Tissue libraries enable rapid determination of conditions that preserve antibody labeling in cleared mouse and human tissue
Figures
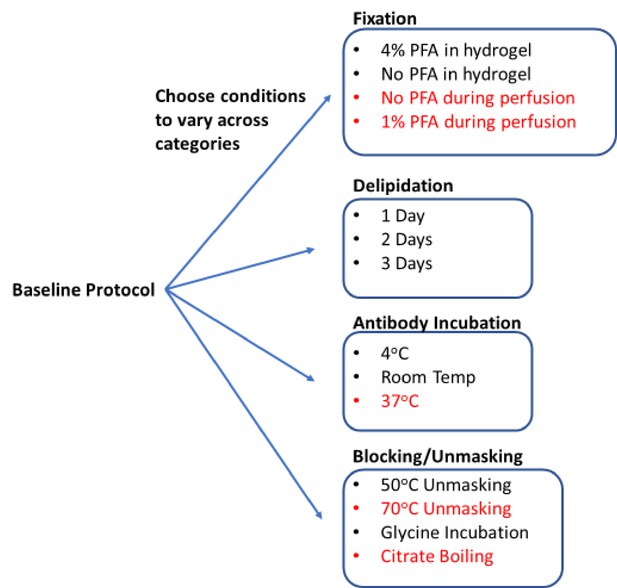
Process for generating a library of tissue prepared with different protocols.
Start with a baseline protocol (see Materials and methods). Choose conditions to test that can be separated into categories believed to influence staining quality. Prepare tissue by varying only one or two conditions from the baseline at a time. Each protocol should only vary by a single condition from at least one other protocol to allow for a complete understanding of how each change influences staining. So resulting immunohistochemistry (IHC) quality can isolate the effect of each condition change. Stored library of cleared tissues then allows for rapid antibody testing to quickly determine optimal IHC conditions for each antibody as well as protocols that allow for multiplexed IHC. We consistently found conditions in red to have exclusively negative effects on IHC or tissue integrity, so they were excluded from testing with most antibodies.
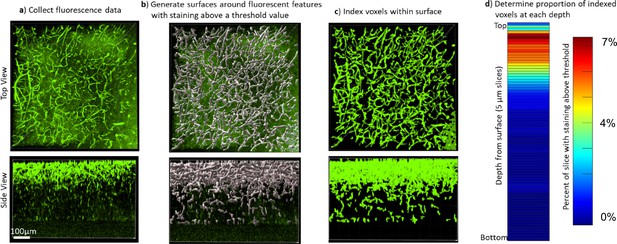
Quantifying the staining quality for comparison across protocols and antibodies.
Immunohistochemistry (IHC) quality comparisons can be difficult to determine by eye due to differences in fluorescence intensity and background, as well as the many ways that software can modify the visual representation of data, so we created a pipeline to quickly quantify staining quality in a way that allows rapid comparison. (a) First, a confocal or lightsheet microscope is used to image a Z-stack throughout the entire thickness of the sample. All imaging parameters are kept identical for a given antibody/fluorophore to allow for comparison between protocols. (b) Fluorescence values were normalized across the sample, then a rolling-ball algorithm was used in Imaris software to generate surfaces around fluorescent features with intensity above a threshold value. Alternatively, segmentation masks were generated using Ilastik following their pixel classifier workflow. (c) The voxels within the surface were indexed to define the array of voxels with and without staining that could then be analyzed in Matlab. (d) The staining quality was quantified and visualized by plotting the ratio of voxels with staining over the total number of voxels in a plane at that depth in the image, the average signal intensity within the segmented region, and the average noise intensity outside of the segmented region (Figure 3—figure supplements 2 and 3, Figure 7—figure supplements 1 and 2). A higher proportion of indexed voxels, higher signal, lower noise, and longer depth to reach half-max staining intensity all represent better staining quality. This can also be used to provide visual representation of reproducibility (Figure 3—figure supplements 4–6). It is also possible to reduce these numbers into a figure of merit that can be quantitatively compared between conditions (Figure 3c, Figure 3—figure supplement 7).
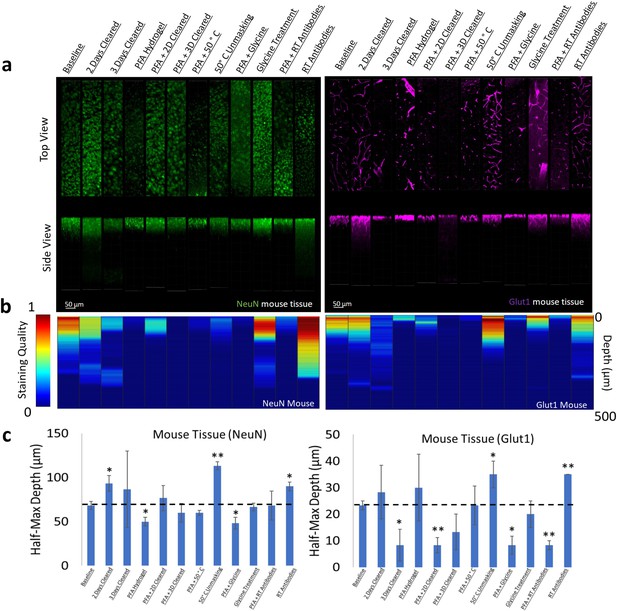
Influence of common conditions on immunohistochemistry in mouse brain tissue.
(a) Representative maximum intensity projection views from the top and side of 500 µm thick, 5 mm wide tissue sections. C57Bl/6J mouse isocortex was processed, stained, and imaged simultaneously with NeuN (green, left panels) and Glut1 (purple, right panels). (b) Quantitative comparison of immunohistochemistry labeling across conditions using Imaris. A rolling-ball subtraction was used to determine the area with signal-to-noise above cut-off for every image plane in the Z-stack. The proportion of indexed voxels in each plane was then divided by the maximum value across all conditions to generate a normalized staining quality score, which allows for quick visual assessment of staining quality differences with depth and between conditions. (c) Quantitative comparison of the depth at which the immunohistochemistry (IHC) staining intensity becomes half of the maximum, using segmentation results from Ilastik. Error bars represent standard error. Two-tailed t-test comparing results to baseline were represented with p<0.05 = *, p<0.01 = **, and no significance = unlabeled. N=3 for all samples.
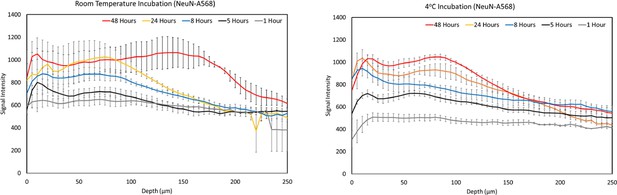
Immunohistochemistry staining depth over time.
Average signal intensity of immunohistochemistry labeling with NeuN-A568 in mouse cortex tissue prepared using baseline conditions, with antibodies incubated at room temperature (left) and 4°C (right) with gentle shaking. For each time point, n=3 independently prepared sections from different mice. Error bars represent standard error of the mean.
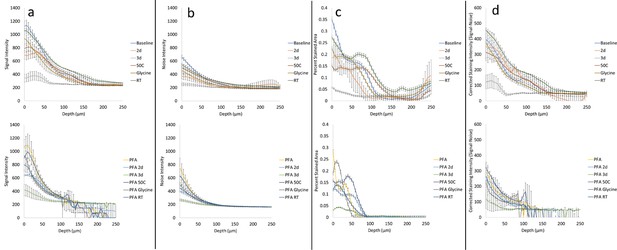
Immunohistochemistry performance of NeuN in mouse tissue.
Quantification of the (a) average signal intensity, (b) average background noise intensity, (c) percent of segmented area, (d) and the average corrected staining intensity versus depth from the tissue surface. Signal, noise, and corrected signal were normalized to the amount of staining in each imaging plane by dividing the percent segmented area in that plane. Immunohistochemistry labeling was conducted with NeuN-A568 in mouse cleared tissue library with three independently prepared sections from different mice for each condition.For each condition n=3 independently prepared sections from different mice. Error bars represent standard error of the mean.
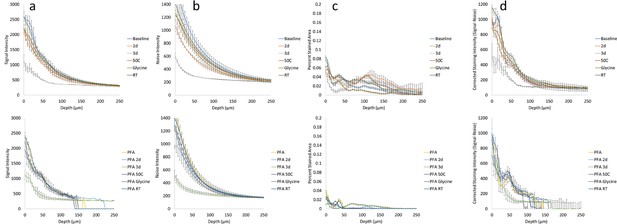
Immunohistochemistry performance of Glut1 in mouse tissue.
Quantification of the (a) average signal intensity, (b) average background noise intensity, (c) percent of segmented area, (d) and the average corrected staining intensity versus depth from the tissue surface. Signal, noise, and corrected signal were normalized to the amount of staining in each imaging plane by dividing the percent segmented area in that plane. Immunohistochemistry labeling was conducted with Glut1-A568 in mouse cleared tissue library with three independently prepared sections from different mice for each condition.For each condition n=3 independently prepared sections from different mice. Error bars represent standard error of the mean.
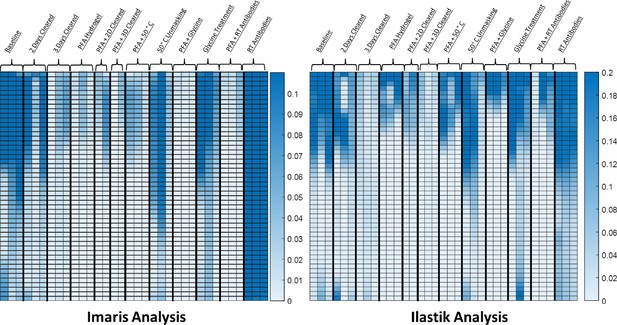
Reproducibility of NeuN immunohistochemistry and analysis in mouse brain tissue.
Quantitative comparison of immunohistochemistry labeling across conditions. N=3 independently prepared tissue sections from different mice for each condition. All conditions were tested with one section each from the same three mice. (Left) For the Imaris analysis, a rolling-ball subtraction was used to determine the area with signal-to-noise above cut-off for every image plane in the Z-stack. (Right) For the Ilastik analysis, the staining was segmented using their pixel classifier workflow. In short, a paintbrush was used to draw over the signal and background to help train the classifier on how to segment each image. Real-time segmentation results were then improved using a minimum of 600 image planes from six different staining conditions that reflect the distribution of staining quality in the entire set. All images were then batch processed through the trained pixel classifier.
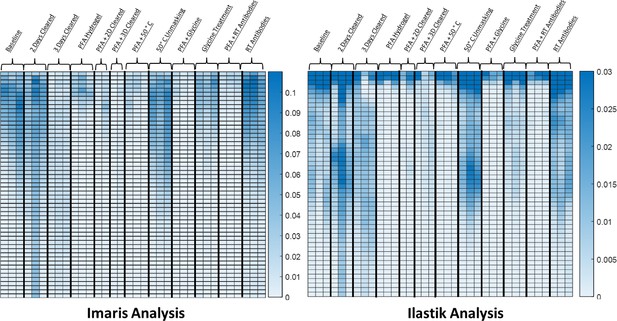
Reproducibility of Glut1 immunohistochemistry and analysis in mouse brain tissue.
Quantitative comparison of immunohistochemistry labeling across conditions. N=3 independently prepared tissue sections from different mice for each condition. All conditions were tested with one section each from the same three mice, with each condition having every condition having. (Left) For the Imaris analysis, a rolling-ball subtraction was used to determine the area with signal-to-noise above cut-off for every image plane in the Z-stack. (Right) For the Ilastik analysis, the staining was segmented using their pixel classifier workflow. In short, a paintbrush was used to draw over the signal and background to help train the classifier on how to segment each image. Real-time segmentation results were then improved using a minimum of 600 image planes from six different staining conditions that reflect the distribution of staining quality in the entire set. All images were then batch processed through the trained pixel classifier.
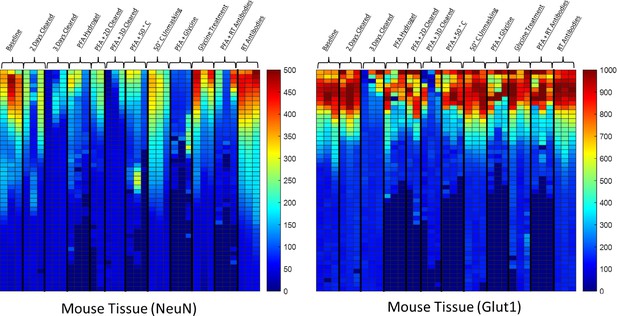
Reproducibility of NeuN and Glut1 immunohistochemistry corrected signal intensity in mouse brain tissue.
Segmentation results from ilastik were used to determine the signal within the segmented area as well as the noise outside of the segmented area. The noise values were subtracted from the signal value to visually represent the staining intensity value over noise. These change with depth in a notably different way than the segmented area (Figure 3—figure supplements 4 and 5) which suggests that conditions differentially influence the amount of staining and the intensity of staining.
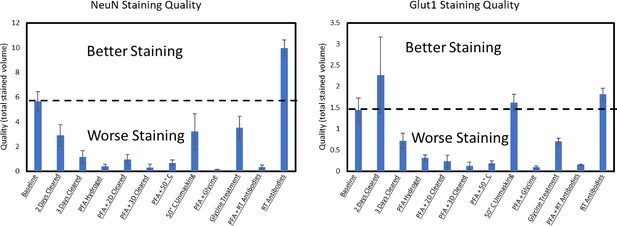
Reducing comparison of total stained volume to one dimension.
Quantitative comparison of immunohistochemistry labeling across conditions using Imaris analysis. A rolling-ball subtraction was used to determine the area with signal-to-noise above cut-off for every image plane in the Z-stack. The total area in each plane with fluorescence above the threshold value was then summed to reduce the dimensionality of comparison and allow for a comparison between conditions with a single number as the figure of merit. This comparison in staining quality is only appropriate when the target antigen is consistently distributed throughout the tissue, which may not always be the case. For each condition n=3 independently prepared sections from different mice. Error bars represent standard error of the mean.
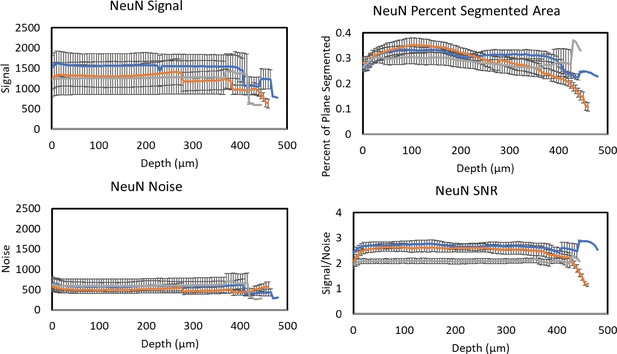
Immhunohistochemistry staining consistency across brain regions.
Average signal intensity of immunohistochemistry labeling with NeuN-A568 in ~500 µm thick mouse cortex tissue prepared using optimized conditions and incubated with antibodies for 3 days. n=6 independently prepared longitudinal sections from three different mice. Error bars represent standard error of the mean. Each section was imaged at the hippocampus (blue), posterior cortex (gray), and anterior cortex (orange).
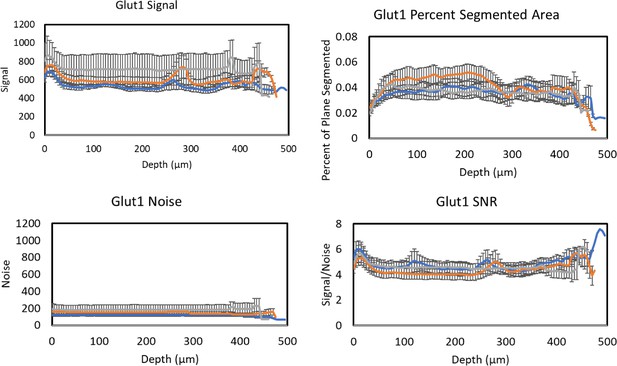
Immhunohistochemistry staining consistency across brain regions.
Average signal intensity of immunohistochemistry labeling with Glut1-A488 in ~500 µm thick mouse cortex tissue prepared using optimized conditions and incubated with antibodies for 3 days. n=6 independently prepared longitudinal sections from three different mice.Error bars represent standard error of the mean. Each section was imaged at the hippocampus (blue), posterior cortex (gray), and anterior cortex (orange).
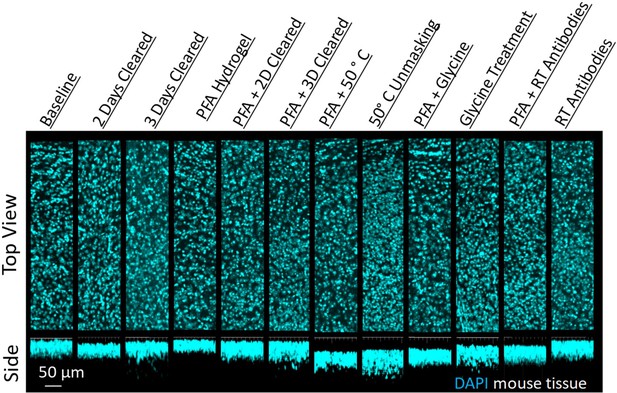
Influence of common conditions on 4′,6-diamidino-2-phenylindole (DAPI) staining.
Representative maximum intensity projection views from the top and side of 500 µm thick, 5 mm wide tissue sections of C57Bl/6J mouse isocortex stained with DAPI.
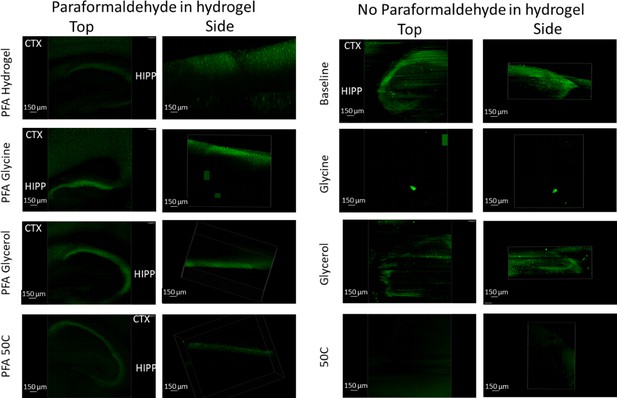
Immunohistochemistry of dopamine receptor 2 in mouse brain tissue.
Representative maximum intensity projection views from the top and side of 1 mm thick sagittal slices of C57Bl/6J mouse brain. Dopamine receptor 2 (green) shows significantly better fluorescent labeling in both the cortex (CTX) and hippocampus (HIPP) when the tissue is prepared with paraformaldehyde (PFA) in hydrogel.
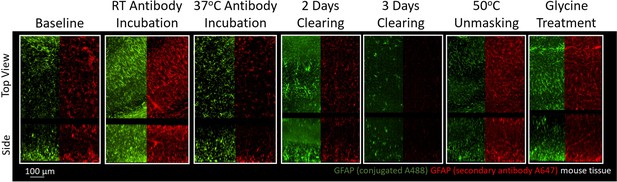
Immunohistochemistry of two different antibodies for GFAP.
Representative maximum intensity projection views from the top and side of 500 µm thick, 5 mm wide tissue sections of C57Bl/6J mouse isocortex stained with two different antibodies for GFAP. Monoclonal GA5 conjugated to Alexa Fluor 488 (green) was stained and imaged in the same tissue as a polyclonal antibody using a goat anti-rabbit secondary conjugated to Alexa Fluor 647 (red). Other protocols show no significant labeling (Figure 5—figure supplement 1).
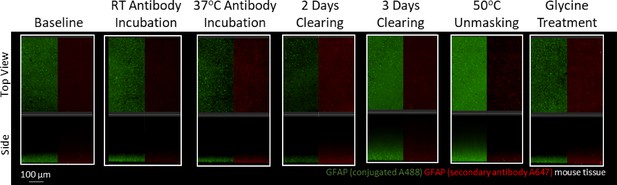
Immunohistochemistry with GFAP antibodies in with paraformaldehyde (PFA)-hydrogelled mouse brain tissue.
Representative maximum intensity projection views from the top and side of 500 µm thick, 5 mm wide tissue sections of C57Bl/6J mouse isocortex stained with two different antibodies for GFAP. Monoclonal GA5 conjugated to Alexa Fluor 488 (green) was stained and imaged in the same tissue as a polyclonal antibody using a goat anti-rabbit secondary conjugated to Alexa Fluor 647 (red).
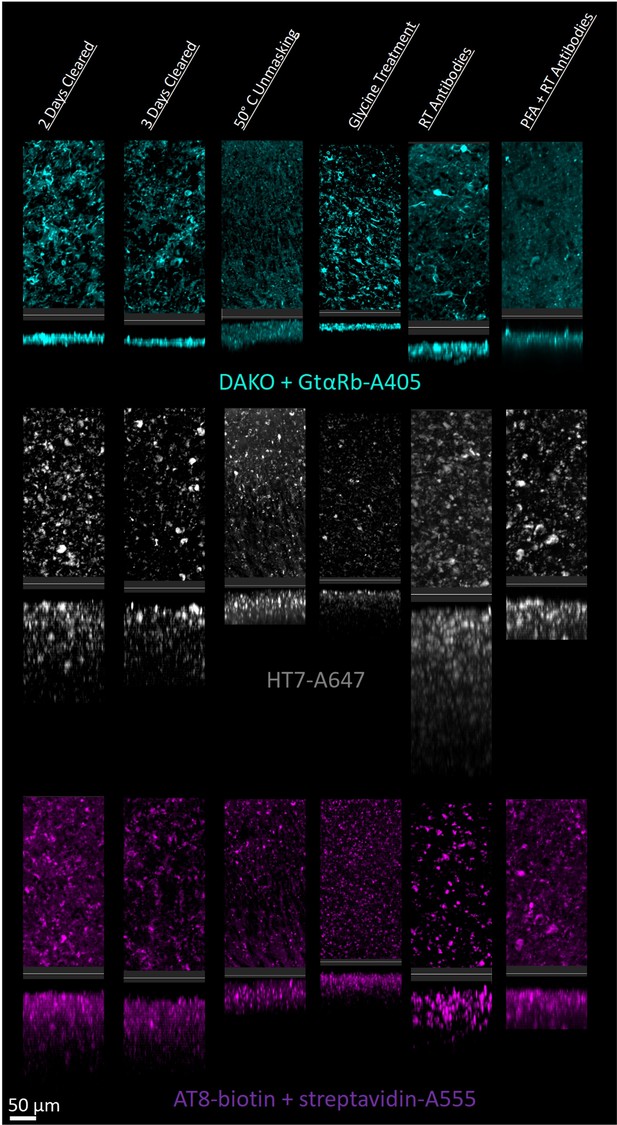
Tau immunohistochemistry in cleared human tissue.
Representative maximum intensity projection views from the top and side of 500 µm thick, 5 mm wide tissue sections. Other protocols showed worse staining quality (Figure 6—figure supplement 2).
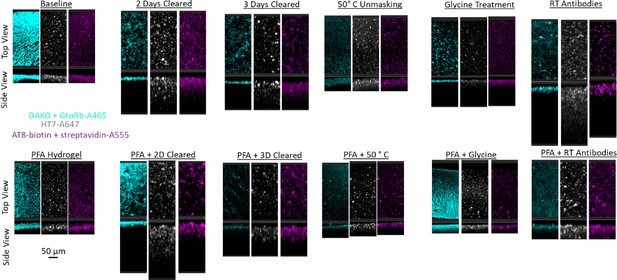
Additional Dako, AT8, and HT7 tau immunohistochemistry conditions in human brain tissue.
Representative maximum intensity projection views from the top and side of 500 µm thick, 5 mm wide tissue sections from frozen human tissue samples.
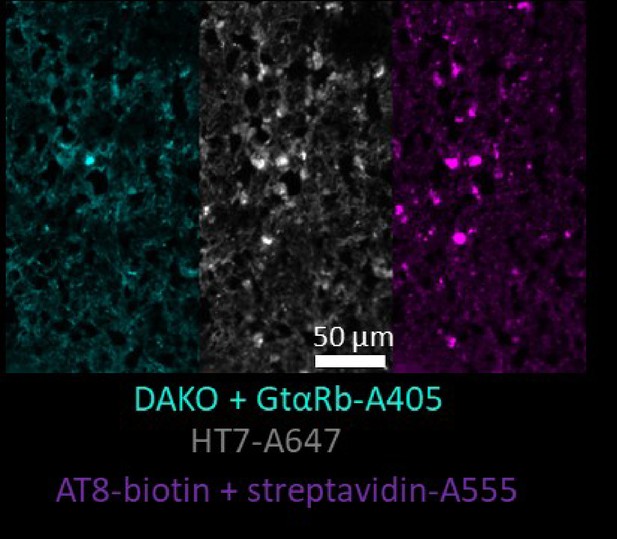
Representative microtome section images taken from the human tissue prior to clearing.
Human tissue was stained with Dako, AT8, and HT7 to confirm the presence of their target tau prior to testing large-volume immunohistochemistry (IHC) conditions.
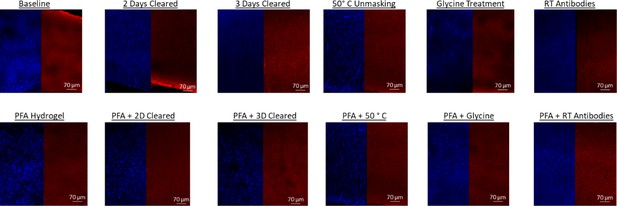
AT8 and HT7 immunohistochemistry in mouse tissue expressing human tau (Htau).
Representative maximum intensity projection views from the top of 500 µm thick, 5 mm wide tissue sections of HTau mouse isocortex AT8 (blue) and HT7 (red) show labeling of tau in mice expressing human tau in the same conditions that show staining in human tissue.
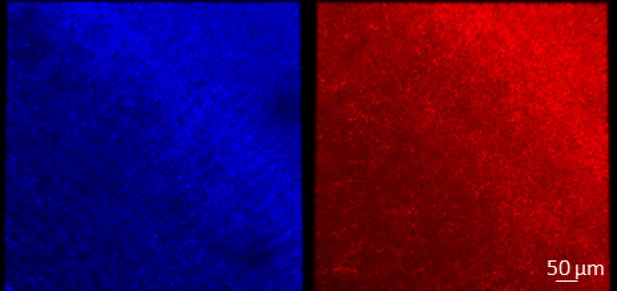
AT8 and HT7 immunohistochemistry in tau knockout mice (Htau KO).
Representative maximum intensity projection views from the top of 500 µm thick, 5 mm wide tissue sections of Htau knockout mouse isocortex. AT8 (blue) and HT7 (red) show no labeling of tau in knockout mice using the same baseline conditions where they show labeling in Htau mice expressing human tau.
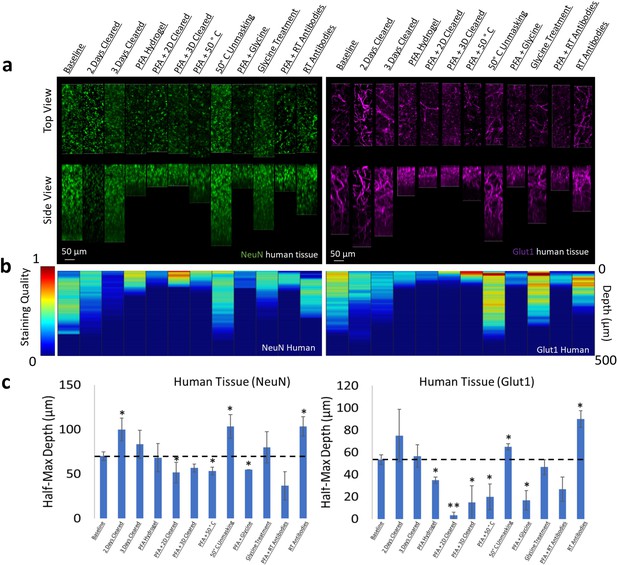
Influence of common conditions on immunohistochemistry in human brain tissue.
(a) Representative maximum intensity projection views from the top and side of 500 µm thick, 5 mm wide tissue sections. Tissue was processed, stained, and imaged simultaneously with NeuN (green, left panels) and Glut1 (purple, right panels). (b) Quantitative comparison of immunohistochemistry labeling across conditions using Imaris. A rolling-ball subtraction was used to determine the area with signal-to-noise above cut-off for every image plane in the Z-stack. The area in each plane was then divided by the maximum value across all conditions to provide a staining quality score, which allows for quick visual assessment of staining quality differences with depth and between conditions. (c) Quantitative comparison of the depth at which the immunohistochemistry (IHC) staining intensity becomes half of the maximum, using segmentation results from Ilastik. Error bars represent standard error. Two-tailed t-test comparing results to baseline were represented with p<0.05 = *, p<0.01 = **, and no significance = unlabeled. N=3 for all samples.
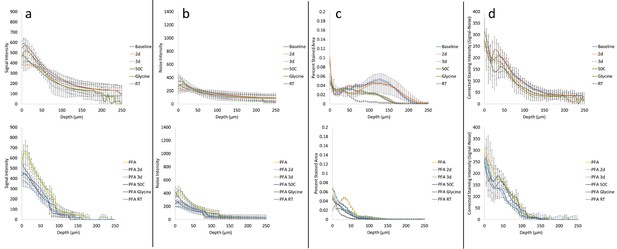
Immunohistochemistry performance of NeuN in human tissue.
Quantification of the (a) average signal intensity, (b) average background noise intensity, (c) percent of segmented area, (d) and the average corrected staining intensity versus depth from the tissue surface. Signal, noise, and corrected signal were normalized to the amount of staining in each imaging plane by dividing the percent segmented area in that plane. Immunohistochemistry labeling was conducted with NeuN-A568 in human cleared tissue library with three independently prepared sections from different donors for each condition. For each condition n=3 independently prepared sections from different mice. Error bars represent standard error of the mean.
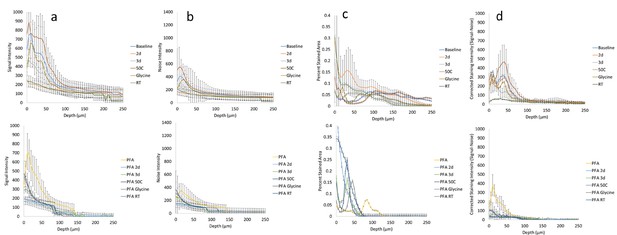
Immunohistochemistry performance of Glut1 in human tissue.
Quantification of the (a) average signal intensity, (b) average background noise intensity, (c) percent of segmented area, (d) and the average corrected staining intensity versus depth from the tissue surface. Signal, noise, and corrected signal were normalized to the amount of staining in each imaging plane by dividing the percent segmented area in that plane. Immunohistochemistry labeling was conducted with Glut1-A488 in human cleared tissue library with three independently prepared sections from different donors for each condition. For each condition n=3 independently prepared sections from different mice. Error bars represent standard error of the mean.
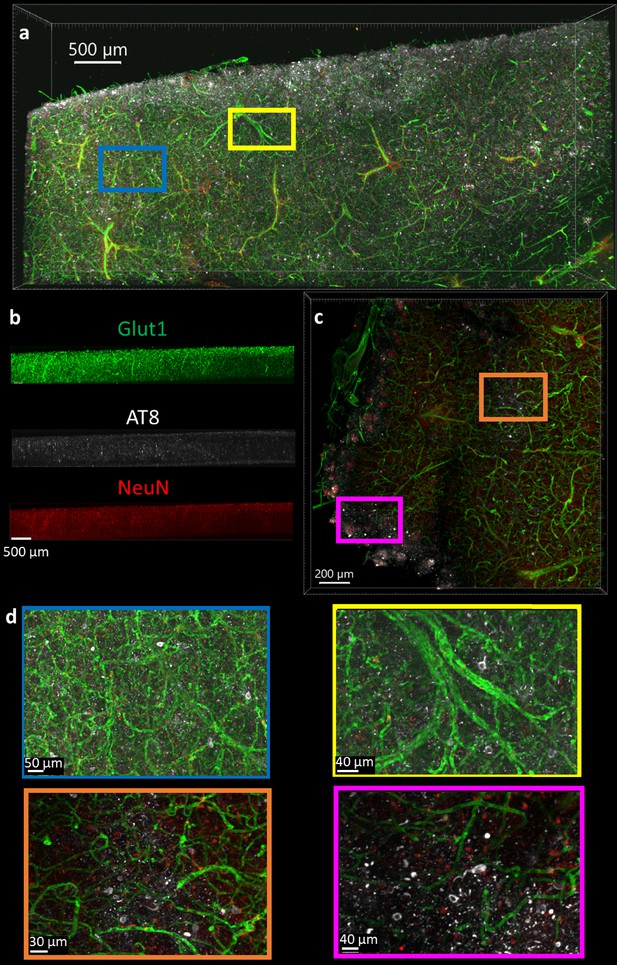
Immunohistochemistry in 1 mm thick human tissue.
The tissue was crosslinked without paraformaldehyde (PFA), cleared for 2 days, rinsed, then the antibodies NeuN-A568 (red), Glut1-A488 (green), and AT8-A647 (white) were incubated with the tissue for 14 days. (a) Top-down view of fluorescence from 1 mm section human brain. (b) Side view of each antibody fluorescence through entire section depth. (c) A 200 µm virtual section of human tissue, from a 1 mm thick sample. (d) Zoomed-in regions from the full images show that AT8-labeled tau tangles are present at multiple locations throughout the human brain sections.
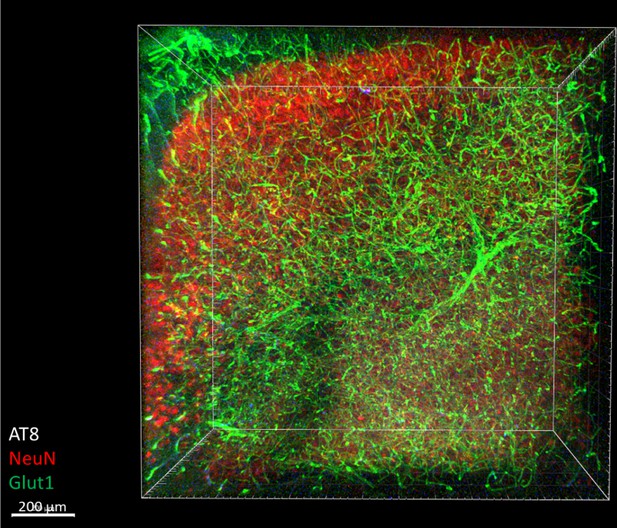
Immunohistochemistry in 1 mm mouse tissue.
The tissue was crosslinked without paraformaldehyde (PFA), cleared for 2 days, rinsed, then the antibodies NeuN-A568 (red), Glut1-A488 (green), and AT8-A647 (blue) were incubated with the tissue for 14 days. A small section of the total stained tissue was imaged and shows complete staining throughout the entire thickness of the sample.
Additional files
-
Supplementary file 1
Table with additional information describing ADRC human tissue samples.
- https://cdn.elifesciences.org/articles/84112/elife-84112-supp1-v3.docx
-
Transparent reporting form
- https://cdn.elifesciences.org/articles/84112/elife-84112-transrepform1-v3.pdf
-
MDAR checklist
- https://cdn.elifesciences.org/articles/84112/elife-84112-mdarchecklist1-v3.pdf
-
Source code 1
ImageJ macro ‘SplitOirToTiff.ijm’ to convert multichannel tiff to single channel prior to conversion to HDF5 for use in Ilastik.
- https://cdn.elifesciences.org/articles/84112/elife-84112-code1-v3.zip
-
Source code 2
ImageJ macro ‘ConvertTifToH5.ijm’ to convert multipage tif to HDF5 for use in Ilastik.
- https://cdn.elifesciences.org/articles/84112/elife-84112-code2-v3.zip
-
Source code 3
ImageJ macro ‘ConvertH5toTiff.ijm’ to convert Ilastik segmentation results from HDF5 to multipage tiff to for use in Matlab analysis code.
- https://cdn.elifesciences.org/articles/84112/elife-84112-code3-v3.zip
-
Source code 4
Matlab code ‘IlastikStainingIntensity.mlx’ to input Ilastik or Imaris segmentation results in multipage tiff format and output staining signal, noise, percent, and signal-noise ratio for each imaging plane.
- https://cdn.elifesciences.org/articles/84112/elife-84112-code4-v3.zip
-
Source code 5
Matlab code ‘FindHalfMaxAndPlot.mlx’ to run after ‘IlastikStainingIntensity.mlx’, which finds the imaging plane at the top of the sample, finds the half-max depth, unblinds data, then provides tables and plots that describe the results.
- https://cdn.elifesciences.org/articles/84112/elife-84112-code5-v3.zip