Inconsistencies between human and macaque lesion data can be resolved with a stimulus-computable model of the ventral visual stream
Figures
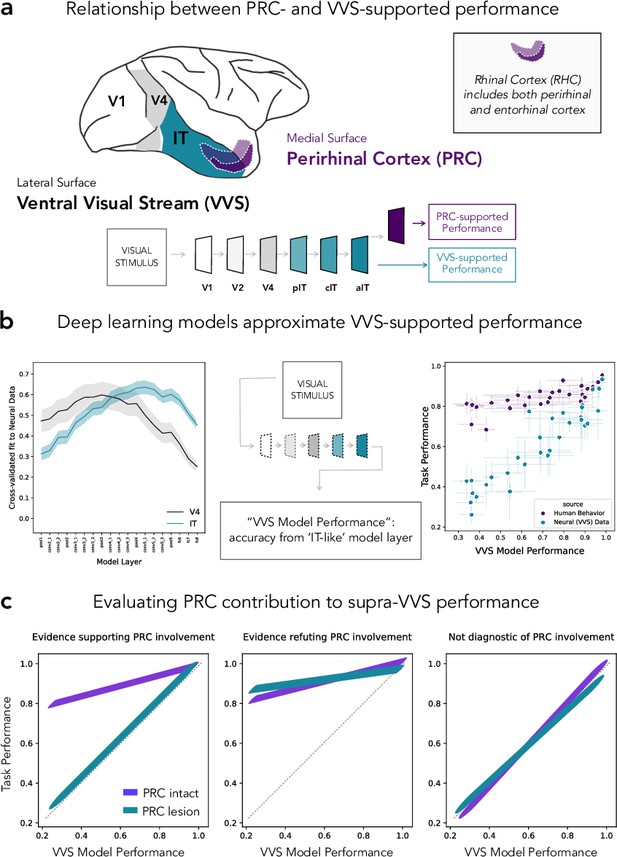
Formalizing medial temporal lobe (MTL) involvement in visual object perception.
(a) Perirhinal cortex (PRC) is an MTL structure situated at the apex of the primate ventral visual stream (VVS), located within rhinal cortex (RHC; see inset). (b) To formalize PRC involvement in visual object perception, here we leverage a computational model able to make predictions about VVS-supported performance directly from experimental stimuli. Early model layers best fit electrophysiological recordings from early stages of processing within the VVS (i.e. V4; left, gray); later layers best fit later stages of processing from the VVS (i.e. IT; left, green). We approximate VVS-supported performance by extracting responses from an ‘IT-like’ model layer (center). Our protocol approximates VVS-supported performance (right; green) while human participants nonetheless outperform model/VVS performance (Bonnen et al., 2021; right, purple). (c) Given that humans can outperform a linear readout of the VVS, here we schematize the pattern of lesion results that would be consistent with the PRC involvement in perception (left), results that would indicate that non-PRC brain structures are required to outperform the VVS (center), as well as results which indicate that a visual discrimination task is supported by the VVS (i.e. ‘non-diagnostic’ because no extra-VVS perceptual processing is required).
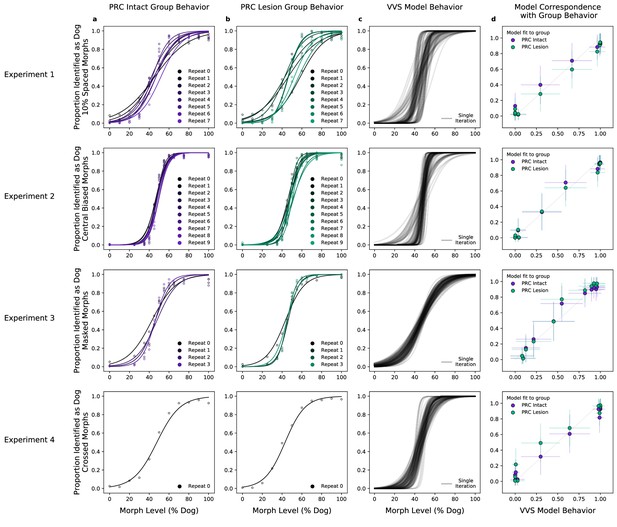
A computational proxy for the ventral visual stream (VVS) predicts perirhinal cortex (PRC)-intact and -lesioned behavior.
Averaging across subjects and morph levels (i.e. all 10% morphs, 20% morphs, etc.), (a) PRC-intact (n = 3) and (b) PRC-lesioned (n = 3) subjects exhibit a similar pattern of responses across experiments (rows 1–4). We present stimuli used in this experiment to a computational proxy for the VVS, extracting model responses from a layer that corresponds with ‘high-level’ perceptual cortex. From these model responses, we learn to predict the category membership of each stimulus, (c) testing this linear mapping on left-out images across multiple train–test iterations (black). (d) This computational proxy for the VVS accurately predicts the choice behavior of PRC-intact (purple) and -lesioned (green) grouped subjects (error bars indicate standard deviation from the mean, across model iterations and subject choice behaviors). As such, a linear readout of the VVS appears to be sufficient to perform these tasks, thus there need be no involvement of PRC to achieve neurotypical performance.
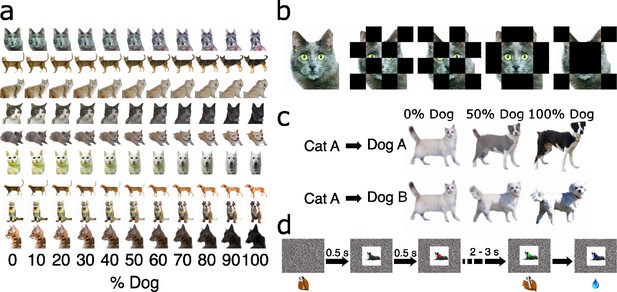
Experimental stimuli and protocol from Eldridge et al., 2018.
(a) Example stimuli from experiment 1, illustrating multiple instances of stimuli across morph levels. (b) Example stimuli used used for masked morphs, in experiment 3. (c) Example stimuli used for ‘crossed morphs’ in experiment 2. (d) Protocol for all experiments. Subject’s initiate each trial with a lever press. A stimulus is presented, followed by a red dot at the central field of view. Subjects could avoid an extended inter-trial delay by releasing the bar in the first interval (signaled by a red target) for stimuli that were less than 50% dog, and were rewarded for releasing the bar in the second interval (signaled by a green target) for stimuli that were more than 50% dog. This amounts to an asymmetrical reward structure. They were rewarded randomly for releasing during the green interval for 50–50 morphs.
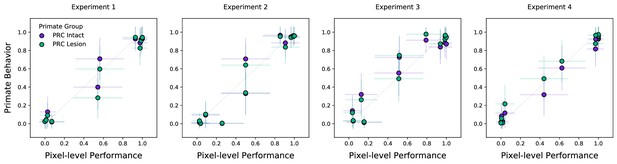
Colinearity within the stimulus set revealed by a pixel-level analysis.
Classification behaviors on this stimulus set are learned and evaluated on images with a high degree of colinearity: stimuli with similar correct answers are highly overlapping, as can be seen in the example stimuli in Figure 1. We formalize this observation by adapting previous analyses (visualized in Figure 2d): In the place of a computational proxy for the primate ventral visual stream (VVS) we use raw pixel-level representations, training a linear readout of stimulus category directly from the vectorized (i.e. flattened) images. We find that these pixel-level representations are sufficient to achieve the performance observed across experimental subjects in both perirhinal cortex (PRC)-intact and -lesioned groups. The colinearity in these data suggests that ’high-level’ representations may not be necessary to classify stimuli in these experiments, as a linear operation over the stimuli themselves achieve group-level performance. Error bars in all experiments indicate standard deviation from the mean, across model iterations and subject choice behaviors.
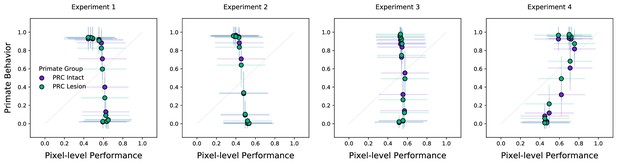
Pixel-level performance fails on a more conservative evaluation metric.
Previous evaluation of model performance employed a train–test split that was ‘naive’ to the colinearity in the available data. Here, we implement and validate a more conservative evaluation metric: To evaluate each image within a given morph sequence (i.e. a unique cat–dog combination that spans from 0% to 100% dog) we remove all instances in that morph sequence from the training data. This ensures that there are no image-adjacent stimuli that the model can exploit (e.g. training on 10% and testing on 0% within the same morph sequence). As anticipated, pixel-level classification only achieves chance performance under this more conservative evaluation metric. Error bars in all experiments indicate standard deviation from the mean, across model iterations and subject choice behaviors.
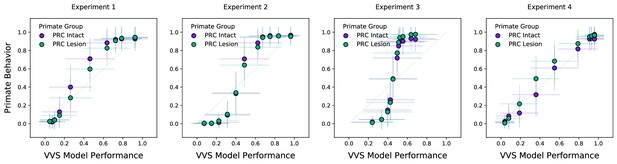
Model approximates primate behavior even with a more conservative evaluation metric.
Here, we evaluate model performance using a more conservative train–test split. While pixel-level representations in Figure 3 fail to approximate group-level performance, a computational proxy for the ventral visual stream (VVS) continues to predict group-level performance of both perirhinal cortex (PRC)-intact and -lesioned participants across experiments. Error bars in all experiments indicate standard deviation from the mean, across model iterations and subject choice behaviors.
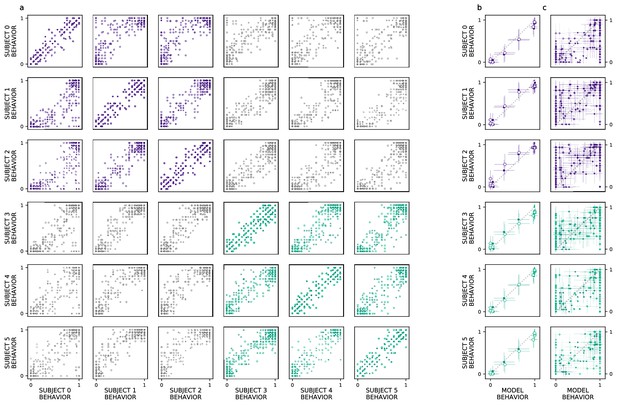
Ventral visual stream (VVS) model fits subject behavior for aggregate but not image-level metrics.
Here, we perform more granular analyses than those conducted by the authors of the original study: evaluating the model’s correspondence with perirhinal cortex (PRC)-lesioned and -intact performance at the level of individual subjects and images. We restrict ourselves to experiments that had sufficient data to determine the split-half reliability of each subject’s choice behaviors. First, we determine whether there is reliable image-level choice behavior observed for each subject, that is no longer averaged across morph levels. (a) We estimate the correspondence between subject choice behaviors over 100 split-half iterations, for both experiments 1 (closed circles) and 2 (open circles), using as a measure of fit. Each row contains a given subjects’ (e.g. subject 0, top row) correspondence with all other subjects’ choice behaviors, for PRC-intact (purple) and -lesioned (green) subjects. We find that the image-level choice behaviors are highly reliable both within (on diagonal) and between subjects (off diagonal), including between PRC-lesioned and -intact subjects (gray). We next compare model performance to the behavior of individual subjects, averaging over morph levels in accordance with previous analyses (i.e. averaging performance across all images within each morph level, e.g. 10%). (b) We observe a striking correspondence between the model and both PRC-lesioned (green) and PRC-intact (purple) performance for all subjects. (c) Finally, for each subject, we estimate the correspondence between model performance and the subject-level choice behaviors, at the resolution of individual images. Although model fits to subject behavior are statistically significant, it clearly does not exhibit ‘subject-like’ choice behavior at this resolution. Error bars in all experiments indicate standard deviation from the mean, across model iterations and subject choice behaviors.
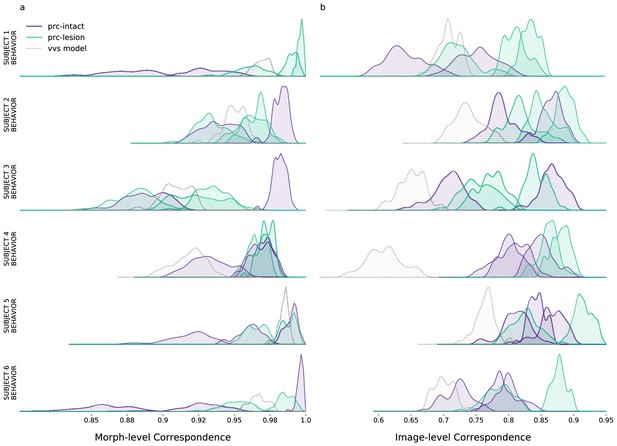
Ventral visual stream (VVS) model is ‘subject-like’ for aggregate but not image-level metrics.
We estimate the correspondence between subject–subject choice behaviors. First, we generate a random split of each subject’s performance. We then compute the between-subject correlation, iterating across 100 random splits. Each row contains a given subjects’ (e.g. subject 1, top row) correspondence with all other experimental subjects, including perirhinal cortex (PRC)-intact (purple) and -lesioned (green) monkeys. Using this same subject–subject measure, we also estimate subject–model correspondence (gray). We visualize our results at two resolutions: (a) for the morph-level analysis, we average performance across all images within each morph level (e.g. 10%, 20%, etc.; as per the analysis in Figure 3b) and compare a single subject’s behaviors to all other experimental subjects, as well as model performance; (b) for the image-level analysis we average performance across a random split of trials containing each image, for each subject, then compare each single subject’s behaviors to all other experimental subjects, as well as model performance (as outlined in Methods: Consistency estimates). For the morph-level analysis, the model choice behavior is ‘subject-like’; the distribution of model–subject correspondence is within the distribution of between-subject correspondence (in Figure 3b, subject-level choices behaviors are on the diagonal). However, at the resolution of single images, model choice behavior is not subject-like; model correspondence to each subject is not likely observed under the between-subject distributions (i.e. subject-level choices behaviors do not fall along the diagonal in Figure 3c). We note the PRC-intact monkeys are subjects 1–3, PRC-lesioned monkeys are subjects 4–6.