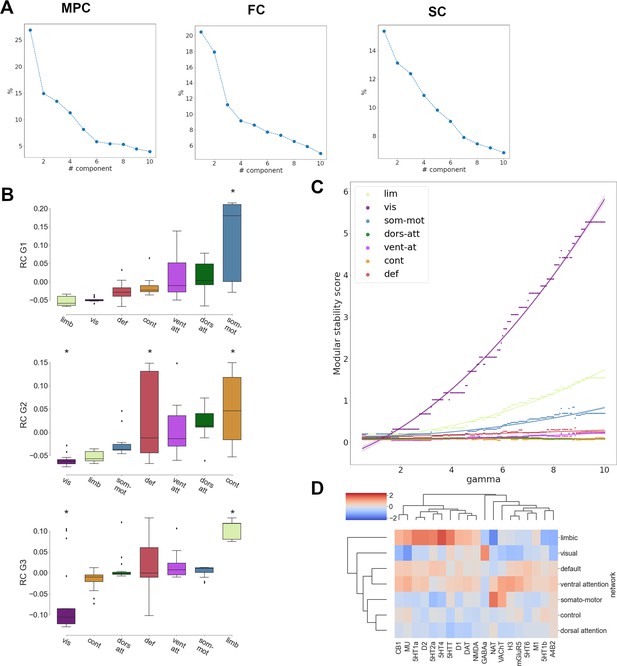
Contextualization of receptome gradients in hierarchical brain organization.
(A) Variance explained by gradient decomposition. Left: microstructural profile covariance (MPC); middle: functional connectivity (FC); right: structural connectivity (SC). (B) Distribution of RC G1 (top), RC G2 (middle), and RC G3 (bottom) values across functional networks. Asterisks indicate statistically significant alignments at p < 0.05. (C) Modular stability of receptome similarity clustering in functional networks. (D) Hierarchical clustering, using Euclidean distance and the Weighted Pair group Method with Arithmethic Mean (WPGMA) algorithm, of neurotransmitter receptor and transporter molecule (NTRM) fingerprints in functional networks.
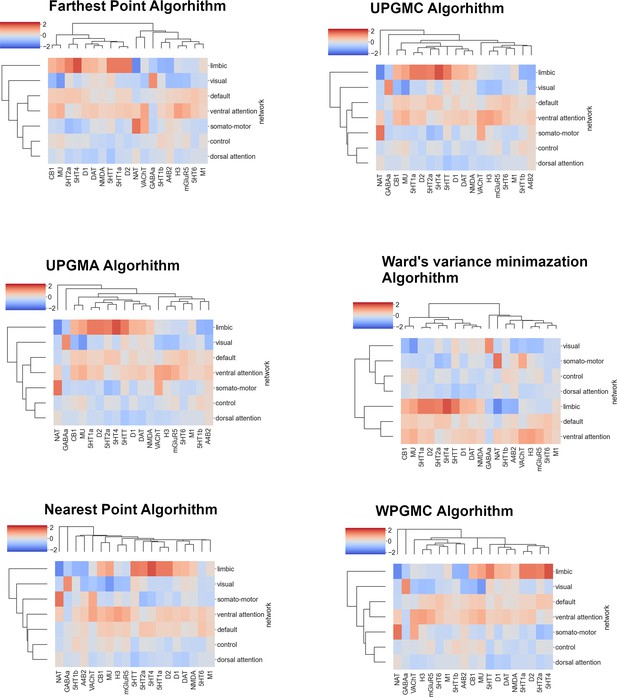
Robustness of agglomerative hierarchical clustering – cortex.
Replication of agglomerative hierarchical clustering of average neurotransmitter receptor and transporter molecule (NTRM) densities in functional networks, using Euclidean distance and different linkage methods.
Additional files
-
Supplementary file 1
Table S1.
Neurotransmitter receptors and transporters included in analyses. BPND, non-displaceable binding potential; VT, tracer distribution volume; Bmax, density (pmol/ml) converted from binding potential or distributional volume using autoradiography-derived densities; SUVR, standard uptake value ratio. Neurotransmitter receptor maps without citations refer to unpublished data. Table adapted from Hansen et al., 2022.
- https://cdn.elifesciences.org/articles/83843/elife-83843-supp1-v2.docx
-
Supplementary file 2
Table S2A.
Replication of multimodal receptome gradient contextualization through correlation using a Schaefer granularity of 100 parcels.
- https://cdn.elifesciences.org/articles/83843/elife-83843-supp2-v2.xlsx
-
Supplementary file 3
Table S2B.
Replication of multimodal receptome gradient contextualization through correlation using a Schaefer granularity of 200 parcels.
- https://cdn.elifesciences.org/articles/83843/elife-83843-supp3-v2.xlsx
-
Supplementary file 4
Table S2C.
Replication of multimodal receptome gradient contextualization through correlation using a Schaefer granularity of 300 parcels.
- https://cdn.elifesciences.org/articles/83843/elife-83843-supp4-v2.xlsx
-
Supplementary file 5
Table S2D.
Replication of multimodal receptome gradient contextualization through correlation using a Schaefer granularity of 400 parcels.
- https://cdn.elifesciences.org/articles/83843/elife-83843-supp5-v2.xlsx
-
MDAR checklist
- https://cdn.elifesciences.org/articles/83843/elife-83843-mdarchecklist1-v2.pdf