Age-related differences in prefrontal glutamate are associated with increased working memory decay that gives the appearance of learning deficits
Figures
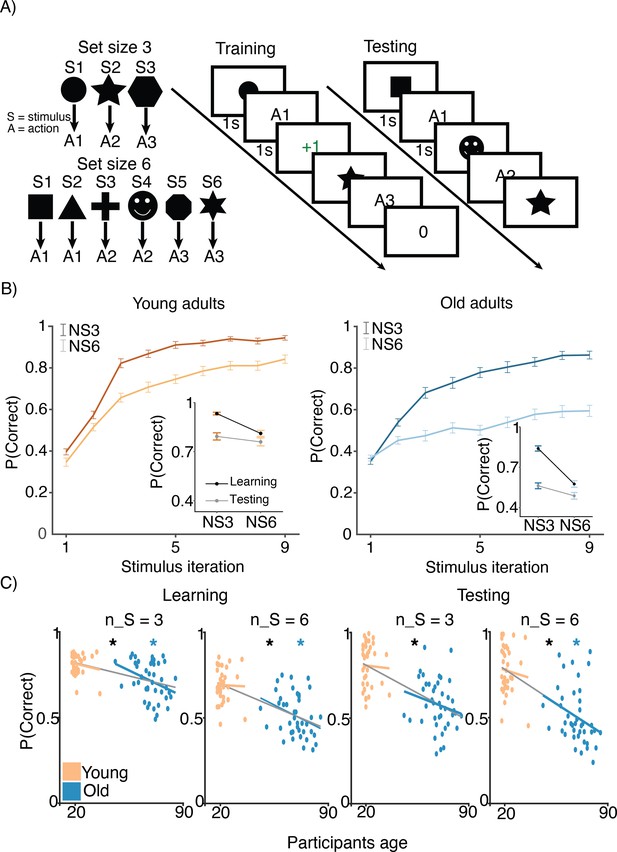
Experimental design.
(A) Reinforcement learning–working memory (RL-WM) task with and blocks. Participants learned three or six stimulus–action associations and received truthful feedback on each trial. In the test phase, participants observed the same images observed during learning and were asked to produce the responses they remembered being correct, without being given the feedback. (B) Learning curves and learning/testing comparison for both set sizes in younger (N=36) and older adults (N=42). Younger adults performed better overall, with smaller difference in performance between the set sizes. The older participants showed greater drop-off in set size 3 between learning and testing compared to young adults. Error bars represent standard error of the mean (SEM). (C) Age spearman correlations with average accuracy in each set size conditions across both learning and testing. All full sample negative correlations between age and performance were significant (gray lines); there were no significant age–performance correlations within young age group (yellow lines). Age and performance were significantly correlated within old group (blue) in all conditions except set size 3 in testing. The correlation significance was evaluated using the p < 0.05 threshold.
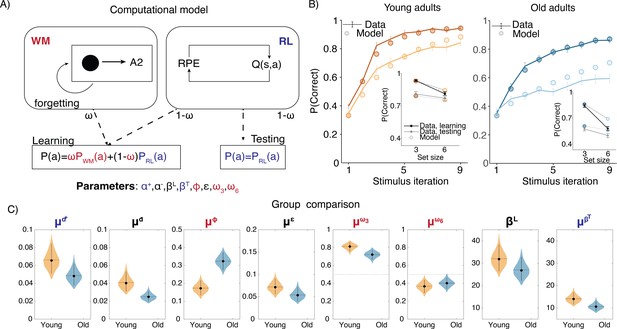
Computational model.
(A) Reinforcement learning–working memory (RL-WM) computational model schematic. (B) Posterior predictive checks for group-level learning curves and asymptotic (last three stimulus iterations) means from our Bayesian RL-WM model. Darker color represents set size 3; lighter color represents set size 6. (C) Comparison of group-level mean parameters shows that primarily WM parameters drive differences between young and older adults, especially the forgetting rate parameter . Dashed lines represent equal contributions of WM and RL processes.
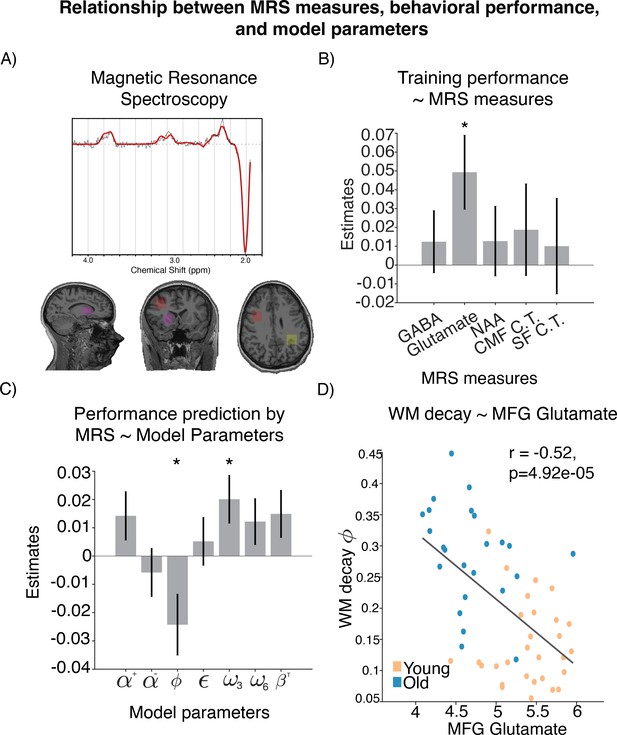
Relationship between magnetic resonance spectroscopy (MRS) measures, behavioral performance, and model parameters.
(A) Location of voxels from which the spectroscopy measures were sampled. (B) Neural measure predictors that provided best out-of-sample prediction of learning performance. (C) Coefficients of modeling brain-predicted performance using all reinforcement learning–working memory (RL-WM) computational model parameters in a linear model (N=53). WM decay and WM weight in set size 3 are the only significant predictors. Errorbars represent standard error of the coefficients. (D) Relationship between WM decay and glutamate levels in middle frontal gyrus (MFG), with Spearman correlation shown for reference.
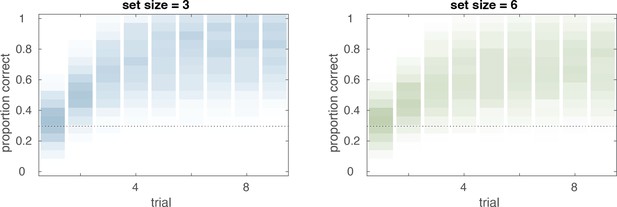
Prior predictive checks.
Prior predictive checks for the hierarchical Bayesian reinforcement learning–working memory (RL-WM) model. The distribution of learning curves in each set size condition that are implied solely by the model specification suggests the model specification is suitable for the RL-WM task data. Group-level learning curves we would never expect to see (e.g. anti-learning) are not given any notable prior weight; while all possible group-level learning curves we could conceivably observe are given some prior predictive weight, and the most likely learning curves are not excessively strongly weighted. Furthermore, it is nice to see that the set size effect is an emergent property of our model specification.
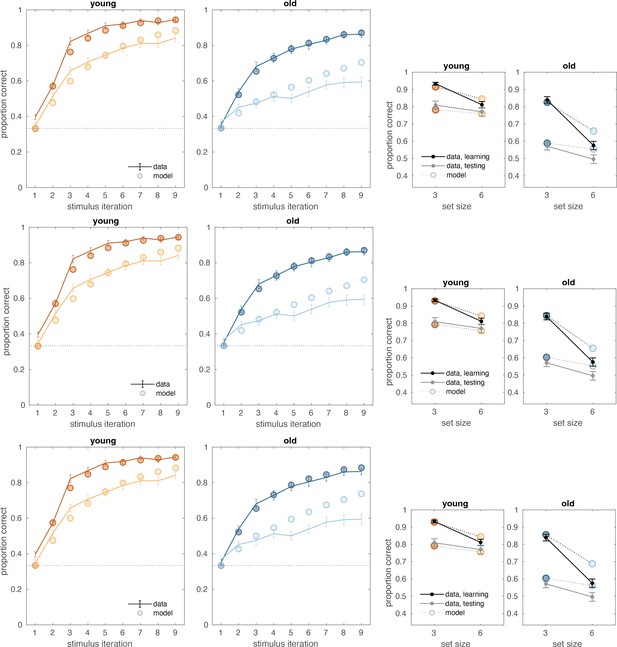
Posterior predictive checks.
Posterior predictive checks for learning curves (left) and asymptotic means (right) for the non-hierarchical (top row), single-group (middle row), and two-group (bottom row) versions of the Bayesian reinforcement learning–working memory (RL-WM) model. (Note that all model-based analyses reported in the main text are based on output from the winning two-group version of the model.)
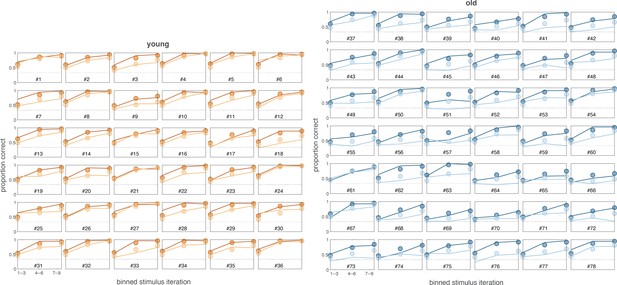
Posterior predictive checks of learning curves for each participant for the two-group version of the Bayesian reinforcement learning–working memory (RL-WM) model.
As a smaller amount of data is available for individual participants, we plot the curve over stimulus iteration bins (of three iterations each). Our Bayesian RL-WM model captures the performance very well for nearly all participants in the young age group and for many participants in the older age group. Some participants in the older age group are not fit as well, such as #63, and a few are severely misfit, such as #51.
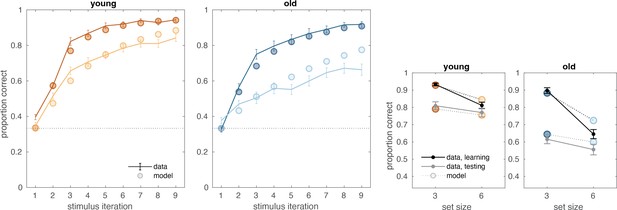
Posterior predictive checks for the two-group version of the Bayesian reinforcement learning–working memory (RL-WM) model when data from 14 participants was excluded.
The criterion for exclusion was that mean accuracy on at least one set size 3 block was at or below chance when missed responses were classed as incorrect (which is only the case for this analysis).
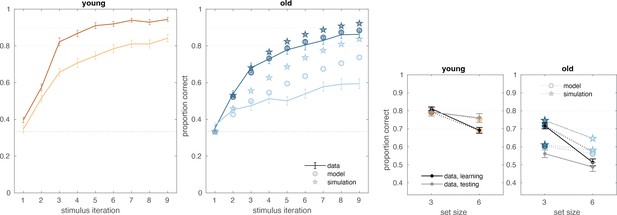
Exploratory demonstration of the role of decay rate in older adults’ performance.
Posterior predictive checks were recomputed after replacing the posterior samples for individual-level decay parameters for all older adults group with the posterior samples for the group-level decay rate of the young adults. While these simulation results are not sufficient as the basis for a quantitative measure or test, examining the difference in the reinforcement learning–working memory (RL-WM) model’s behavioral predictions before (circles) and after (stars) this substitution is revealing: it suggests the lower decay rate might be responsible for most of the difference in behavioral performance between the age groups during the learning phase, particularly for set size 6. Decay rate can recover less of the group difference during the test phase (as the test-phase inverse temperature exerts stronger control in this portion of the RL-WM model). Lighter dotted lines are included to facilitate comparison across groups; the black dotted lines represent chance performance.
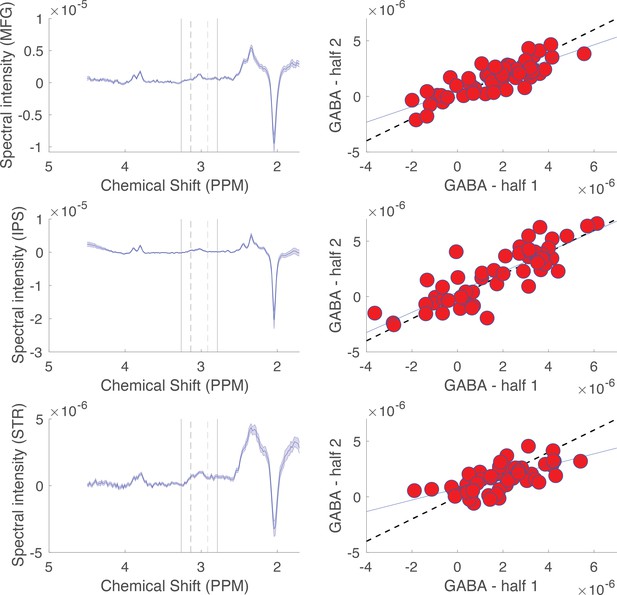
Description and validation of GABA quantification methods.
The GABA peak in megaPRESS difference spectra was quantified by integrating chemical shifts ranging from 2.9 to 3.1 ppm and subtracting out the integrated signal in a surrounding reference window (2.8–2.9, 3.1–3.2 ppm). The reliability of this quantification method in our dataset was tested by splitting megaPRESS data into two halves, the first corresponding to the ‘odd’ difference spectra and the second corresponding to the ‘even’ difference spectra. Split half correlations between peak integrals for the two halves served to measure the reliability of our analysis method. Left: averaged difference spectra for the three brain regions: middle frontal gyrus (MFG, top), intraparietal sulcus (IPS, middle), and striatum (STR, bottom). Dotted vertical lines mark the edges of the integration window, whereas solid vertical lines mark the edges of the reference window. Right: split half correlations for each brain region (top = MFG, middle = IPS, bottom = STR) were relatively high (R = 0.8, 0.8, and 0.68, respectively, for the three regions). GABA quantification on even trials (ordinate) is plotted against GABA quantification on odd trials (abscissa) for each participant (red points). Dotted line reflects the unity line, and solid line reflects a least-squares linear fit.
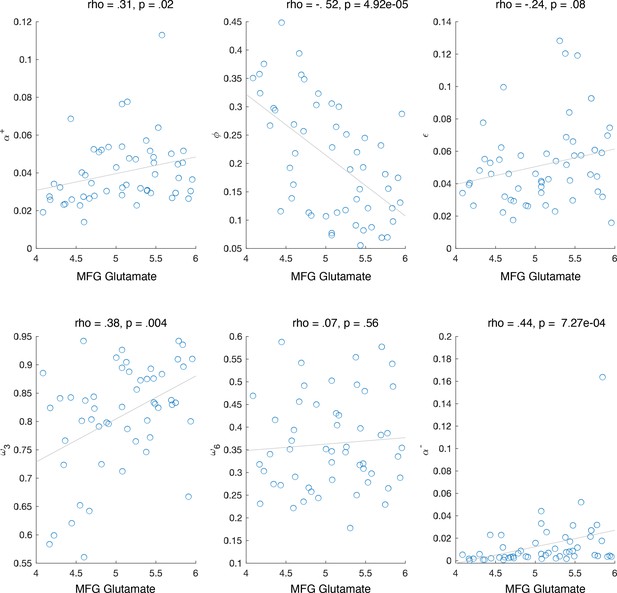
Correlation between middle frontal gyrus (MFG) glutamate and model parameters.
The working memory (WM) parameters and correlated with MFG glutamate; reinforcement learning (RL) parameter also correlated with MFG glutamate, but not when controlling for. Negative learning rate also correlated with MFG glutamate. Note that negative learning rate is included in updating of both RL and WM, and thus cannot be used to make claims about the specificity of WM or RL relationship with MFG glutamate.
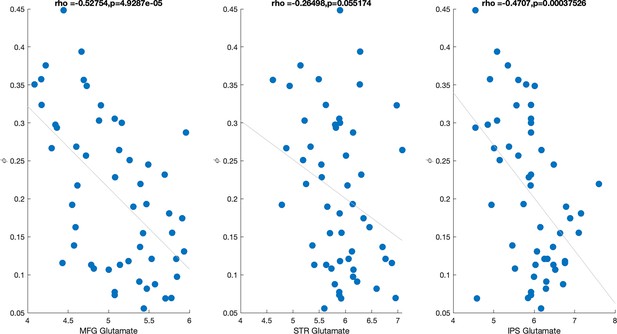
Correlation between working memory (WM) decay parameter () and glutamate sampled from three different brain regions (middle frontal gyrus [MFG], striatum [STR], and intraparietal sulcus [IPS]).
Both MFG and IPS glutamate were significantly correlated with WM decay; when all three measures are entered as predictors of WM decay in the same model only MFG glutamate remains a significant predictor (see text).
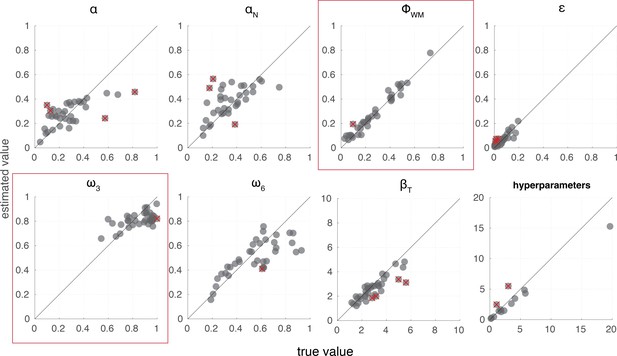
Reinforcement learning–working memory (RL-WM) model parameter recovery.
We ran a recovery study to demonstrate the ability of the model to generate parameter estimates that correspond usefully to known, true parameter values. Data were simulated according to the RL-WM model specification using the same number of blocks, trials, etc., as in our experiment. The number of simulated participants matched the minimum number of participants in either of the two age groups (i.e. 36 simulated participants). Overall, 92.5% of parameters’ credible intervals included the true value; both individual-level parameters and group-level hyperparameters were able to be recovered successfully.
Tables
IPS | MFG | STR | |
---|---|---|---|
GABA | 1.82 | 1.57 | 1.43 |
Glutamate | 4.60 | 3.24 | 1.50 |
NAA | 4.53 | 2.85 | 2.07 |
Gray matter | 12.70 | 20.78 | 28.01 |
White matter | 10.27 | 17.11 | 28.98 |
CMF | SF | RMF | |
Cortical thickness | 3.98 | 4.01 | 5.44 |
GABA | 1.16 |
---|---|
Glutamate | 2.06 |
NAA | 1.76 |
CMF cortical thickness | 2.71 |
SFcortical thickness | 2.37 |